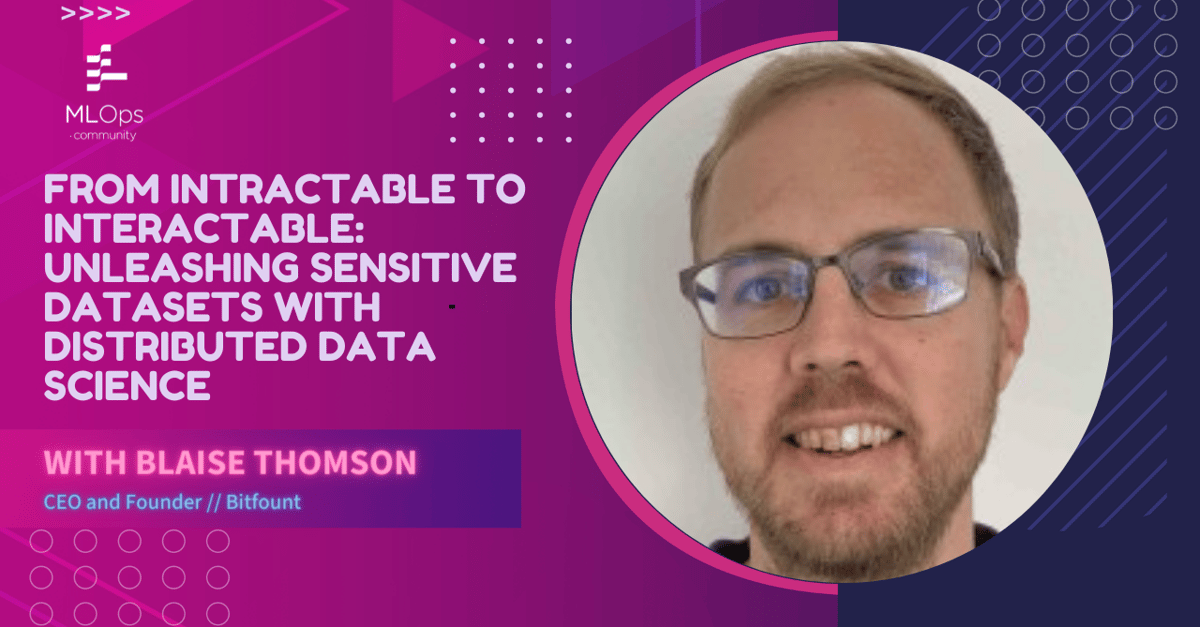
LIVESTREAM
From Intractable to Interactable: Unleashing Sensitive Datasets with Distributed Data Science
# Datasets
# Distributed Data Science
# Federated Machine Learning
Some of the most valuable data is also data that is not easily shared. Distributed data science is a new technique for overcoming this issue by leaving data ‘in place’; sending algorithms to the data. This enables data scientists to extract value from these datasets while ensuring strict privacy and security guarantees can be upheld.
In this talk, we’ll briefly introduce the fundamentals of distributed data science, including federated machine learning with additional privacy measures. We’ll then show how a new, easy-to-use platform can be used to easily train models at scale on sensitive datasets. We will also run through example experiments showing how without such approaches we simply cannot train ML models of sufficient quality.
Speakers
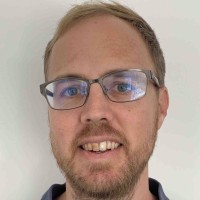
Blaise Thomson
CEO and Founder @ Bitfount
Agenda
Track View
4:00 PM, GMT
-
4:15 PM, GMT
Stage 1
Opening / Closing
Musical Intro
4:15 PM, GMT
-
4:55 PM, GMT
Stage 1
Presentation
From Intractable to Interactable: Unleashing Sensitive Datasets with Distributed Data Science
+ Read More
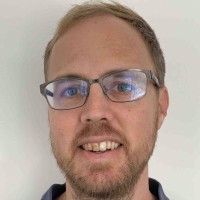
4:55 PM, GMT
-
5:00 PM, GMT
Stage 1
Opening / Closing
Q&A
5:00 PM, GMT
-
5:15 PM, GMT
Stage 1
1:1 networking
Networking
Event has finished
October 12, 4:00 PM, GMT
Online
Event has finished
October 12, 4:00 PM, GMT
Online