Nearly 25% of Data Teams Struggle with Communication When Building Data and ML Workflows – Here’s Why It Matters
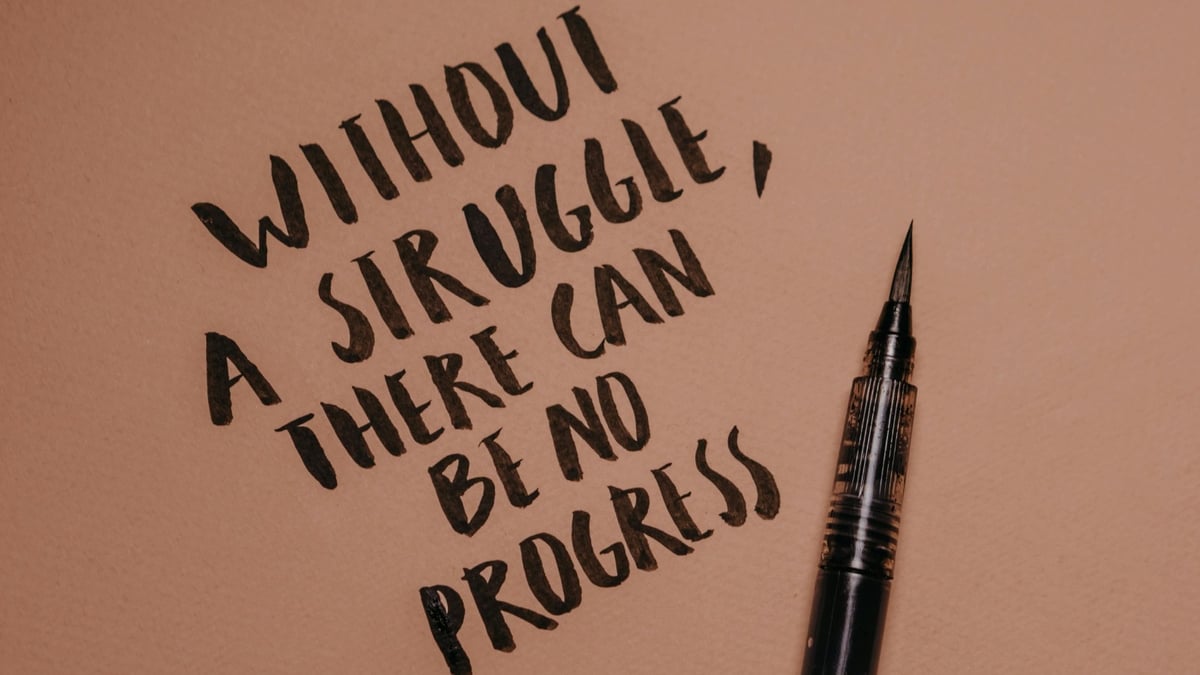
Nearly 25% of Data Teams Struggle with Communication When Building Data and ML Workflows – Here’s Why It Matters
November 1, 2024
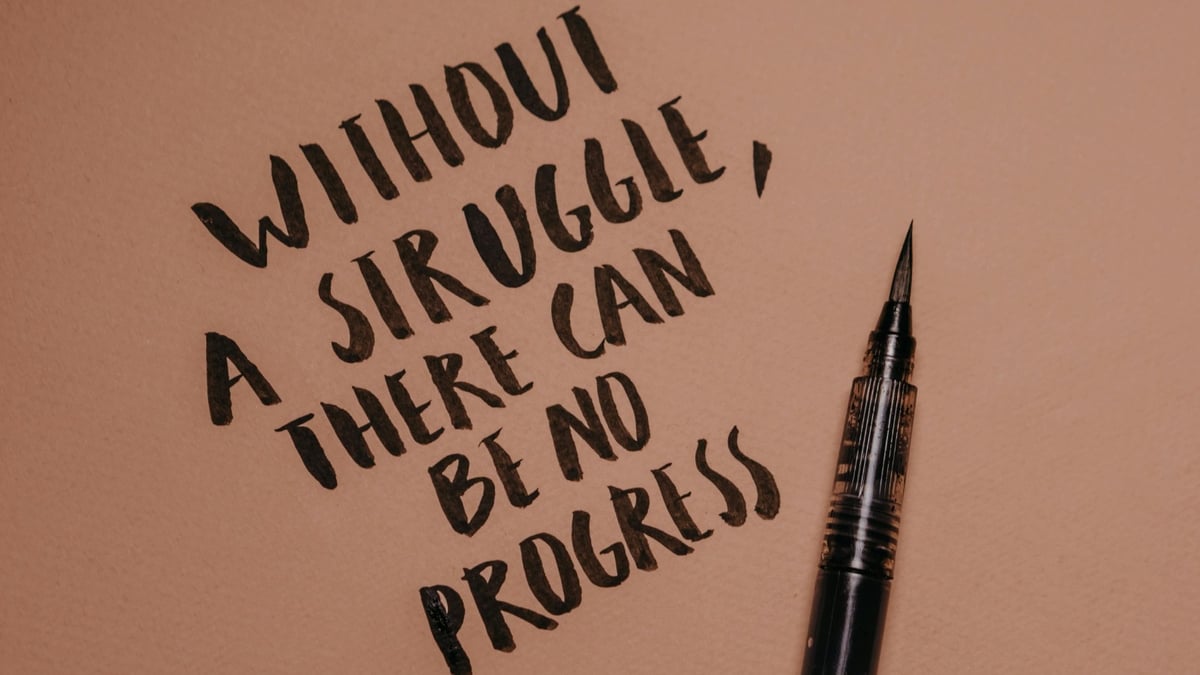
The world of Data Engineering is evolving rapidly, becoming the backbone of scalable machine learning (ML) and AI solutions. In collaboration with the MLOps.community, YouGot.us recently conducted a comprehensive study to uncover the key challenges and practices shaping the future of AI pipelines. The study, conducted in September 2024, gathered insights from over 200 participants across various organizations. The