The Role of AI Safety Standards in Modern MLOps
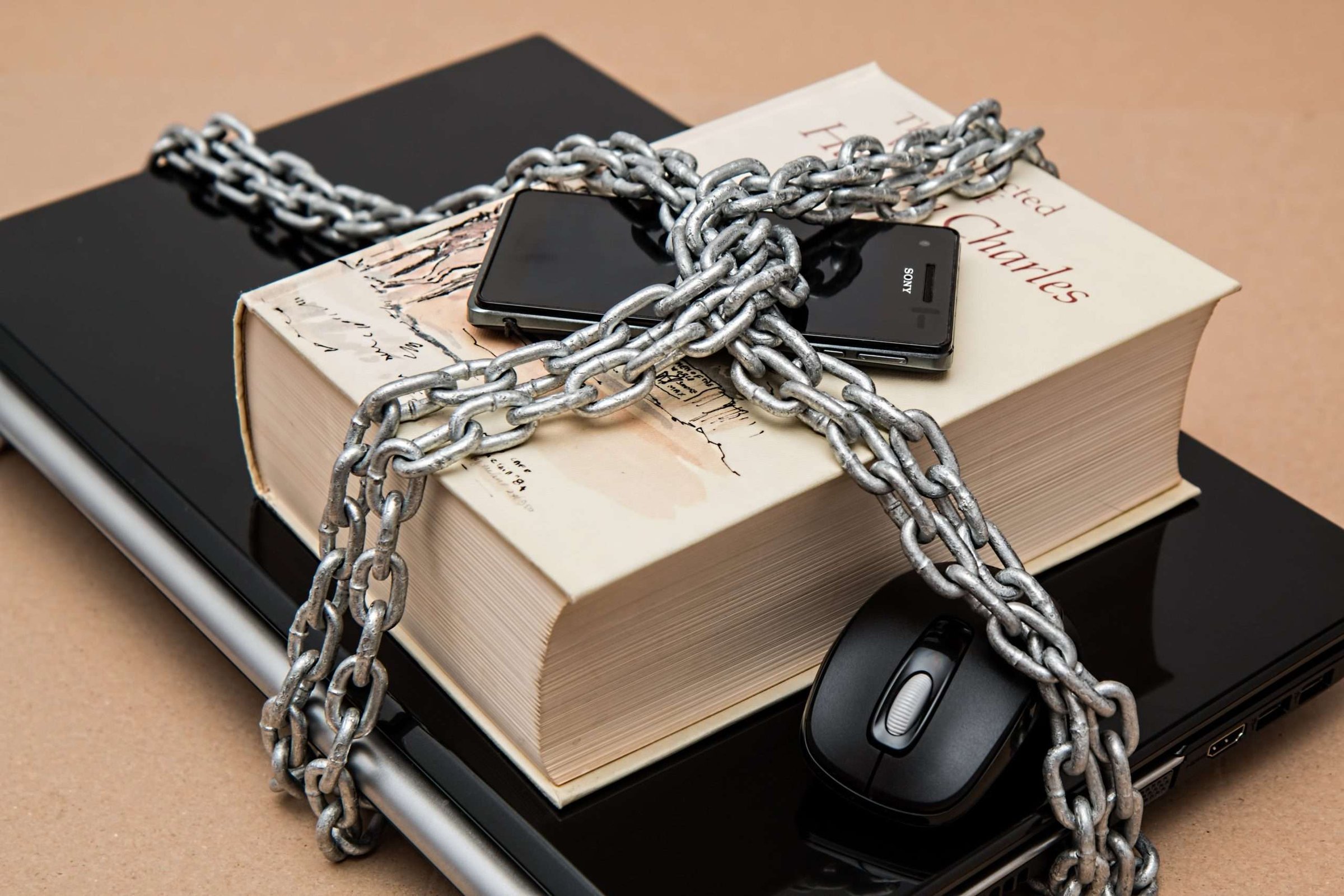
With the recent explosive growth of AI, particularly in Generative AI, the importance of safety and reliability has surged as a paramount concern for businesses, consumers, and regulatory bodies
