AI Agents for Consumers
speakers
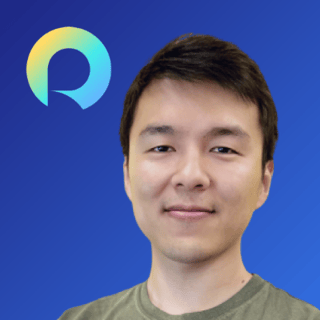
Shaun is the co-founder and CEO of HeyRevia, an AI company transforming the way healthcare providers manage complex phone interactions. With a background at Google Assistant and experience leading autonomous vehicle projects at Pony.ai, Shaun brings deep expertise in AI to his current venture. HeyRevia aims to streamline healthcare processes by automating tedious phone calls, making providers' lives easier and more efficient. Backed by Y Combinator (S24), Shaun and his team are committed to pushing the boundaries of AI voice technology to empower both healthcare professionals and patients.
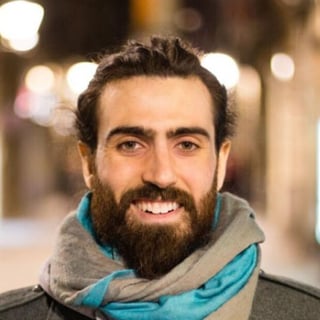
At the moment Demetrios is immersing himself in Machine Learning by interviewing experts from around the world in the weekly MLOps.community meetups. Demetrios is constantly learning and engaging in new activities to get uncomfortable and learn from his mistakes. He tries to bring creativity into every aspect of his life, whether that be analyzing the best paths forward, overcoming obstacles, or building lego houses with his daughter.
SUMMARY
Explore the groundbreaking work RealChar is doing with its consumer application, Rivia. This discussion focuses on how Rivia leverages Generative AI and Traditional Machine Learning to handle mundane phone calls and customer service interactions, aiming to free up human time for more meaningful tasks. The product, currently in beta, embodies a forward-thinking approach to AI, where the technology offloads day-to-day burdens like scheduling appointments and making calls.
TRANSCRIPT
Shaun Wei [00:00:00]: I'm Shaun Wei. I'm the CEO of real char, and I take my morning coffee with oat milk. I do half, half coffee, half milk. I do canned sugars, and I like to light my days up and have little bit sweet in my mouth.
Demetrios [00:00:27]: What's going on? Everyone? Welcome back to another Mlops community podcast. I am your host, Demetrios. And today we're talking with Sean all about what he is working on, his product, Rivia, and the company realchar. Oh, my God, I love it. I'm so excited by what he's doing because it is a consumer application using genai and traditional ML, and he basically pulls open the curtain to show us what and how they are combining the two. I cannot say I fully understand what exactly he's doing, but he broke down for me after we stopped recording, of course, this idea on how in robotics, it's very common to run everything in parallel and look at something. I think he called it design Os. And everything needs to be done in parallel so that you can scale and so that you can do things quickly.
Demetrios [00:01:35]: Now, his product is a consumer app that you can basically use AI to call different customer service lines and have AI take a your customer service burdens off your shoulders. So we as consumers are getting put up to the task of having to deal with a bunch of shitty AI. Why don't we fight back by giving all of these shitty implemented AI, probably a little bit half baked AI solutions that are everywhere with enterprises and companies. Why don't we start to implement our own? And so that's the idea. You get AI to make calls for you, wait over 3 hours on a customer service phone call and press buttons for you. If it's like, if you'd like to go to whatever, be transferred to this, then press one. So they're doing incredible things with that. If you want to check it out, you can go to revia tech signup.
Demetrios [00:02:43]: We left the link in the description. I know I've already signed up because I get the feeling it's not going to be fully polished. He said it in the call right now. He said, you know what? It's not like we are 100%. We're basically, if you analyze this like a self driving car, we can get the car to drive straight on the road for 15 meters. And so going into it with the expectations that it's not polished, but it is going to be something that is immensely valuable if it can at least offload 10%, 15% of those phone calls that you have to make that you don't want to make because they're unnecessary. All right, that's it. Let's get into it with Sean.
Demetrios [00:03:29]: As always, if you enjoy this, share it with one friend so we can get the word out about the good old mlops community. And Spotify has that cool feature where you can leave comments. I read them all, the good and the bad. Actually, I love the bad ones. Talk as much shit as you want. To me, that is my daily fuel. I'll see you on the other side. So, Sean, dude, I'm excited to talk to you again.
