Data Privacy and Security
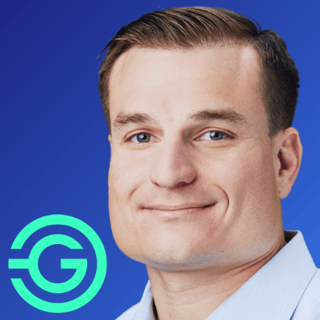
Diego Oppenheimer is a serial entrepreneur, product developer and investor with an extensive background in all things data. Currently, he is a Partner at Factory a venture fund specialized in AI investments as well as a co-founder at Guardrails AI. Previously he was an executive vice president at DataRobot, Founder and CEO at Algorithmia (acquired by DataRobot) and shipped some of Microsoft’s most used data analysis products including Excel, PowerBI and SQL Server.
Diego is active in AI/ML communities as a founding member and strategic advisor for the AI Infrastructure Alliance and MLops.Community and works with leaders to define AI industry standards and best practices. Diego holds a Bachelor's degree in Information Systems and a Masters degree in Business Intelligence and Data Analytics from Carnegie Mellon University.
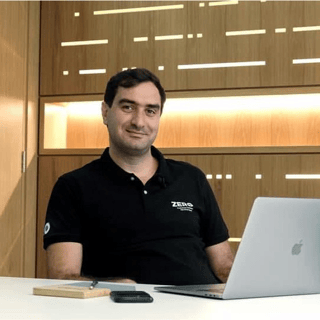
Gevorg Karapetyan is the co-founder and CTO of ZERO Systems where he oversees the company's product and technology strategy. He holds a Ph.D. in Computer Science and is the author of multiple publications, including a US Patent.
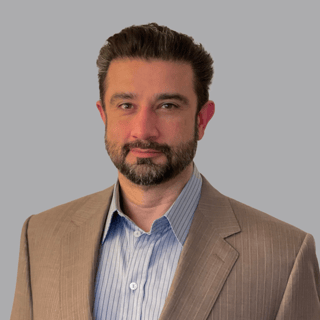
Vin Vashishta is the author of ‘From Data to Profit’ (Wiley), the playbook for monetizing data and AI. He built V-Squared from client 1 to one of the oldest data and AI consulting firms. For the last nine years, he has been recognized as a data and AI thought leader. Vin is a LinkedIn Top Voice and Gartner Ambassador. His background spans over 25 years in strategy, leadership, software engineering, and applied machine learning.
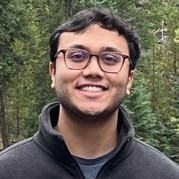
Saahil Jain is an engineering manager at You.com. At You.com, Saahil builds search, ranking, and conversational AI systems. Previously, Saahil was a graduate researcher in the Stanford Machine Learning Group under Professor Andrew Ng, where he researched topics related to deep learning and natural language processing (NLP) in resource-constrained domains like healthcare. Prior to Stanford, Saahil worked as a product manager at Microsoft on Office 365. He received his B.S. and M.S. in Computer Science at Columbia University and Stanford University respectively.
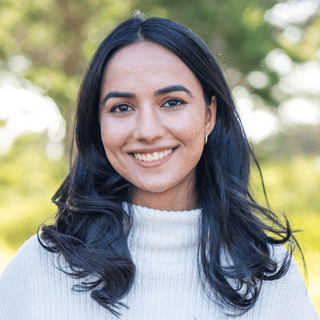
Shreya Rajpal is the creator and maintainer of Guardrails AI, an open-source platform developed to ensure increased safety, reliability, and robustness of large language models in real-world applications. Her expertise spans a decade in the field of machine learning and AI. Most recently, she was the founding engineer at Predibase, where she led the ML infrastructure team. In earlier roles, she was part of the cross-functional ML team within Apple's Special Projects Group and developed computer vision models for autonomous driving perception systems at Drive.ai.
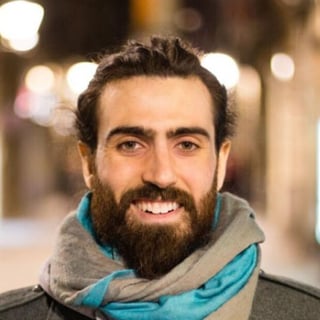
At the moment Demetrios is immersing himself in Machine Learning by interviewing experts from around the world in the weekly MLOps.community meetups. Demetrios is constantly learning and engaging in new activities to get uncomfortable and learn from his mistakes. He tries to bring creativity into every aspect of his life, whether that be analyzing the best paths forward, overcoming obstacles, or building lego houses with his daughter.
This panel discussion is centered around a crucial topic in the tech industry - data privacy and security in the context of large language models and AI systems. The discussion highlights several key themes, such as the significance of trust in AI systems, the potential risks of hallucinations, and the differences between low and high-affordability use cases.
The discussion promises to be thought-provoking and informative, shedding light on the latest developments and concerns in the field. We can expect to gain valuable insights into an issue that is becoming increasingly relevant in our digital world.