results highlight the critical hurdles teams face in building, orchestrating, and maintaining data and ML pipelines at scale.
In this post, we’ll dive into some of the most impactful findings from the survey, including the top challenges, collaboration structures, and responsibility practices in the world of Data Engineering.
Top Challenges in Data and ML Pipeline Creation
One of the standout findings from the YouGot.us survey is that teams face significant challenges when combining data from various sources, with inconsistent or incomplete data following closely behind ([Image: orgchallenges_Percentage.png]):
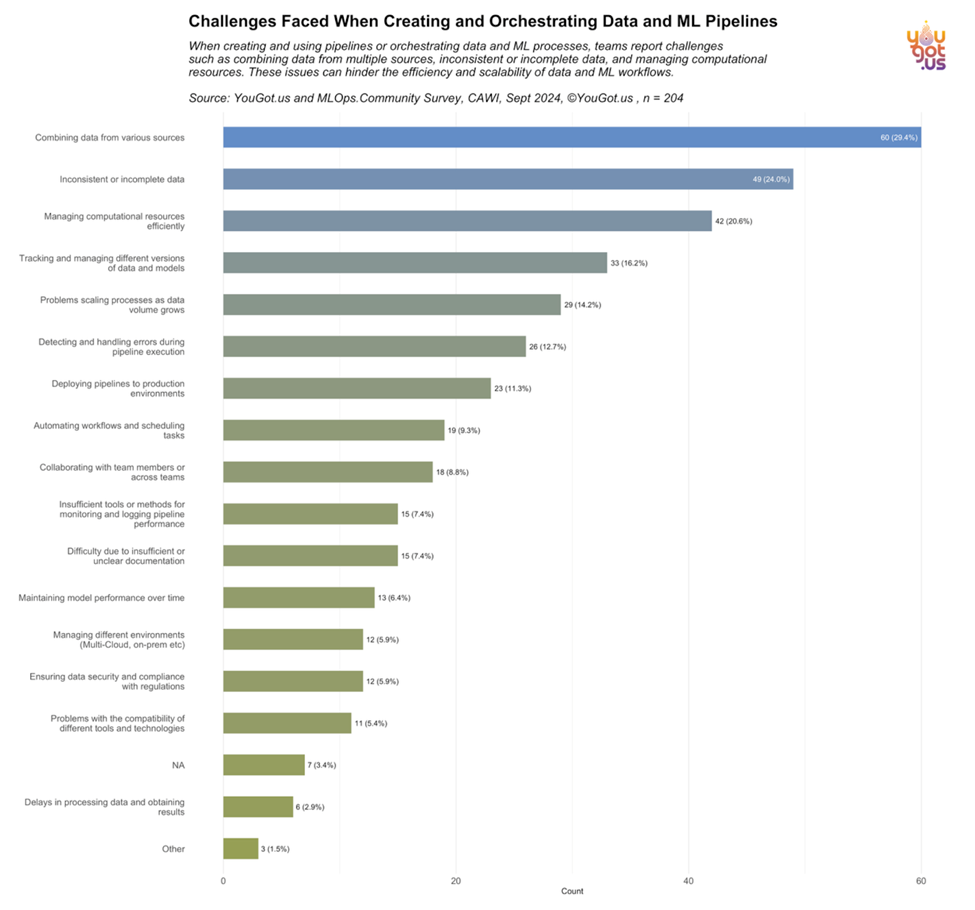
- Nearly 29.4% of respondents cited difficulties combining data from multiple sources.
