Agentic Relationship Management
speaker
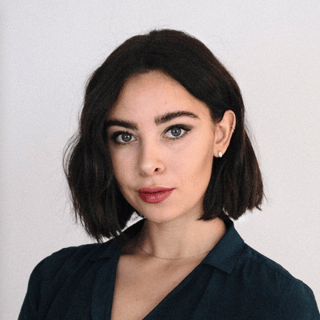
Currently I'm working on Hearth AI in NYC & SF ⚒️ - to build out agentic relationship management. Previously I worked as an Engineering Manager at Schmidt Futures and in Democratic politics. Before that I worked at Apple, Airbnb, the Bridgestone World Solar Challenge, Pear VC, NASA Ames, Facebook, and Stanford.
SUMMARY
Today, our personal and professional networks have reached an unprecedented level of complexity. The last two decades of tech innovation have connected us to more people, across more communication channels, spanning a wider range of contexts than ever before. Our growing networks have become intractable to manage as individuals, let alone teams.
TRANSCRIPT
Hello everyone. My name is Ashe Magalhaes and I'm the founder of Hearth A I. Um, above is my Twitter. Um, pretty responsive on there. So if you have any questions, uh, please call me. Um, so I'm gonna talk about agentic relationship management. Um, Hearth is still pretty stealthy, but here's our website with our waitlist, um, if you're interested. Ok, sounds good. Hello everyone. My name is Ash Magali and I'm the founder of Hearth A I. Um, above is my Twitter. Um, pretty responsive on there. So if you have any questions, uh, please call me. Um, so I'm gonna talk about agentic relationship management. Um, Hearth is still pretty stealthy, but here's our website with our waitlist, um, if you're interested. Ok. So I know this is a lightning talk. I'm gonna try to move pretty quickly. Um, and it was also meant to set a timer to make sure I was doing that. I'm gonna talk about, um, who am I, uh, who I'm assuming you are? What is, what do we mean by agentic? What do we mean by agentic relationship management and a case study that talks about a specific user flow we think about at heart, our system, um, some production benchmarks, we think through the mission of Hearth and then finally careers because we're hiring. Ok. So I know this is a lightning talk. I'm gonna try to move pretty quickly. Um, and it was also meant to set a timer to make sure I was doing that. I'm gonna talk about, um, who am I, uh, who I'm assuming you are? What is, what do we mean by agentic? What do we mean by agentic relationship management and a case study that talks about a specific user flow we think about at heart, our system, um, some production benchmarks, we think through the mission of Hearth and then finally careers because we're hiring. Ok. So who am I? Um, here is my personal website at A dot um A I which is cool. I got the A I Domain a while ago. Um, so I'm actually based here in New York City. I've lived here in New York, in Palo Alto, San Francisco in Berlin and in Edinburgh, I've been working in the machine learning space for about a decade now. I did some early internships at, Ok. So who am I? Um, here is my personal website at A dot um A I which is cool. I got the A I Domain a while ago. Um, so I'm actually based here in New York City. I've lived here in New York, in Palo Alto, San Francisco in Berlin and in Edinburgh, I've been working in the machine learning space for about a decade now. I did some early internships at, I worked for NASA. Um I worked at M L Airbnb and Apple. Uh I spent two years building and racing a solar powered car which was pretty cool. I went to Australia to race it and was there for a while. And then um I spent two years working in American politics as a machine learning engineer in 2020. Um and then went to Eric Schmidt's philanthropy after and um have now started hears about a year ago. So my main mission is really to build A I that augments the human experience. I worked for NASA. Um I worked at M L Airbnb and Apple. Uh I spent two years building and racing a solar powered car which was pretty cool. I went to Australia to race it and was there for a while. And then um I spent two years working in American politics as a machine learning engineer in 2020. Um and then went to Eric Schmidt's philanthropy after and um have now started hears about a year ago. So my main mission is really to build A I that augments the human experience. Um So this talk kind of assumes that you all are builders and you're currently working in A I and you're looking to learn more about our technical approaches to agentic products. Um So this talk kind of assumes that you all are builders and you're currently working in A I and you're looking to learn more about our technical approaches to agentic products. OK. So what is um kind of the problem we think about is that today, our personal and professional networks have reached an unprecedented level of complexity. Like even for this chat, I would love to have all of you automatically added to my linkedin as a Twitter as a kind of systematized keeping in touch because you all are interested in A I um as I am, but that would actually be really hard to do. Um So, you know, even today, despite being glued to our screens, we're still dropping relevant communication threads, wasting time on manual data entry tasks. So OK. So what is um kind of the problem we think about is that today, our personal and professional networks have reached an unprecedented level of complexity. Like even for this chat, I would love to have all of you automatically added to my linkedin as a Twitter as a kind of systematized keeping in touch because you all are interested in A I um as I am, but that would actually be really hard to do. Um So, you know, even today, despite being glued to our screens, we're still dropping relevant communication threads, wasting time on manual data entry tasks. So a lot of us have like spreadsheets or notion databases. We're tracking people on, we're losing key network details. We're