I will let Diego take over because he is the moderator of this panel and I will let Diego take over because he is the moderator of this panel and there we go. All right. Uh oh, looks like rea may have had some technical difficulties. So I'll bring her up on when she is ready. In the meantime, Diego get cracking, man. there we go. All right. Uh oh, looks like rea may have had some technical difficulties. So I'll bring her up on when she is ready. In the meantime, Diego get cracking, man. Oh, you're on mute. Oh, you're on mute. Uh Of Uh Of course, I had to do that one, right? I know I'm just calling your um your, your, your example here. Hey, everyone. Uh So really excited about, about kind of leading this panel. So like the core of it is uh you know, as it is in the title data Privacy and security. So, you know, we've talked about course, I had to do that one, right? I know I'm just calling your um your, your, your example here.
Hey, everyone. Uh So really excited about, about kind of leading this panel. So like the core of it is uh you know, as it is in the title data Privacy and security. So, you know, we've talked about some of these uh you know, what does it mean uh to um you know, the, the, the privacy and security in the context of uh large language models. Uh What does it mean to trust these new A I systems? So there's a lot of questions around uh you know, hallucinations and, and we, I I talked a little bit in my, in, in my note around the, you know, low affordability and like high affordability use cases. So some of these uh you know, what does it mean uh to um you know, the, the, the privacy and security in the context of uh large language models. Uh What does it mean to trust these new A I systems? So there's a lot of questions around uh you know, hallucinations and, and we, I I talked a little bit in my, in, in my note around the, you know, low affordability and like high affordability use cases. So without further ado I have some amazing panelists here, uh, who are at the, at the core of this on both.
Uh Is it fair to call the previous generation of a male? It feels like it makes me feel so old. Uh And, uh, but, um, you know, uh, so I'm gonna really quickly start, uh, and let them introduce themselves to and ask you to, uh, without further ado I have some amazing panelists here, uh, who are at the, at the core of this on both. Uh Is it fair to call the previous generation of a male? It feels like it makes me feel so old. Uh And, uh, but, um, you know, uh, so I'm gonna really quickly start, uh, and let them introduce themselves to and ask you to, uh, you know, uh, you know, your name, what do you do? Um And kind of like, give me one little thing about like, you know, in the space, what you've been thinking about. So you've all been thinking about this space a lot. So uh I'm a little nugget uh about it. So, so he, we'll start with you since you're on my top right here. you know, uh, you know, your name, what do you do? Um And kind of like, give me one little thing about like, you know, in the space, what you've been thinking about. So you've all been thinking about this space a lot. So uh I'm a little nugget uh about it. So, so he, we'll start with you since you're on my top right here.
Hey folks. Um Thanks for having us. Um So my name is I am an engineer at U dot com, which is a conversational A I search engine. And one of the topics I've been thinking about a lot is how do we best combine a lot of these advances in generative A I with advances in information retrieval. Hey folks. Um Thanks for having us. Um So my name is I am an engineer at U dot com, which is a conversational A I search engine. And one of the topics I've been thinking about a lot is how do we best combine a lot of these advances in generative A I with advances in information retrieval.
Then Then I'm Vin Vita founder and CEO of V SQUARED. Been in technology for over 25 years. Uh Data science, machine learning for over 11 I do data strategy and A I strategy because, well, they wouldn't let me do any cool projects until I figured out how to get them paid and make people money. So that's now my majority specialty figuring out how to make money with these models. I'm Vin Vita founder and CEO of V SQUARED. Been in technology for over 25 years. Uh Data science, machine learning for over 11 I do data strategy and A I strategy because, well, they wouldn't let me do any cool projects until I figured out how to get them paid and make people money. So that's now my majority specialty figuring out how to make money with these models. What I've been thinking about is what comes after this because these, this is just the beginning and we've got probably a few other evolutions coming afterwards. Things like robotics are going to come to the front too. So that's what I've been thinking about what's next. What I've been thinking about is what comes after this because these, this is just the beginning and we've got probably a few other evolutions coming afterwards. Things like robotics are going to come to the front too. So that's what I've been thinking about what's next.
Right. Right. Hello, I'm, I'm co-founder and CTO at X Systems. Uh I'm leading the both uh product strategy and the technology strategy. Hello, I'm, I'm co-founder and CTO at X Systems. Uh I'm leading the both uh product strategy and the technology strategy. And uh what we do in zero, we develop um compilers for knowledge workers which are uh augmenting them in very like, you know, high value sophisticated tasks and actually what we do, what we think like, you know, uh the future will be in the intersection of uh automation of sophisticated workflows with like uh And uh what we do in zero, we develop um compilers for knowledge workers which are uh augmenting them in very like, you know, high value sophisticated tasks and actually what we do, what we think like, you know, uh the future will be in the intersection of uh automation of sophisticated workflows with like uh uh foundation models. And we are super excited to see uh how the businesses are being transformed with this technology. uh foundation models. And we are super excited to see uh how the businesses are being transformed with this technology. Excellent, Excellent, right?