February 15, 2024
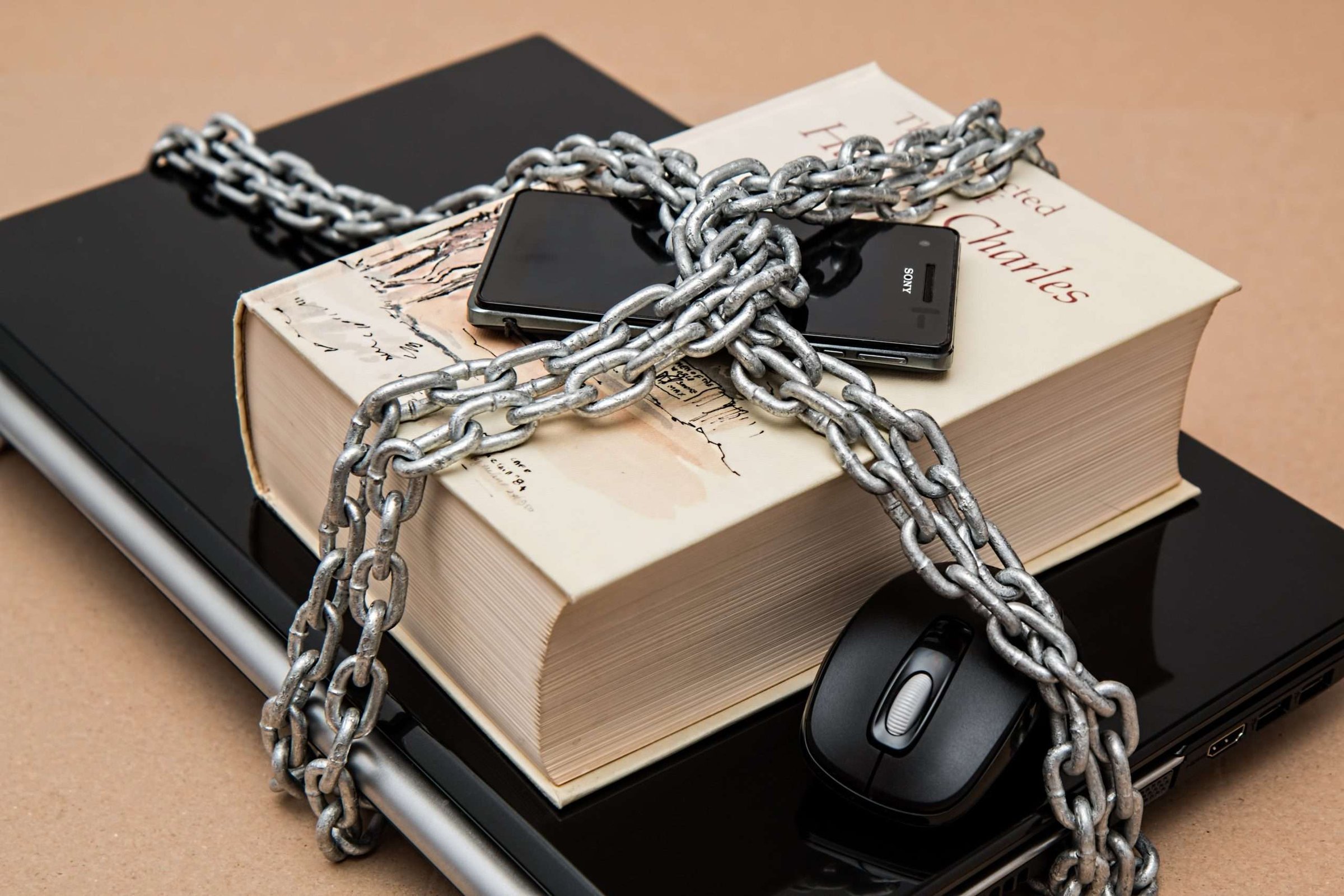
With the recent explosive growth of AI, particularly in Generative AI, the importance of safety and reliability has surged as a paramount concern for businesses, consumers, and regulatory bodies.