failing to match the right people with the right opportunities at the right time and we're letting our important relationships grow cold. So the solution that we think about is agentic relationship management. Um And this is a suite of products that leverage A I agents to learn from, understand and act on behalf of the user. So A I will synthesize and manage your network complexity so that you can focus on connection. a lot of us have like spreadsheets or notion databases. We're tracking people on, we're losing key network details. We're failing to match the right people with the right opportunities at the right time and we're letting our important relationships grow cold. So the solution that we think about is agentic relationship management. Um And this is a suite of products that leverage A I agents to learn from, understand and act on behalf of the user. So A I will synthesize and manage your network complexity so that you can focus on connection. So what do we actually mean by agentic? Um These are A I systems that leverage large language models that would be the simulated world over here and human feedback to determine which actions to take and in which order kind of the key here is that these products are executing a series of observations, thoughts and actions to meet an objective. And we'll kind of talk through how we're thinking about that in a few slides. So what do we actually mean by agentic? Um These are A I systems that leverage large language models that would be the simulated world over here and human feedback to determine which actions to take and in which order kind of the key here is that these products are executing a series of observations, thoughts and actions to meet an objective. And we'll kind of talk through how we're thinking about that in a few slides. So what do we mean by a genetic relationship category? Uh management? Um So we kind of see this as a new category and in this world, agents are A I systems that are personalized to a persona, think like a sales person, partnerships B D capital allocator, um A specific team or an individual through communication and social network integrations and their objective is to maximize different network work flows. So So what do we mean by a genetic relationship category? Uh management? Um So we kind of see this as a new category and in this world, agents are A I systems that are personalized to a persona, think like a sales person, partnerships B D capital allocator, um A specific team or an individual through communication and social network integrations and their objective is to maximize different network work flows. So what do I mean by network work flows? Um The world of what this could look like is pretty vast. Um Some examples include, you know, which of my relationships are growing cold. You know, I'm in a new city. I'm traveling to Austin Seattle, who should I meet with, who are the people in this sales meeting that I'm about to go on? Um What is my network news today? So if I'm a capital allocator and my portfolio company hires a new candidate, you know, I should know that and I congratulate my team. what do I mean by network work flows? Um The world of what this could look like is pretty vast. Um Some examples include, you know, which of my relationships are growing cold. You know, I'm in a new city. I'm traveling to Austin Seattle, who should I meet with, who are the people in this sales meeting that I'm about to go on? Um What is my network news today? So if I'm a capital allocator and my portfolio company hires a new candidate, you know, I should know that and I congratulate my team. Um So auto add conference attendees to my socials that would be really relevant here. And then who does my teammate know that I should actually know? Um So auto add conference attendees to my socials that would be really relevant here. And then who does my teammate know that I should actually know? OK, so we'll narrow this to kind of one case study and that is this user flow for keeping relationships warm. So here, Hearth sends me a daily briefing essentially saying, you know, here's Jody, you're about to go on an investor intro call. Here's her linkedin her Twitter, here's kind of the summary OK, so we'll narrow this to kind of one case study and that is this user flow for keeping relationships warm. So here, Hearth sends me a daily briefing essentially saying, you know, here's Jody, you're about to go on an investor intro call. Here's her linkedin her Twitter, here's kind of the summary what we have on her and here are some uh mutuals. And then interestingly, I might tell Hearth, hey, keep my relationship with Jody warm and then Hearth lets me know if there are no communications exchanged. Um On May 10th, uh Hart will ping me and I'll remember to like reach out to Jody and that's kind of an example of like this agentic structure within the user flow is one case study. what we have on her and here are some uh mutuals. And then interestingly, I might tell Hearth, hey, keep my relationship with Jody warm and then Hearth lets me know if there are no communications exchanged. Um On May 10th, uh Hart will ping me and I'll remember to like reach out to Jody and that's kind of an example of like this agentic structure within the user flow is one case study. Um So what does this actually mean for our agent? Um Here's where we kind of step through levels of like thoughts, actions and observations. So we start with the user query of like keep this relationship with Jody Worm. The agent thinks, you know, I need to keep this relationship with Jody Worm. And then we'll move to an action model to see if this fits into a hard action workflow or if it should be somewhere else. So it does look like it's a hard action