And finally, uh last but not least trea right? And finally, uh last but not least trea uh hey, everyone very excited to be here. Um My background is also in uh machine learning and a lot of like previous generation of machine learning as, as Diego said. So I've been working in M L since. Uh yeah, I think eight or nine years, everything from M L research. And um uh hey, everyone very excited to be here. Um My background is also in uh machine learning and a lot of like previous generation of machine learning as, as Diego said. So I've been working in M L since. Uh yeah, I think eight or nine years, everything from M L research. And um you know, classically I had decision making under uncertainty, deep learning, um also worked in uh autonomous systems and self driving, doing machine learning and deep learning for uh a few years and then most recently, the founding engineer at an M L startup, also doing machine learning infrastructure, applied M L. you know, classically I had decision making under uncertainty, deep learning, um also worked in uh autonomous systems and self driving, doing machine learning and deep learning for uh a few years and then most recently, the founding engineer at an M L startup, also doing machine learning infrastructure, applied M L. Um I'm a top of mind for me. And I guess also the reason that I'm here um talking on this panel is that I'm the creator of an open source library called Guard rails, which um as you would expect adds guard rails to the output of large language models and makes them a little bit more reliable and safe. Um Yeah, excited to be here. Um I'm a top of mind for me. And I guess also the reason that I'm here um talking on this panel is that I'm the creator of an open source library called Guard rails, which um as you would expect adds guard rails to the output of large language models and makes them a little bit more reliable and safe. Um Yeah, excited to be here.
Great. Awesome. Great. Awesome. Um So let's just start about like kind of like let's high level, like how should we think about uh you know, the difference in some of these data privacy concerns that exist today between like kind of, you know how we were building models and, you know, maybe a couple of years ago and now using some of these kind of foundational model API S like, how do we frame this? Uh Anybody can go, can go ahead like, I mean, I'm trying to think how we should think about the framing here. Um So let's just start about like kind of like let's high level, like how should we think about uh you know, the difference in some of these data privacy concerns that exist today between like kind of, you know how we were building models and, you know, maybe a couple of years ago and now using some of these kind of foundational model API S like, how do we frame this? Uh Anybody can go, can go ahead like, I mean, I'm trying to think how we should think about the framing here. I'm like, I'll just pick on Ben because he's right there in front of me. I'm like, I'll just pick on Ben because he's right there in front of me. It's always me. How should we think about these? I think it's the biggest piece that's missing from the conversation is that we're not thinking about the patterns they can uncover it used to be, we could find fairly simplistic patterns and the more complex machine learning and then eventually deep learning models got the more complex the patterns that could be discovered. It's always me. How should we think about these? I think it's the biggest piece that's missing from the conversation is that we're not thinking about the patterns they can uncover it used to be, we could find fairly simplistic patterns and the more complex machine learning and then eventually deep learning models got the more complex the patterns that could be discovered. And so when we think about data privacy, data security, with respect to these types of models, it's no longer the data itself. It is the patterns within the data that can be uncovered and create vulnerabilities for anyone that has a significant amount of data out in public. There's a study that was done by uh Cole Short at Pepperdine And so when we think about data privacy, data security, with respect to these types of models, it's no longer the data itself. It is the patterns within the data that can be uncovered and create vulnerabilities for anyone that has a significant amount of data out in public. There's a study that was done by uh Cole Short at Pepperdine where they realized they could through some creative prompting, get these models to give a basically craft A V C pitch in the style of different V CS and it was, it was convincing. So there are patterns that are built into the data sets that we throw out there all the time on social media and they allow for more than just our data to be learned. where they realized they could through some creative prompting, get these models to give a basically craft A V C pitch in the style of different V CS and it was, it was convincing. So there are patterns that are built into the data sets that we throw out there all the time on social media and they allow for more than just our data to be learned. There are deeper patterns now and I think we need to start thinking about the implications. There are deeper patterns now and I think we need to start thinking about the implications. So, so how do we think about to kind of continue on that? I'll, I'll ask you like, you know, you're, you're working on information retrieval uh And, and at a large scale, like how do you, how are you thinking about like the framing around these kind of like this patterns? Like when do you use, when do you think you can use these open generic A P, well, generic API S versus kind of like need to bring in models in house? Like how do you frame that? So, so how do we think about to kind of continue on that? I'll, I'll ask you like, you know, you're, you're working on information retrieval uh And, and at a large scale, like how do you, how are you thinking about like the framing around these kind of like this patterns? Like when do you use, when do you think you can use these open generic A P, well, generic API S versus kind of like need to bring in models in house? Like how do you frame that? Yeah. No, I think um that's uh an interesting point around some of these patterns and some vulnerabilities there. I think there's probably might have kind of two ways in which we approach thinking about some of these issues. So, on one hand, um a lot of these models hallucinate, so they'll make up content. I think we're all aware of that. Um So in some ways, you know, there's obviously a lot of technical work to be done on reducing hallucination that grounding essentially a lot of these models. So that's one area. But then on the other side, Yeah. No, I think um that's uh an interesting point around some of these patterns and some vulnerabilities there. I think there's probably might have kind of two ways in which we approach thinking about some of these issues. So, on one hand, um a lot of these models hallucinate, so they'll make up content. I think we're all