- 24% of respondents struggle with inconsistent data, signaling a need for improved data governance.
As ML models and AI solutions scale, efficient data integration becomes critical to pipeline success. This finding underscores the need for better data architecture and validation tools to streamline the data flow.
Coordination and Collaboration Hurdles
Teams not only face technical challenges but also coordination barriers. The survey reveals that ensuring clear and consistent communication across teams is the top collaboration challenge ([Image: challenges_Percentage.png]):
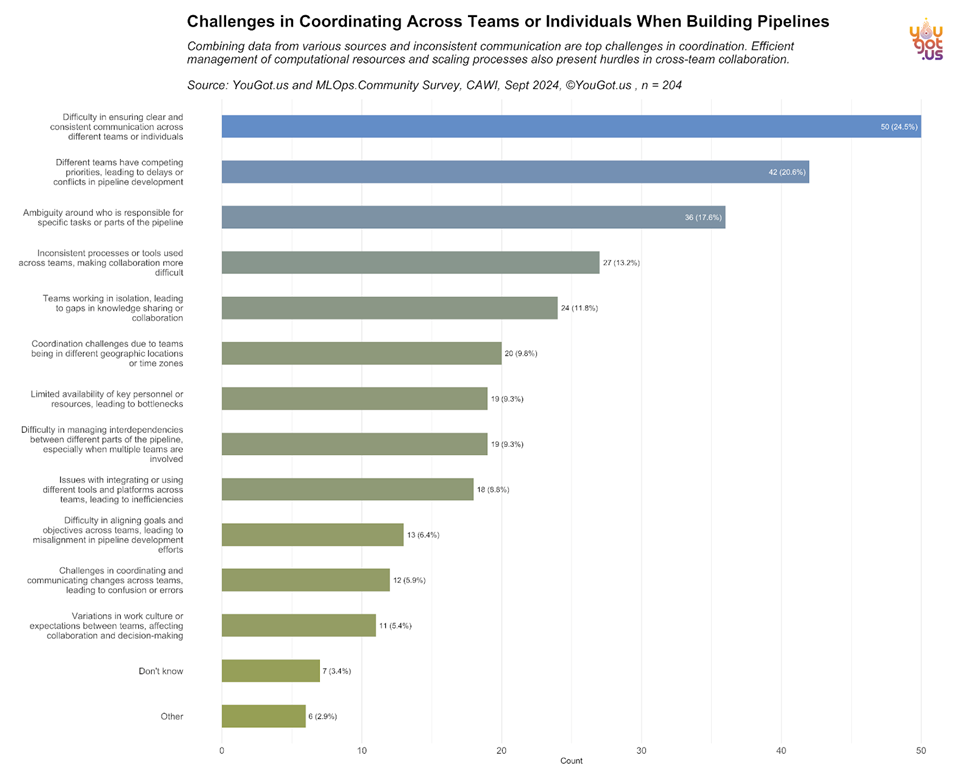
- 24.5% of respondents struggle with communication breakdowns across different teams.
- 20.6% reported conflicts due to competing priorities between teams.
