Data Quality: Preventing, Diagnosing & Curing Bad Data // Shailvi Wakhlu // DE4AI
speaker
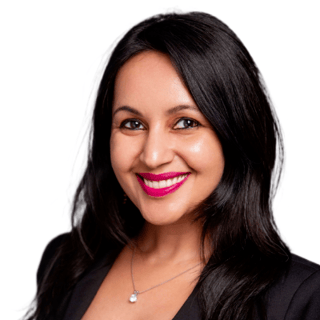
Shailvi is a seasoned Data Leader with over seventeen years of experience growing impactful teams and building technology products used by hundreds of millions of users. Her career includes notable technology roles at Salesforce, Fitbit, and as the Head of Data at Strava. As a fractional executive, she has consulted, advised, and invested with multiple high-growth startups. Shailvi has spoken at nearly 100 global conferences, coached more than 500 individuals, and authored the best-selling book "Self-Advocacy."
SUMMARY
Uncover the secrets to harnessing quality data for amplifying business success. This talk equips you with invaluable strategies and proven frameworks to navigate the data lifecycle confidently. Learn to spot and eradicate low-quality data, fortify decision-making, and build trust with data. With streamlined prevention strategies and hands-on diagnostics, optimize efficiency and elevate your company's data-driven initiatives.
TRANSCRIPT
Skylar [00:00:07]: Next up, we can bring up our next speaker. Welcome. Thank you so much for spending some time with us. I'm super excited to learn everything you have to share about data quality.
Shailvi Wakhlu [00:00:20]: Awesome.
Skylar [00:00:21]: Go ahead and bring your screen up and.
Shailvi Wakhlu [00:00:25]: All right, just confirming that you're able to see the screen.
Skylar [00:00:29]: Yes, I am able to see it.
Shailvi Wakhlu [00:00:30]: Okay, perfect. I will get started. All right. Hello, everyone. Welcome to my session. I hope you're all really pumped about data quality. I'm always very excited when conferences care about this topic. Anything with data, I think it's super important.
Shailvi Wakhlu [00:00:47]: So today we're going to be talking about active ways to prevent diagnosis and cure bad data. My name is Shalvi Bakhlu. I go by the she, her pronouns. And let's kick it off. So we have a few quick topics for today. We'll examine what is unfit or bad data, why anybody should care about it, and finally, what we can do about it. And before we get into all of that, want to set the table stakes of what is bad data even mean? Anything that is, any data that is inaccurate, incomplete, or misleading in any way is referred to as bad data. Some people also call it low quality or unfit data.
Shailvi Wakhlu [00:01:30]: And the problem with it is that it eventually leads to some sort of biased decisions, which is what we want to avoid here. I always also make it clear that just because data is showing you something that you don't want to see, that is not what makes it bad. If the data is accurate, if it is complete, if it's not misleading people in any way, it is not bad data. So why should anybody care about this? And this is something that I think a lot of people, especially in data roles, want to figure out how to best position it with their stakeholders, with their executive, the C suite. How can you convince other people, besides people who experience data quality problems, to care about bad data? So the most important reason is cost data. When it is low quality, it is a burden on your resources to try to maintain it. You're constantly trying to guess, like, is this right? Is this not? Because when you have historically had a lot of bad data, there develops almost this mistrust of accuracy. And so you end up as a team wasting a lot of effort on identifying what the issue is.
Shailvi Wakhlu [00:02:44]: There's a lot of time and money that's spent trying to reconcile information and all the extra like time, resources, tools that you have to spend on fixing issues is ultimately a cost through a business. There is also sort of a more, you know, there's the upfront cost that you have to fix it. But you can also lose opportunity or directly lose revenue because you have data quality issues. So something simple like, you know, you were using, you was using, you were using an automated pricing model. And if that is incorrect and your, your product is not priced the way it's supposed to be, like, that can, that can lead to revenue loss, or you can also just have something like the data tells you to do something, and that is not what you should have done. You should have done. You should have picked option a instead of option b. That is an opportunity lost where you could have made more money, and that is a problem that you didn't make it.