aware of that. Um So in some ways, you know, there's obviously a lot of technical work to be done on reducing hallucination that grounding essentially a lot of these models. So that's one area. But then on the other side, um just because something is hallucinating doesn't mean that it's not a useful tool for people. So I think it also is somewhat of a product question. So how do we make sure that people have the right expectations when they're using a product that in most large language model, they can get the most out of it. So I think when we're thinking a lot about this type of stuff, those are the two angles in which I think a lot about it is, it's not only a technical question but also a product question around, you know, what are the right expectations that, you know, we present to people using these tools? But how do we make sure that they're not gonna be misled um just because something is hallucinating doesn't mean that it's not a useful tool for people. So I think it also is somewhat of a product question. So how do we make sure that people have the right expectations when they're using a product that in most large language model, they can get the most out of it. So I think when we're thinking a lot about this type of stuff, those are the two angles in which I think a lot about it is, it's not only a technical question but also a product question around, you know, what are the right expectations that, you know, we present to people using these tools? But how do we make sure that they're not gonna be misled and they know how to responsibility is it? and they know how to responsibility is it? That's, that's we have a great, great point in terms of like how to use it. And so I'm, I'm gonna, I'm gonna move to and kind of like maybe for the audience kind of frame some of the challenges around hallucinations and kind of like how you need to think about it. And then obviously, you know, tell us a little bit about your work in terms of That's, that's we have a great, great point in terms of like how to use it. And so I'm, I'm gonna, I'm gonna move to and kind of like maybe for the audience kind of frame some of the challenges around hallucinations and kind of like how you need to think about it. And then obviously, you know, tell us a little bit about your work in terms of uh what's your hypothesis here? Like, you know, you obviously built this like fairly popular uh open source project that's having a lot of adoption. So uh very curious to hear from you, uh you know, kind of like how you, how you frame it in, in this context. uh what's your hypothesis here? Like, you know, you obviously built this like fairly popular uh open source project that's having a lot of adoption. So uh very curious to hear from you, uh you know, kind of like how you, how you frame it in, in this context. Yeah. Yeah, I think hallucination is a very um interesting problem. Like when people think about hallucination, it's actually, you know, like a combination of problems that all kind of get grouped under this umbrella term of hallucination. Yeah. Yeah, I think hallucination is a very um interesting problem. Like when people think about hallucination, it's actually, you know, like a combination of problems that all kind of get grouped under this umbrella term of hallucination. Uh I think like some of those problems are basically falsehoods, et cetera, even like you have multiple conflicting sources and you aren't able to trust like which one um you know, is, is the golden um is the golden source that you should kind of base your answer on. So it's a bunch of like kind of complex problems going on here. Um I think like grounding honestly um is is the way to go is the way to kind of solve all of these uh solve, you know, very domain specific Uh I think like some of those problems are basically falsehoods, et cetera, even like you have multiple conflicting sources and you aren't able to trust like which one um you know, is, is the golden um is the golden source that you should kind of base your answer on. So it's a bunch of like kind of complex problems going on here. Um I think like grounding honestly um is is the way to go is the way to kind of solve all of these uh solve, you know, very domain specific um hallucination problem. So I do think that um trading better models, training bigger models, you know, that are kind of like um uh primed to uh you know, be less susceptible to this is like one way to go about this. But at the end of the day, you know, as, as all of us have worked with them, uh we kind of know that it's really hard to kind of get that level of certainty with any machine learning model. And so being able to take something that is so powerful, um hallucination problem. So I do think that um trading better models, training bigger models, you know, that are kind of like um uh primed to uh you know, be less susceptible to this is like one way to go about this. But at the end of the day, you know, as, as all of us have worked with them, uh we kind of know that it's really hard to kind of get that level of certainty with any machine learning model. And so being able to take something that is so powerful, um and, you know, then add constraints on top of that, that make it work really well for your specific use case, I think is, you know, um more, just more like more tractable essentially as a problem to solve rather than just make hallucination go away. Um You know, as, as a blanket thing for L MS. Um And so one of those things I, I, I, I believe people have touched on this before as well, but like it is the way to go is um um and, you know, then add constraints on top of that, that make it work really well for your specific use case, I think is, you know, um more, just more like more tractable essentially as a problem to solve rather than just make hallucination go away. Um You know, as, as a blanket thing for L MS. Um And so one of those things I, I, I, I believe people have touched on this before as well, but like it is the way to go is um you can essentially connect what you believe are like good data sources or good kind of like fact checking. Um um you know, either agents or tools or um um or, or even just like um embeddings of um you know, good data sources with your L M outputs. Um And then any kind of like L M outputs that are generated would get, you know, validated against those like ground rules. So that's kind of like the guard rails way to do it, which is that you can essentially connect what you believe are like good data sources or good kind of like fact checking. Um um you know, either agents or tools or um um or, or even just like um embeddings of um you know, good data sources with your L M outputs. Um And then any kind of like L M outputs that are generated would get, you know, validated against those like ground rules. So that's kind of like the guard rails way to do it, which is that um you use your large language model to um generate something that, you know, functions. And then on top of that um for your domain you think about like what are my constraints? Um and then impose them, you know, using like external