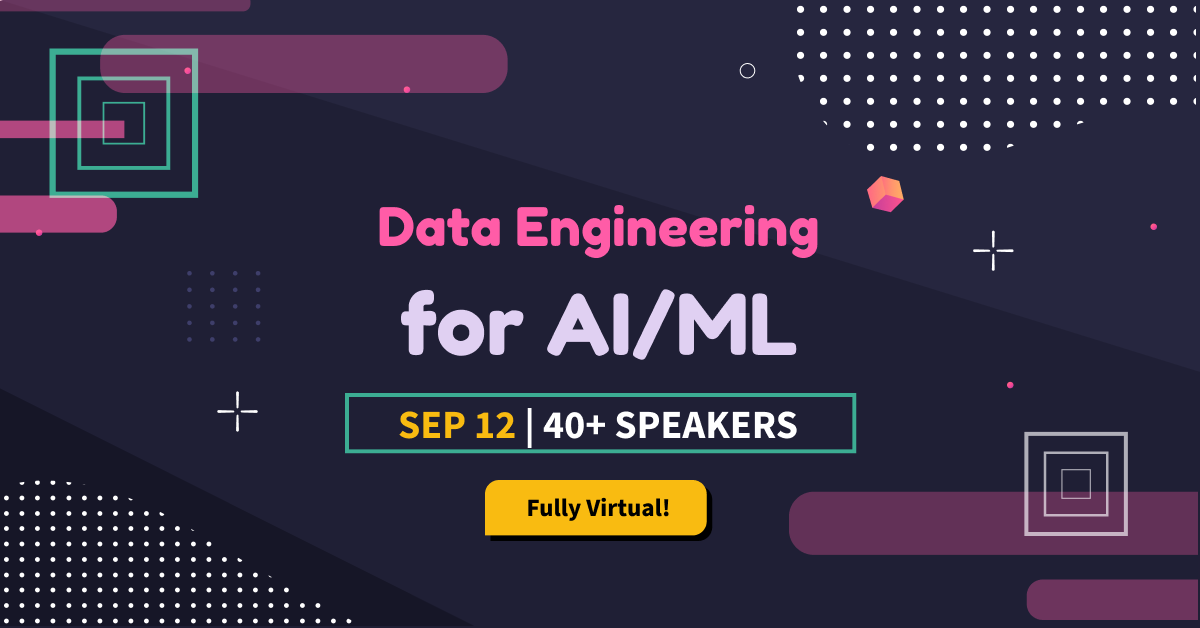
LIVESTREAM
Data Engineering for AI/ML
Your AI Means Nothing Without the Data.
Everyone in data engineering is fighting a hard battle you know nothing about.
This is a conference at the intersection of Data and AI. It will be fun and educational. Don't believe me? Check out what past guests have said.
Oh yeah, and the speakers are top of their game.
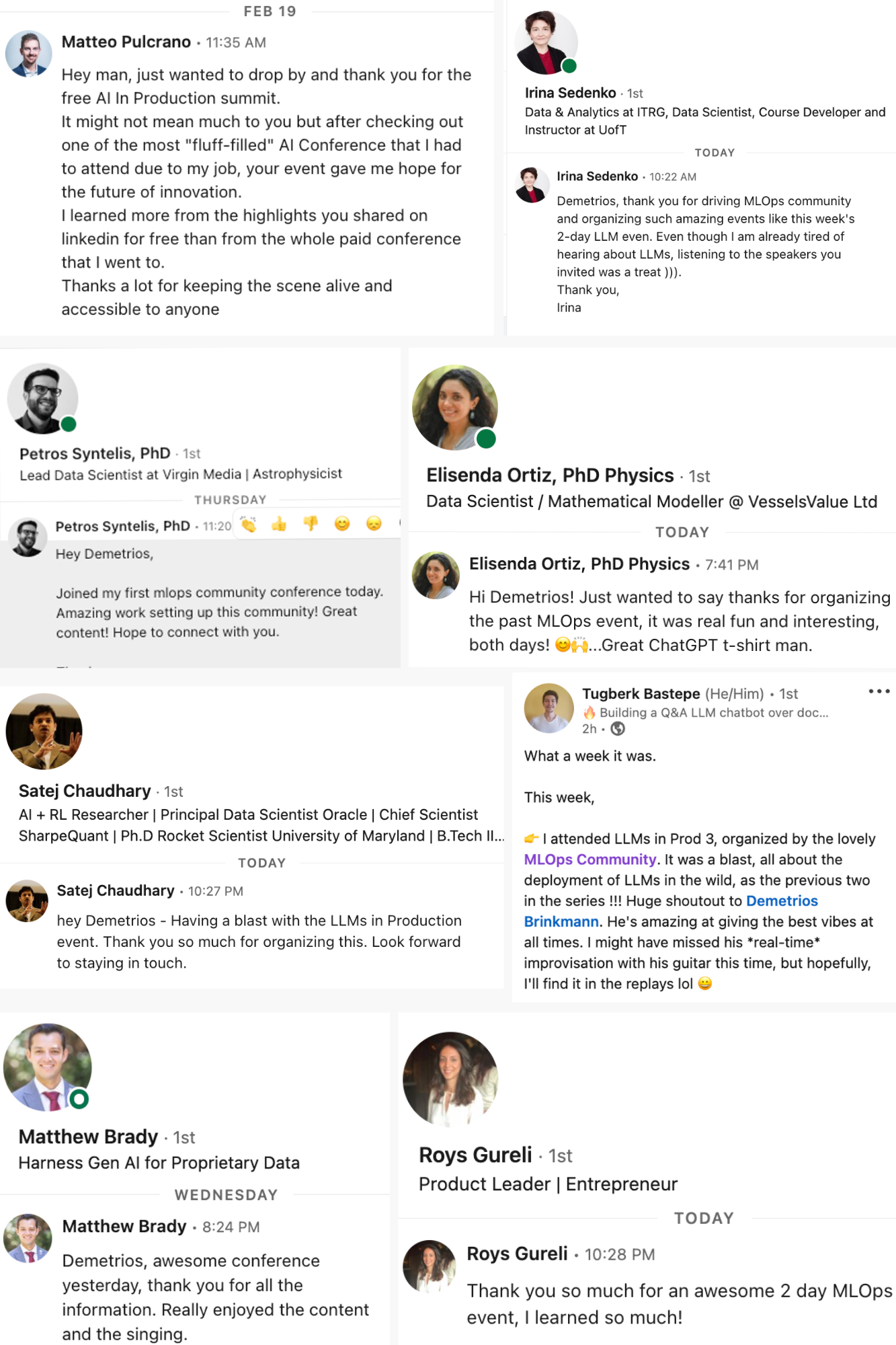