Shailvi Wakhlu [00:03:43]: Losing trust. So when you have data quality issues, especially if they're consistent and they kind of show up, you lose trust. And that's not just a monetary cost. Like if your reputation is damaged, then people don't trust your results. And this can be internal, that you have internal stakeholders who don't believe, you know, the data is telling them something that they don't want to hear and they blame it on quality issues. But you could also have it external, that maybe your customers are inconvenienced. They don't, they have seen data quality issues in the past. So when you as a, as a product, tell your customer base to do something, maybe they're not, maybe they're not convinced and just reassured.
Shailvi Wakhlu [00:04:25]: Like your competition is always going to take advantage of the fact that you have data quality issues internally, people may not trust your work, they may not trust your competence. So whether it's internal or external, your brand can be affected by that. In some industries, and I've worked, like, I myself have worked in healthcare and a lot of industries where there is a lot of compliance and regulation. So there is a legal liability for data quality issues. Again, in some industries, there's very standard rules, there's very standard guidelines, and there are monetary and other consequences if you are not compliant. And compliance can show up if your data quality is messed up. But even in other cases, even if you're not in a regulated industry, if your data quality ends up showing up to the consumer in a way that causes harm, you are like, as an individual or as a company, you can be held liable. So there are a lot of legal standards here.
Shailvi Wakhlu [00:05:29]: We're going to talk about this a little bit later. But the cost of bias and how that relates to ethical behavior, did you know that your data quality could have caused harm in some way? All of that are potentials to think about. And yeah, the bias part is, I think there can be a talk just on this topic, like how bias in data can lead to harm. Real people can be affected by data quality issues that are essentially incorporating some hidden biases. It might be unintentional, but there is a cost. You could have some unanticipated scenarios where maybe you made a decision on the, on your data architecture. Maybe it really didn't meet the moment of what it was supposed to do. And there's a, there's a, there's a domino effect of problems that happen downstream.
Shailvi Wakhlu [00:06:30]: You know, you're excluding some individuals from the benefit of a product or causing some very, very direct harm. So this especially, like, I think, I think, I think rightfully so. The industry cares about things like this. And there is a cost that if you have biased data decisions, if they lead to harmful consequences, it is costly. And causing harm is never, never a good sales switch for your product productivity. So this is, again, more of an internal, internal cost. So it's just time consuming. When you don't have clear processes for thinking about data quality and tackling data quality, then you're going to keep fixing the problem.
Shailvi Wakhlu [00:07:13]: So anytime it shows up, you're going to fix it. And that can take more time. Like, it can take more time to keep fixing data quality issues than to just go upstream and have better processes that prevent data quality issues from occurring in the first place. So anybody, data scientists, like, their job is typically, they find the error, they communicate it, they hunt for the source, they validate, cross check. All of that takes a lot of time, and it is not the best use of your resources. And that dovetails into sort of the final piece of it, which is just morale. You know, I truly believe that. I think data folks or any, anybody in any profession, you thrive when your skills are effectively utilized.
Shailvi Wakhlu [00:08:03]: If you feel you are just doing very low sort of low skill work in some way, you know, as in like, there are, there are more important things that you could be doing. And it's just like, okay, I don't want to be fixing sort of something that can be a larger agreement within the company that, you know, we want to prioritize this. You want to put the tools and the processes and the responsibilities behind it. Instead, if you feel that it just ends up being my problem because nobody else will take care of it, that is something that leads to disillusionment and it leads to loss of talent. So these are hopefully some, some good reasons why companies should care. And of course, I think, you know, data folks care about data quality issues, but it's something rango bell. I hope that's helpful. Okay, so moving on to sort of the more tactical, tactical pieces.
Shailvi Wakhlu [00:08:59]: What can you actually do about, about low data quality? And if you're disciplined, this is almost a plan. Like, what can you do before, what can you do during, and what can you sort of do downstream? So think of it in that sense that when we think about bad data quality, the first thing that we should think about is how do you actually prevent it in the first place? Prevention is usually like, it's hard, but it's something that can be planned for. So in this, I think the first stage that I'd like to set is to actually look at the lifecycle of data, because I think it's much easier to figure out how to prevent something when you understand how it kind of flows through, flows through the sequences. Anyways, so data is typically the first stage is definition. We define features, and we aligned different teams on those definitions. And at this space, like product and data, teams might be working together on that alignment, like, what is even a piece of data? Next, you log it, so you track it, you store it, and it goes through some sort of an engineering process. After that is when you transform it. So you are applying business rules to that data.