Recent safety standards and regulations as outlined in the EU AI Act and Biden’s executive order underscore the imperative to ensuring safe and trustworthy AI. Furthermore, the existence of over 20 ISO standards dedicated to AI safety presents a formidable challenge in integrating them effectively into operational frameworks.
Beyond considerations of safety and adherence to regulations, the issue of trust remains a significant challenge. How can MLOps integrate best practices to develop and deploy AI models that inspire confidence and trust among consumers, while ensuring compliance?
According to KPMG, a significant 66% of executives harbor reservations about trusting AI.
Addressing the operational risks and complexities inherent in the large-scale deployment of high-stakes AI models is indeed a formidable challenge. To explore these concerns, a round table discussion titled ‘The Next AI Frontier: AI Safety Audits and Standards’ convened leading experts from academia, industry, AI auditing firms, governmental bodies, and standardization agencies. The conversation delved into the practical dimensions of AI safety standards and audits, particularly focusing on the critical safety hurdles encountered in deploying AI within high-stakes applications.
According to a survey by Model Ops, 80% say difficulty managing risk and ensuring compliance is a barrier to AI adoption for their enterprise.
Key Takeaways
- AI safety should not be an afterthought
The panel emphasized the importance of ensuring AI reliability and safety for high-stake AI applications by implementing AI safety standards and conducting third-party audits to ensure a baseline level of safety assurance in the industry. A recurring challenge in the industry, raised by Dr. Pavol Bielik, Co-founder and CTO at LatticeFlow AI, is the tendency of companies to prioritize optimizing their AI models for perfect scenarios, often considering safety only as an afterthought. He advises that AI safety should be implemented from the beginning of the AI development lifecycle. “It is important that AI safety topics are incorporated from the start, not as an afterthought” – Dr. Pavol Bielik, CTO and co-founder of LatticeFlow AI.
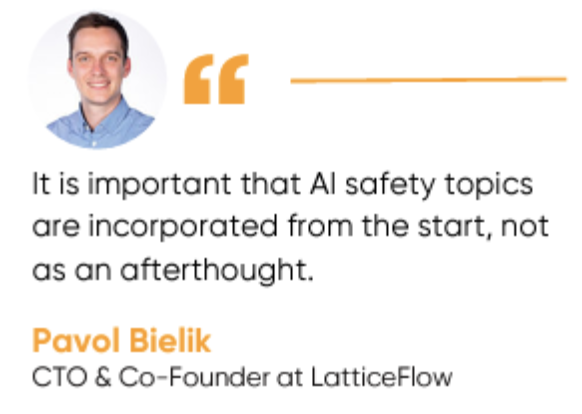
- Development of AI Safety Standards – Basic standardizations are needed to address issues like cybersecurity and biases. While the EU AI Act outlines basic principles around AI regulations, they are still rather vague and the need to translate them into actionable steps remains a top priority. NIST sees standardization as crucial for mapping out risks and providing practical suggestions for mitigation. Rather than enforcing regulations, the organization focuses on offering technical guidance through various initiatives and workshops. NIST has taken several initiatives including the release of an AI framework last year. Most recently, they issued a taxonomy of adversarial attacks and mitigations to help the community of stakeholders understand what kind of problems they are facing when they’re deploying specific processes of AI technology and different use cases.
- Measurement Criteria for AI Robustness
AI systems should be resilient and maintain accuracy when faced with unexpected or adversarial data inputs.An AI expert at NIST highlights that making AI safety measurable is crucial for two main reasons. First, it enables stakeholders to establish acceptability criteria based on these measurements. Second, it aids in making progress and safety improvements visible. NIST is actively developing specific safety measures for use cases such as autonomous driving. It is looking into establishing measurement criteria that characterize the robustness of AI systems. For instance, object detection and classification are critical core tasks in autonomous driving as they help the car to identify and locate objects to understand its surroundings better. Developing a system to guide the industry in setting up measurement criteria to score their systems with respect to the acceptable ranges of inputs is a key initiative NIST is working on. Last but not least, NIST believes that certification processes should not burden innovation and should adapt to the evolving AI landscape to ensure the timely assessment of AI systems.