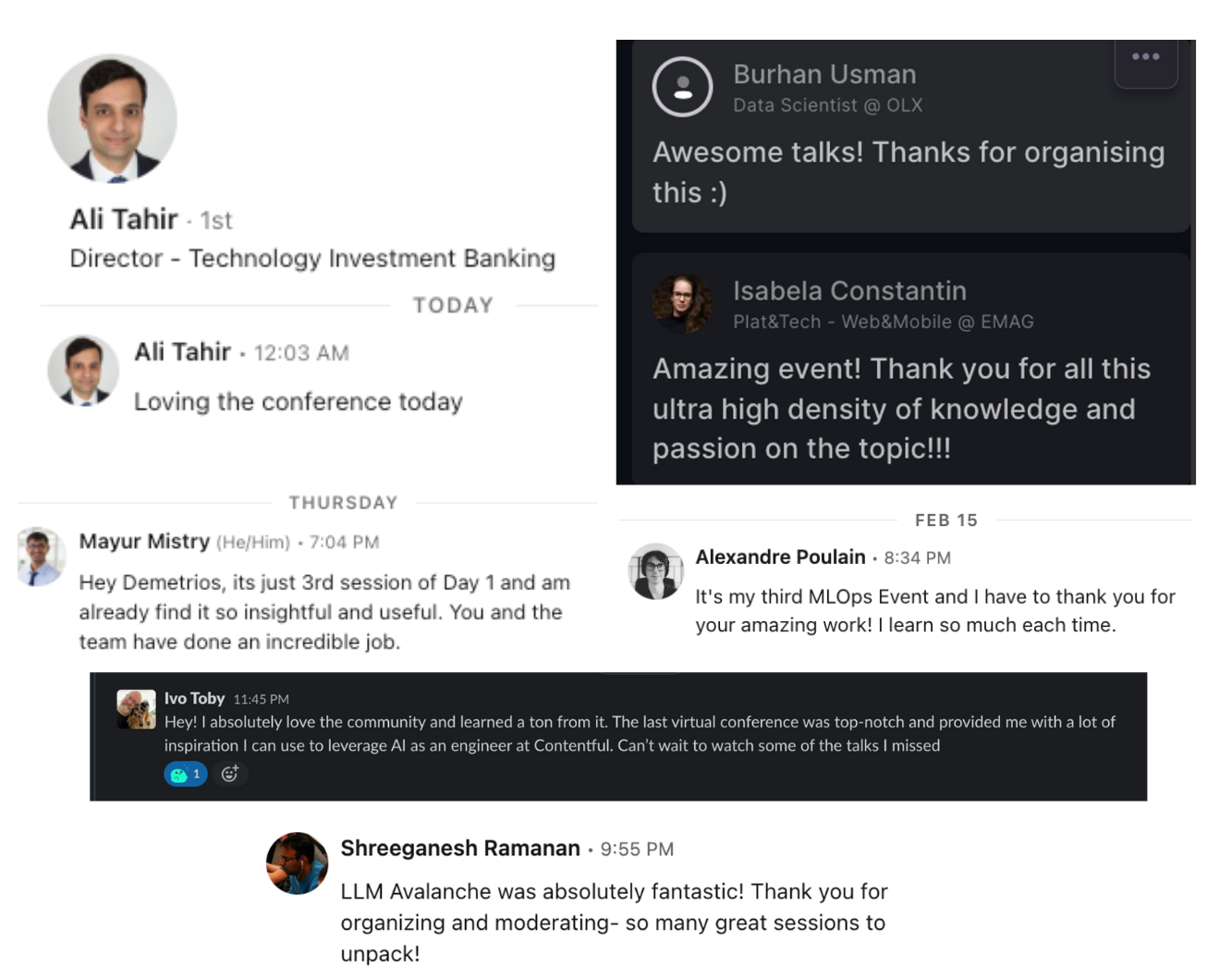
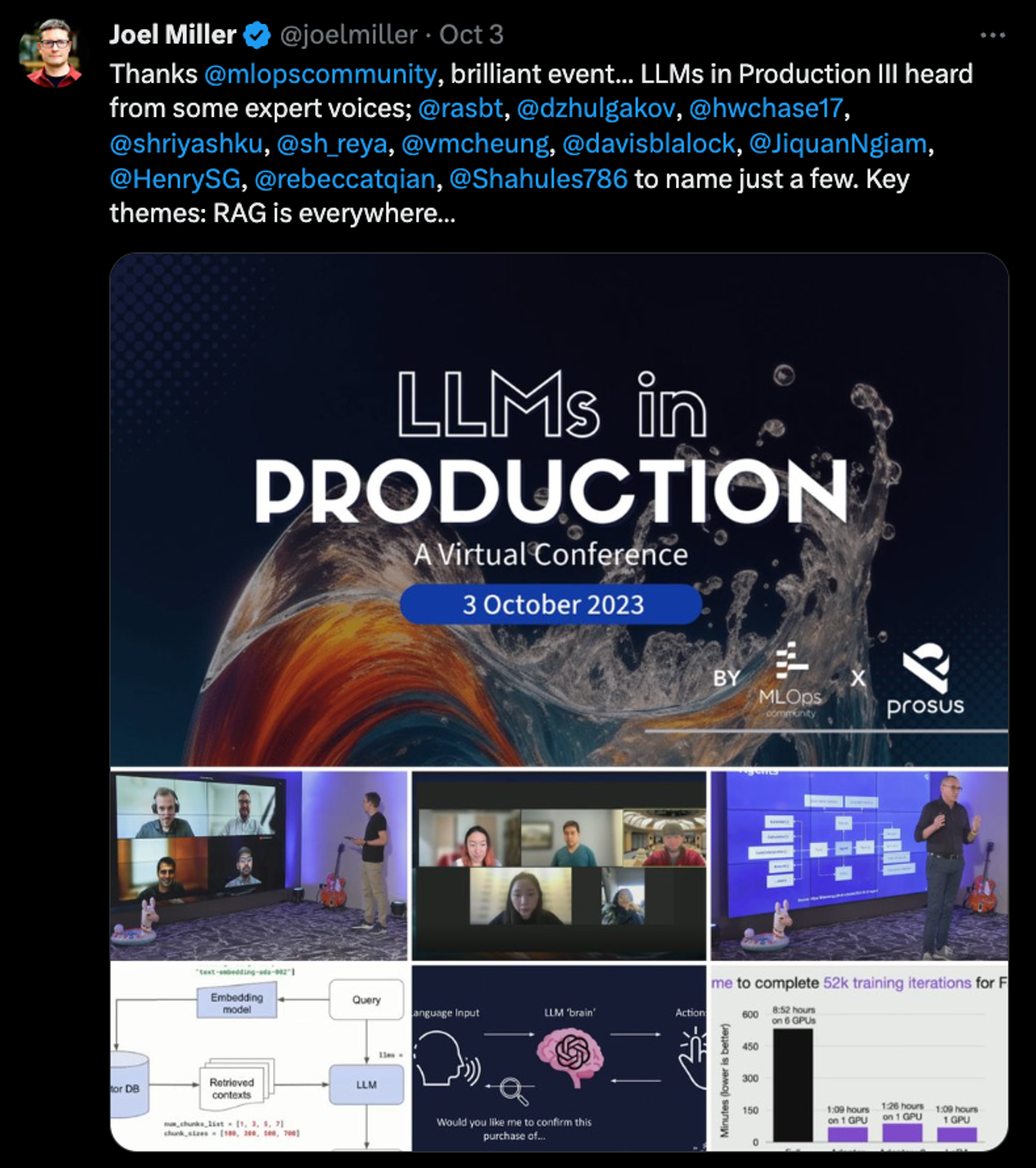
Speakers
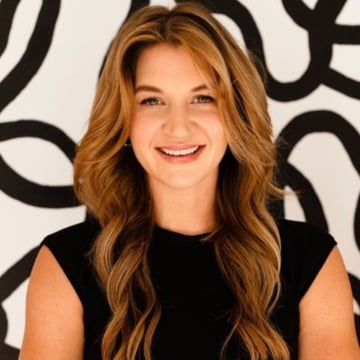
Sadie St. Lawrence
Founder / AI Instructor @ Human Machine Collaboration Institute / LinkedIn Learning
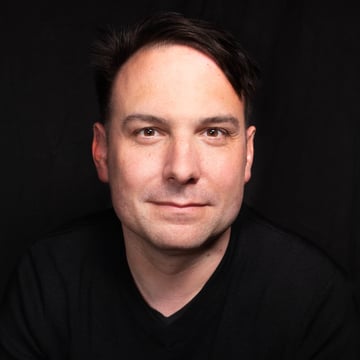
Hannes Mühleisen
Co-Founder & CEO @ DuckDB Labs
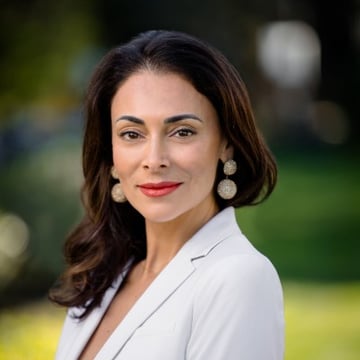
Sol Rashidi
CEO and Founder @ ExecutiveAI
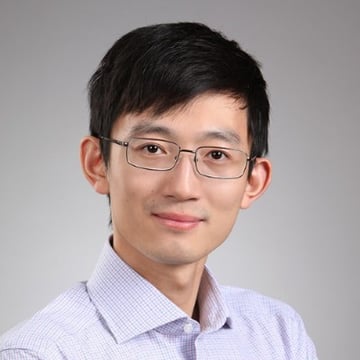
Tengyu Ma
