Understanding LLMOps: Navigating the waters of large language models
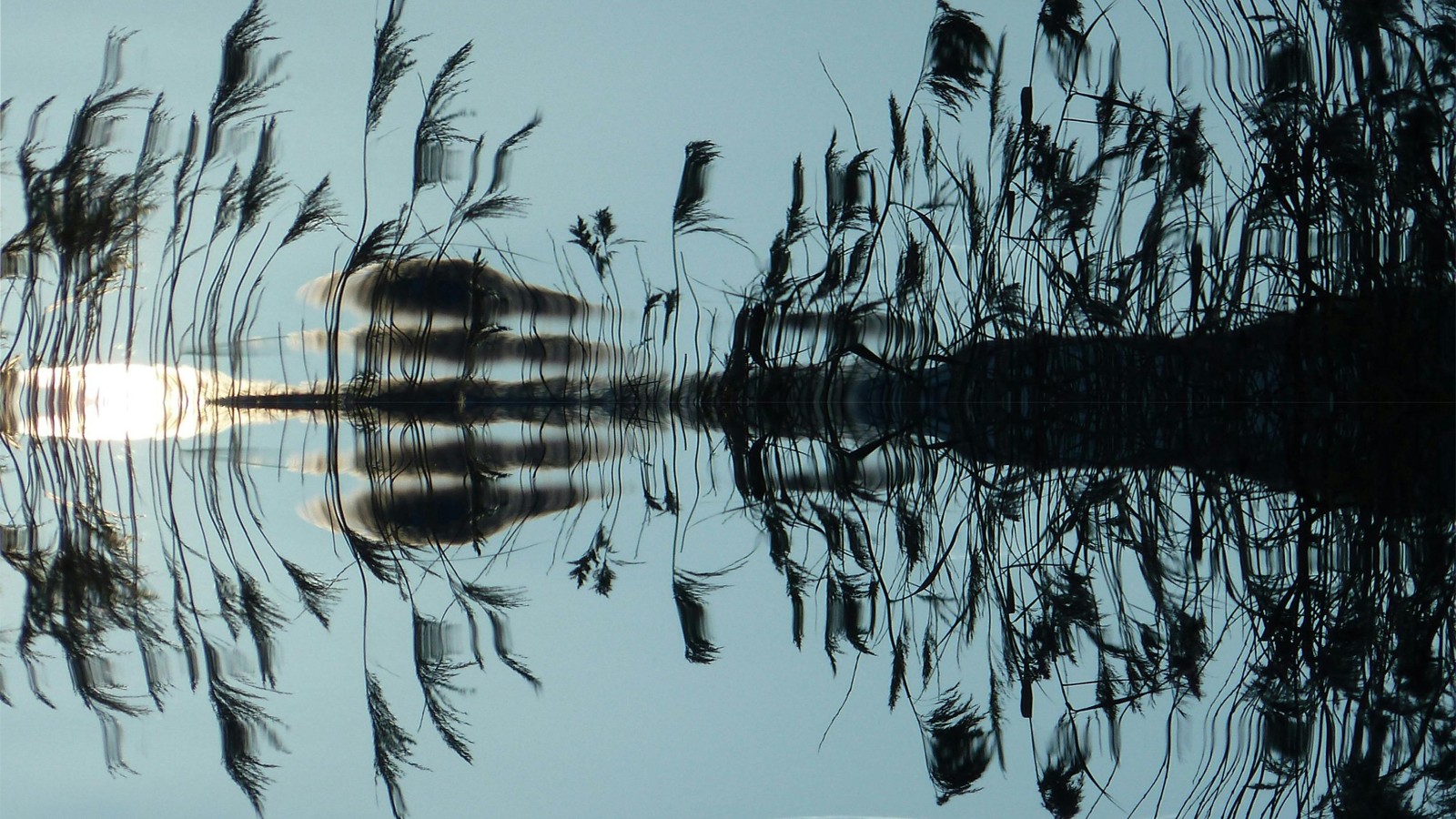
Large language models (LLMs), like ChatGPT and Microsoft Copilot, have moved quickly out of the novelty phase into widespread use across industries.