Demetrios [00:04:00]: I know that we had our first conversation on the Deep Gram podcast that I run, and we got to talk about your founders journey and being a founder and what it means to develop AI products these days. But I told you, come on the Mlops community podcast, because I had such a great time talking to you and learning from you and your experience in being, like, in the weeds on the engineering is so valuable. And so being a technical founder that you are, I thought you would be able to still talk to us about coding and some of the stuff really putting AI into production is, I think, the theme of today's chat that we want to talk about. But as a quick overview for those who did not listen to the AI Minds podcast that we did, I want to let everybody know that you were working on Google Assistant, and then you transitioned from there. You said, you know what? I'm going to go do something a little bit more audacious and help build self driving cars. You were doing that for a while, and I guess all the while you had this bug that was telling you there was something there when it comes to Google Assistant. And so now you started your own company. You guys are about to be featured in the next YC batch, and it's all about assistance for humans.
Demetrios [00:05:26]: And so let's just break down a quick overview of what the product is before we go into the tech of it.
Shaun Wei [00:05:34]: Yes. So what we build is called Rivia Revia, and we are soon to have a version on app store. So I think by the time you listen to the podcast, you should be able to download that. But we're still doing, like, a small beta testing right now, and hopefully I can give this, like, a assistant to power your daily life.
Demetrios [00:06:03]: Yeah. And it's. Is it only in the US?
Shaun Wei [00:06:07]: It's in the app store. I think we can open up to all the regions.
Demetrios [00:06:12]: Oh, that's. Dude. So that's ambitious, right? Because there's so many different cultural things and languages and pieces that you have to deal with, especially when it comes to me not knowing. Basically you're making it open ended. You're saying that this tool can do anything that you want, right? When it comes to like dealing with customer service type issues, phone calls with products or product companies. Like I think about my mobile company, they call me all the time trying to offer me upgrades and I just want to know what the upgrade is. I dont want to sit on the phone call with them for a long time or if I need to get my plumbing redone, I break a pipe or something springs a leak in the house and I need to have a plumber come by. You can go and have the AI assistant call the plumber and schedule a time for them to come over and you just get a text message that says plumber will be over in again tomorrow or Tuesday between four and six, something like that.
Shaun Wei [00:07:17]: Yeah, that's the hope. Right? So like based on my past experience, like that's. People really want, like they want using the AI to replacing you in those like really boring phone calls. So that's the mission. Like we're building on this. Like people should be spending your time on meaningful tasks with your families, with your work. You don't want to like really spending 2 hours like dealing with like the plumbers, dealing with like your utility companies.
Demetrios [00:07:50]: None of them. Yeah. Like the least amount that I have to be organizing my life, the better. Yes, that is for sure. Dude. That's the vision. There's a ton of technical challenges that you have with this. So why don't you walk us through what some of these have been and how you've taken.
Demetrios [00:08:12]: Like I imagine you had a ton of learnings when you were working on Google Assistant and that was back in the day. I remember being so inspired when I saw that and I remember a specific point in my life when I saw that and I thought, wow, the world's going to change if this is real.
Shaun Wei [00:08:29]: Uh, yes. I think the uh, the Google Assistant capability you were referring to was Google Duplex. Uh, so uh, Pichai announced the Google duplex in Google I o. I think 2018, uh, when this AI was supposed to representing you to make phone calls, to like to book hair salons for you, book like restaurant for you. Uh, so I, I was actually on both ends, right. So on the building end, on the receiving end. So I interviewed people from both ends. So what I found was firstly, during 2018 we were trying to build an NLP and NLU model that can handle natural conversation in a phone call setting.
Shaun Wei [00:09:18]: It's extremely hard. You have to have tons of labeling data to handle a lot of corner cases when you're trying to make it sound natural. Right. So it requires a ton of training and it's spanning a larger team, actually just making that two use cases. And it may not work most of the time. So that's on the engineering side. During that time, it's really hard. And we don't have a larger model by that time.
Shaun Wei [00:09:53]: So what we did was trying to do a lot of classifications. Whenever you're trying to say, okay, I want to book a hair salon in this location at this time. So we capture those intent, trying to understand the user intent and map those to the actual sentence, so the audio bytes. So during that time, we were using, I think, CN or RN models trying to do that. And there's NLP's in the, in the front to do the Internet classifications. Then there's like the speeches of text or text to speech. So in the end, so. But CNR was like running extremely fast.
Shaun Wei [00:10:40]: So that's like a advantage over, like the larger model. Right. But on that part is like, since there's so many intent you have to capture, there are so many quantum cases, why interview the other end who were receiving the phone calls? They're very, very interesting for them. It's a little bit odd. In the beginning, there's starting, there's AI starting calling them. That's the first experience. They starting to experience all. There's AI actually holding another conversation with them.
Shaun Wei [00:11:12]: Then soon they realized the AI wasn't able to handle all the cases like back humans. So one of the example they gave me was they were a hair salon. So they were trying to receive a phone call from the good pacs saying, okay, the client want a haircut. But when they asked, what type of haircut do you want, a female haircut or a male haircut, that's when the system break. So that's why during that time, the NRP wasn't there to understand all the smaller cases. That's what eventually break. I think they were eventually receiving human phone calls. I think Google might be using human to represent the users to call them.