As organizations scale, maintaining effective communication and collaboration becomes a pressing issue, especially in distributed teams. Aligning priorities and streamlining workflows is essential for smooth pipeline execution.
Who’s Responsible for ML Pipelines?
When asked about primary responsibility for ML pipelines, the survey shows that Data Engineering leads the charge, with 27.5% of respondents pointing to data engineers as the key players ([Image: data24_Responsibillty.png]):
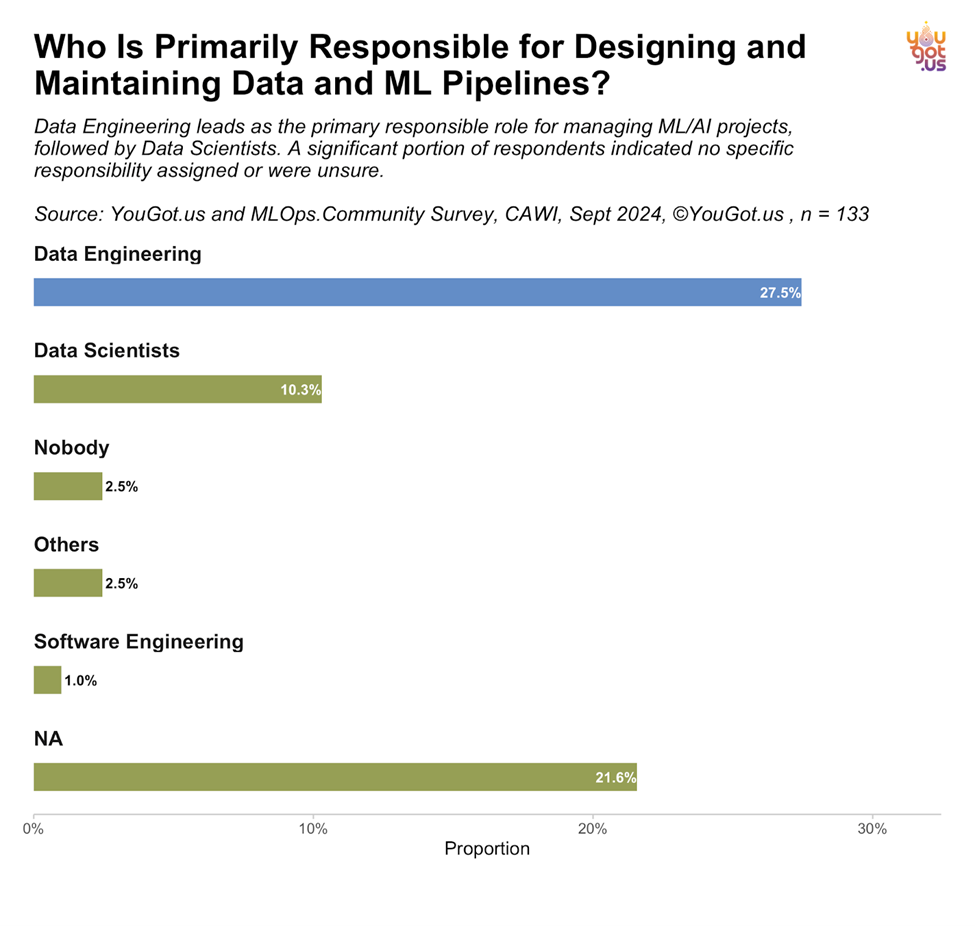
- A notable 21.6% of participants were unsure or reported no specific responsibility, indicating a lack of clarity in some organizations regarding pipeline ownership.
This finding highlights the increasing demand for well-defined roles and responsibilities within organizations to ensure that data pipelines are managed effectively.
