MLOps is maturing, and here’s the evidence
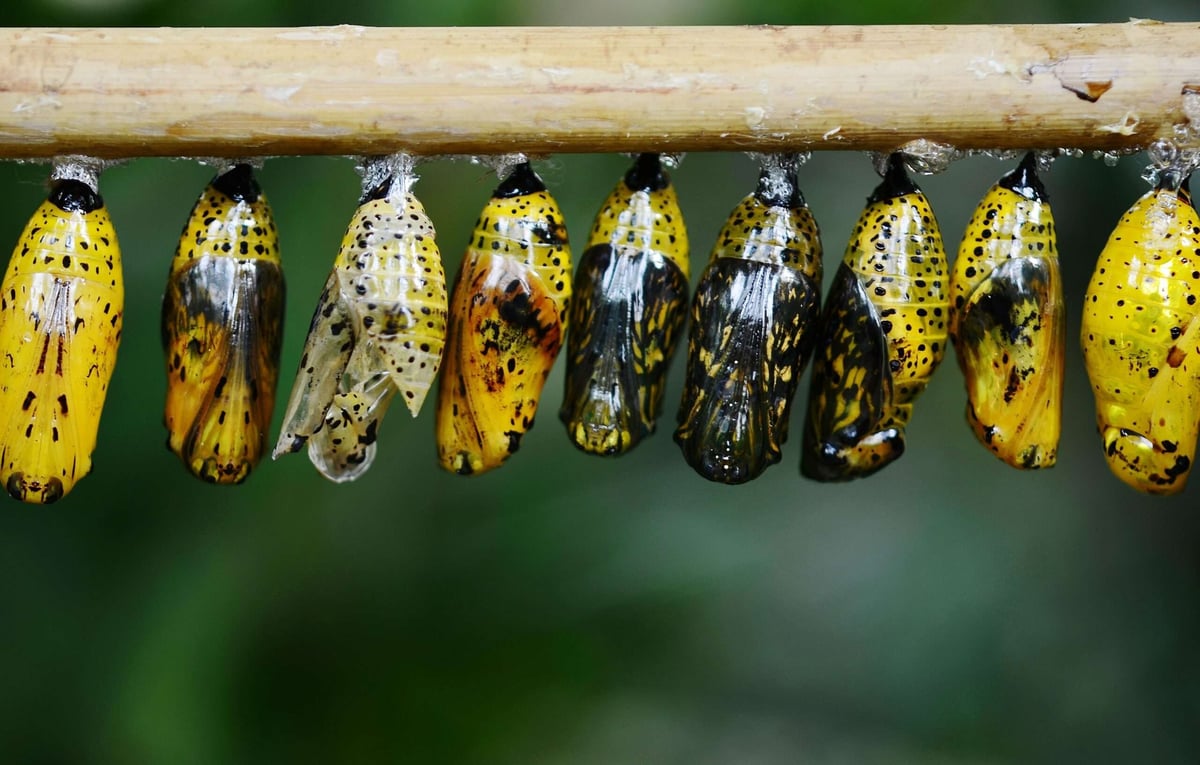
Back in machine learning’s early days, every project was a trial run and many didn’t see daylight
June 21, 2021

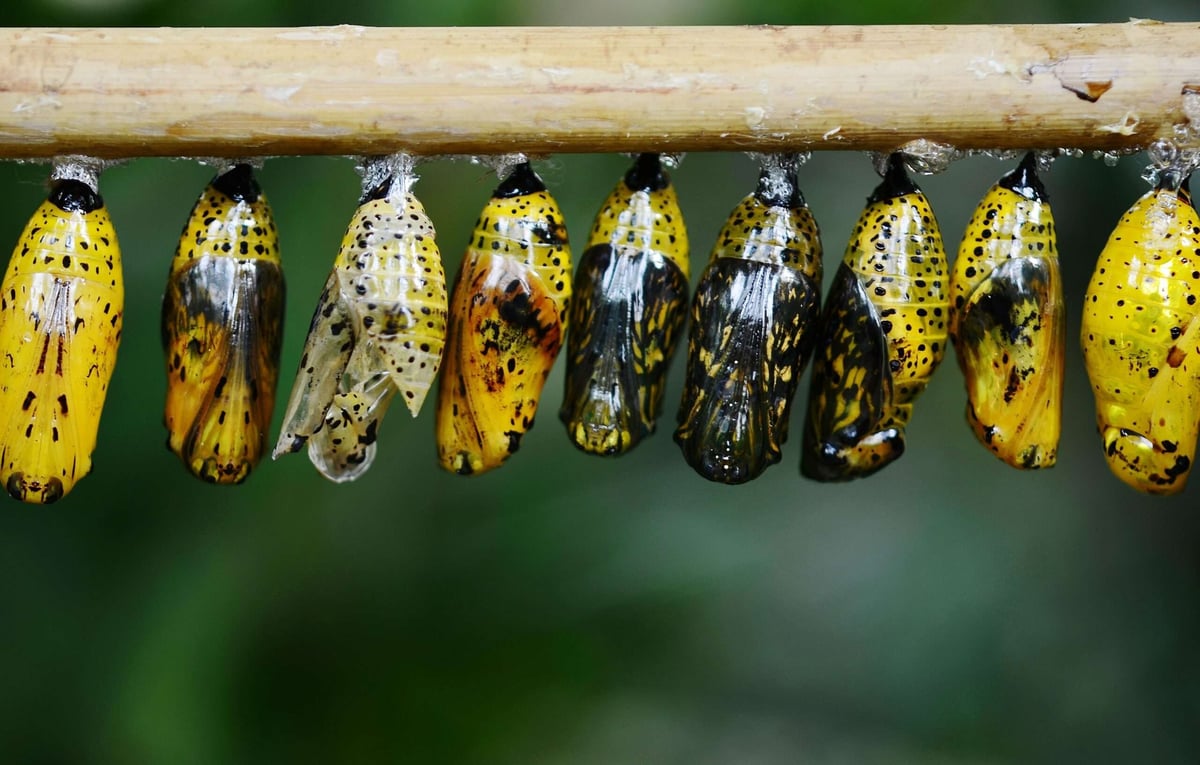
Back in machine learning’s early days, every project was a trial run and many didn’t see daylight. Then big names with deep pockets like Google, Facebook and Amazon started pouring money into pioneering projects, and some of them have delivered a return on the investment — along with a lot of technical debt.