Shaun Wei [00:12:09]: It's just like so hard during their time. We realized that people really want to kind of hunt off this phone call experience to the e assistant because those are really boring. And like, the phone call by nature is a blogging experience, right. When you're making phone calls, you cannot do anything. So people really hate that experience. So that's why I got a lot of inspiration for Google. Do hacks when they made that slack work.
Demetrios [00:12:36]: All right, real quick, let's talk for a minute about our sponsors of this episode. Making it all happen. Lattice flow AI are you grappling with stagnant model performance? Gartner reveals a staggering statistic that 85% of models never make it into production. Why? Well, reasons can include poor data quality, labeling issues, overfitting, underfitting, and more. But the real challenge lies in uncovering blind spots that lurk around until models hit production. Even with an impressive aggregate performance of 90%, models can plateau. Sadly, many companies optimize for prioritizing model performance for perfect scenarios while leaving safety as an afterthought. Introducing lattice flow AI the pioneer in delivering robust and reliable AI models at scale, they are here to help you mitigate these risks head on during the AI development stage, preventing any unwanted surprises in the real world.
Demetrios [00:13:36]: Their platform empowers your data scientists and ML engineers to systematically pinpoint and rectify data and model errors, enhancing predictive performance at scale. With lattice flow AI, you can accelerate time to production with reliable and trustworthy models at scale. Don't let your models stall. Visit latticeflow AI and book a call with the folks over there right now. Let them know you heard about it from the Mlops community podcast. Let's get back into the show.
Demetrios [00:14:08]: All right, so moving on from, from that Google experience, you still set out to create something that is more open ended, and that just feels like really hard. How have you been able to deal with some of these issues that you encountered back in 2018 when you were doing this now? Is it just, okay, cool. NLP is much better with transformers and OpenAI, or is it a much more in depth problem than that?
Shaun Wei [00:14:43]: Yeah, I think the first experience I learned from Google washing for a lot of scenarios, cases, you have to do like human labeling, or you have to have some type of fallback mechanism. If it doesn't work, if you still won't get this work done, you have to have some type of fallback. And yeah, I also learned how, how human interacts with AI for the first time. What are the like, how they actually train themselves to be able to understand AI. Then I think the second experience of why building self driving car, that really helped me to understand, to deploy an AI in a actual world. Thinking about, yeah, you know, we can talk more about like what I have learned in self driving cars and, or if there's any topic you want to, like me to like, discuss a little bit more.
Demetrios [00:15:51]: Yeah, I would love to go into that because it basically went from like, now you're learning about. You're almost learning about the. The way that humans interact and the design piece, the product piece. And then you went into the technical piece, and you were like, all right, how do I deploy this? And how do I make it super fast? Right? Because with self driving cars, you can't be slow.
Shaun Wei [00:16:20]: Yes. Yeah. So for the Google experience, I learned the problem and the potential solution. So then in the self driving industry, when I was employee, that's when I really learned how to actually solve this few things, like for self driving cars. But most people probably don't realize this. We will think about self driving car. It's pretty much the most advanced AI integrated systems humans have ever deployed on the road, right? It's trying to negotiate with humans, behave like humans. It's trying to replace a human driver in a way, like using all the different AI models.
Shaun Wei [00:17:03]: That's like what we have been building for the last couple of years where the Waymo, or all the self driving car company has been building. It's trying to replace or at least mimic how human interacts traffic or control cars on the road. A few things in the self driving cars I really have learned are first, like, how do you simulate an environment that's safe in a virtual world, but still can representing a actual human world, right. Because it doesn't make sense for a car to rev around, then hit something that stops. That's not safe. But in a virtual world, you can do that a million times with no human hard in the simulation. Second is like, how do you make everything running super fast in milliseconds, but it can still, like, scale, right? So that's second things like, you'll be able to learn, like in the suburban car. The third thing you will learn is, like, people also probably don't realize is subframe car is also multi model in a sense, right? It's have the lighter data, the camera data.
Shaun Wei [00:18:27]: Later on, they also added, like, the audio input because you have to hear the sirens. Because when you hear the sirens, you have to know where the sirens coming from. And how do I pull over on a straight that's safe. So the server card itself, also a body model. So we can talk about each one of those and give you a little bit more understandings how the technical behind it to make it more reliable and be able to conquer the actual tasks in the human world.
Demetrios [00:19:04]: Oh, that's so cool. And that's so true. The multimodal. Now, how does that play into what you're doing today?
Shaun Wei [00:19:11]: Yes. So let's start with multi model, right? When people thinking about phone calls, you think it might be just all, it might be just audio. The reality is a little bit more complex than that. So when you're making phone calls as a human, what you already do is you have a mental model of what you're trying to do. Like dearly phone calls, it can be audios also. Like during phone calls there's very interesting, there's button presses, right? So like when you're dealing with all the custom support and everything, you have to understand those button presses and they take actions instead of just the audio bytes you are hearing. And when you deal with the phone call, thinking about the scenario, you usually have to also deal with incoming text messages. They will say, I will send you a message, I will send you a link to this, the model or the AI we are building.