sources or um um yeah, or, or, or external data connections. Um um you use your large language model to um generate something that, you know, functions. And then on top of that um for your domain you think about like what are my constraints? Um and then impose them, you know, using like external sources or um um yeah, or, or, or external data connections. Um It's give, I I'm, I'm gonna move over to give over here on this one. So you, you know, you, you, you work in a very applicable space that you're building for information workers inside enterprises, kind of like these assisted like accuracy matters, trust matters, security and privacy matters. Like as you lead, like how do you think like, you know, kind of what are, what are some of the that you're thinking about? Uh you know, today as you develop the product, obviously don't reveal anything proprietary or uh you know, a trade secret. But I'm very curious like It's give, I I'm, I'm gonna move over to give over here on this one. So you, you know, you, you, you work in a very applicable space that you're building for information workers inside enterprises, kind of like these assisted like accuracy matters, trust matters, security and privacy matters. Like as you lead, like how do you think like, you know, kind of what are, what are some of the that you're thinking about? Uh you know, today as you develop the product, obviously don't reveal anything proprietary or uh you know, a trade secret. But I'm very curious like how you're thinking about it because it has to be relevant. I mean, you're trying to get the trust of these enterprises to not only connect their data but also like use your system inside their workflows, like walk us through that. how you're thinking about it because it has to be relevant. I mean, you're trying to get the trust of these enterprises to not only connect their data but also like use your system inside their workflows, like walk us through that. Absolutely. So Absolutely. So currently the enterprises already see and believe in the power of the generative uh A I the power of the large language models. So it is like very close to them, like they can play with that, the GP T for all, for their like, you know, personal use cases. currently the enterprises already see and believe in the power of the generative uh A I the power of the large language models. So it is like very close to them, like they can play with that, the GP T for all, for their like, you know, personal use cases. But when it comes to the enterprise use cases and we are working with for large enterprises such as Fortune 500 companies, the largest law firms in the world. So their data is very confidential and it's actually their clients data and they have like, you know, contractual obligations about how they're going to govern that data. And of course, like, you know, now there's like, you know, there's a huge like, you know, chasm between the opportunities and the reality But when it comes to the enterprise use cases and we are working with for large enterprises such as Fortune 500 companies, the largest law firms in the world. So their data is very confidential and it's actually their clients data and they have like, you know, contractual obligations about how they're going to govern that data. And of course, like, you know, now there's like, you know, there's a huge like, you know, chasm between the opportunities and the reality and uh what we are doing, um we strongly suggest not to use the API S like pity for the confidential data. Uh And for those cases, just bring A I inside the organizations because nowadays, there are already a lot of great models for like, you know, several billion parameters. You can bring inside the security perimeter of the enterprise, fine tune on, on their domain specific data, and uh what we are doing, um we strongly suggest not to use the API S like pity for the confidential data. Uh And for those cases, just bring A I inside the organizations because nowadays, there are already a lot of great models for like, you know, several billion parameters. You can bring inside the security perimeter of the enterprise, fine tune on, on their domain specific data, just give a product, it right on the human feedback and reach the level of the quality when the users will trust your system. So uh Diego, you, you are absolutely right to trust for enterprise systems is absolutely must, in case they will lose the confidence in, in your product, you'll just ignore that just give a product, it right on the human feedback and reach the level of the quality when the users will trust your system. So uh Diego, you, you are absolutely right to trust for enterprise systems is absolutely must, in case they will lose the confidence in, in your product, you'll just ignore that totally ab absolutely. And so, you know, moving forward and kind of like the trust. So I, I, you know, I think we have to frame one of the things I'm always kind of curious about. I've been thinking about like there seems to be this um totally ab absolutely. And so, you know, moving forward and kind of like the trust. So I, I, you know, I think we have to frame one of the things I'm always kind of curious about. I've been thinking about like there seems to be this um people applying the, the, the, you know, kind of like the use cases to a one size fits all, like, framework and like, you know, like, and, and, and, and it's problematic. Right, because there's times that, like, you shouldn't care. Right. Go use an API get whatever's faster, cheaper, whatever gets you there. And there's other times that you should spend the time to bring it all, you know, to your point, bring all the A I into the enterprise, people applying the, the, the, you know, kind of like the use cases to a one size fits all, like, framework and like, you know, like, and, and, and, and it's problematic. Right, because there's times that, like, you shouldn't care. Right. Go use an API get whatever's faster, cheaper, whatever gets you there. And there's other times that you should spend the time to bring it all, you know, to your point, bring all the A I into the enterprise, but that has a cost, right? It's, it, it can be expensive, you need to have knowledge and stuff like that. And so being able to do the back and forth based on like how you're thinking about security and privacy and not applying everything with, uh you know, a one size fits all I think is really important. I want to kind of push a little bit on the, um you know, like, but that has a cost, right? It's, it, it can be expensive, you need to have knowledge and stuff like that. And so being able to do the back and forth based on like how you're thinking about security and privacy and not applying everything with, uh you know, a one size fits all I think is really important. I want to kind of push a little bit on the, um you know, like, what have you seen in the industry in terms of people? Like, let's talk about that framing, right? Like how to think about the use cases, how to frame how to, you know, if I'm listening to this talk right now and I'm trying to bring some of