workflow, it's something we support. So the next level is who is Jody, like who is that Um So what does this actually mean for our agent? Um Here's where we kind of step through levels of like thoughts, actions and observations. So we start with the user query of like keep this relationship with Jody Worm. The agent thinks, you know, I need to keep this relationship with Jody Worm. And then we'll move to an action model to see if this fits into a hard action workflow or if it should be somewhere else. So it does look like it's a hard action workflow, it's something we support. So the next level is who is Jody, like who is that in the knowledge base? And that would be then be a search on Jody and kind of the context which in this case is the briefing or the nudge. And then from the previous context, we identify like Jody's full name. And then that third level is, you know, I need to determine when this relationship is, is cold and kind of warn the user. So from there, Harth would have a relationship model where it's basically coming up with an amount of time that might pass before this relationship is cold. And you can imagine like starting pretty naive with, in the knowledge base? And that would be then be a search on Jody and kind of the context which in this case is the briefing or the nudge. And then from the previous context, we identify like Jody's full name. And then that third level is, you know, I need to determine when this relationship is, is cold and kind of warn the user. So from there, Harth would have a relationship model where it's basically coming up with an amount of time that might pass before this relationship is cold. And you can imagine like starting pretty naive with, you know, maybe people are important to me that I spent a lot of time with and then growing in complexity to what we mean by like an underlying preference model. Um And then finally, there's a reminder system or workflow where if there's no communication Harth will then send me a slack message. And uh let me know to reach out. you know, maybe people are important to me that I spent a lot of time with and then growing in complexity to what we mean by like an underlying preference model. Um And then finally, there's a reminder system or workflow where if there's no communication Harth will then send me a slack message. And uh let me know to reach out. So kind of visually what does this mean? It means that users really only see the slack dynamic. But behind the scenes, there's this hearth agent which is then taking the query and putting it in a query model which it then determines, you know, is this search through a document store? Is it an action that we support or is it feedback um around like a So kind of visually what does this mean? It means that users really only see the slack dynamic. But behind the scenes, there's this hearth agent which is then taking the query and putting it in a query model which it then determines, you know, is this search through a document store? Is it an action that we support or is it feedback um around like a previous interaction that's telling me I did a good or bad job. And so if it is an action model from there, uh like we discussed, we would then kick it to a relationship model, determine the amount of time that should be passed and then have a reminder workflow that's running. And if it's not, we have search um which can then query hearts enriched contact system. That's refreshing. previous interaction that's telling me I did a good or bad job. And so if it is an action model from there, uh like we discussed, we would then kick it to a relationship model, determine the amount of time that should be passed and then have a reminder workflow that's running. And if it's not, we have search um which can then query hearts enriched contact system. That's refreshing. OK. So there is a lot to test in production and I think a lot of us are all working through this at the same time. And that means our team really focuses on kind of a benchmark and test suite that captures a lot of this. So prompts um this needs pretty rigorous aval setup. Uh It's kind of wild to see what seems like minor changes in prompts, really changing the output um embeddings vector stores. Um I would urge everyone to like watch OK. So there is a lot to test in production and I think a lot of us are all working through this at the same time. And that means our team really focuses on kind of a benchmark and test suite that captures a lot of this. So prompts um this needs pretty rigorous aval setup. Uh It's kind of wild to see what seems like minor changes in prompts, really changing the output um embeddings vector stores. Um I would urge everyone to like watch out for sneaky costs, which is something our team has had to work through this week. And the whole landscape of vector stores is changing really quickly. Um especially when we talk about like production level data augmenting. So again, even simple things have had a big effect for us, like indicating chronological order for meetings um as a feature um results in like better outputs or more sensical outputs from our model. Um an agent's ability to self refine um and search action feedback loop is really important out for sneaky costs, which is something our team has had to work through this week. And the whole landscape of vector stores is changing really quickly. Um especially when we talk about like production level data augmenting. So again, even simple things have had a big effect for us, like indicating chronological order for meetings um as a feature um results in like better outputs or more sensical outputs from our model. Um an agent's ability to self refine um and search action feedback loop is really important and