Shaun Wei [00:20:19]: You have to understand, if you receive something additional, then the audio bytes, how do you deal with that? So that's why the multi model has to be there before you can complete the circle handling the phone calls.
Demetrios [00:20:37]: Wow, it's so complex because sometimes you get like, yeah, I'm sending you the text message with your code on it. And then you have to say the code and so you have to have the system understand all of that. How are you going about understanding it? And it seems like you are really doing stuff with agents that people only wish they could be doing. But from what it sounds like, you figured out a way to put them into production.
Shaun Wei [00:21:09]: Yes. So like I said, I'm highly inspired by the subtlinic cart, right? Like self driving car was able to take the text or take the audios and you can also command it, like take the text, take the different lidar radar data in real time and make decisions on those. So when we build the essential rivia, we use the similar methodology. So the system taking any type of streams, it can be audio streams, can be text stream, can be any type of data. It will be able to understand it on the fly. Yeah, we have a, we call it also called perception models. It understands like any type of data format and try to understand those like on the fly.
Demetrios [00:22:02]: And what does that look like? Technically?
Shaun Wei [00:22:04]: Yeah, for technically thinking about, thinking about the video recording, right? So when you record a video, you have this audio bytes, like streaming in using websockets or web RDC to the backend. Then you have the video stream, that's the frames also streaming into your backend. So in the perception models, you have to handle two streams at once. Right. Then you have your ML models, like once the, like, once the audio bytes or the video bytes comes in, the models will be able to start to handle those. You can handle those in a batch setting. For example, you can process this every two frames, right? Or every like hundred bytes of audio bytes. So that depends on like how frequent or like how fast your system runs.
Shaun Wei [00:23:01]: Like, for most of self random cars or most like, not just self driving cars. All the robotics, it's like processing the data in a EA frequency. So you see, probably figure you see the processing as probably hundred hertz. So even more faster. So we are adopting the similar technology is like the system runs on a clock cycle, right? Once like 100 milliseconds, you start into processing all the frames. For the last 100 milliseconds. You use that data to generate signals for the downstream systems.
Demetrios [00:23:41]: Damn, that is wild. So basically you're doing it at consistent intervals and just checking out what's going on. And it's almost like you're processing whatever comes in. It could be any modality.
Shaun Wei [00:23:58]: Any modality, yes.
Demetrios [00:23:59]: And then once you get a trigger for a certain modality, you know, okay, if it's speech, we're going to translate that to text and then we're going to work with that through transformers. Like a transformer model or what is. I'm not sure how you can actually like take action on it. I understand the, the data coming in part and then you saying, okay, now we have this data, but how do you actually go and take action on it and do that in a reliable way?
Shaun Wei [00:24:29]: Yeah, so I think eventually it will be end to end models, right? So it will be like the, any type of data, like, like the how the GPT four o does, right. Either will take the omni data, like for all my channels, like format into the systems, you have a dedicated, like models. You take in those different inputs, then map to like whatever actions you want to take. For example, it can be speak, can be voice, can be anything, right? It eventually will become any, to any model. But right now, since we are still in the like, you know, processing a collecting database, right. You do need a lot of data, like, to train our model. So you will have what I call is more like a, um. You, like you just described you.
Shaun Wei [00:25:14]: You have those informations. Then that's when you engage in like traditional, like NLP's, right? So you starting to have those like intent or triggers or signals you can use for your downstreams. So for those you're starting to engage with like the, like first of traditional piece, then there's like the newer generated AI's because you do have to understand like what's what happening. Like for, for all the audios, like videos, like for the last frame, right? So then you use those to generate even more signals, kind of signal or expanding the context. You can think of that way, expanding the context, just how you do a vector search, like rack, right? So you kind of enhance the context so they, so the model does not hallucinate, right, on a task because you still need to finish the user's task they assigned to you. So you do the enhancements due to validations. Then once you have enough context, you send us to the downstream. For example, if that's a transformer, general AI models, then you have a text, speech or direct.
Shaun Wei [00:26:30]: Direct text depends on your output, right? So yeah, that's the second part, right. So once you have enough signals, you can use that to ask your general AI models to generate the response for you.
Demetrios [00:26:46]: I like this description of saying you're just trying to gather as much information and as much context through the device itself and through the interaction itself. It's not like you're going to a vector database to gather this information. You're gathering it on the fly with all the different inputs that are coming through on the phone. I presume it's only through phone, right?