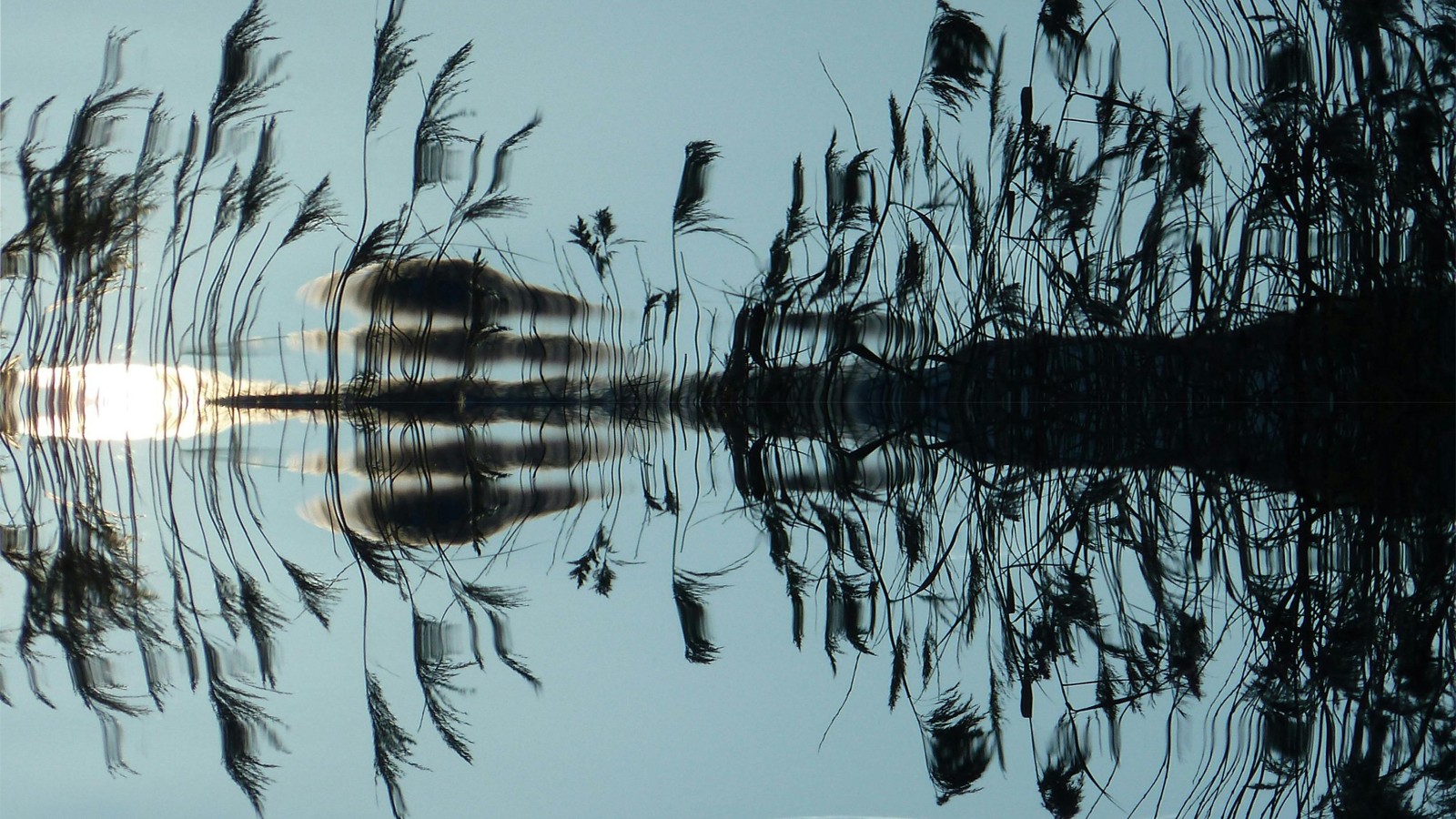
Among other examples, these new technologies are being used to generate customer emails, summarize meeting notes, supplement patient care and offer legal analysis.
