Collections
All Collections
All Content
Popular topics
# LLMs
# LLM in Production
# AI
# LLM
# Machine Learning
# Rungalileo.io
# MLops
# MLOps
# RAG
# Interview
# Machine learning
# Generative AI
# Tecton.ai
# Arize.com
# AI Agents
# mckinsey.com/quantumblack
# Redis.io
# Zilliz.com
# Humanloop.com
# Snorkel.ai
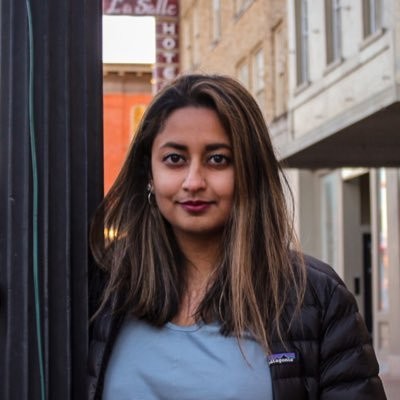


Shreya Shankar, Willem Pienaar & Demetrios Brinkmann · Jun 13th, 2025
Willem Pienaar and Shreya Shankar discuss the challenge of evaluating agents in production where "ground truth" is ambiguous and subjective user feedback isn't enough to improve performance.
The discussion breaks down the three "gulfs" of human-AI interaction—Specification, Generalization, and Comprehension—and their impact on agent success.
Willem and Shreya cover the necessity of moving the human "out of the loop" for feedback, creating faster learning cycles through implicit signals rather than direct, manual review.
The conversation details practical evaluation techniques, including analyzing task failures with heat maps and the trade-offs of using simulated environments for testing.
Willem and Shreya address the reality of a "performance ceiling" for AI and the importance of categorizing problems your agent can, can learn to, or will likely never be able to solve.
# Production failure
# AI system
# Observability


Jukka Remes & Demetrios Brinkmann · Jun 10th, 2025
AI is already complex—adding the need for deep engineering expertise to use MLOps tools only makes it harder, especially for SMEs and research teams with limited resources. Yet, good MLOps is essential for managing experiments, sharing GPU compute, tracking models, and meeting AI regulations.
While cloud providers offer MLOps tools, many organizations need flexible, open-source setups that work anywhere—from laptops to supercomputers. Shared setups can boost collaboration, productivity, and compute efficiency.
In this session, Jukka introduces an open-source MLOps platform from Silo AI, now packaged for easy deployment across environments. With Git-based workflows and CI/CD automation, users can focus on building models while the platform handles the MLOps.
# Open Source Platforms
# AI Act Regulation
# Haaga-Helia UAS


Michael Del Balso & Demetrios Brinkmann · Jun 6th, 2025
Tecton Founder and CEO Mike Del Balso talks about what ML/AI use cases are core components generating Millions in revenue. Demetrios and Mike go through the maturity curve that predictive Machine Learning use cases have gone through over the past 5 years, and why a feature store is a primary component of an ML stack.
# AI Adoption
# LLMs
# Tecton



+1
Prateek Chhikara, Arthur Coleman, Nehil Jain & 1 more speaker · Jun 5th, 2025
Memory is one of the thorniest challenges in deploying LLMs. Mem0 introduces a scalable long-term memory architecture that dynamically extracts, consolidates, and retrieves key information from conversations. By using graph-based structures to model relationships between conversational elements, Mem0 enables AI agents that are more accurate, coherent, and production-ready.
# MEM0
# LLMs
# OpenAI memory


Raza Habib & Demetrios Brinkmann · Jun 3rd, 2025
Raza Habib, the CEO of LLM Eval platform Humanloop, talks to us about how to make your AI products more accurate and reliable by shortening the feedback loop of your evals. Quickly iterating on prompts and testing what works, along with some of his favorite Dario from Anthropic AI Quotes.
# Generative AI
# LLMs
# Humanloop

Han Lee · Jun 3rd, 2025
An analysis of the May 2025 incident where xAI's Grok chatbot began inappropriately referencing 'white genocide' in South Africa. This post-mortem delves into the probable cause—a flawed post-processing prompt—framing it as a critical MLOps failure. It underscores the necessity of treating prompts as key artifacts, implementing progressive deployment strategies, and using appropriate metrics for AI safety and reliability.
# LLMs
# Prompt Deployment
# White Genocide


Vaibhav Gupta & Demetrios Brinkmann · May 30th, 2025
It's been two years, and we still seem to see AI disproportionately more in demos than production features. Why? And how can we apply engineering practices we've all learned in the past decades to our advantage here?
# Programming Language
# LLM
# BAML


Prithviraj Ammanabrolu & Demetrios Brinkmann · May 27th, 2025
Prithviraj Ammanabrolu drops by to break down Tao fine-tuning—a clever way to train models without labeled data. Using reinforcement learning and synthetic data, Tao teaches models to evaluate and improve themselves. Raj explains how this works, where it shines (think small models punching above their weight), and why it could be a game-changer for efficient deployment.
# Fine Tuning
# Synthetic Data
# Databricks

Médéric Hurier · May 27th, 2025
This article provides a quick guide for building and deploying a Retrieval Augmented Generation (RAG) application in minutes, perfect for hackathon environments. It details how to leverage Google Cloud's Vertex AI Studio and Vertex AI Search to create a grounded Large Language Model (LLM) application that can answer questions based on a specific knowledge base, such as company documentation.
The process involves preparing data in Google Cloud Storage, creating a searchable data store with Vertex AI Search, crafting and grounding a prompt in Vertex AI Studio, and then deploying the AI assistant as a web app using Cloud Run. The guide emphasizes speed and automation, allowing users to focus on data and user experience rather than complex technical setups.
# Data Science
# Machine Learning
# Generative AI Tools
# Artificial Intelligence
# Retrieval Augmented Generation System


Mohan Atreya & Demetrios Brinkmann · May 23rd, 2025
Demetrios and Mohan Atreya break down the GPU madness behind AI — from supply headaches and sky-high prices to the rise of nimble GPU clouds trying to outsmart the giants. They cover power-hungry hardware, failed experiments, and how new cloud models are shaking things up with smarter provisioning, tokenized access, and a whole lotta hustle. It's a wild ride through the guts of AI infrastructure — fun, fast, and full of sparks!
# GPUs
# AI infrastructure
# Rafay