Co-Founder and CEO @ Voyage AI
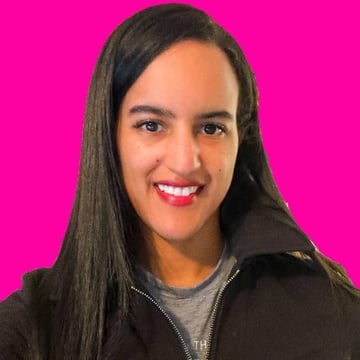
Shelby Heinecke
Senior AI Research Manager @ Salesforce
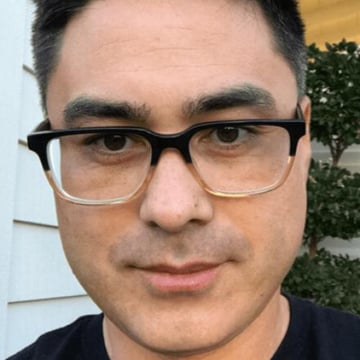
Joe Reis
CEO/Co-Founder @ Ternary Data
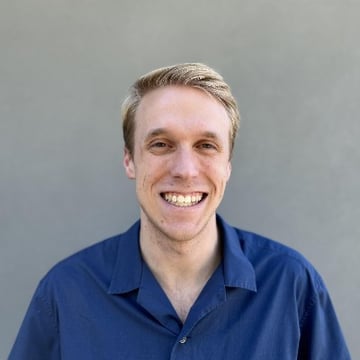
Ryan Wolf
Deep Learning Algorithm Engineer @ NVIDIA
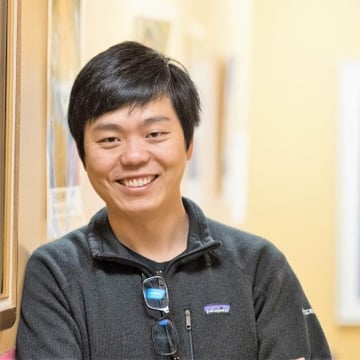
Yangqing Jia
Founder @ Lepton AI
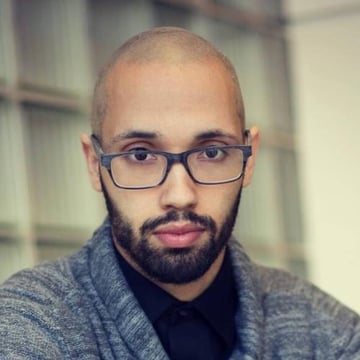
Chad Sanderson
CEO & Co-Founder @ Gable