how this persists in memory. Um So I know B A G I has made a lot of news this week and it's really cool. And when we think about like what is a long term feedback loop look like where the model is retaining the user preferences over like weeks. It's really interesting. Um And then a vows on stacking L M. So kind of going back to our visual. This is actually a suite of many different models and we want to make sure our benchmarking covers like their interactions. The key insight here is like the agentic Deb ecosystem is very new and how this persists in memory. Um So I know B A G I has made a lot of news this week and it's really cool. And when we think about like what is a long term feedback loop look like where the model is retaining the user preferences over like weeks. It's really interesting. Um And then a vows on stacking L M. So kind of going back to our visual. This is actually a suite of many different models and we want to make sure our benchmarking covers like their interactions. The key insight here is like the agentic Deb ecosystem is very new and the longer term actually calls for agent monitoring systems in the wild as this action layer grows. So right now, the action is pretty benign. It's a reminder system in the future. When it's integrated with other platforms or socials, we want, you know, a lot more monitoring and ability to kind of call things and the longer term actually calls for agent monitoring systems in the wild as this action layer grows. So right now, the action is pretty benign. It's a reminder system in the future. When it's integrated with other platforms or socials, we want, you know, a lot more monitoring and ability to kind of call things back or have a human in the loop. So especially if it's like auto ad folks on Twitter, that's something we'd want more sophistication around. And then uh I wanted to plug John Na's work who I think is amazing. And he's thinking a lot about these like monitoring systems in the wild as they pertain to the legal space, which I think is fascinating. back or have a human in the loop. So especially if it's like auto ad folks on Twitter, that's something we'd want more sophistication around. And then uh I wanted to plug John Na's work who I think is amazing. And he's thinking a lot about these like monitoring systems in the wild as they pertain to the legal space, which I think is fascinating. OK. So just to kind of take a step back, the mission of KTH is to build a new category of a genetic relationship management products that do uh four things that centralize, that enrich on your contacts that recommend and that act on your network. Um So you and your team can focus on connecting. OK. So just to kind of take a step back, the mission of KTH is to build a new category of a genetic relationship management products that do uh four things that centralize, that enrich on your contacts that recommend and that act on your network. Um So you and your team can focus on connecting. Um And finally, we're hiring for an M L chief scientist and another M L function here in New York City. So come work with us and please reach out if you have any questions. OK. Thank you everyone. Um And finally, we're hiring for an M L chief scientist and another M L function here in New York City. So come work with us and please reach out if you have any questions. OK. Thank you everyone. Oh OK. I see a question from the chat. How are L L MS helping you manage the risk of this self spamming? Like Google email, self reminders. Um The answer is even before we have that L L M layer, we have like a pretty thorough processing. Um Even when we think about meeting, Oh OK. I see a question from the chat. How are L L MS helping you manage the risk of this self spamming? Like Google email, self reminders. Um The answer is even before we have that L L M layer, we have like a pretty thorough processing. Um Even when we think about meeting, we're focused on meetings with other attendees in them. So we actually haven't gotten into like an L L M processing out emails level yet we've been able because we're focused on outbound network to I think, minimize that risk so far with our private data. we're focused on meetings with other attendees in them. So we actually haven't gotten into like an L L M processing out emails level yet we've been able because we're focused on outbound network to I think, minimize that risk so far with our private data. But I think it's a super interesting space and I think um so many of our products will be like stacked L L MS um determining other agentic work flows in the future. But I think it's a super interesting space and I think um so many of our products will be like stacked L L MS um determining other agentic work flows in the future. Awesome. Thank you so much. I think that was great. I'm not seeing any other questions at the moment. So we might be able to let you go. Um, are you based in San Francisco or New York? I miss Awesome. Thank you so much. I think that was great. I'm not seeing any other questions at the moment. So we might be able to let you go. Um, are you based in San Francisco or New York? I miss that. I'm based in New York City but I'm in San Francisco, like, once a month. But I'm mostly here. So reach out. Oh, that. I'm based in New York City but I'm in San Francisco, like, once a month. But I'm mostly here. So reach out. Oh, cool. Nice. You should, there is the next MLS community meet up in New York is on the 27th. So you should try and join speak at one. I think that would be so cool. Nice. You should, there is the next MLS community meet up in New York is on the 27th. So you should try and join speak at one. I think that would be so fun. fun. Yeah, that sounds good. Yeah, that sounds good.