- Role of Third-party AI Audits in Establishing Customer Trust
For companies developing AI for high-stakes applications, having their models evaluated by an independent third party is crucial for gaining customer trust. Regardless of regulations, we still need to understand how the system is working from a bottom-up approach that is starting to get adopted by companies where you can dive deeper into the level of data, models, and properties. The emerging bottom-up demand from companies building AI applications was further confirmed by Pavol Bielik. Instead of telling customers that your AI is safe, demonstrating its safety and robustness is much more effective through a comprehensive technical assessment.
- A systematic approach to evaluating your AI systems
The key to managing risk is assessing, understanding, remediating, and monitoring model behavior across the AI lifecycle. Instead of providing ad-hoc “points-in-time” assessments, it is much more effective to adopt a systematic approach to assessing your model performance and data quality across the entire AI lifecycle while facilitating CI/CD pipeline.
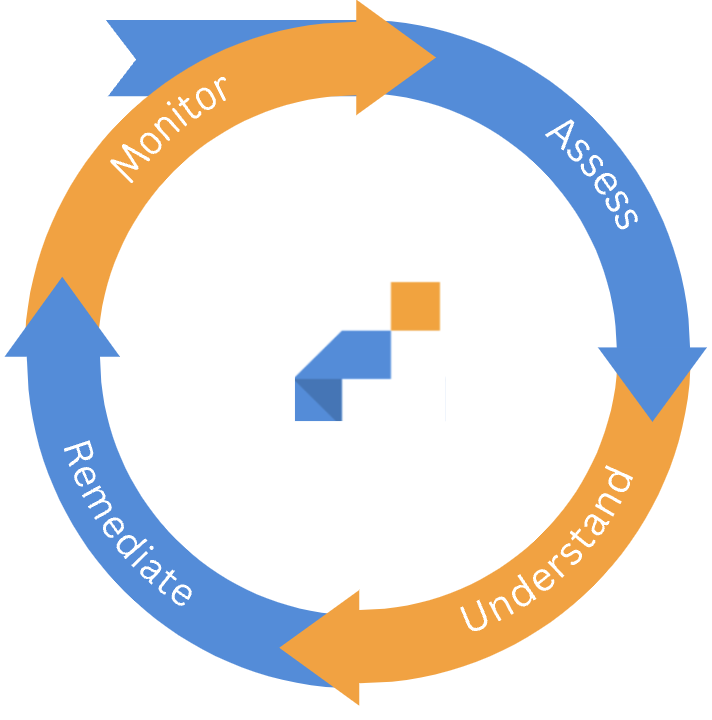
By systematically identifying model and data issues, you can improve their model performance and data quality to extend beyond aggregate performance.
The importance of safety and compliance cannot be overstated, particularly in mission-critical AI applications. For larger organizations, the implementation of robust AI governance and Responsible AI programmes is not merely an option but a critical necessity for long-term, sustainable operation. In addition, the long-term costs of quick fixes under tight deadlines can eventually lead to technical debt, thus compromising quality and reliability. A model with a complex architecture might initially perform well, but it could become a maintenance nightmare as new data streams in or if the model needs adapting for new tasks. Consequently, the ML system often does not address the business needs in a technically sustainable and maintainable manner.
For development teams that lack the bandwidth to perform effective independent reviews and validations, external third-party experts can provide invaluable perspectives to challenge and validate internal results. This is crucial for ensuring the responsible deployment and maintenance of AI/ML systems. Periodic external red-teaming can also help in identifying risks in live systems.
These key takeaways underscore the necessity of integrating AI safety, robustness, and ethical considerations into all stages of AI development and deployment to ensure trust, reliability, and compliance.
Does your model have blind spots?