MLOps Critiques Recap
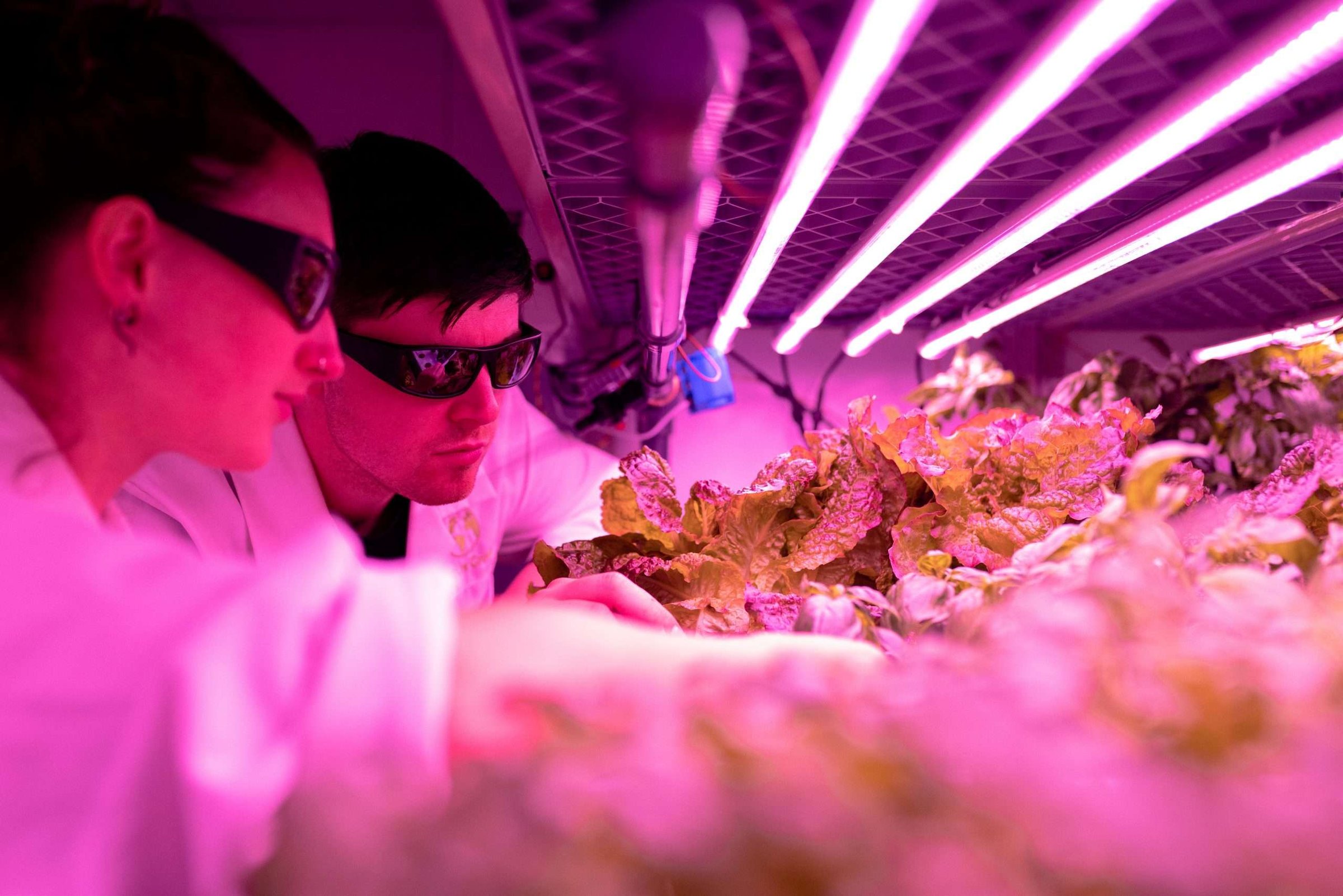
MLOps Community Coffee Session #100 Takeaways: MLOps Critiques “ML is only such a small part of the picture and there is so much software around it…And for some reason, all the MLOps monitoring tools forget that
June 14, 2022
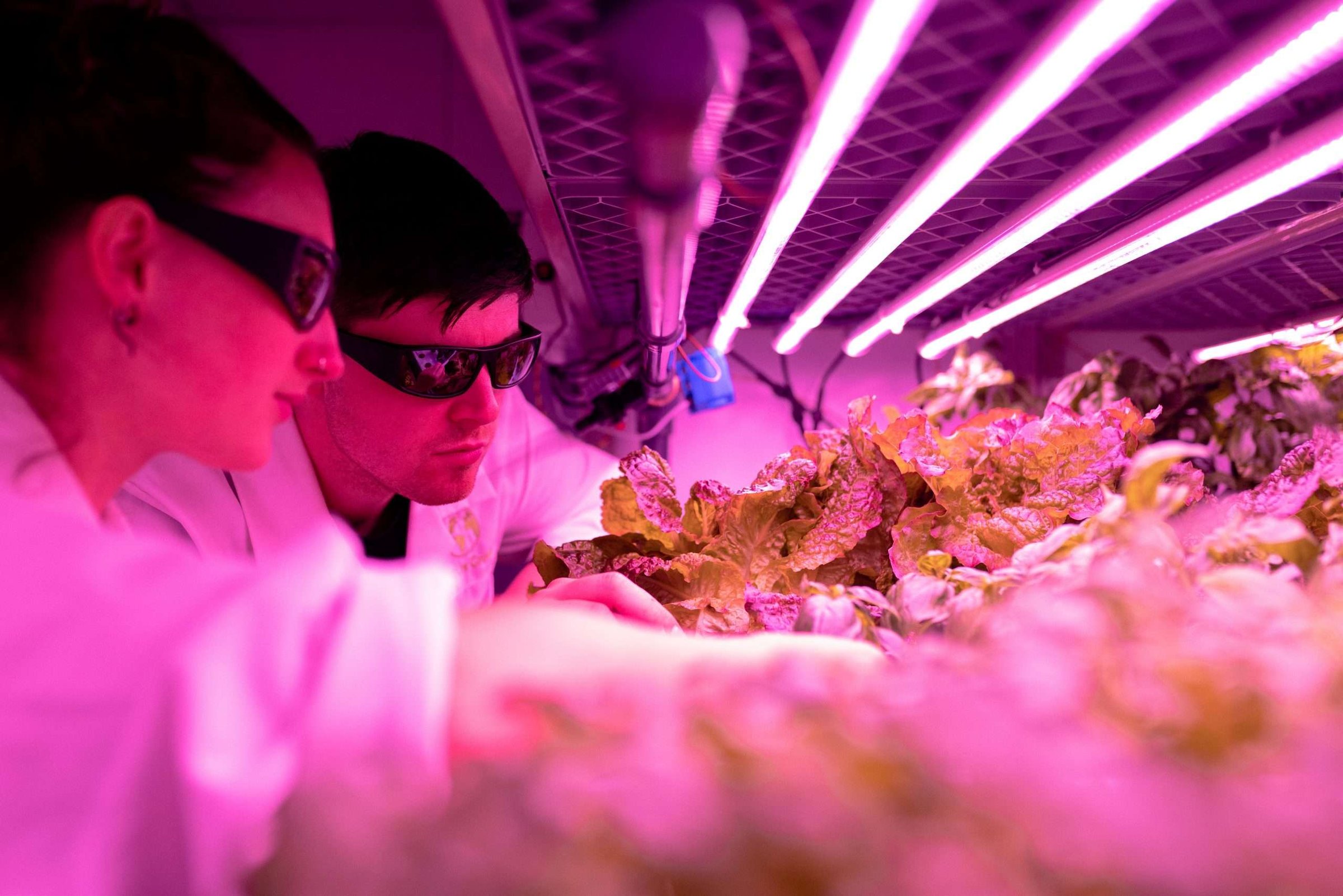
MLOps Community Coffee Session #100 Takeaways: MLOps Critiques
“ML is only such a small part of the picture and there is so much software around it…And for some reason, all the MLOps monitoring tools forget that. They forget that this software stack around it exists and also needs to be monitored. And if there is something I don’t want is monitoring in different tools. That seems horrendous!” – Matthijs Brouns
TL;DR
Working in the ML consultancy and training field got Matthijs Brouns first-hand experience with a multitude of use cases as well as a plethora of MLOps tools. He sat with us to share his thoughts on productionizing ML in a low-risk manner, building your MLOps stack without reinventing the wheel, and keeping vendor lock-in away for good. Ultimately nobody wants a million moving parts or tools, so simplicity and clear value are key for a successful ML pipeline.