Shailvi Wakhlu [00:10:24]: You are pre processing the data. You are transforming it into something that is actually useful. Next up, you are analyzing it so you can model the data, you can interpret it to solve various, various problems. And finally, you will share out the results with stakeholders. And again, there can be many different ways of sharing it. Whether it's a dashboard or a model that predicts something, all of that is part of that sharing piece. So that wraps up the data lifecycle. And if you think about it at every stage, you can, you can have, you can have situations that occur that introduce those data quality issues.
Shailvi Wakhlu [00:11:04]: So bad data during the definition definition phase, you know, you can have something like an uneven feature definition. An example is, you know, I still like to use a lot of healthcare examples, but, like, if you're trying to say that, okay, this disease is what I'm trying to track, like, when you define the disease, one person can say, this is a very broad definition. Anybody who tests positive for this disease has that disease. Or you can have something else, like having the disease could just be that, okay, you have these disease markers, and that implies that you have that disease. If you have that uneven definition, that some people think this is the definition versus another, that leads to problems downstream, you could also have a very myopic definition, like, you know, a very narrow definition that the COVID example that people initially said you have Covid, if you have the alpha variant and then the delta variants and flirt variants, I've lost track where we are at with that thing. But you could keep having other things that represent that same initial thing that you were trying to track and, you know, but your original definition was too narrow. And finally you could just have incorrect input parameters, like maybe you made a typo and you misspelled something. So the definition is not reflecting what you truly wanted to track.
Shailvi Wakhlu [00:12:35]: The next stage in the data lifecycle is the logging stage. So this is where you actually track the features that you have defined. So there's a lot of potential for confusion and inaccuracy at this stage because it is possible that you are, you think you are tracking everything, but you have something broken or something incorrect, that you completely missed out, that some people might be, some people might be, the data could be coming from this other pipeline that you're not even thinking about. So you think you are logging all the data you need, but there is some piece of it that is just missing or incomplete. The other piece could be like a faulty pipeline. So, you know, you are, you have every intention of tracking, tracking all the data, but then some part of it broke. You know, data coming from mobile is not being tracked any longer or something, or something like that. And then there is, you know, inconsistent timeframes.
Shailvi Wakhlu [00:13:38]: So when you are thinking about data, thinking about how long that data is being stored, when does it get aggregated, what time zones are used? I think this is a problem that is pretty common that you are. For example, some people are aggregating it based on day, and there is a difference in the time zone expectations from who's doing that logging versus who's viewing it. This is the equivalent of there being a broken connection between the data that you want and the data that you are actually tracking. And as you can imagine, it ends up leading to a lot of unintended consequences. The next phase is the transforming phase. So this is where you are trying to pre process your log data into a usable format with the rules. And when you talk about rules, the first thing is, are the rules even understood? You know, does everybody, does everybody think of the rules in the same way? Have you labeled data in a way that is unambiguous? I think this is a place where I highly recommend the use of data dictionaries and more intentional documentation of what's going on with the data, because I think it really helps to just remove some of the assumptions that people might make when they are looking at something. Another common thing is just meaningless aggregation.
Shailvi Wakhlu [00:15:08]: Different people think about logic and algorithms in a different way. And some people, they jump a few steps. So maybe you start with this clean table and then you're trying to get to that endpoint. But the aggregations you make along the way of sort of the step by step pre processing, maybe they're not, maybe they're not the same sort of assumptions that people would have to make. So the situation that results is that if you spot an issue, you're trying to go sort of back up every step to figure out where things broke. And it's unnecessary. Like, you have to do a lot of data gymnastics with raw data. I think this is why DBT became popular, because you could kind of see the lineage more clearly.
Shailvi Wakhlu [00:15:56]: Like, what's going on? What did it start with? What happened over time. But otherwise, that's why in the transforming phase, a lot of things can go wrong. And finally, logical errors, like, you know, your rules were created in a test environment, maybe they didn't include real world scenarios. So it is similar. You know, the analogy that I use is I can give people the exact same raw ingredients. Like, I can give them flour, egg, butter, and everybody can take that and turn it into a different finish product. And the reason is because everybody's using a different recipe, everybody's making different sort of, like using different sort of pieces. So that's why, like, that transformation, if you want it to be predictable, if you want it to be consistent, you have to very clearly define and agree on those rules.