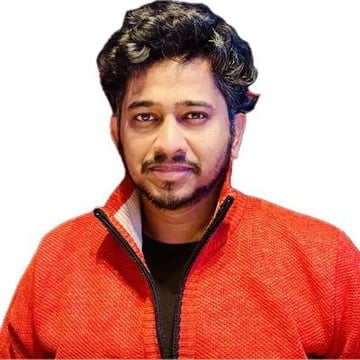
Vinoth Chandar
Founder/CEO @ Onehouse
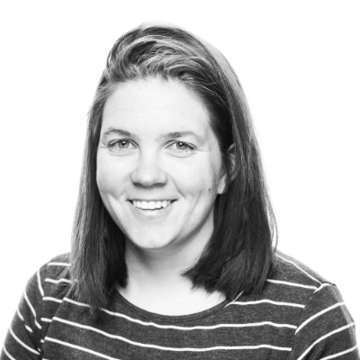
Miriah Peterson
Data Engineer @ Soypete tech
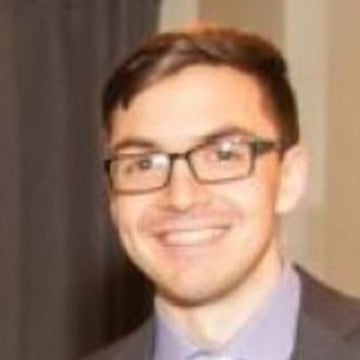
Benjamin Rogojan
Data Science And Engineering Consultant @ Seattle Data Guy
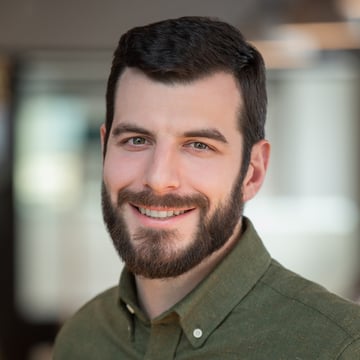
Michael Del Balso
CEO & Co-founder @ Tecton
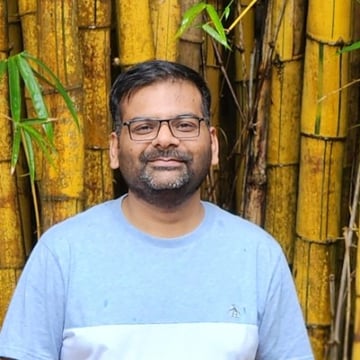
Pushkar Garg
Staff Machine Learning Engineer @ Clari Inc.