How Teams Collaborate on Pipelines
Multiple collaboration models were found across organizations. The most common approach involves multiple teams working together on different parts of the pipeline, followed by specific teams managing certain stages ([Image: data24_collab.png]):
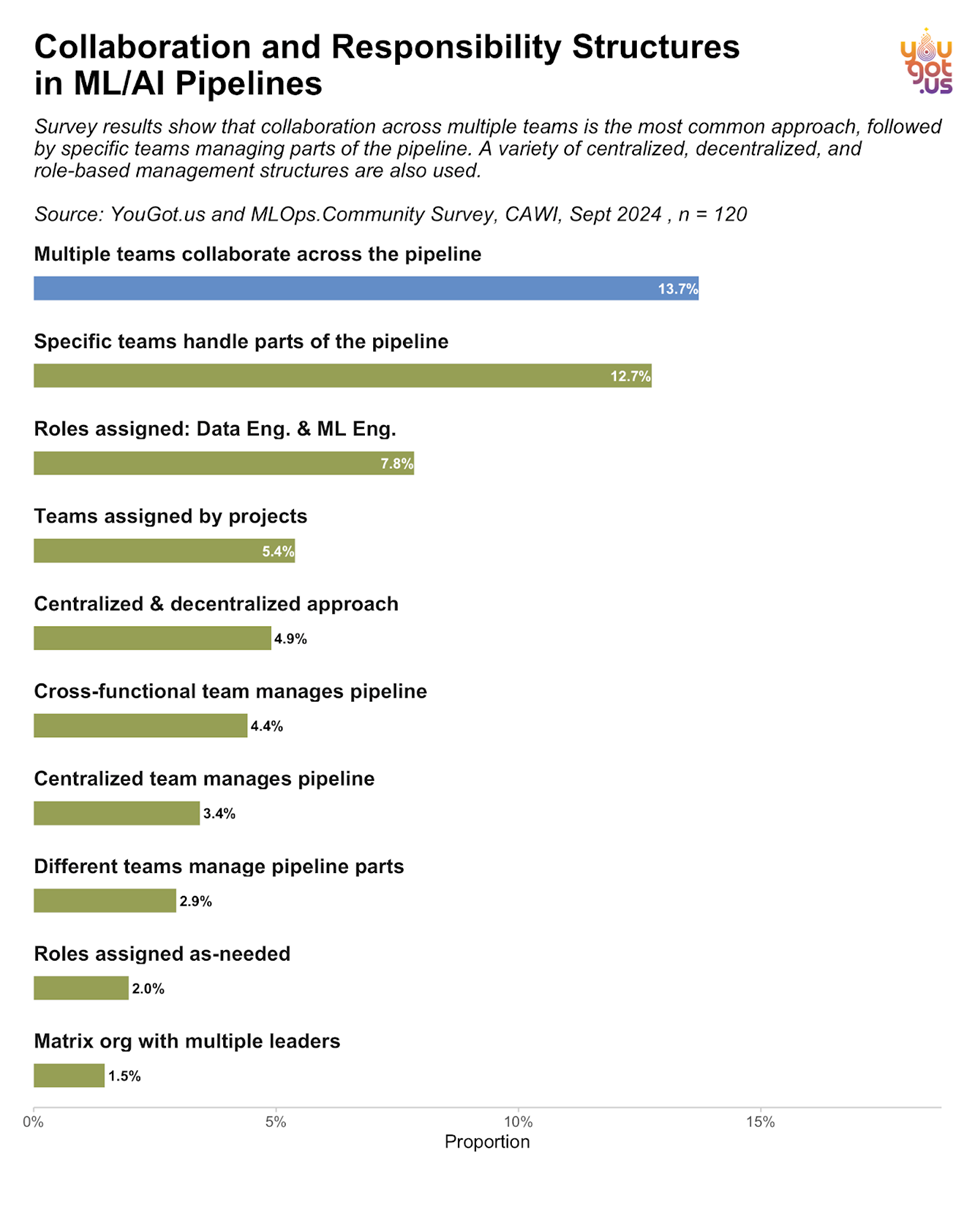
- 13.7% of respondents indicated that multiple teams collaborate across the pipeline.
- 12.7% favor a model where specific teams manage individual pipeline stages.
Depending on organizational size and complexity, a tailored approach to team structure can improve efficiency and reduce bottlenecks.