Shailvi Wakhlu [00:16:46]: The next phase is the analyzing phase. So this is where, you know, you really try to just make sure that everybody's trying to answer the same question. You know, you can say, like, something as innocuous as, how many users do we have? Do you mean how many users do we have in our universe? Or do you mean how many users do we have who are active? Or do you mean how many users do we have who are currently, like, still want to be associated with our platform? So, you know, like, arguing out those tiny word, even word like wording differences can go a long way in making sure that the problem is something that everybody is aligned with. This is, you know, my favorite part in some way. But when you have, like, humans make errors, you know, you could, you could even if you try to automate as many things as possible, there are still, like, you could use the wrong ML model or technique or there's a mistake in your formula. So there's a lot of things that can, that can sort of go wrong. And the final piece, again, biased algorithms, is something that we can talk about it endlessly. But how you gathered your data, what you chose to include in your data, what is related to your insights, what training data did you use, all of those things can really make a difference in the final insights that you get from your thing.
Shailvi Wakhlu [00:18:23]: And mistakes in this part can lead, again, to severe data quality issues that are just, again, they're inaccurate, they're incomplete, or they are misleading in some way. And the final phase is the sharing phase. So here, this is like an actual graphic that I found. I love finding bad charts. It's a hobby of mine, so I think someone has shared this with me. But, you know, this is a bad chart. I think everybody can agree that it's not great. And so, but even outside of that, like, even if you don't have a glaringly bad chart, that doesn't even add up to a whole pie.
Shailvi Wakhlu [00:19:06]: Faulty reporting is something that, you know, it, again, it can happen very innocently, that you had a dashboard, you stopped maintaining it actively, and then someone went and tried to get information and thought it was the truth. So that is, you know, it's not, it's not intentional, but, like, there can be faulty reporting, which is, which is, you know, that it's the information that is wrong or there can be information that is unintentional. Also, you know, you, you can't. People, people often make assumptions for what they're seeing. So when, when you have some, when you have information out there, if people don't interpret the results correctly, you know, for example, like, they look at this chart and they don't realize that it's overlapping. Like, you know, somebody could have two different worries. That's a, that's a bad thing because you, you don't, you want to really work very hard to prevent people from misinterpreting results. And you can't do that all the time.
Shailvi Wakhlu [00:20:08]: But focusing on the sharing phase, giving it, like, how will different people read it? I think it allows you to prevent some of those, some, those issues and the final piece that can happen. And again, you know, this is not meant to be an exhaustive list, but the minute you put information out there, the minute you share it, you can't stop people from using it for something that it was not intended for. The only thing that you can do is you can give that nuance upfront, that this is what the data is good for, this is what it's not good for. Please do not use it for this other thing. So having those documentation and caveats outlined upfront, I think help prevent some unintended downstream use, which eventually causes a data quality issue that you don't really have control over. So I'll pause here for just a second because I know some people like to take a snapshot of this graphic. So like I said, these are nothing. This is not an exhaustive list of every possible way something during your data lifecycle can cause a data quality issue, but it is a starting point.
Shailvi Wakhlu [00:21:20]: So I think some people like to look at it as a checklist and at least go through some very common scenarios. But feel free to take a snapshot if you like. All right, next up is actually diagnosing. So hopefully, once you understand how bad data gets created, it's a little easier to diagnose what exactly is going on. And here I start with, how do people even notice that there is bad data? I think a very common way is that people notice results that don't match. Maybe they have a source of truth or something they trust a lot. And then some other data shows up and it doesn't match either in absolute or in aggregation. Or you see something that you consider suspicious.