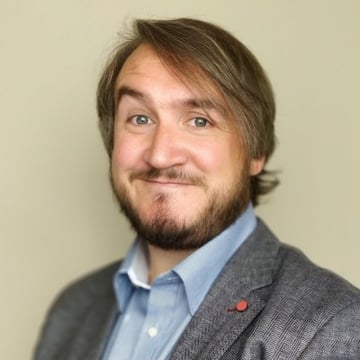
Simon Whiteley
CTO & Co-Owner @ Advancing Analytics
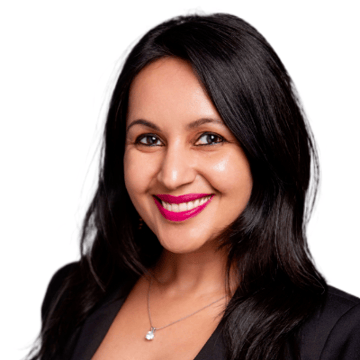
Shailvi Wakhlu
Founder @ Shailvi Ventures LLC
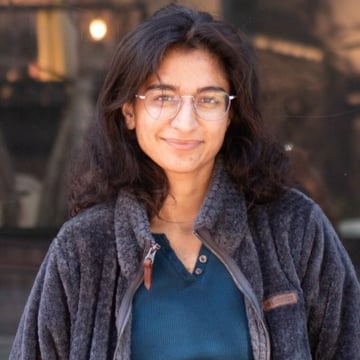
Aishwarya Joshi
Machine Learning Engineer @ Chime
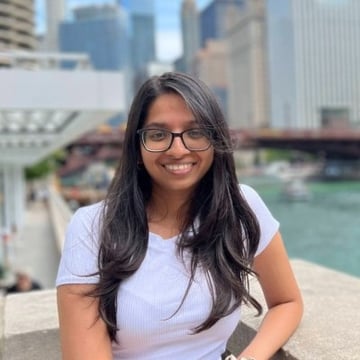
Aishwarya Ramasethu
AI Engineer @ Prediction Guard
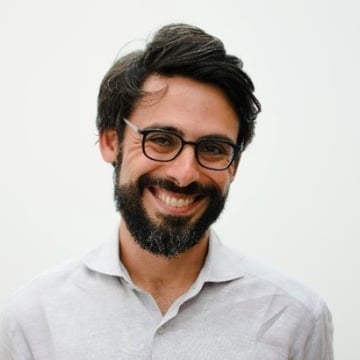
Ciro Greco
Founder and CEO @ Bauplan
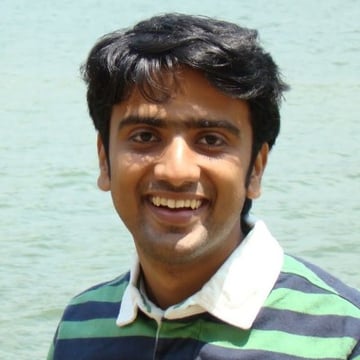
Sridhar Natarajan
Senior Software Engineer @ Intuit
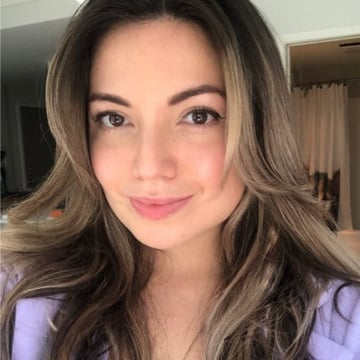
Daniela Santisteban
Product Manager - Data Taxonomies @ Numerator
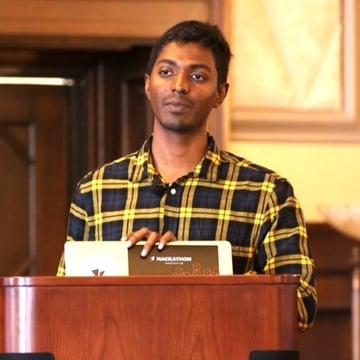
Nikhil Simha
CTO @ Zipline AI
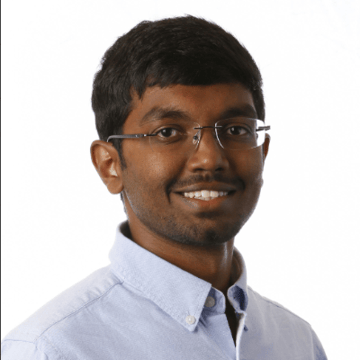
Sri Harsha Yayi
Product Manager @ Intuit
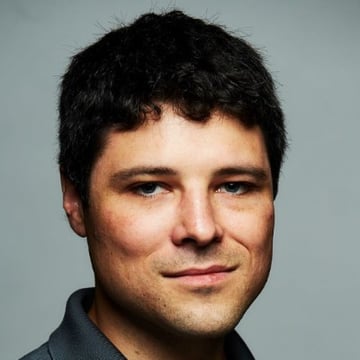
Tobias Macey
Associate Director of Platform and DevOps Engineering @ Massachusetts Institute of Technology (MIT)
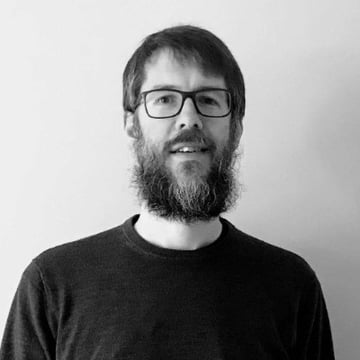
Alex Strick van Linschoten
ML Engineer @ ZenML
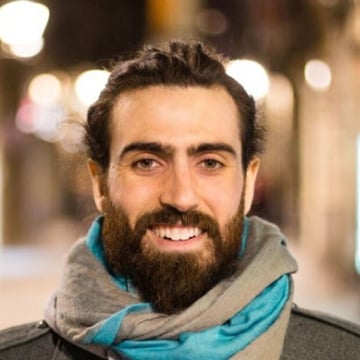
Demetrios Brinkmann
Chief Happiness Engineer @ MLOps Community
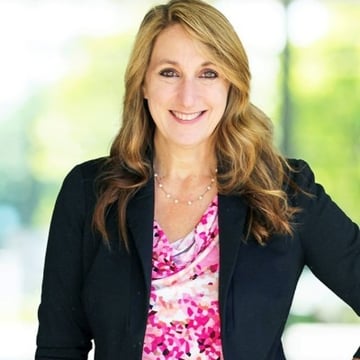
Beverly Wright
VP - Data Science & AI / CAIO @ Wavicle Data Solutions
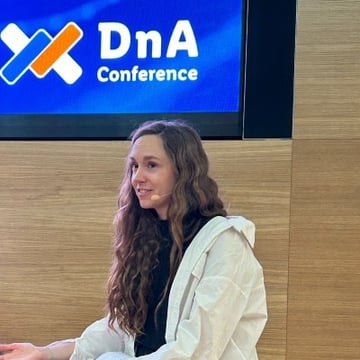
Rebecca Taylor
Tech lead: Personalization @ Lidl e-commerce
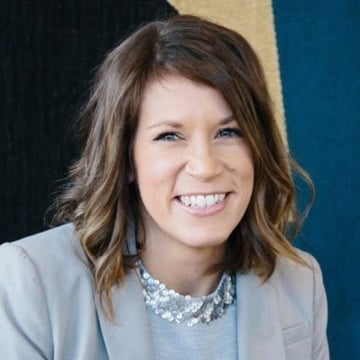
Emily Ekdahl
AI Ops Engineer @ Gusto

Jacopo Himberg
Director, Data @ Wolt
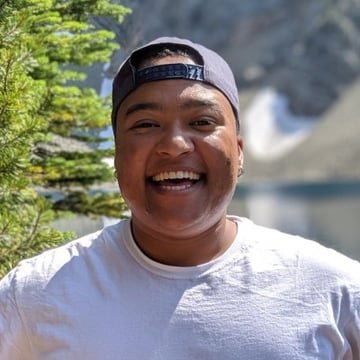
Devon Mittow
Staff Software Engineer @ Lyft
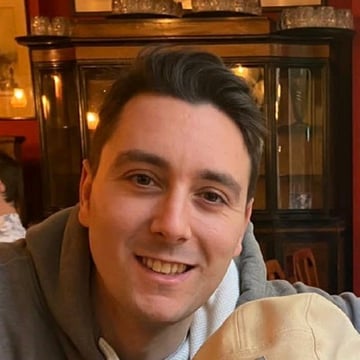
Jose Navarro
MLOps Engineer @ Cleo
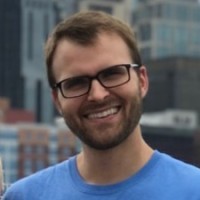
Stephen Bailey
Data Engineer @ Whatnot
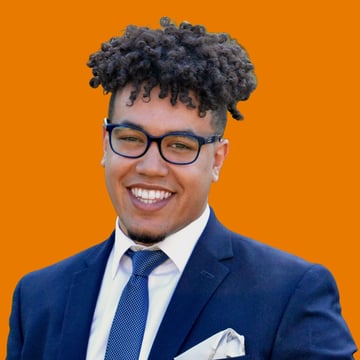
Mark Freeman
Tech Lead, GTM Engineering @ Gable
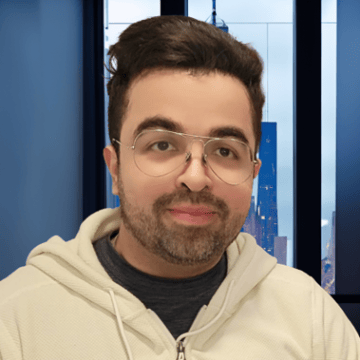
Victor Cuadros
Senior Software/Data Engineer @ Microsoft
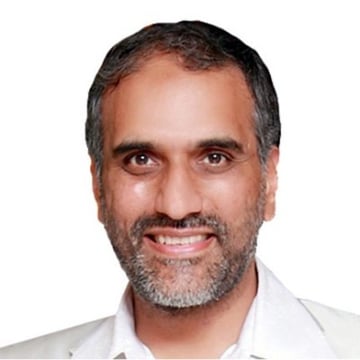
Akmal Chaudhri
Technical Evangelist @ SingleStore
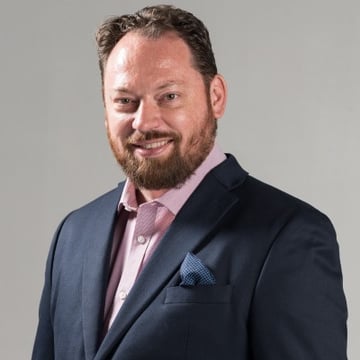
Jesse Anderson
Managing Director @ Big Data Institute
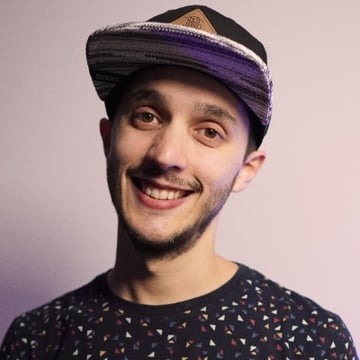
Mehdi Ouazza
Data Eng & Devrel @ MotherDuck

Michelle Leon
Staff Product Manager @ Databricks
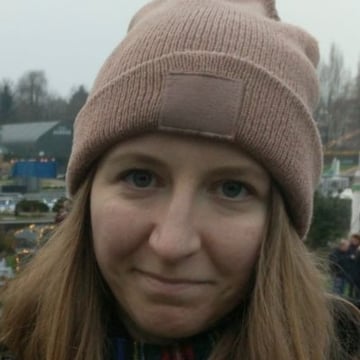
Victoria Bukta
Member of Technical Staff @ Databricks
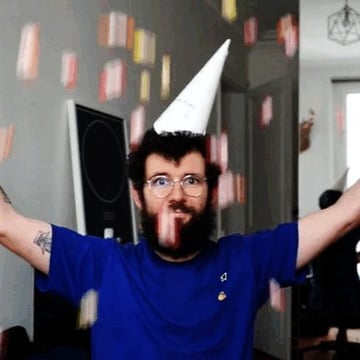
Christophe Blefari
CTO & Co-founder @ NAO
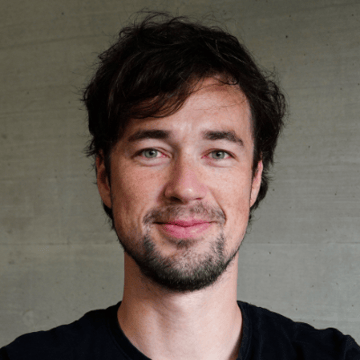
Daniel Svonava
CEO & Co-founder @ Superlinked
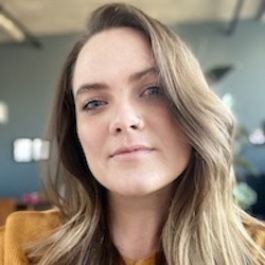
Maggie Hays
Founding Community Product Manager, DataHub @ Acryl Data
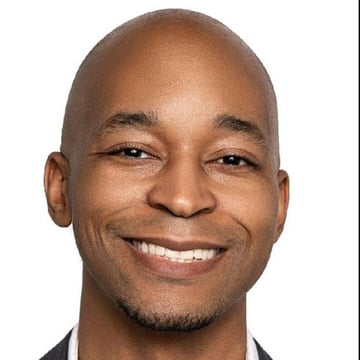
Korri Jones
Senior Lead Machine Learning Engineer @ Chick-fil-A, Inc.
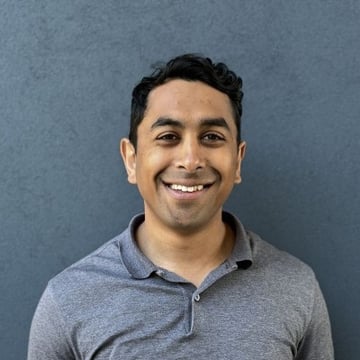
Nehil Jain
MLE Consultant @ TBA
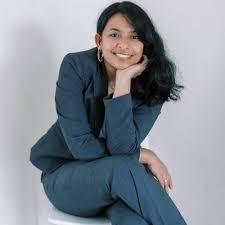
Sonam Gupta
Sr. Developer Relations @ aiXplain
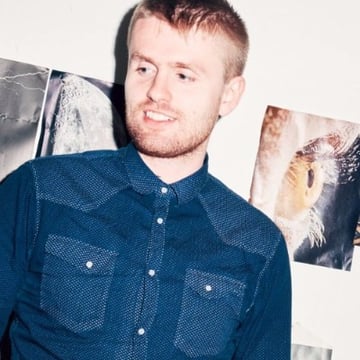
Valdimar Eggertsson
AI Developer @ Snjallgögn (Smart Data inc.)
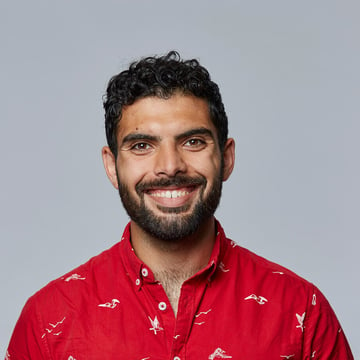
Simba Khadder
Founder & CEO @ Featureform
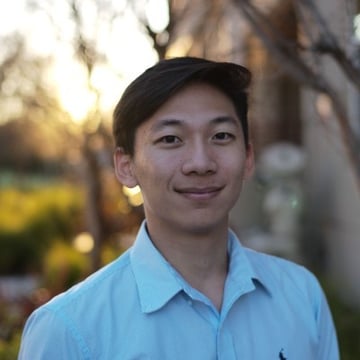
Jay Chia
Cofounder @ Eventual

Colleen Tartow
Field CTO @ VAST Data
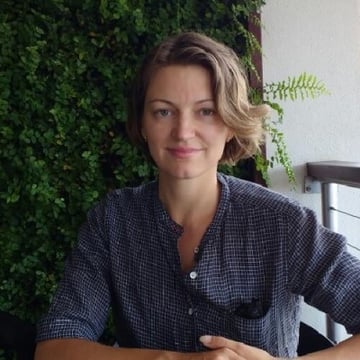
Elena Boiarskaia
Head of Applied Machine Learning @ Snorkel AI
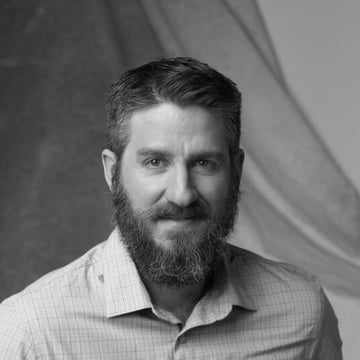
Ben Wilson
Software Engineer, ML @ Databricks
Agenda
Track 1
Track 2
Track 3
Track 4
1:00 PM, GMT
-
1:20 PM, GMT
Opening / Closing
Welcome - Data Engineering for AI/ML
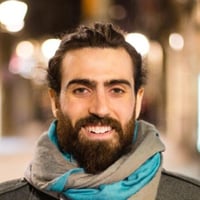
1:20 PM, GMT
-
1:45 PM, GMT
Keynote
11 lessons learned from doing deployments
+ Read More
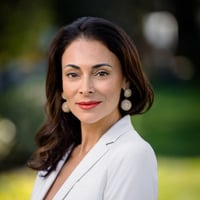
1:50 PM, GMT
-
2:15 PM, GMT
Presentation
Data Teams survey 2024
+ Read More
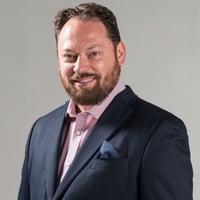
2:20 PM, GMT
-
2:45 PM, GMT
Keynote
Building Data Infrastructure at Scale for AI/ML with Open Data Lakehouses
+ Read More
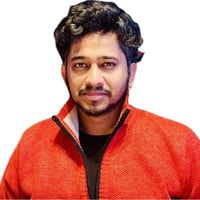
2:50 PM, GMT
-
3:15 PM, GMT
Presentation
Going Beyond Two Tier Data Architectures with DuckDB
+ Read More
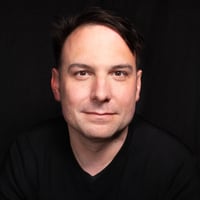
3:15 PM, GMT
-
3:35 PM, GMT
Break
The Booth Crawl
3:35 PM, GMT
-
3:45 PM, GMT
Lightning Talk
Data Infrastructure Cost: Tips to Keep Our CFOs Happy
+ Read More
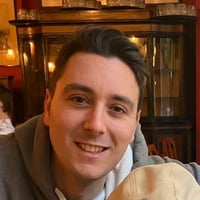
3:50 PM, GMT
-
4:15 PM, GMT
Presentation
Building Hyper-Personalized LLM Applications with Rich Contextual Data
+ Read More
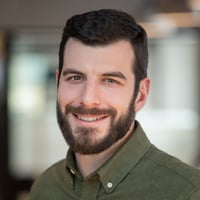
4:20 PM, GMT
-
4:45 PM, GMT
Presentation