Matthijs’ Day-to-Day
Matthijs Brouns is a Machine Learning Engineer and currently, he acts as a CTO at Xccelerated.io which is a training/consulting firm. Matthijs divides his time between building new training materials and exercises for MLEs, giving live training sessions, and acting as a sparring partner for the Xccelerated at their partner firms, as well as doing some consulting work on the side.[06:35]
Matthijs spent a fair amount of time contributing to their open scientific computing ecosystem through various means. He maintains open source packages (scikit-lego, seers) as well as co-chairs the PyData Amsterdam conference and meet-up.
What’s Hot Right Now?
When it comes to skills that are hot on the market some of the top picks are neural net-based things at medical companies, Kafka, Spark, deployment and orchestration tooling. [08:24]
“How do we get rid of barriers to getting things into production today or tomorrow?”
Low-Risk Releases
One of the biggest barriers Matthijs sees there is not being able to do this safely and reliably. There is some tooling here and there, but he has not found anything good enough that offers enough flexibility, so in most cases, you end up building your own tool. [13:20] The market is wide open for creating such tools.
On Picking MLOps Tooling
Matthijs is one of the many ML practitioners who agrees that you don’t want to build everything yourself when it comes to tooling but is also not a fan of vendor lock-in. When he evaluates tools one of the core things he measures is if this is a core component of his product and how easy it is to migrate away in case it no longer serves him down the road. This is one of the reasons he opts for libraries, instead of frameworks.
Most MLOps tools are not too mature and become bloatware very fast because they try to solve too many problems at once. This makes it a headache to extend and maintain as well as see their value clearly.
For example, when it comes to the monitoring space, it seems like most providers forget that ML is only a small part of the product and this software stack around it exists and also needs to be monitored. Monitoring each part of your product in a different tool is a concept no engineer would find pleasing. [24:02]
Technology Recommendations For a Chaotic Space
As we all know the MLOps space is far from offering standardized solutions yet, but the only piece that Matthijs sees is close to this concept is Kubernetes which is well adopted amongst his customers. Usually, when Matthijs gives recommendations on tooling to his clients he doesn’t think about the highest payoff but rather goes for what will cause the least regret down the line. [30:25]
Big barriers in production
Productionizing ML is still a struggle, and even before getting to deployment, you have barriers such as the regular need for manual validation steps like people checking the output, going through the code and making sure it works, as well as checking in with stakeholders that might be unavailable for long periods. From then on, common challenges are lack of process, no way to rollback if something breaks, and missing communication interfaces between different team members as well as between whole teams. [35:52]
Good Automation vs Bad Automation
When developing something for a customer, the time to leave and hand over the project always comes. Matthijs and his team found a cheat way to leave the client in a good position by leaving some of the people working on the project behind permanently.
In general, his advice would be to try to automate the software as much as possible before handing it over. Still, you always need to be mindful that in some cases too much automation might become a pain. Make sure you always sit with the stakeholders and see what they want to know before leaving them.[37:57]
Listen to the episode on Apple Podcasts, Spotify, Anchor, Listen notes, or you can watch the interview on YouTube here.