Data Contract Practices: Formal Agreements Still Lacking
Interestingly, the survey revealed that many organizations don’t have formal data contracts in place, despite the growing importance of data quality and compliance. 14.2% of respondents indicated no formal agreements exist between teams ([Image: data24_datacontracts_v2_sorted.png]):
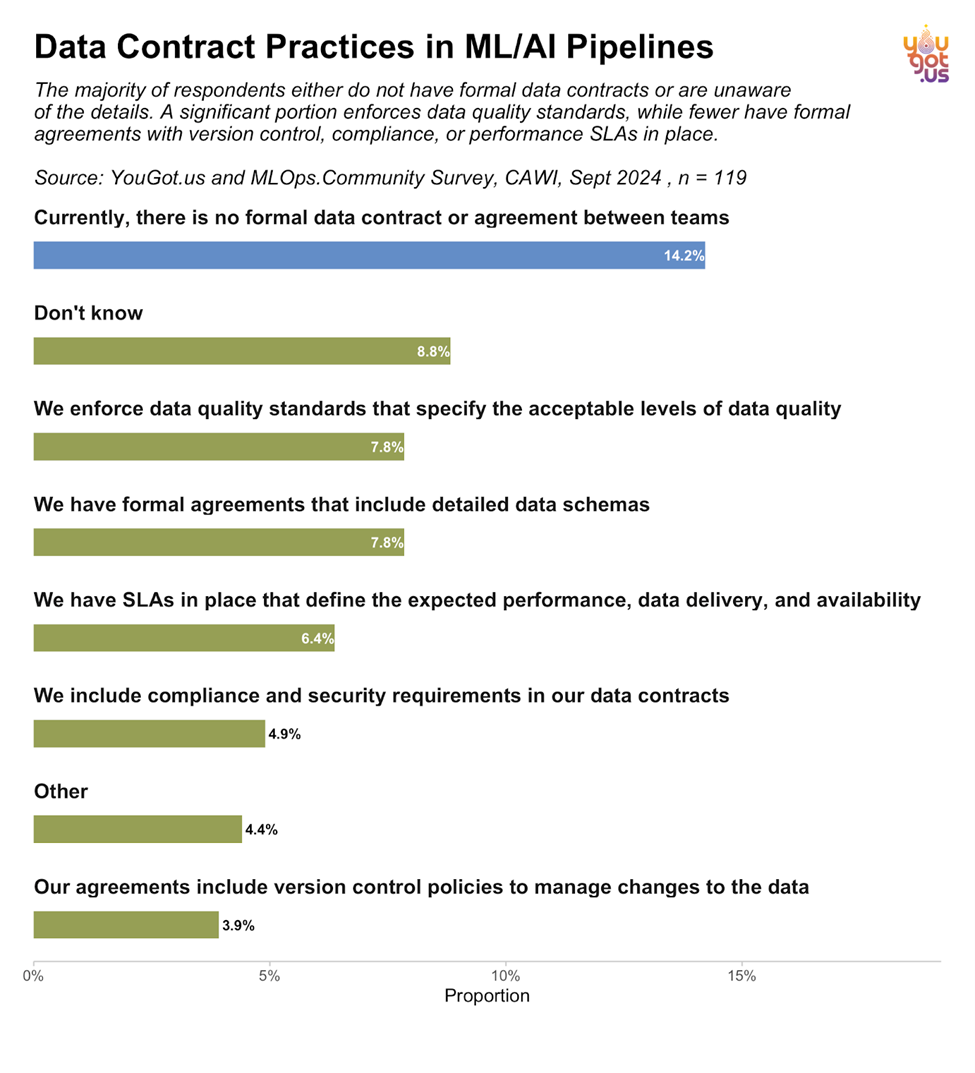
- Only 7.8% of organizations have formal agreements that include detailed data schemas, while another 7.8% enforce data quality standards.
Formalizing data contracts can help teams set clear expectations around data quality, performance, and compliance, particularly as data pipelines grow more complex.
Conclusion and Next Steps
This study, conducted by YouGot.us Research, highlights both the technical and organizational challenges that teams face when building ML and AI pipelines. From managing data complexity to improving cross-team collaboration, there’s a clear need for more robust processes and clearer responsibility structures.
For organizations looking to dive deeper into the data or receive a custom analysis of their specific challenges, YouGot.us Research offers an in-depth look at the survey results and personalized reports. Visit [YouGot.us](https://yougot.us) and [MLOps.community](https://home.mlops.community/) to stay updated on the latest insights and best practices for Data and ML Engineering. For a deeper analysis or custom insights, contact our research team or email us at [email protected].
Related Post: