A Blueprint for Scalable & Reliable Enterprise AI/ML Systems
speakers
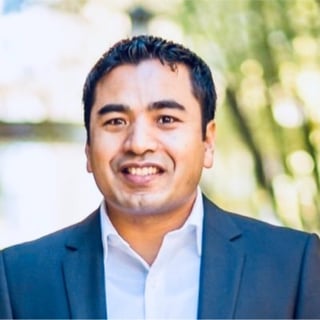
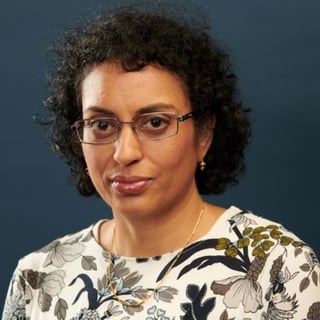
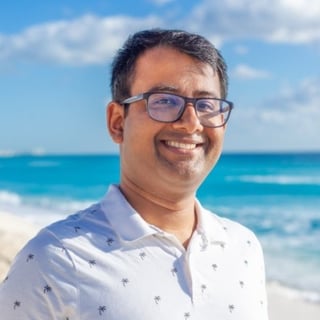
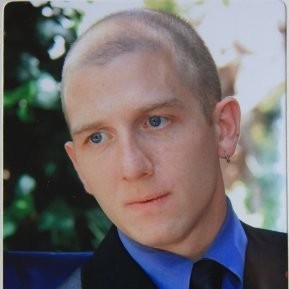
SUMMARY
Enterprise AI leaders continue to explore the best productivity solutions that solve business problems, mitigate risks, and increase efficiency. Building reliable and secure AI/ML systems requires following industry standards, an operating framework, and best practices that can accelerate and streamline the scalable architecture that can produce expected business outcomes. This session, featuring veteran practitioners, focuses on building scalable, reliable, and quality AI and ML systems for the enterprises.
TRANSCRIPT
PANEL 1 Host [00:00:10]: All right, we'll go ahead and get started here. So once again, our next topic is a blueprint for scalability, scalable and reliable enterprise AI ML systems. Please help me welcome to this stage our next four speakers, Hira Dangol with Bank of America, Rama Akkiraju with NVIDIA, Nitin Aggarwal with Google, and Stephen Eliuk with IBM.
PANEL 1 Host [00:00:33]: Come on up.
Hira Dangol [00:00:56]: Good afternoon, everyone. I hope each one of you are having incredible time out here at San Francisco based this AI quality conference that's happening. So super excited to be part of today's this phenomenal discussion. My name is Hira Dangol. I work for bank of America and I lead AI ML and automation platform development. So for the next 30 minutes, we will be spending on this specific topic called the blueprint for AI ML systems. Right. It's kind of like the interesting timing for us and how you can get some of the insights you interest, and information from my incredible panel out here from the enterprise landscape.
Hira Dangol [00:01:47]: So we'll try to see if we can get some time for Q and a towards the end. So I'm joined by my incredible panelist fellow from across this kind of the industry spectrum, Rama, who is from the Nvidia, and then Nitin. In fact, I think the slide needs to have changed. He just moved to the Microsoft, so it's more like Google to Microsoft kind of the transition phase. And then we have Steve from IBM. So without further ado, I think we can get started with some quick intro to begin with, to stay distant, and then we'll talk about the biggest kind of the blueprint, the focus of our discussion, to think about how vision and the strategy execution from the GNAI perspective. Maybe we'll cover a little bit about the more on the value proposition aspect of this blueprint, and then I. AI risk management is the incredible end of the most topic today.
Hira Dangol [00:02:50]: We need to focus on and then how this leads up to the adoption phase. Right. So with that, I think I'll let Rama to quickly introduce herself and what's the most fascinating things happening in Nvidia side. And I will take on the other side, too. Yeah.
Rama Akkiraju [00:03:05]: Thank you, Heera. Thanks for having me here. Can you all hear me okay? Yeah, great. My name is Rama Akkiraju. I lead the AI and automation team at Nvidia, specifically reporting to the CIO. My mission is to transform Nvidia's own enterprise and leverage as much of Nvidia's technology as possible along the way. So I look at not only it transformation, which is where I sit in the CI organization, but HR, sales, marketing, supply chain, finance, all of those business processes and business functions are up for transformation with AI and generative AI. So I work with all of those business functions to apply AI, generative AI.
Rama Akkiraju [00:03:49]: I look forward to sharing some of those lessons learned and experiences. I've been with NVIDIA for two years. Prior to that I was an IBM fellow. I worked at IBM for much of my career. I was the CTO for AI Ops and also in the initial phases of Watson development, led the Watson platform and natural language processing, speech recognition and all of those foundational AI services which we now take for granted. So that's about me.
Nitin Aggarwal [00:04:17]: Nitin, can you hear me? Hey everyone, I'm Nitin. The title that you see, that's my old life. So I currently lead the generative AI team for marketing at Microsoft. We are building various kind of systems, in a simpler terms you can call it as a copilot for marketing, focusing on content creation. We're talking about ideation, talking about analytics platform, creative assistants and so on and so forth. So that's a part of our team, including product managers and engineers. This particular topic is very close to my heart. It's about like the scalable and enterprise ML systems and the way.
Nitin Aggarwal [00:04:48]: And he asked that question, what's most fascinating? It's the consumer for these kind of systems. In the past, those were very boring systems typically being used in the form of an APIs integrated with some fancy UI, but with these kind of generative AI and LLMs, the AI systems, the core AI systems are being used by executives and business audience. So how those kind of a system has been evolved and they are evolving over a period of a time, that's what fascinates me a lot. So great to see you all.
Steven Eliuk [00:05:18]: A pleasure all. Steven Eliuk from IBM. I've spent the better part of seven years building out the corporate services for IBM in terms of data, data standards, data data governance, governance around AI and mlops and aiops and AI platforms, data privacy, doing all of that internally for our chief financial officer, all the way to our legal officer and to our privacy officers, building the services that make up the backbone for IBM, all the way through from corporate to software to consulting. So supercharging about 300,000 employees throughout the company, working across 150 plus countries, working across tens of thousands of AI and data assets, trying to aid in that consistent approach where you have a consistent platform for all users, you have a consistent governance structure for all users and all applications, and really trying to add as much automation to these plays as possible. So a lot of instrumentation, a lot of understanding as a practitioner, what goes into building out AI use cases and trying to take that know how to supercharge those that have not had the experience working in these domains. Now, before that, go back to things like Imagenet and sponsoring interesting peoples at interesting universities, and had the pleasure to work at Samsung electronics and built supercomputers and worked on deep learning and distributed deep learning and model parallel distributed deep learning. And just love that we're all here talking about this ever evolving space.
Hira Dangol [00:06:52]: All right, thank you, Steve. So today's topic as we were talking about blueprint for the scalable, reliable and the secure deployment of the AI system. We're talking about that, I think to begin the conversation on here, rama, I think, how would you make sure that the AI ML initiatives or the work that you drive aligns with the business objectives? I think you might be working mostly with the leaders out there. Right. So what are the kind of, the basic requirements are needed to have that kind of alignment and the buy in from the business objectives standpoint?
Rama Akkiraju [00:07:30]: Yeah, yeah, of course. That's where it all has to start, right? AI, or any technology for that matter, is a means to an end. That end is that the business, whatever business metric that you are trying to improve, you have to have a plan for how this technology that you're going to use is going to help you improve the state from where you are to the next state. So I can give some specific examples. We are working on applying AI generative AI for it transformation, and that includes it. Operational improvements could be improving SRE productivity. So how do we measure, so before we start these projects, we say, well, what are our current set of metrics that we are measuring? What is the baseline, the mean time to detect incidents, the meantime to resolve them, meantime to diagnose them? And how much is the business impact that's happening? How many incidents are happening within a month? So these are all the metrics that we take as baseline and say, okay, if we do incident summarization, if we did better detection of incidents, and if we implemented additional genai capabilities for SRE productivity in terms of chatbots or better integrations and better alert noise reduction capabilities, better anomaly detection capabilities, let's say how are all these metrics going to improve? And from time to time, with each iteration of the AI model deployments, we have to measure and see if we're making progress in the right direction to improve these business metrics or not. That's one example, and I can talk about other examples in employee productivity domain and supply chain domain.
Rama Akkiraju [00:09:03]: If planners are taking 5 hours, 3 hours to do what if scenario analysis each time, let's say we now have capability to do GPU optimization and apply the GPU optimization for redoing the planning. But with what if scenarios, now you can do it in three, five minutes. You know, what is the amount of time that we are saving for the planner? So that's another business metric, productivity metric. So we, for every project there is a baseline, a set of metrics, and then as we are making iterative improvements, we continue to measure those and see if you are making improvements or nothing. You know, it's easy to get carried away with technology and get excited. And sometimes, you know, you say, you think, you know, conversationalizing everything, building chatbots is the right way to do it. Maybe it is, maybe it isn't. If something can be done within one click, you don't want to make it like a five different turn conversation.
Rama Akkiraju [00:09:54]: In the end, the experience wears off after the initial month or two and people will say, why do I want to come here? You know, I just want one click and things should get done automatically or even maybe automated. No click is the right solution for it. So you really have to understand what is it that you're trying to optimize and what is the metric that you're going after and design and use technology accordingly for that purpose. It's a means to an end.
Hira Dangol [00:10:19]: Yeah, that's definitely help out, right. In terms of defining the use cases. And I think from the audience standpoint, our discussion will, most of our discussion will align to when we say AI ML system means there is two kind of distinct phases, right? There is a generative AI component and then we have traditional data centric model. That's how most of the enterprise are kind of currently deploying those kind of use cases out in the production. I think, Nitin, maybe you might be able to shed some lights based on your previous roles, working a lot of field work in terms of taking, if there is some sort of the best practices, taking any use cases from prototype to production, what involves in this kind of the phases, that would be something you can give some examples too, right? So for this could be helpful to us to understand.
Nitin Aggarwal [00:11:10]: That's an interesting question. So when talking about any production level systems, two key things that business audience are looking out for, either to gain some operating efficiencies or to get some new net revenue. These are the two key metrics that has been seen across any AI ML domain. We started with data analytics, back in 2012, 2013 moved into statistic analysis, we moved into machine learning, then deep learning, now genitive AI. These two metrics remains the same. When talking about production level systems, again, these two kind of a metrics start coming to the picture. Who is your end user? Is it a business user, is it a technical users, how you are integrating it, what kind of an accelerators, what kind of a platform that you are using it, what kind of a metric that you are currently monitoring? Metrics, it can be business metric, it can be technical metric. In the old ways, we used to say precision, recall, reference score accuracy, those things these days, we started replacing them with blue score, ru gel, everything but that kind of a metric.
Nitin Aggarwal [00:12:10]: But few metrics at the system level. For example, latency throughput, that remains the same. Interestingly, in my experience, people are getting little bit impatient. They used to wait for a few minutes, few seconds to make a call to their AI models, but with Genai, they need everything in real time. Hey, I'm typing it. I don't even type. I don't want to type. You should be able to understand what I'm saying and make a call and generate a response.
Nitin Aggarwal [00:12:35]: So those system level metrics are being changing. We used to say that, okay, 5979 of system availability, that might be going to ten nines. So even like a one failure in one year, that will be considered it as a deal breaker for those kind of systems and accelerators. So talking about some of the best practices, definitely going back to the first principles to whom you are building that particular system, what kind of a metrics that you are monitoring. And the second thing is that, okay, how robust and how futuristic it can be, just having a fancy layer of technology may not be able to help you out.
Hira Dangol [00:13:07]: Yeah, that's so true. I think everyone agree that data today is more like oil to economy. So without data, there is no AI solution, without data, there is no model or data centric models can be built. I think, Steve, maybe you can help us to kind of like uncover some of the insights around how the data silos can be managed to ensure that there is efficient and seamless integration that can happen across the enterprises, right? Today is very much fragmented across data infrastructure. What kind of like the concept and the governance piece could be involved there?
Steven Eliuk [00:13:50]: So just, I think it's the consistency of the practices, right? So everyone has a justification of why their data should be siloed. And sometimes it's rightfully so, sometimes it's customer data, where you can't have that data co mingled with other pieces of data, but it's really about the understanding of the data that needs to be consistent. And data standards really play a key role in that, because if you have the same level of standards, then you can search across that data in very consistent way. So let's say you're looking for certain aspects of privacy related data. If you have the same standards for privacy related data across the business, you're all measured against that same standard, right. Let's say you're trying to bring together similar types of data for a specific use case. You do searches across the standards. You can bring that data together.
Steven Eliuk [00:14:35]: Right? And we all know the data game, right? So the more data that you have, the better the models get. So that siloed approach is really problematic. You just basically want to be able to understand when there's restrictions around the data that might prevent you from, you know, taking it out into product or releasing to a customer or things like that. But I think the other really important thing to realize is that, like, it's never one and done. This stuff is changing all the time. There's new regulation that's coming. There's new use cases that are coming. This stuff is always evolving.
Steven Eliuk [00:15:03]: So think of the sustainability aspect. So don't make a decision today that will be really hard to implement tomorrow. So, like, think of regional constraints of data or sovereignty aspects that are coming from certain countries in the world. These are real constraints that will hit you. And it's not just about your data. There's country restrictions, there's geographical restrictions about how data can move throughout your own systems. So always understanding the implications of the data that you're working with and then the benefits of potentially consortiums of groups that come together as well. There's different ways that we can share data sharing weights.
Steven Eliuk [00:15:38]: You don't actually have to share the raw data. There's different ways that you can handle the same problem. It's about the awareness about, you know, how it plays in practice and latency constraints, too. Is it a latency aware problem? Do you have to bring that data close? Do you have to cache it? Like, all of the basis is, go back to understanding what the problem is and the use case that you're trying to reflect. Right. But again, inconsistent approaches at very large companies just doesn't scale. So at a small company, you might be doing your own things, but when you want to really scale things, consistent approaches across the company are really the ones that really matter. I can tell you probably, you know, hundreds of use cases internally that have unfolded just because these consistent practices.
Steven Eliuk [00:16:19]: You can think of financial reportings, you can think of normalization of it, metrics that are coming back if it's inconsistent. How do you commingle that data? How do you create a viable, you know, AI use case? Right? So consistency standards, this will help you with regulation, this will help you with your ROI and sustainability.
Rama Akkiraju [00:16:38]: You know, Hira, I want to add something to what you said. You know, all of those things hold, and we've noticed that in the world of Genai, there are a whole new set of interesting things that Genai exposes us to on the data dimension that we have to take care of. You know, these include sensitive, this is derivative risks with generative AI. So what do I mean by that? In enterprise, there are a lot of documents that are out there that are not properly protected. Now, with Genai, you have powerful tools with LLMs and vector databases to improve your search and to summarize all of that information. So now suddenly what starts to happen is that all of this data is now exposed and is searchable and findable. And so if you have any technical debt in terms of documents that are out there that are improperly protected, now everybody out there can find it, and that it starts to expose a lot of your, you know, risk, increase the risk of exposing sensitive data. And, and so there is new sets of things that come up every time when we advance the technology that we are noticing that we have to take care of this.
Rama Akkiraju [00:17:47]: So then we go actually apply generative AI technology itself to now classify sensitive documents and to automatically change the permissions on all of those enterprise documents that are improperly protected first before you can ingest and make all of these data searchable and findable. So there is another new kind of risk that's coming up. And also in order to establish trust associated with the responses and the suggestions that generative AI provides, you now have to provide citations, references and all of those things better and pointers back to the data with right kind of snippets and chunks. So I feel that, you know, we, there is a lot of stuff that's happening in data. All of those things are absolutely right on. But there is a whole new set of risks and interesting problems that we have to solve in the world of generative AI as it pertains to data. And we are only scratching the surface in finding those things out. And all these data platforms that are out in the industry will now start to also build some of these capabilities around finding sensitive data, classifying sensitive data, and having the right kind of controls guardrails for controlling the derivative risks associated with LLMs and generative AI.
Hira Dangol [00:19:01]: That's so true. Right. I think knowing data, where the data is coming from, who's generating data and where data sits, that's the crucial steps before you go into mlops or llmops with respect to gene AI, I think maybe that's where I think we need to spend more time talking about, you know, if you are talking about LLM ops, how do you know where to rag versus, you know, maybe fine tune or bring in some kind of like in house bring your own model kind of the view, right. And then you can do all kinds of evaluation to get ready for the production deployments. Right. And also traditional ML offs have similar kind of the viewfind, which is more like training of the model. Right. Where do you see the gap and the challenge that today we need to a better understand on those.
Nitin Aggarwal [00:19:48]: France that's an interesting concept. When talking about llmops, it's very different than mlops. And definitely these are my personal views. If you are cohort, I'd love to get your feedback. The concept of CI CD has changed. There is nothing called a CI, there's nothing called a CD in case of llmops, even if you're talking about model retraining, there is no concept of model retraining either. We are going for a rag based implementation, but we are going for a fine tuning the whole concept that, okay, hey, you will be getting that particular data training that model on, then you will be getting those metrics, comparing it, model management, and then talking about model versioning. That particular concept has been changed.
Nitin Aggarwal [00:20:27]: So the way that we are envisioning the llmops, the whole system has been changed by itself. So if we're talking about the kind of a system that are running in production in today's world that's dependent on data. I just want to quote one thing. One recent study published by Stanford Hai team and they did some analysis on the legal reports and they say that, okay, some of the results that they were getting from the LLM systems, they hallucinate more on the legal documents and the legal data as compared to the retail data or the marketing data. The question is obvious because these kind of a system, that kind of a data is not available in open source domain, you will start getting that particular feedback. The question is who will be providing that feedback? In that case, how the CD will look like and how the CI will be integrated back to the system. So if we're talking about those basic concepts, three basic concepts like CI CD and CT, those are getting changed with LLM coming into that particular picture. And I really cohere with that point that I think Stephen mentioned is about the data.
Nitin Aggarwal [00:21:29]: Definitely data is a moat, but at what point of a time, until what section it will be providing you that kind of a competitive advantage? That's not very clear the way that models are learning. Previously, some systems used to be calling us that, okay, this is a differentiating factor. Now they are not a differentiating factor. Now you need more data. Now you need more kind of a different nuance into that particular data so that things are changing and the whole system design for llmops are changing.
Hira Dangol [00:21:55]: I think at some point, let's say you are ready to deploy in a model or integrate the model output to some applications, right? There is a process involved. I think that from technology standpoint, CI CD integration, deployment layn out there. But I think in most of the enterprises, whether you are operating within the regulatory heavy spectrum versus none, I think maybe, Steve, what's your experience of deploying the model in the productions? What are the process involved in that to operate within the risk frameworks? Maybe you might be following more risk management functions or if it is kind of bringing the vendor solution in house, there is a tool gets involved. Do you have experience of like following those as risk framework and what is out there? I'll come back the same question to Rama as well, to understand, I think.
Steven Eliuk [00:22:47]: We take it back to the use case specific situation. What are the implications of that use case when things go wrong? We always take that approach to understand, like, there's definitely a workflow that we followed to build the use case and to understand its implications and the ROI there. But then what are the implications of when it gets it wrong? Basically. So in those types of cases, false positives, false negatives, all these, all these items propagate, right? So looking at those implications internally is really, really important. Now, normalizations of practices and continuously monitoring use cases as they go into production is critically important because what we often find is like a great release only to find in a week or two or a month, you know, it's no longer performing as the team expected it and they're already on to the next process. So building automation and tooling around, validating that it's doing what you think it's supposed to be doing. And usually what we found too, is that having the group who created the model create the criteria for the evaluation of the model is a bad idea, because often the criteria is like kind of self absorbed. They're trying to showcase something that it does really well.
Steven Eliuk [00:23:51]: You need an outside group to audit and validate and justify kind of the metrics that you're tracking. So continuously monitoring these releases to validate they are doing what you're doing, what you're expecting those releases to be doing. And then I think the other one would be like that I mentioned in the opening regards creating consistent platforms of releases, right? So if you do a consistent platform, like the same process and the same platform for your releases, then if you do need to augment something in that platform, then everything benefits from it. If you do it in a silo and you do an individual way that one project benefits from that know how, why don't you try to bring that across the organization? So the whole organization, you know, benefits from that. So I think consistency in the, in the platform approach that you're releasing on really understanding the, the implications of the use case and practice and then potentially outside views, an audit committee red teaming, things like that to validate and to justify how the model is performing.
Hira Dangol [00:24:49]: So let me ask this question a little bit slightly to your AMA, right? So from the timeline perspective, how long it does take to take the models, maybe like the traditional predictive models or generative AI kind of the models to production in your case, what's the kind of duration? Did it take like three months, six months or maybe weeks? What's kind of the duration view from like a non production environment to deploy in production?
Rama Akkiraju [00:25:16]: Well, it depends. It depends on what, what you're actually building and what kind of a model that is. Now if it's a, but for every model there are a set of processes that we have to follow. Testing is the one that takes the most amount of time. It's easy to build, especially with Genaii, easy to build proofs of concepts and prototypes and get super excited about deployments. But the road from pocs to pilots to actual production is a long one, especially in case of Genai. That's because, you know, in the case of traditional models, at least you can have, you know, what is false positive, what's true positive, false negatives. You can have ground truth data sets and you can measure the accuracy of the models.
Rama Akkiraju [00:26:02]: And so you kind of know if you have a threshold, say I want the accuracy to be at least 85, 90% for this use case and you have a good representative data set, you can test it against that. And if you pass the bar, you can put the model in production. In the case of Genai, let's take chatbot for example that you're building. The responses are natural language based. Right. Sometimes they're very subjective. And so creating ground truth and evaluating that ground truth is not an automated process. I mean, yes, you can use LLM itself as a judge or LLM as jury and measure, you know, how good the response is.
Rama Akkiraju [00:26:41]: But a lot of times humans have to really evaluate the answer, and that because it's a natural language answer, if it's creating a summary, and that makes it kind of human in the loop kind of a testing process, which increases the time it takes to take it to production. But overall, the principles are the same, whether it's classical AI versus generative AI. You have to start with a good representative test data set with good ground truth, and you are always measuring against that and to see how far you got and have some threshold to see if you have reached that threshold and if the users who are actually going to use it have tested it and they're comfortable with it. Yeah, I mean, in our case, it really varied. In the case of some of the chatbots that we deployed, it took maybe six to eight weeks to build the first version. But, you know, the road to deployment was long because we, our bot was answering questions that are sensitive, not because of anything that wrong that it's doing, but because of some of our sensitive documents had improper access controls. And so we had to stop that bot from actually getting deployed, put it still in early access testing, and first go fix the access control problems and documents. And imagine that is a long process.
Rama Akkiraju [00:28:01]: You know, in a company that may have, you know, hundreds and thousands of documents, fixing the access control permissions could be a long road.
Steven Eliuk [00:28:09]: Right.
Rama Akkiraju [00:28:09]: And you have to use different techniques to do that. So some of those bots haven't even made to production, but some of the more bots that are operating on open data that are more public, you know, we have them in production from six weeks. It went to production in three months. And so it really kind of depends on the domain, the use case and such. But the principles are the same. Test, test, test, evaluate accuracy thresholds.
Hira Dangol [00:28:32]: Yeah. And that's so fascinating. Right. I think in enterprise landscape, that's so true across, you know, regulatory heavy function because of the rigor and the testing and the RICS framework that you need to operate on any kind of model function that you need to put in the production because of the implications. Right. And there is responsible AI and AI governance come into play as well to support your kind of deployment schema. So, Nitin, I'll come back to you on terms of, like, now we set the production deployment, right. I think there is a beyond steps involved in that, which is kind of like monitoring, which is what we talk about.
Hira Dangol [00:29:10]: You know, do we monitor like performance level of the models, or we look at from like, governance angle, right. Which is kind of like, you know, in JNA space, we heavily talk about the responsible AI components, which is like, you know, there is a risk AI mean, risk management functions, things like the security, privacy and the compliance. And then there is whole piece of the, you know, standards of ethics. Right. The bias check. Biasness check. So where do you see that from the monitoring standpoint? We need to do at least the minimum of these components view as you deploy the models in production. And from the day one, you need to start supporting and monitoring those kind of the insights and the metrics around that.
Nitin Aggarwal [00:29:52]: Thank you so much for asking that question. So, I'm totally agreeing with what Rama mentioned about data. So we are working with some human labelers and they were saying, is that, okay, hey, you need to provide the feedback to a model. And we asked them a question that, okay, hey, you gave thumbs down to this thing, and you give thumbs up to this particular response. What's the reason? They say, I like it. Okay, be more specific. What do you like it say, this is more relatable. The question is, how will you make that subjective decision and quantify it so that the model will be able to understand the relativity and as well as the likeness for that particular output? And that's the biggest confusion.
Nitin Aggarwal [00:30:31]: That's the biggest question for the responsible AI. Some of the questions, and you're absolutely right. On the governance side, we are tagging some flags, like, for example, toxicity. We're talking about unfriendliness. We're talking about some sort of an adult content. There are some quantification of the factors currently available, but it's very hard to measure that thing, and that's really making it very hard to govern these kind of systems. So if we talk about any of the models, we're talking about Google, Gemini, talking about chat, GPT, talking about fi, talking about Sydney, any of those kind of systems, none of these systems, we can say that, okay, this is foolproof. Each and every of these systems failed to a certain extent.
Nitin Aggarwal [00:31:14]: The question is, which one got the more marketing and more visibility? That's another point. But are we saying, is that, okay, these kind of an organization have not built a robust framework, or they have not tested their system very well before launching? No. They must have gone through all those kind of processes but the challenge is the base technology is so subjective, it's so hard to measure and governing those things. So I'm very hopeful that in future, stronger, robust and quantified, responsible AI metrics will be coming to the picture. We will be able to govern these kind of models with better feedback and we will be able to generate those responses. But I feel it's not just the onus on the model creator, it's on the user as well. If you want to make this system or if you want to make this AIML model a success or not, in my experience, again, in my limited experience, I might be wrong. If I give a model to an individual and say that, okay, test it out, that person will test that model for all the use cases.
Nitin Aggarwal [00:32:13]: That model was not intended to accept everything inside the world. So the focus is more about, hey, I got that model. How can I make sure that, okay, this model is failing on those things and testing the limits and dimensions of those models other than making it work for certain use cases? So at this moment, I don't say that, okay, there is a one approach, there is one framework that's already available. There are multiple things that are available. Nexus flow is there. We're talking about like, Lang graph is coming up with some sort of a matrix around it. Kolina is like building up some, some sort of a system around it. The multiple system exists, but I can't see a system where I can say that, okay, this is the holy grail or the golden truth that you can use anywhere.
Nitin Aggarwal [00:32:57]: And if it's passing this particular test, you are good to go. So I'm very hopeful in future, just.
Steven Eliuk [00:33:02]: A really quick follow up, and I think we're over here, is that even if we could do everything and create that holy grail of, of a model, et cetera, today, tomorrow, it's going to be different. So the criteria, the eval, the reg, the information going into, it's going to change tomorrow. So just expect that. So if you're building a model, look at the frequency of the updates to the models, look at that, you know, feed that into your ROI to really understand the true cost. That creates a sustainability outlook for the model and the AI use case in practice. But, sir, I think you're trying to come on stage.
PANEL 1 Host [00:33:31]: I was just trying to say this is a great conversation. I would love to continue if we can wrap up with just about 30 more seconds. So we have one more speaker.
Hira Dangol [00:33:38]: Yeah, I'll ask last questions. I think this would be very interesting to learn from our panelists. Is like, what's the next big trends happening in the AI? Right? Are we going from like, classic RPA to copilot to agentic workflow or something else? Just maybe a couple of thoughts, Rama, starting from you, and then we'll wrap up.
Rama Akkiraju [00:33:59]: Yeah, I'll say. When Janai came out, everybody thought, oh, let's go build things with LlMsdev. Then it turned out it's not just LLMs. If you are building chatbots and such, retrieval is really critical. Getting the retrieval accuracy is the most important thing now. It's not just retrieval and LLMs, it's agentic workflow. So it's really the good old software engineering where you have good agents, good orchestration, that's bringing everything together with good retrieval and good LLMs. So the whole thing has to come together for it to work.
Rama Akkiraju [00:34:31]: That's my experience, shifting from LLMs to, you know, retrieval to agentic workflows.
Nitin Aggarwal [00:34:39]: I see LLMs or genai as an orchestrator, as a tool for automation, whether it's agents, chatbots, anything, in such a way that anybody can use AI, it's not just touted for some data scientist or engineers, just making things simple, just really quickly.
Steven Eliuk [00:34:56]: Yeah, I think the agentic view is really an important one. Those agents that are able to do real tasks within the companies, and then I think just the general access and understanding of general data. I think we are able to access data like we've never been able to access data before, both internally and externally. And this is just going to get better over the next couple of years. And ultimately, as a society, we're going to get better. I just hope we don't lose our original thought. Okay, so that's good.
Hira Dangol [00:35:22]: All right, thank you. I think we're on the top of the hour. Thank you, all the panels for your incredible naval insights to our audience and everyone, for joining us on this session. Thank you very much.
Nitin Aggarwal [00:35:32]: Yeah, thank you. Thank you.
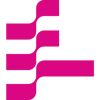