Shaun Wei [00:27:12]: Yes, it's only through phone. Yeah, yeah. But thinking about like let's refer back to subframe car, right? When you think about when you try to build a subframe cardinal, what is the first thing you're trying to do? You're trying to ask a submarine driving car to start driving in a street line, then stop. So that's the first simple task you have to ask a sub car to do. Similar to our cases, we start with something really simple, right? For example, Ken is like starting making phone calls and say hello to the, at the end, then hang out the phone call. So once you're starting to collect those data, you have better understanding how the AI and the human interacts. Just like how you understand like when the subgram cards going to drive the straight line, like what are the signal it's trying to collect? It's a similar, similar methodology.
Demetrios [00:28:09]: So basically these basic agents are very much like my 14 year old self prank calling different people and saying hello and then hanging up.
Shaun Wei [00:28:20]: Well, think about like, we don't want to harm the human in the process, right? So think of like what described like the virtual world. We, we put them into a virtual world, meaning like we are not using like the actual humans to sometimes to engage those phone calls, we have the other end. It can be recorded to humans to interact with the AI. So it won't not be like a lot of random spamming costs. We have this like testing a control environment.
Demetrios [00:28:55]: So this is fascinating to me because your training, or it's not training, it's really like validating your capabilities through this virtual world.
Shaun Wei [00:29:07]: Both, right. Like people talk a lot, especially for General AI. Like people talk a lot about how to create a synthetic beta. You can think of that. We also use that to generate sensitive data so we can later on use that to trend model. The second is the validation part. You asked me, multi agents, once they hit the human world, it starts to break. And then it's so easy to create a, a really appealing demos with your AA agents.
Shaun Wei [00:29:42]: You know, work only 1% of time, they have recordings and send out. Right. But how do you repeat the process, make it reliable? That's much harder. Much, much harder. I would think like you spend actually 90% of time effort to make it reliable. So that's why we have this like virtual world. We can, you know, if something breaks, we will able to detect by millisecond what happened on that particular moment. And also I inspired a lot of on the several card, right.
Shaun Wei [00:30:13]: I will be able to detect what happens on a millisecond to find, oh, is that something like related to the generate AI model or is related to the machine learning traditional NLP NLU model that make the wrong decision? Or is that because like open edges went down for, for like a couple seconds? Right. So we have those like simulations, like in the systems, so we will be able to like inspect and you know, to kind of fine tune the system in a way that will be able to reliably finish the task you have given it.
Demetrios [00:30:49]: Well, I know that the last time we talked it was how you can hand over the controls to a human almost. And so it's like you have real time observability to know when things go off the rails. Is that a homegrown solution that you created or are you using some like Datadog plus whatever like evaluation tool out there? How does that even look under the hood?
Shaun Wei [00:31:17]: If you search like the real chart AI, you will find like, we have open source products, right? And my team is really specialized in the audio processing, audio and streaming processing. So we build a lot of websockets in a very low level of websocket to handle all the incoming traffics and forwarding the audio bytes, like across different systems. So it's more like a homegrown systems like we, we have built in house and be able to, you were able to pretty much like observe what, what's going on and take over the control. If you think the AI is making run decisions, it's also like, you know, like self driving or like autopilot, right? So when you are, you can still like supervise the phone call or you can still supervise the driving. And if you find something odd like you can always grab the driving wheel saying, okay, I'm in control, right. But when you say oh, I think it's much safer for you to like take control now you can still engage the autopilot. I want to build the same experience. I think it's for, for people didn't realize this.
Shaun Wei [00:32:28]: If you're trying to build that like experience the audio bytes, the audio processing is very hard problem because like for, for text you don't feel is when you see a text, you know, if it's lagged for a second or 2 seconds, you don't feel the text slagging, right? So you find oh, it's, it's fine, it's like transformer is slow, but when the audio bytes slow, you will immediately realize it. You know, even by probably half second you start to realize oh, there's like really odd audio is starting to cut off or it's starting to slow, you realize immediately. So that's why it require a really fast system. And when you process in those audios, it has to be really mindful, making sure you don't generate lags into the systems. That's pretty hard. So that's another hard problem we have encountered because you know how the transformer works, right? If you have ever monitors how the open air gave you a response, you starting to realize their response is not stable. It can very interesting, like for the GPT four turbo because we have the internal data, we have internal benchmarks for all the different larger models, how fast they run and how stable they run. And you were surprised, GPT four turbo, the first token they were running is about 800 milliseconds to 1 second, the first token.
Shaun Wei [00:34:06]: So that's way slow for a, way too slow for the human conversation, like the real time conversation. And they were not really stable. So they can go up to like 3 seconds to 4 seconds. And we encountered this probably at 20% of time, right? That's the AA agent, right? If you're trying to handle the audio bytes then they announced this GPD 40, the even faster model they said oh, this like is the 50% like latency reduction. So yeah, they were true. Like for the, for the launch date we were seeing like 300 milliseconds, like 500 milliseconds for the first token. But then it's starting to like getting slow again, I think with the traffic, like they started receiving. Right.