Shailvi Wakhlu [00:22:10]: You see something and you're like, this data does not make sense. It doesn't match what I logically thought would happen. Sometimes maybe it is actually correct, but it is still a prompt for investigating what's going on. Either way, the first step is to look for obvious reasons. Why do you think you are encountering or suspecting bad data? The first question to ask is, is it actually bad data? You know, if you were trying to match two numbers, were they supposed to match or were they always meant to represent two different things? And the suspicious results, like what assumptions did you make which now cause you to be suspicious? Was the data set built for your use case? So this we discussed before that there is unintended downstream uses that people can come up with. So, you know, is it just a matter of you looking for the right answer but in the wrong place? And finally, is there a known bug or a pipeline issue that can explain the data quality issues that you're seeing? Like, you know, did something happen in your pipeline that you have already documented? So if none of the obvious answers, if none of the obvious reasons are the answer, then it's time to sort of go, go through the data cycle but in reverse order. So, you know, for each stage, verify, like does the phase before it have the right data? And that helps you pinpoint, like where our problem is occurring. And then when you identify the phase, think about like, okay, like, what are the hypothesis of what might be happening? And then for each of those, like, just, you know, create that hypothesis test, validate, and keep repeating that process until you find something that explains what your issue is.
Shailvi Wakhlu [00:23:55]: Here's a sanity checklist so people will, you know, some of you will recognize that this is the journalistic standard of documenting a story. I find it very useful because you, when you, when you start going through the what, where, when, why, who, how of the problem, you start documenting some of the assumptions. And if you do this as a combined exercise, you might actually find through that discussion that, oh, there is a disconnect between some things that you thought were true, which are not. So this is a good way to figure out diagnostic that problem. All right, so now we are onto a final sort of piece curing, like strengthening data quality here. I always say that data quality is a cross functional effort. It is not just the data team that has to focus on it. You know, hopefully you can use some of the things I outlined in the beginning as reason for why everybody should get involved, because the answers are not just in the data team.
Shailvi Wakhlu [00:24:59]: Some of the assumptions may be product assumptions or marketing assumptions or design assumptions. And I think it is helpful when you have a bunch of people coming together to fix the issues. I list out a few comments, scripts like, you know, just being able to compare and data across sources, being able to quickly identify which data is missing or duplicate. And my favorite, like comparing data trends by dimensions, I think it's a way to sort of quickly identify what is going wrong with your, with your situation. And the final piece is just some coding practices. I always am a big fan of reusable modules and making sure you have documentation, especially if you have remote teams. You want to, you want to make sure that everybody can access what you intend. And if you add alerts to your pipelines like now, there's a lot of automated tools that do that for you.
Shailvi Wakhlu [00:25:54]: But if you add alerts, at least for the important stuff, you will be able to identify issues early. And yeah, prevention is better than cure. So maintain stuff, reconcile things, automate where you can simplify everything. And governance, you know, cannot stress it enough that it is super important to think about this. And the final thing is like, have an actual plan to audit, measure your data quality issues, you know, hit me up later if you want ideas on how to do that. And with that, I think that's, that's my time. So, yeah, thank you all so much for attending the session if I think I'm at time. So I don't know if I have time for questions.
Shailvi Wakhlu [00:26:37]: But you can connect.
Skylar [00:26:39]: We have a few minutes.
Shailvi Wakhlu [00:26:40]: Okay, cool. Cool. Yeah, but yeah, otherwise people can connect with me later as well if, if you don't get to the questions.
Skylar [00:26:47]: Cool. So one of the questions that popped up in the chat during us, Rohan asked how can continuous validation and testing be implemented to ensure data quality is maintained during real time processing?
Shailvi Wakhlu [00:27:01]: Yeah, it's interesting because I think when you are continuously validating, I think it helps to sort of again, go back to the basics of like, you know, where is data quality issue popping up that you have noticed? I think keeping anchored on that, that, like, you know, if I'm expecting that this is the part that's, that's going to break, then you can, then you can sort of like create a process specifically in that zone that helps you, that helps you catch it early, that helps you sort of like fix it early as you, as you, as you sort of go along. So I think there's like specific techniques for like each part of like, you know, the validation phase and things like that that you can use. But like, again, I would always encourage people to go back to the why, like what can go wrong and how do you do it?
Skylar [00:27:53]: Awesome. Thank you so much. I think we're at time now, but really appreciate it. We all learned a lot. Thanks.
Shailvi Wakhlu [00:28:00]: Thank you.
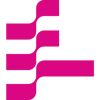