these use cases into my organization. Like, how should I frame it right? In terms of thinking about it, how should I break down the problem? Should I go in route A? Should I go on route B? Like who can help me kind of like guide the kind of like questions that I should be asking myself in terms of like where to run this and how to run it. what have you seen in the industry in terms of people? Like, let's talk about that framing, right? Like how to think about the use cases, how to frame how to, you know, if I'm listening to this talk right now and I'm trying to bring some of these use cases into my organization. Like, how should I frame it right? In terms of thinking about it, how should I break down the problem? Should I go in route A? Should I go on route B? Like who can help me kind of like guide the kind of like questions that I should be asking myself in terms of like where to run this and how to run it. Any of you can jump in. Any of you can jump in. Yeah. So like one thing like, you know, when you are currently thinking about the use cases, at first, you need to understand that currently you need to put every single truth that you have in the future like under the question because you had some understanding like what is solvable, what is not solvable right now, everything changed, you need to come to your business and understand Yeah. So like one thing like, you know, when you are currently thinking about the use cases, at first, you need to understand that currently you need to put every single truth that you have in the future like under the question because you had some understanding like what is solvable, what is not solvable right now, everything changed, you need to come to your business and understand which are like, you know, the, the major K P I s uh uh that will contribute to the like, you know, growth of the your business. Where, where, where are you, you know, that your obstacles, you know, where the protest are sophisticated. You are spending a lot of like, you know, money with uh uh like, you know, less input and understand if you can like automate that and bringing the people who understand that. And they say, oh, you know, with new technology, it is possible. which are like, you know, the, the major K P I s uh uh that will contribute to the like, you know, growth of the your business. Where, where, where are you, you know, that your obstacles, you know, where the protest are sophisticated. You are spending a lot of like, you know, money with uh uh like, you know, less input and understand if you can like automate that and bringing the people who understand that. And they say, oh, you know, with new technology, it is possible. Got it. Got it. Then you work on data strategy a lot. How are you, you know, if I came to you today and I need, I said, I need some advice on how to think about this. Can you frame it for me? Then you work on data strategy a lot. How are you, you know, if I came to you today and I need, I said, I need some advice on how to think about this. Can you frame it for me? Yeah, definitely. What I tell companies is I tell them this is kind of an arc. There's a whole bunch of use cases that you could have, but they haven't been proven yet. And if you're not meta, don't be first Yeah, definitely. What I tell companies is I tell them this is kind of an arc. There's a whole bunch of use cases that you could have, but they haven't been proven yet. And if you're not meta, don't be first because you don't have the capabilities, you don't have the background. You don't really have the domain expertise in this area to be the pioneer for a particular use case. Wait until someone else proves it out and then because you don't have the capabilities, you don't have the background. You don't really have the domain expertise in this area to be the pioneer for a particular use case. Wait until someone else proves it out and then enter in. But that doesn't mean you have to wait till your competitors get into the market. You can look at each use case as a category. You look at the ability to service customers and there's so much that you can do there. But you also at the same time have to be protecting your customers, which is not something most companies are thinking about. What happens when their customers submit a query across your website. enter in. But that doesn't mean you have to wait till your competitors get into the market. You can look at each use case as a category. You look at the ability to service customers and there's so much that you can do there. But you also at the same time have to be protecting your customers, which is not something most companies are thinking about. What happens when their customers submit a query across your website. Where does that go? How is it stored? What are the privacy implications that you're not thinking about? And that's why I say a lot of times you don't want to be the first company to do this because you don't have that in-house capability to really at an expert level, think about these things. But at the same time, what you should be doing is some really targeted opportunity, discovery Where does that go? How is it stored? What are the privacy implications that you're not thinking about? And that's why I say a lot of times you don't want to be the first company to do this because you don't have that in-house capability to really at an expert level, think about these things. But at the same time, what you should be doing is some really targeted opportunity, discovery thinking about different categories of capabilities and problems that you've seen solved in other industries. And then asking yourself how could I apply that problem solution thinking about different categories of capabilities and problems that you've seen solved in other industries. And then asking yourself how could I apply that problem solution and fit it into something that I have in my current business. Internal use cases are always great for proof of concepts because you're banging at it inside and the risks are significantly lower than turning around. And you see, you know, companies like Google did this, Microsoft did this, and fit it into something that I have in my current business. Internal use cases are always great for proof of concepts because you're banging at it inside and the risks are significantly lower than turning around. And you see, you know, companies like Google did this, Microsoft did this, they consumed it internally and then they turned around and let companies externally play with it. And so a lot of these paradigms are what I'm talking to clients about now, but it's really important to be thinking through use cases and connecting to value propositions. Not, well, it sounds cool. So let's do it. No, no. Start with the R O I. If there's not a significant R O I, you know, if there's not a big dollar value on the other side of this, they consumed it internally and then they turned around and let companies externally play with it. And so a lot of these paradigms are what I'm talking to clients about now, but it's really important to be thinking through use cases and