Shaun Wei [00:34:54]: So that's why I will be super surprised if they're like end to end model can reach to the level they demoed during their opening adaptation.
Demetrios [00:35:07]: Yeah, yeah. It's a really hard problem to think about at the scale and then that time to first token. Yes, I know when you think about, I'm sure a lot of people, whenever they think about time to first token and the fast providers out there, I always think about perplexity and how fast they are. But you can't really use perplexity as an API I'm imagining for your use case.
Shaun Wei [00:35:35]: Well, perplexity, they do have an API, but perplexity is they have all their own models. I think that's mostly used for rack, not for a natural conversation. So that's why when we're finding the models to make our solution run faster. So that's why we use deep gram. So that's why you use deep grams like speech attacks, attacks to speech models. So it's help us on the both ends, try to reduce the latency.
Demetrios [00:36:12]: Yeah. And then the models in between. Are you just putting something in house?
Shaun Wei [00:36:18]: Because we run everything in parallel. Right. So we have built a mechanism like it doesn't matter, like if OpenAI is running slow, so there's always easy to fall back to a faster systems. So like the different tiers of systems to handle the request, to make it running faster and accurate at the same time. And also like I said, we have live time, but we have real time tracings on each of the requests. So if it's going like beyond 13 thresholds, we have a fallback mechanism. Right. Think about like, so that's why I also use like seven cars.
Shaun Wei [00:37:04]: Like if the self driving car want the AI models starting to break, does the system going to like stop on the road? This does not. Right. So it has a way better nothing. Yeah, it has a way to recover itself. So we are using the same mechanism to recover from like if there's any like general EM models running slow and we can recover from that.
Demetrios [00:37:26]: So I like this design pattern. It's basically saying you have a preferred model that you're going to be trying to use unless it's too slow and then you have fallback options. And I've also seen a different design pattern where you've got like the gateway, and depending on the prompt, it can choose which model would be best. If it's not that complex of a prompt, it can go to a smaller open source model and save a little bit of money or whatever. But it's a little different when you think about it as a gateway because you hit the gateway and then you kind of make the best choice from the gateway. What you're saying is we have a priority list, and we are always going to try and go for this model, whatever that top model is. But if we can't get that top model, then we're going to try and go down the priority list.
Shaun Wei [00:38:20]: It's slightly more complex than that. So there's a priority list for a certain request. We also have, like I said, everything is running in parallel, so we have similar to your gateway. So we have also a gateway for distributing tasks for different requests, different prompts, different identified intent, so they all play in a role for the downstream system to decide. Okay, should I pause, should I wait, or should I start and press buttons?
Demetrios [00:38:53]: Okay. Oh, cool. And I know that you mentioned you are dealing with genai and traditional ML.
Shaun Wei [00:39:02]: Yes.
Demetrios [00:39:02]: Are there pieces? First of all, just break down how that looks like that. You have the gen AI side, and then it goes down and, and it tells the actions to the traditional ML and then it ends there. Or what does that look like? And how do those two play together?
Shaun Wei [00:39:17]: Like I said, everything is running in parallel, so you can think of something used the terminal, like the event bus. Everything happening in millisecond is going to publish into an event bus. So any downstream systems, if you think you can handle the tasks, you're going to subscribe to those events, or like in the event bus, then once you're processing those, you're going to submit your response back to the event bus, then the downstream system, if they are interesting, your response is still like similar to subscribe to QI event. So that way you have a unified communication channel. It will make your system much simpler to handle.
Demetrios [00:40:12]: Wow. Okay. This is really cool to think about. So it's this event bus. And basically, if there is the notion that, hey, this might be for me, then whatever the task or the system is going to subscribe to it, and then if it is for it, it'll publish back the results, and if it's not for it, it'll also publish back results. Like, nope, this actually wasn't for me.
Shaun Wei [00:40:37]: Something like that, yes. Yeah, like the simple, it's a version, right? Like most people can understand, but there's like, you know, in practice, like it's in engineering, it's all in the details. There are so many things you have to make it work.
Demetrios [00:40:52]: Yeah. Like what?
Shaun Wei [00:40:54]: Yeah. So, for example, how do you scale? So that's like, really our problem. Right. How do you scale? Like, now you have millions of requests coming in milliseconds. Remember, you have to make sure the audio bias doesn't cut off. Right. In a high resolution. And there's like, the critical path cannot be slowed down because of all.
Shaun Wei [00:41:17]: You have suddenly have more events. But then there's things like, oh, if the system breaks, you have a fallback. All of those, like, play into make the system run faster. Right? Yeah. So those are the, like, a lot of engineering efforts going to make the system reliable.