As LLMs proliferate across organizations, it becomes important to evaluate where we can apply traditional business processes to monitor and ensure the accuracy of the models.
Let's look at the role that large language model operations (LLMOps) can play in the explosion of LLMs in business and discuss additional challenges that LLMOps could help solve.
A quick background of MLOps
Monitoring machine learning models after they’ve been put into production has long been an important part of the machine learning process. While still fast evolving, the field of machine learning operations (MLOps) has come into its own, with large and active online communities and many expert practitioners.
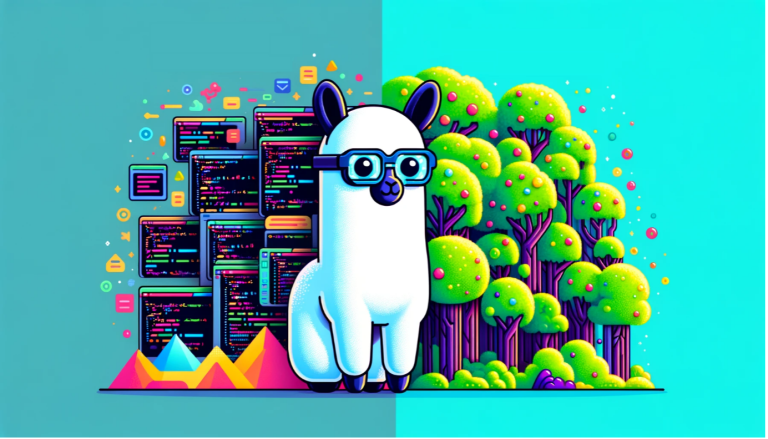
This image was generated using ChatGPT-4
The value and need for MLOPs are clear since models in production tend to degrade over time as user behavior shifts. As these shifts occur, it’s important for the business to ensure consistency in the data and the quality of the models. The demand for documentation and verification of model accuracy is on the rise due to regulatory initiatives like the EU AI Act.
Like so many other areas across society, the introduction of ChatGPT in Nov 2022 changed the landscape of MLOPs. With more and more businesses using LLMs, the topic of LLMOps is gaining traction. After all, if monitoring and maintaining models in production has value for other types of machine learning, it surely has value for LLMs, too.
Since then, LLMs have become indispensable tools for professionals across various sectors, offering unprecedented capabilities in natural language understanding and content generation. Using these powerful tools in practical applications introduces a specialized operational domain: LLMOps. This concept extends beyond traditional machine learning operations, focusing on the unique challenges associated with the deployment and maintenance of LLMs for end-users.
Navigating LLMOps: A user's guide
LLMOps encompass the strategies and practices required to effectively integrate LLMs into business processes. Unlike traditional MLOps, which may cater to various machine learning models, LLMOps specifically addresses the intricacies of employing LLMs as tools rather than developing them.
The core challenges with LLMOps involve managing the unstructured nature of input data and mitigating the risks of misleading or inaccurate outputs, commonly referred to as hallucinations. Let’s look at each of those areas in more detail.
The challenge of unstructured inputs
One of the primary hurdles in employing LLMs is their reliance on vast amounts of unstructured data. In practical applications, users often feed LLMs with real-world data – including images, text and videos – that can be messy, inconsistent and highly varied.
Data can range from customer queries and feedback to complex technical documents. The goal for LLMOps is to implement systems that can preprocess and structure this data effectively, ensuring that the LLM can understand and process it accurately, thus making the tool more reliable and efficient for end-users.
Addressing output hallucinations
Another significant challenge is the potential for LLMs to generate plausible but incorrect or irrelevant responses, known as hallucinations. These inaccuracies can be problematic, especially in critical applications like medical diagnoses, legal advice or financial forecasting.
Here, the goal for LLMOps should be to focus on strategies to minimize these risks, such as implementing robust validation layers, incorporating user feedback loops and establishing clear guidelines for human intervention when necessary.