connecting to value propositions. Not, well, it sounds cool. So let's do it. No, no. Start with the R O I. If there's not a significant R O I, you know, if there's not a big dollar value on the other side of this, why distract yourself from your core strategy and your current competitive advantages? You have to adopt this at some point. So you should be forward looking and prescriptive. But at the same time, it's all about the returns. If there's no significant use case that fits, that will deliver cash, don't do it. why distract yourself from your core strategy and your current competitive advantages? You have to adopt this at some point. So you should be forward looking and prescriptive. But at the same time, it's all about the returns. If there's no significant use case that fits, that will deliver cash, don't do it. Yeah. Yeah. Yeah, I actually um I was gonna, I was gonna ask Saw Hill about his. So, you know, you work in search, you work in the future of search, right? And retrieval and personalization. And I'm kind of curious like how you think about Yeah, I actually um I was gonna, I was gonna ask Saw Hill about his. So, you know, you work in search, you work in the future of search, right? And retrieval and personalization. And I'm kind of curious like how you think about you let go, you know, like, you know, if one argues, not only company, but our personal data is kind of like the most valuable thing we have, right? But we obviously want to contribute that data to search experiences that are great for us. Uh How do we think about like, you know, what, what's the future look like? Can you guide us through that? Like uh in terms of like how you maybe you let go, you know, like, you know, if one argues, not only company, but our personal data is kind of like the most valuable thing we have, right? But we obviously want to contribute that data to search experiences that are great for us. Uh How do we think about like, you know, what, what's the future look like? Can you guide us through that? Like uh in terms of like how you maybe how you're thinking about it at you or maybe how you're just thinking about it personally, we can detach those two things if you want. Uh But I'm very curious, uh you know what the, what the future of personalized search looks for, look, looks, look looks like. how you're thinking about it at you or maybe how you're just thinking about it personally, we can detach those two things if you want. Uh But I'm very curious, uh you know what the, what the future of personalized search looks for, look, looks, look looks like. Yeah, I think it's honestly, I think it looks super exciting. But um yeah, I think the future of search is probably Yeah, I think it's honestly, I think it looks super exciting. But um yeah, I think the future of search is probably more exciting now than I think it's been in, you know, the last last couple of years, last five years maybe. Um And I think there's a number of reasons why I think a lot of it is obviously the recent advances in, you know, natural language processing, et cetera. Um I think when it comes to personalized search, um I think it comes down to, you know, how like I guess when, when we think about, if we're thinking a little bit more into the future, more exciting now than I think it's been in, you know, the last last couple of years, last five years maybe. Um And I think there's a number of reasons why I think a lot of it is obviously the recent advances in, you know, natural language processing, et cetera. Um I think when it comes to personalized search, um I think it comes down to, you know, how like I guess when, when we think about, if we're thinking a little bit more into the future, um you know, what can we do to really allow you to control your search experience? So I think that's something that we have a lot more ability to control. I think a lot of times now when we're interacting with some of these models, for example, just think of the concept of a system message. um you know, what can we do to really allow you to control your search experience? So I think that's something that we have a lot more ability to control. I think a lot of times now when we're interacting with some of these models, for example, just think of the concept of a system message. So when you're using, you know, one of these models, um you know, there's this idea where you can specify a system message and it will basically, you know, dictate how the model is able to adapt to that. So that's that, that in itself is a, a degree of personalization of A I that we have not been able to do in the past. Um So I think ideas like that basically will allow us to really personalize the results that we get from search in the future if we kind of apply that more broadly. So when you're using, you know, one of these models, um you know, there's this idea where you can specify a system message and it will basically, you know, dictate how the model is able to adapt to that. So that's that, that in itself is a, a degree of personalization of A I that we have not been able to do in the past. Um So I think ideas like that basically will allow us to really personalize the results that we get from search in the future if we kind of apply that more broadly. And then also in the, in terms of bringing your own data, I think there's gonna be a lot of, we have a lot of ideas at least where we're thinking about, you know, building an open platform where other people can contribute their data and essentially what we call apps and to search and we'll incorporate that into our chat slash results. So I think there's, you know, a combination of open community efforts that can make you more personalized um as well as um personalization enabled by technology itself. And then also in the, in terms of bringing your own data, I think there's gonna be a lot of, we have a lot of ideas at least where we're thinking about, you know, building an open platform where other people can contribute their data and essentially what we call apps and to search and we'll incorporate that into our chat slash results. So I think there's, you know, a combination of open community efforts that can make you more personalized um as well as um personalization enabled by technology itself. Cool. So um we're gonna go do uh we're gonna have to wrap this up even though I could probably talk about this uh you know, all day. So I'm gonna do one quick uh you know, rotation through everybody. And I'm gonna ask you to either recommend a tool, it's totally fine to recommend the tool that you're working on a uh you know, recommend uh a resource or kind of like give a thought to the audience to go chase in terms of like how to be thinking about data privacy and security and trust in these A I systems. So, Cool. So um we're gonna go do uh we're gonna have to wrap this up even though I could probably talk about this uh you know, all day. So I'm gonna do one quick uh you know, rotation through everybody. And I'm gonna ask you to either recommend a tool, it's totally fine to recommend the tool that you're working on a uh you know, recommend uh a resource or kind of like give a thought to the audience to go chase in terms of like how to be thinking about data privacy and security and trust in these A I systems. So, so I'll start with you uh and uh go for and go and go that way. so I'll start with you uh and uh go for and go and go that way. Yeah, I guess maybe I would start, I guess one thought would be um maybe just, you know, what you currently find um these tools to be very useful to you right now where, you know, I think sometimes it's easy to think of tools as useful um in abstract or maybe on a couple of examples. But what are some ways in which you've been using some of these technologies and it's been consistently useful for you? Um And then, you know, from there, I think one can imagine what the future gifts will be Yeah, I guess maybe I would start, I guess one thought would be um maybe just, you know, what you currently find um these tools to be very useful to you right now where, you know, I think sometimes it's easy to think of tools as useful um in abstract or maybe on a couple of examples. But what are some ways in which you've been using some of these technologies and it's been consistently useful for you? Um And then, you know, from there, I think one can imagine what the future gifts will be awesome. Give work. awesome. Give work. Yeah, Yeah, it's a great question. So since we have like a very uh uh like, you know, a big audience here, so, and I, I believe that not everyone uh started to like, you know, work and experiment with like, you know, uh generative A I and like, you know, bring the applications via link chain. So I strongly suggest uh to do that because you will be very impressed how fast you can have a, a great result. it's a great question. So since we have like a very uh uh like, you know, a big audience here, so, and I, I believe that not everyone uh started to like, you know, work and experiment with like, you know, uh generative A I and like, you know, bring the applications via link chain. So I strongly suggest uh to do that because you will be very impressed how fast you can have a, a great result. And then you already would have a good use case. You can already like, you know, double down on the accuracy at advance, like, you know, user experience. And then you already would have a good use case. You can already like, you know, double down on the accuracy at advance, like, you know, user experience. Great Great Vin Vin uh I would start looking at auto GP T the whole concept of self healing self correcting. Those are some really fascinating use cases, there's danger, but a lot of potential in that direction. So I would say, look at those tools if there's anything that comes out of this, that I think becomes an exceptionally powerful construct going forward, that's the one to keep an eye on from a forward looking perspective. uh I would start looking at auto GP T the whole concept of self healing self correcting. Those are some really fascinating use cases, there's danger, but a lot of potential in that direction. So I would say, look at those tools if there's anything that comes out of this, that I think becomes an exceptionally powerful construct going forward, that's the one to keep an eye on from a forward looking perspective. Got it. Got it. And, and I, you know, I, I, I expect what you're gonna, you know, which, what, what tool are you gonna expect it to push here? So do it uh totally happy with that. But And, and I, you know, I, I, I expect what you're gonna, you know, which, what, what tool are you gonna expect it to push here? So do it uh totally happy with that. But yeah, I, yeah, yeah, having to do that. So I can uh I think I'm going to basically talk about guard rails and uh I'm going to talk about it more in the context of like this idea that I want the audience to kind of like, think about and like a take home, you know. Um um uh Yeah, some take home inspiration and like, why guard rails kind of fits inside that. Um So I think essentially um yeah, I, yeah, yeah, having to do that. So I can uh I think I'm going to basically talk about guard rails and uh I'm going to talk about it more in the context of like this idea that I want the audience to kind of like, think about and like a take home, you know. Um um uh Yeah, some take home inspiration and like, why guard rails kind of fits inside that. Um So I think essentially um we like these tools are, these uh these models are really performant, right? And we see like really interesting use cases and everything, but like, are they ready to be deployed into production where they can, you know, like work reliably work like 100% of the time and not, you know, um return like awful messages to your potential users, et cetera. Right? And so I think the idea I kind of want to share is that this isn't going to be um the, this isn't sufficient enough to like actually put a lot of, you know, the uh applications that we're building into production and the actual solution would be a hybrid of, you know, more traditional we like these tools are, these uh these models are really performant, right? And we see like really interesting use cases and everything, but like, are they ready to be deployed into production where they can, you know, like work reliably work like 100% of the time and not, you know, um return like awful messages to your potential users, et cetera. Right? And so I think the idea I kind of want to share is that this isn't going to be um the, this isn't sufficient enough to like actually put a lot of, you know, the uh applications that we're building into production and the actual solution would be a hybrid of, you know, more traditional machine learning, uh more traditional like rule based and heuristic based methods in addition to like large language models. And I think like that kind of gives us both the performance as well as the kind of reliability safety guarantees we care about. And I think that's a very powerful construct of like ensemble, those two kinds of methods together. And so in that context, I think like guardrails is a good tool uh that I want to push. Uh uh obviously I'm biased, but I think it's a great tool that, you know, allows you to kind of like get a lot of those um a lot of those guarantees straight out of the box. Yeah. machine learning, uh more traditional like rule based and heuristic based methods in addition to like large language models. And I think like that kind of gives us both the performance as well as the kind of reliability safety guarantees we care about. And I think that's a very powerful construct of like ensemble, those two kinds of methods together. And so in that context, I think like guardrails is a good tool uh that I want to push. Uh uh obviously I'm biased, but I think it's a great tool that, you know, allows you to kind of like get a lot of those um a lot of those guarantees straight out of the box. Yeah. Awesome. Well, hey, thanks all of you for, Awesome. Well, hey, thanks all of you for, for uh a great panel. Uh I believe we're uh we're, we're, we're here wrapping up uh Vin uh Sahi Andrea. Thank you so much for taking the time.