Demetrios [00:41:35]: That's. And for anybody out there listening, we're laughing about the scaling part because I think before we hit record, we were saying that the typical question you'll hear at talks or meetups is somebody has to raise their hand and say, okay, this is great, but how does it scale? And so now we just basically did that to ourselves here. This is great, but how does it scale? Oh, that is classic. So this is fascinating to think of. I'm still trying to, like, get the picture in my head, but I think I have a more clear idea of it. Are there other challenges that you've encountered that you're like, damn, that one was particularly nasty and I'm glad I'm over it.
Shaun Wei [00:42:22]: Now how I feel is like I built on the traditional AI. I find everything we're trying to build on the general AI is nasty because you are constantly dealing with, like, slow response or inconsistent response or like, or like, the agent doesn't behave like what we expected or like, oh, it works yesterday, but why is not working this today? Oh, because opioid just upgrade their model or they just make the model like, dumber. Right. Those are the things we are constantly dealing with. The non deterministic behavior of the general AI is hard. We have never encountered things like this before. That's why I mentioned you can build a really cool demo. Probably worked only one out of 100 times.
Shaun Wei [00:43:28]: But if you try to make sure everything is working, like, from 90% of time, you lead to like, that's a very hard engineering problem to solve. Yeah. So that's why I find, like, anything that's involved in the general AI model is going to be hard. And if you really want to productionize it, it's going to really, really even harder problems. Um, so another thing I realized, I.
Demetrios [00:44:01]: Felt the long nights when you said that just all of that stuff is what we've been hearing time and time again on this podcast, and you're not alone there. The inconsistent responses, that's why like, evaluation is such an important piece of this. The slow responses. I think people have, a lot of people have abandoned a lot of use cases because they don't want to have to try and figure out how to make it faster. And so it's like we'll just wait until it gets faster because for some people, that's not how the beer tastes good. It's not like, if I make this and spend all this time and energy making this faster, what am I going to have to trade off in order to do that? Right. And so that's, that's another hard problem. So I feel for you.
Shaun Wei [00:45:02]: Yes. Yeah. I think it makes it stable, makes it run faster. It's going to be, it's a so different experience, like in the, in the, in the traditional AI world. And yeah, you have, so that's why you have to have really good engineering practice, like monitoring, benchmarks, evaluations. Most people, when they launch the product, they don't talk about those. They only talk about all the fancy products. But the harder problem is to how do you make sure you have all the tools you need to make it running stable?
Demetrios [00:45:40]: Yeah, the reliability piece is so important, especially if you're going to put something out there for the public to use. One thing is putting it in a video and cutting out the pieces that you like. But we've seen time and time again that people will put it out for the public and then it just gets demolished because it's not that reliable. And so you were in. I think what happens is you get the marketing teams that will create these videos and show that like, this is magical and then it gets out into people's hands and they have such high expectations for what it's going to do and it doesn't live up to that. It doesn't even live up to half of it. And so then you're like, this is a scam. What is this crap?
Shaun Wei [00:46:29]: Yeah, I think this is so true. Like, because like think about it, there's so many economists out there, right? You have to get your story heard first. Right. But also like on the other side is we all know the general AI model is growing super fast. Right? Maybe like the claims you trying to claim right now, you cannot achieve that within a year or within half a year, you might be able to do that. Right? So who knows? Yeah. So that's why like people, people freight like if they don't talk about this, they're going to miss out the opportunity, right. Another thing is like I'm not claiming I had no odd answers, right? I was still like trying to improve the system at the same time, right.
Shaun Wei [00:47:27]: I can only claim, oh my self driving car couldn't drive, driving street like for like 10 meters. Like that's I can guarantee. But it can go into like highways. Like to do like detours, a lot of things I don't have like the everything like ready like right now. Right. So yeah.
Demetrios [00:47:47]: And do you think that you just will need the training data like once you put it out there, just like with self driving cars, the more miles that a car drives, the better it performs. Or is it something that because you are using so many third party models.
Shaun Wei [00:48:06]: Yes, I think when I design the systems, the intent was as we collecting more data, more real interactions, the system going to get smarter automatically, right. So that's why we trying to launch early. Maybe it doesn't work like however some time, but we are able to collect in those interactions during the phone calls to see how AI interacts with the actual humans in those phone calls. So by then we were starting to have more data. I think like I mentioned, eventually for different use cases, you will have a dedicated model for that case, right. For us, we want to have that dedicated model to, for you to represent you, to make phone calls.
Demetrios [00:49:00]: And when you say use cases, you mean like if I call my plumber versus if I call my hair salon.
Shaun Wei [00:49:07]: It'S more like what we are thinking of is people feel most painful as to dealing with custom support, dealing with random phone calls, we want to have those data like how people actually engage in those conversations and how AI deciding to DNS phone calls, for example, if you're trying to call your utility companies saying, oh, you have a power outage, what did their system look like for example? It's more like, oh, you're driving to a new city, how their road looks like, how to get to the destination. And we want you to know, okay, how you get actually be able to navigate through that. And we are recording kind of like to understanding during the process. So, yeah, something like that.
