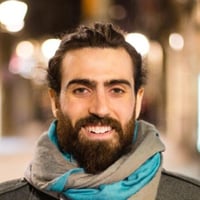
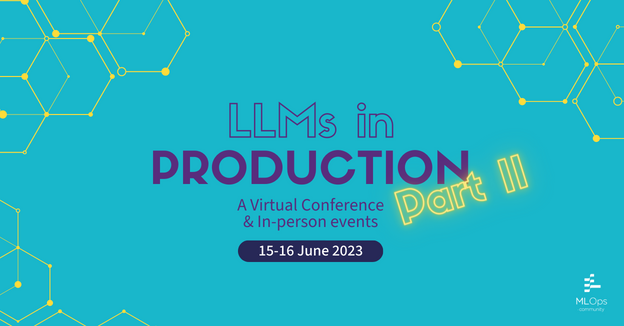
LLMs in Production - Part II
Large Language Models in Production Conference
Join us for two days of talking with some of our favorite people at the forefront of using LLMs in the wild, and an in-person workshop in San Francisco on how to build and deploy LLM based apps hosted by Anyscale.
There will be over 50 Speakers from Stripe, Meta, Canva, Databricks, Anthropic, Cohere, Redis, Langchain, Chroma, Humanloop and so many more.
This all started after we put together the LLM in-production survey and realized there are still lots of unknowns when dealing with LLMs, especially when dealing with them at scale. We open-sourced all the responses and we decided if no one was going to talk about working with LLMs in a non-over-hyped way, we would have to.
Let's discover how to use these damn probabilistic models in the best ways possible without sacrificing the necessary software design building blocks.
Expect all the fun and learnings from the first one. DOUBLED.
And remember, there will be some sweeeet sweet swag giveaways.
Huge Shoutout to all our sponsors of this event, find more info about them below.
Speakers
Agenda
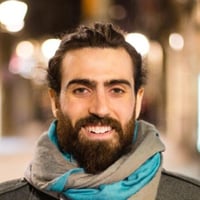
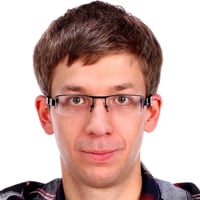
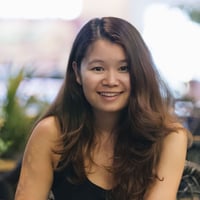
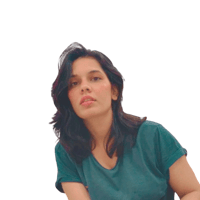
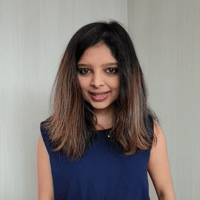
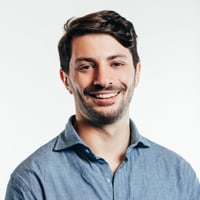
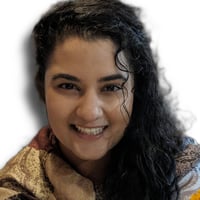
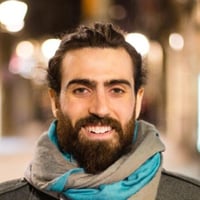
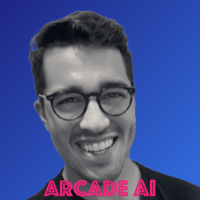
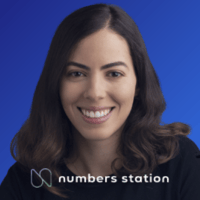
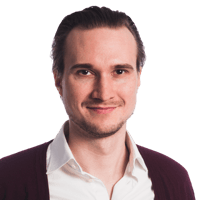
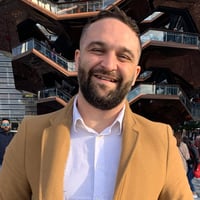
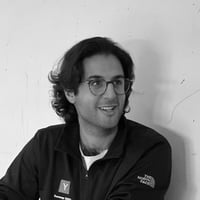
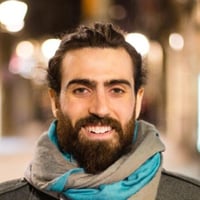
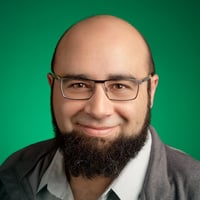
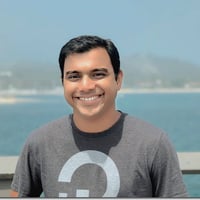
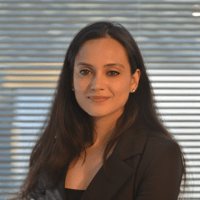
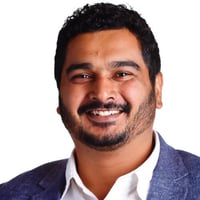
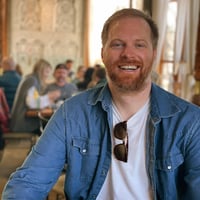
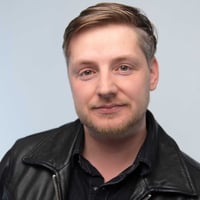
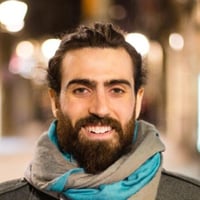
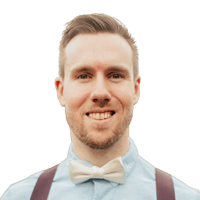
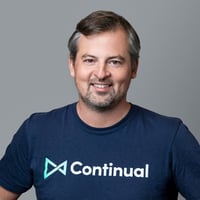
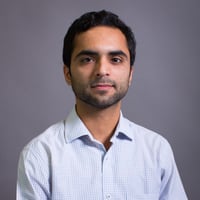
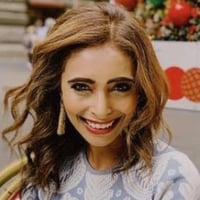
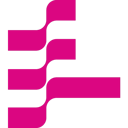
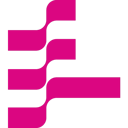