The Daft distributed Python data engine: multimodal data curation at any scale
+ Read More
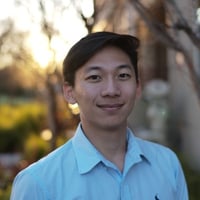
4:50 PM, GMT
-
5:15 PM, GMT
Presentation
How Feature Stores Work: Enabling Data Scientists to Write Petabyte-Scale Data Pipelines for AI/ML
+ Read More
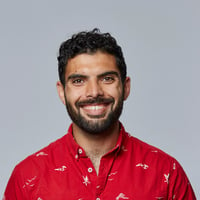
5:20 PM, GMT
-
6:00 PM, GMT
Roundtable
Guest Roundtable Discussion
6:05 PM, GMT
-
6:15 PM, GMT
Lightning Talk
DuckDB is fast for analytics, but what can it do for AI?
+ Read More
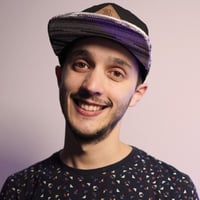
6:20 PM, GMT
-
6:30 PM, GMT
Lightning Talk
From Notebook to Kubernetes: Scaling GenAI Pipelines with ZenML
+ Read More
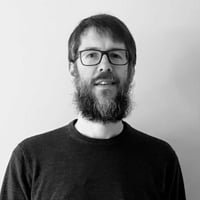
6:35 PM, GMT
-
6:45 PM, GMT
Lightning Talk
LLMs in Financial Services: Personalized Portfolio Recommendation Engines
+ Read More
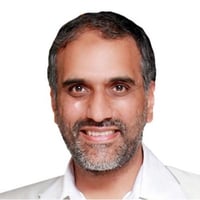
6:50 PM, GMT
-
7:15 PM, GMT
Presentation
Unified Data + AI Governance with Unity Catalog
+ Read More
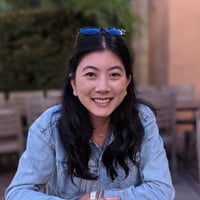
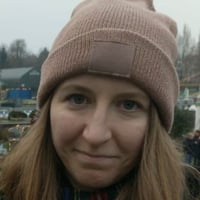
7:15 PM, GMT
-
7:35 PM, GMT
Break
Musical Entertainment By Yours Truly
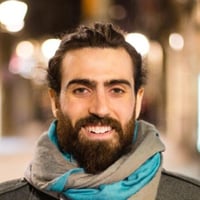
7:35 PM, GMT
-
8:05 PM, GMT
Panel Discussion
Data Scientists & Data Engineers: How the Best Teams Work
+ Read More
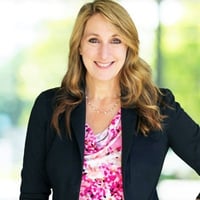
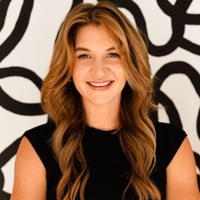
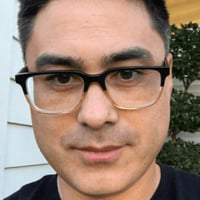
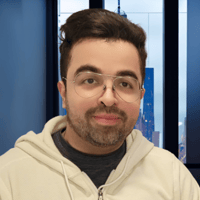
8:05 PM, GMT
-
8:30 PM, GMT
Keynote
Supercharging Your RAG System: Techniques and Challenges
+ Read More
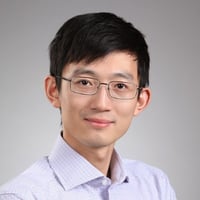
Event has finished
September 12, 1:00 PM, GMT
Online
Organized by
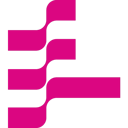
MLOps Community
Event has finished
September 12, 1:00 PM, GMT
Online
Organized by
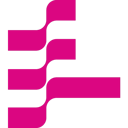
MLOps Community