Demetrios [00:50:02]: I see. So right now you're just exploring roads and gathering that data. And so different companies that you call into, you're getting their customer service agents which are probably also using AI these days.
Shaun Wei [00:50:19]: Yeah, so, so that's why I talk about like a business has been using this for ages. They're trying to take advantage of you, right? Like because you don't have like 2 hours or 3 hours waiting, like for their humans. Like saying, oh, I want to cancel my subscription. You know, they're going to say, oh, just get on this phone call, like 3 hours later, they're going to cancel your conversion. They know you won't do that, right? So they're taking advantage of you. But now we are giving you the similar or even better AI to like to give the option back to you saying, oh, you don't need to wait in line for that, right. Your AI going to do everything for you and then the business won't be able to take advantage of you.
Demetrios [00:51:02]: Yeah. And it's almost like throwing it back in their face. Like if I'm going to have to deal with these shitty chat bots, then I might as well have my own chat bot that can deal with them. And then if I need to be plugged in at some point, I can be. That's incredible. I'm very happy with the vision and I appreciate you kind of peeking behind the hood or under the hood and showing us what you're doing and how you're working on these hard challenges. Is there any other thing that comes up that you might want to give as words of wisdom to the listeners that are dealing and working with AI and ML day in, day out?
Shaun Wei [00:51:49]: There's like the newer AI going to redefine all the new systems, but it's not going to be a easy path, an easy solution, easy way out, how to deploy those in the systems. It's going to take probably it still take years for the AA agents really representing you to do a lot of things. I think right now be open minded to all the potential solutions and try them out. Try all the AI tools, try the AI things like, I think in the future you will be the level of intelligence you can leverage only really going to define how productive you are thinking about. I can probably utilize ten gpu's, GPU's intelligence to make my life easier or with someone can use utilize hundreds GPU's of AI to make their life better. I think in the future that's how we think you are rich, right? You got to leverage even more intelligence to make your life better.
Demetrios [00:53:03]: That is a wild way of looking at it. Like it's going to be, it's not currency. We're not going to play in us dollars, we're going to play in Nvidia chips.
Shaun Wei [00:53:13]: Yes.
Demetrios [00:53:15]: Wonderful, man. Well, I am super thankful that you came on here and you did this with me. It was a blast talking to you. I am so excited for what you're building. And I mean, I wonder though, on your launch, not that I know much about launching companies or any of that, so it's almost like a armchair quarterback right here. So take it with a grain of salt, but don't you want to take a page out of the self driving car book and just start in one area of like the US and start with one specific accent or one specific, like, company that you're trying to do or one specific use case?
Shaun Wei [00:54:00]: Yes. Like I mentioned, we don't claim we have all the solutions, right. But we really want to hand the technology to you. I want you like we have our own critical use cases we think we're going to handle really well, but most of the cases we may not handle really well. But we want you, the user, to get a feeling of how powerful AI is and be able to utilize this to battle the bigger corporate. So that's why we're trying to make sure people who were suffered from the phone calls and at least get access to see our mobile app first.
Demetrios [00:54:47]: What does the price structure look like for the consumer?
Shaun Wei [00:54:51]: Yes. So for us, we want a user mind. And very interesting, because whenever making phone calls, it's a really, I don't know, like why, just like in the US, like the first time I'm trying to make phone call, it's making me super nervous. I want this to be ease of mind. Like when you're trying to make phone calls, you think of like the solution we have built for you. And so that's why we charge a kind of flat subscription models. So then for any phone calls you can go over that, so you don't need to worry about, oh, I have to make phone calls today or I have to make phone calls tomorrow, or like there's something like, or the other side is speaking some language I don't understand.
Demetrios [00:55:38]: Right? Yeah.
Shaun Wei [00:55:39]: So I want a ease of mind. So, so that's why we are charging a subscription fee, so that you don't need to worry about any phone calls at all.
Demetrios [00:55:47]: And the reason I ask that is because I've heard people talk about this idea of I really want my customers to engage with my product, but because I'm using third party APIs, the more a customer engages with my product, that means the more API calls I'm making and the more money I'm spending.
Shaun Wei [00:56:09]: A few things, like we know everyone is doing token economy, but I think as an end user, you care more about end user, you care more about. Can I get ten years solve my problem as a company, if we find cheaper and still working solutions, we are making more profit. Think of it that way. We do our own calculation on how much token we consume for each phone calls. I think it still makes sense for us to open up this in a subscription models me as a consumer.
Demetrios [00:56:53]: I love that much more. I would rather do it like that than have to think about like, okay, should I use my credit for this AI phone call or should I just get on the phone?
Shaun Wei [00:57:06]: Yeah.
Demetrios [00:57:07]: So I feel you 100%. I'm super excited about what you're doing, Sean. This is awesome.
Shaun Wei [00:57:12]: Okay, see ya. Thanks.