Despite the cloudy picture, there’s loads of anecdotal evidence that we’re entering the phase where we’ll look back and say ‘here’s when ML really arrived as a profession’.
With so many businesses launching their own machine learning initiatives, best practices are starting to emerge that could someday lead to agreed standards and sustainable investments that will keep machine learning moving to full maturity.
ML is still a young science and of course I’m learning along with you. After speaking with the community about this topic over the last few months some common themes have cropped up — let’s call them Bumps On The Road to ML Maturity. I’ve boiled them down here.
Barriers to ML progress
1. Siloes
One is the stubborn persistence of siloes. ML projects are multidisciplinary by their very nature. You need data science, data engineering, development, IT, DevOps, and senior management to collaborate and contribute. If goals aren’t aligned between stakeholders across the organisation, the project is doomed to fail.
The truth is, ML initiatives ‘can’ work in isolation from each other, but they’ll break down later due to the challenges of coordinating workflows between different teams. Sometimes senior management isn’t fully bought-in. Mahogany Row doesn’t always see ML as strategic, making it difficult to measure and deliver value. As proof of this I remember in slack once someone asking what they should tell their manager who is inquiring why they haven’t created anything productive in the last 2 sprints.
After speaking to so many practitioners in roles ranging from DevOps to MLE to Platform teams it has become abundantly clear tools can help demolish silos, but what is truly needed begins on a cultural level. As our recent coffee session guest Jet Basrawi put it “The MLOps Kung Fu is in the culture”
2. Training costs
Two is the cost and resourcing required to do training. You need high-quality data in huge volumes, and there are significant overheads associated with ongoing data access, preparation, and management.
3. Lack of repeatability
Machine learning projects still need a lot of trial and error before they succeed, making it hard to commit to firm timelines for successful completion.
All in all, there’s still a cultural shift waiting to happen, and the creation of a technology environment with people, processes, and platforms operating in alignment to reach ML objectives.
Signs of progress
In that sense, MLOps is still as much an organisational problem as a technical one. Creating a culture that supports ML success won’t happen overnight. Those of us at the vanguard of ML will have to map the potential of MLOps, share the success stories, codify best practice, and show organisations how ML can help them realise practical business goals.
Happily, there are signs that process is underway. Researchers at Cambridge University recently published research that suggests 2021 could be the ‘Year of MLOps.’
They point to the expanding array of tools MLOps has at its disposal. The second half of 2020 saw a new crop of tools and platforms designed to operationalise ML. At the same time, cloud platforms like AWS are adding services to cover aspects of data acquisition, modelling, continuous integration and deployment, and data tracking and monitoring with effective feedback loops.
How well these tools work is a matter of debate, but the fact that the options for MLOps teams are expanding is clearly a positive.
Investment in MLOps tools is also rising. A report from Cognilytica predicts exponential growth in the range of USD 125 billion by 2025. That would represent a 33 per cent annual growth rate and ample evidence that organisations now realise that new tools and platforms are needed for ML deployments to be successful.
Skills sets are expanding, and roles are evolving. We’ve talked about the crossover between data science and data engineering, and how that’s changing how ML teams are comprised. The Cambridge research also points to an expanding AI and ML talent pool with professionals gaining skills on both the operational and development sides of MLOps. Apprenticeships and courses for MLOps are also growing, which suggests enterprises are starting to take it seriously.
Pros on the front lines agree
Inside the MLOps Community, some of the leading voices are also reporting progress on the ground.
We recently caught up with Timothy Chen, Managing Partner at Essence Venture Capital. Chen told us that the business requirements for AI are becoming more sophisticated, and that’s helping to pull machine learning forward.
‘In the past, when you did monitoring, say in Datadog, it was all pure metrics to let you see any drift or differences. But now increasingly you have KPI metrics attached to those drifts, or models built on top of monitoring. Questions are now being asked about security-related issues. So I think we’re seeing MLOps moving along a maturity timeline more or less in parallel with the needs and concerns of the companies using ML products.
The further along a business is in embracing ML, he says, the more likely it is to think about these higher-level issues.
‘Take AI security. It wasn’t really something I’d been thinking about, but then suddenly we had three customers. So that’s really where it becomes interesting. What’s next? I think the lay of the land will keep changing. The buckets are still going to be there, of course, but the definition of those buckets might change.
‘Labelling may just become annotation one day. Or maybe we stop doing human labelling, or automated supervision labelling. There might be something else in the future.’
He says he sees more emphasis on visualisation tools, allowing data scientists to actually see apps being created and how they are consuming data models.
‘The MLOps space is fascinating. I expect it to see big changes every six months.’