LLMOps vs. traditional MLOps
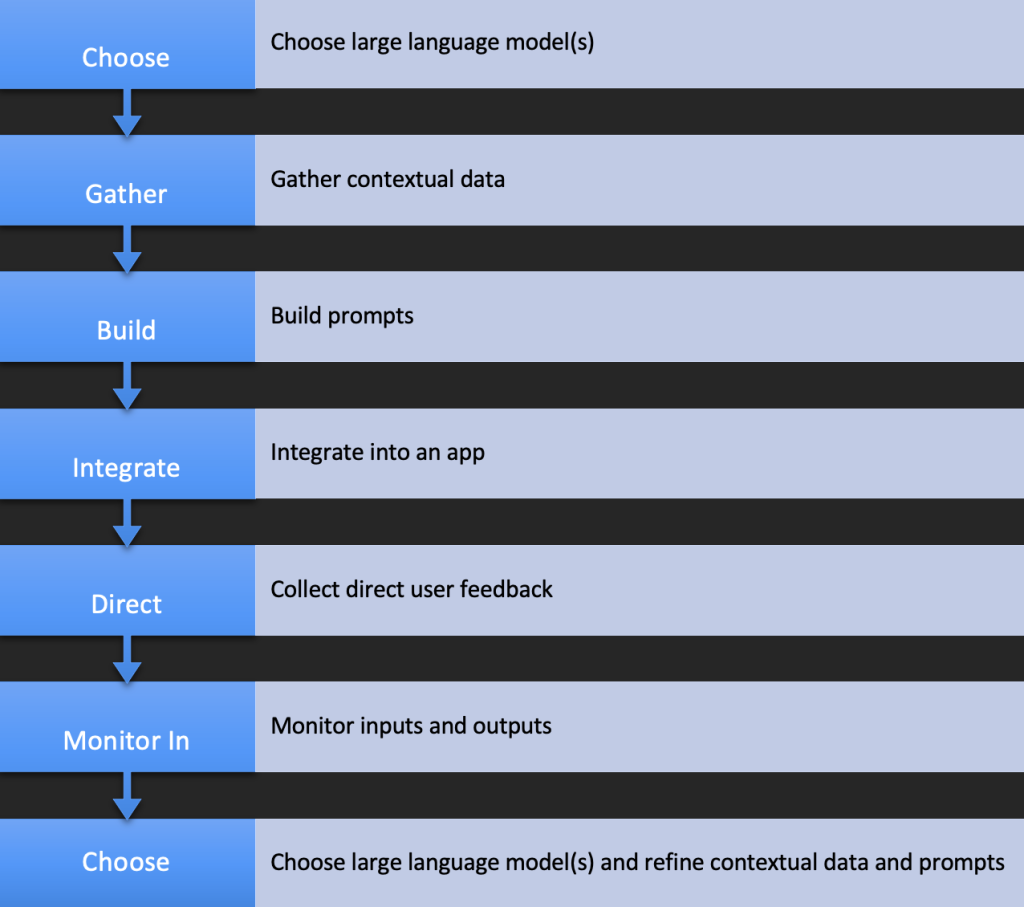
The lifecycle of large language models in practical applications
While LLMOPs share some common ground with traditional MLOps – such as the need for model monitoring and performance tracking – the focus significantly shifts toward managing the user interaction with LLMs. The goal is to maintain the model's performance and ensure that the LLM is an effective, reliable tool for its intended application, accommodating the unstructured nature of human language and thought.
As LLMs continue to permeate various sectors, the importance of specialized operational practices cannot be overstated. LLMOps stands at the forefront of this new era, addressing the unique challenges of employing LLMs as dynamic, interactive tools. By focusing on the specific issues of unstructured data management and output validation, businesses can harness the full potential of LLMs, transforming them from mere technological marvels into indispensable assets in their operational arsenal.
Adapting to LLMOps is not merely an operational upgrade but a strategic necessity for any organization looking to use the transformative power of LLMs.
Read: Generative AI and Large Language Models Demystified
Originally posted at: