AI Operations Without Fundamental Engineering Discipline
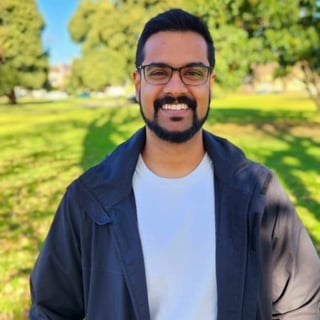
Nik is the author of ludic.mataroa.blog, who wrote "I Will [REDACTED] Piledriver You If You Mention AI Again", and mostly works in the data engineering and data science spaces. Nik's writing and company both focus on bringing more care to work, pushing back against the industry's worst excesses both technically and spiritually, and getting fundamentals right.
Nik also has a reasonably strong background in psychology. His data science training was of the pre-LLM variety, circa. 2018, when there was a lot of hype but it wasn't this ridiculous.
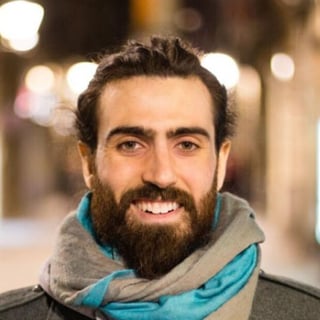
At the moment Demetrios is immersing himself in Machine Learning by interviewing experts from around the world in the weekly MLOps.community meetups. Demetrios is constantly learning and engaging in new activities to get uncomfortable and learn from his mistakes. He tries to bring creativity into every aspect of his life, whether that be analyzing the best paths forward, overcoming obstacles, or building lego houses with his daughter.
Nik joins the podcast to discuss an interesting trend: the anti-AI hype. Dive into what many companies might be missing when non-technical management rushes to roll out machine learning initiatives without bringing on the technical experts who can set them up for success. It's great to converse about bridging the gap between management and tech to make AI work effectively!
Nikhil Suresh [00:00:00]: Nikhil Suresh. I just go by Nik Suresh. So I work for a couple of people I won't name here, but the company I can't talk about without worrying about anything is I work at Hermit technology. It's a consultancy I founded with some friends. It's just six of us. We have no employees or anything. It's just six directors hanging out. I'm a director there.
Nikhil Suresh [00:00:21]: If you have a completely flat structure. And as far as coffee goes, it gets me way too jittery. I just drink a lot of straight Ceylon tea.
Demetrios [00:00:30]: Welcome back to the Mlops Community podcast. I am your host, Dmitri Ose, and today we're talking with Nick. This episode was all about AI projects, ML projects, data projects, and how you can have more success. What we can do to make sure that we have our feet on the ground when we are creating the projects so that we can ensure success. Nick has gone a little viral recently because of a blog post that he wrote, which is hilarious. And if you have not read it, we'll put a link to it in the show notes. It's all about if you say AI one more time, I will pile drive you. Hopefully you read it.
Demetrios [00:01:16]: If not, go read it right now and then come back to this. It's well worth the read. It will make you laughter and we dive into the blog post a bit, but we also dive into these underlying themes of why he was talking about, what he talked about in the blog post and where they came from. Hope you enjoy. Let's get into the episode. As always, if you like it, make sure to share it with just one friend so we trigger that algorithm. I've gotta start with. Obviously, we're gonna talk about the article that you wrote, which I think, hopefully most people have read by now.
Demetrios [00:02:07]: I've seen it on so many different slacks and so many people getting very energized about it. But it is basically, if you say AI one more time, I will fucking pile drive you. It makes me laugh so hard because it echoes so many sentiments. And as soon as I read it, I was like, oh, I should reach out to you and see if you want to come on the podcast. And so I did that, and you responded like, yeah, I've been getting a lot of requests, but I would appreciate to come on a small podcast. And I was like, I don't know if that was a backhanded compliment or if I should feel honored that you're coming on the podcast. So, Nick, it's great to have you here.
Nikhil Suresh [00:02:52]: Thank you so much. I'm going to apologize on air for that one. So what actually happened was I'm just some guy, right? So the issue is that when people said, you want to come on the podcast, I'm like, no big podcast would want me. So I knew there'd been one big one. I thought all the others were small. I got asked to do a clip for a documentary last week, and I genuinely, I thought the guy asking me was asking me for a student project. And I was like, that would be a nice thing to do. And then two weeks later I found out he's like the director of a university in the US.
Nikhil Suresh [00:03:24]: I just had to calibrate everything up.
Demetrios [00:03:29]: You've been launched into a different stratosphere because of your eloquent use of words and writing. And the funniest thing, I literally laughed out loud when. Because basically, for those who haven't read your post, you kind of make a point and at the end of each paragraph you, in different words say like, stop saying this or else I'm gonna kick your ass. And there was one I can't even remember, I should pull it up, but it's like you put it into a formula. You were like, if you say AI again, you will meet these hands or something like that.
Nikhil Suresh [00:04:05]: That's it. That. Try this bullshit. Catch these hands, dude.
Demetrios [00:04:09]: I laughed out loud. It was the first time I laughed out loud reading something in a while. And so I'm glad to have you on here. I know that the quick history of what you've been up to and why I think you are able to speak about this is you been through the gauntlet like a lot of us have, on trying to implement machine learning or AI projects in companies, and you've seen them fail time and time again, uh, like we all have, or you've seen leadership be like, hey, these guys are just like a cop. They're just a cost center. There's no profit coming out of here. What the hell is this data scientist doing? Or what is this machine learning effort? We're not like seeing returns here. How do we justify it? Or even like just data engineers, I imagine.
Demetrios [00:04:57]: So you want to give us the quick rundown on that? You don't have to say any specifics, but some of the shit, yeah.
Nikhil Suresh [00:05:05]: So kind of at a high level. So the trajectory, I think everyone goes through this. So I came from psychology, I moved over to data science because my stats background, I was a stronger statistician in psychology than most people, which is then I, then I went to meet real statisticians in data science. And I'm like, is the next level. But, you know, I did my best. I got good at it. And you go through that early phase where you're just so excited. You're just like, I want to build.
Nikhil Suresh [00:05:30]: I want to do deep learning. That was the thing when I started studying in 2017, right? I want to build my own neural networks. I want to do super complicated stuff. I actually did some complicated stuff at university. It was much more classically classical, statistics oriented. So it was a lot of information theory and expectation maximization and all sorts of weird model fitting entered the workforce. I'm like, this is where we'll do the deep learning. And all I could find was people.
Nikhil Suresh [00:06:00]: Well, all I could find. The market was huge in Melbourne, right? I found a job in one day. I didn't even have a full work visa. They just, like, snapped me up, and the pay was great, but no one even knew why they hired me. They would just land me at a company and I would be like, all right, like, what do you need me to do? And they're like, ah, we got a spreadsheet. You know, could, could you look at the spreadsheet? It has 10,000 rows. Is that big data?
Demetrios [00:06:26]: I feel seen.
Nikhil Suresh [00:06:27]: Yeah. Bounced around for a bit. I ended up doing. I even found one place where we did deep learning, but within two days, I'm like, this should just be like, this should just be xgboost, right? Like, the model works, but it's degenerated into a keyword search. You just get your bigrams and you go like, it's fine. And I gradually came to the conclusion that, like, the market for specialist data scientists, it's actually relatively small in terms of number of use case, but the hype around the market has really overproduced graduates and it's opened tons of roles, but only some of those roles are of. Are real. So that's kind of how I got to where I am.
Nikhil Suresh [00:07:08]: Like, I moved to data engineering. It's not because I even that article, it's not because I don't respect machine learning. It's the thing I chose to study. It's just that I couldn't stand people disrespecting the thing I did. So much.
Demetrios [00:07:20]: Yeah. And so painful just seeing, like, projects being funded, but with no clear objectives, no metrics, no. No clear success, and then you're being blamed for it, I imagine. It's like, why aren't you making this work? You're like, well, I don't really know what work means.
Nikhil Suresh [00:07:43]: Yeah, yeah. A huge one is the business, like, doesn't even understand how a machine learning intervention would interact with their business. So I remember when I started work, the first thing I got asked to do was predict government funding for something the next year. And I was like, look, I could fit whatever you want to this. But I go, it's not a statistical process. Right? Like, I can't model the probability that a politician pulls funding next year. I can't get in his brain. We only have, like, ten years of data.
Nikhil Suresh [00:08:18]: And also, I was like, what would you do with this number? And the answer is, they didn't know. They just wanted a line on a graph. And, yeah, it makes it very hard. First, it makes you very feel insecure at your job because you know you're not producing results. But it also means when you land in a job, you're not even in control. You're just hoping it is a job where they even conceptualize the role correctly before you got in, because it doesn't matter how good you are at machine learning, if they don't know how you would intervene, and there's no mechanism, and they don't want to do that work, it doesn't matter how good you are at it.
Demetrios [00:08:56]: Dude, you said something so important there, which I don't think enough people ask. And I had first heard it when we had this woman, Michelle, on from the Financial Times, and she was very strict about it in the financial times, like, building the data culture, because data engineers and data scientists and just, like, analysts, the data team was so overbooked or, like, asked questions of them all the time that didn't necessarily have a clear outcome or a reason for being asked. It was just like, oh, can we get this information? Let's ask the data team. And then next thing you know, they're flooded with questions. And she said that they needed to always have very clearly and almost, like, have an internal evangelist going around and making sure that before any questions went to the data team, there was someone that was, like, interfacing with the person, trying to ask the question and saying, okay, so, yeah, let's imagine that you got all that information, like, whatever the data question is around how many people from this region churned in the last quarter or whatever. Now you have that information, what are you going to do with it? And if people couldn't give actionable insights on that, it was like, well, I don't think we need to really answer that question. Then if you're just, like, doing it to scratch an itch, and that is what happens. You see it play out time and time again where you have all these questions, you have all this stuff that's like, yeah, wouldn't it be great to know that? And then later, you're like, well, um.
Demetrios [00:10:41]: So we know it now. What are we doing with it? And the sad part is people's jobs, like you said, like, there's jobs that rely on that.
Nikhil Suresh [00:10:51]: Yeah, yeah. I guess what you're saying is you almost need them to give you, like, a human decision tree before you give them a statistical one. Like, unless. Unless you show me, like, the forking path. I'm not giving you anything. I got out of data science around 2021. I moved to engineering because I found most businesses didn't have good infrastructure. Around that time in Melbourne, the jobs were drying up, and if it wasn't for GBT three coming out, it's a real field.
Nikhil Suresh [00:11:24]: So the jobs wouldn't have disappeared, but salaries would have taken a bit of a dive. And instead, we've gone the other way, which is businesses haven't figured out that core issue, or at least most of them haven't. I know a tiny handful. There's one in the city that was talking about. They were asking like, hey, could we hire you when we're ready for this big project? And I go, all right, so you want me as a data scientist, not an engineer? And they go, yeah, yeah, yeah. Okay, when do you want me? And they had that plan. They knew exactly what they wanted me to calculate and what they would do. So they were willing to pay tons of money, but they're like, we'll get back to you in two years, because that's how long it would take to put the infrastructure in place.
Nikhil Suresh [00:12:05]: And so I'll probably take that job in two years. But I'm also like, you know, it's hard once people are actually doing the math, the number of roles is much smaller. But the flip side is those roles are way more valuable.
Demetrios [00:12:18]: Yeah. If you can find a way to make it work or show the value, then exactly that it is immensely valuable. I've seen a lot of people go from the data science role to data engineering. Actually, right now, I am reading the fundamentals of data engineering by Joe Reese, and he did that exact same thing. He was like, and he considers himself, like, a recovering data scientist because of it, because he's just like, I saw data science is great, but what you really need is a strong data foundation. And so data engineering comes before AI, and you've probably seen the memes of, like, the company is just trying to jump straight to AI without having any data foundations. And so it is a strong argument. I do feel like though, one point that you mentioned in the, the blog post is that it's almost like there's been this resuscitation of the hype because of GPT 3.5 or chat GPT that came out.
Demetrios [00:13:28]: And so now, just as we were starting to like acclimate, like, oh yeah, like data science roles may not be as valuable as those articles of like the sexiest job of the 21st century said that they were, or every company is AI or ML company now we are just thrown right back into it and everybody is expected to be doing AI.
Nikhil Suresh [00:13:56]: Yeah. The first thing I'll say is fundamentals of data engineering slaps. It's such a good book.
Demetrios [00:14:02]: It's so good, right?
Nikhil Suresh [00:14:03]: Yeah, just really good common sense stuff in there. But yeah, to the broader point, yeah, we're just back in this kind of crazy cycle. And I remember before GPT-3 came out about a year beforehand, I felt like I was finally making progress. I could tell someone like, hey, we need to talk about model drift. And everyone was starting to get bored enough that they were like, all right, I'll listen to this nerd talk about his thing. And the moment GvT three came out, everyone's an AI expert and you just look at their cv's for 2 seconds and it's like you've never shipped anything in your life. It's so obvious.
Demetrios [00:14:44]: And the hype, because there's so much attention going in different directions. Like the attention dispersed. Now, model drift is not important. Traditional ML, nobody cares about that anymore. It's all about how are we going to implement LLMs into our workflow, how are we going to power up our workforce. And the funny part is that I've heard stories of how hiring has gone on a freeze because they're like, oh, well, we're going to be able to get more out of our employees since we're leveraging AI. And then now that it's been six months, a year of people really trying to leverage AI, actually, we're not seeing that productivity gain. If anything, it's kind of taking a toll on our workforce because you have to go back and fix stuff that the AI fucks up.
Nikhil Suresh [00:15:41]: Yeah, yeah. Also something to think about as a big organization, a huge issue is the amount of pointless work that gets done right. It is a systemic issue. When you have a large company, you just have a lot of people who end up pushing emails in circles. And it's very easy. I don't know if you're familiar with David Graber, the old kind of a sociologist or anthropologist, but he has this book called Bullshit Jobs. I think it was originally Atlantic article. And it's funny because if you go to a company, you can go to people individually, and sometimes you can find a whole department who are like, this department shouldn't exist.
Nikhil Suresh [00:16:18]: Chat GPT is not necessarily removing their work, it's making them, it's forcing them to generate more nonsense more quickly. Like, sometimes I think about how many departments are just people. It's just chat GPT emailing other people in circles, right? Like, I prompt an email, I send it to a guy, he goes, summarize this in reply. And then we just do the back and forth for a while. It must be happening somewhere, right? Like some government departments are just chat GPT talking to itself.
Demetrios [00:16:52]: Yeah. And it's not fixing the underlying issue. Exactly that.
Nikhil Suresh [00:16:57]: Yeah. And there's a lot of factors with GPT that are a little bit worse than, I think, the previous iteration in 2019. When people got excited about ML, they didn't even know what they were thinking, right? So if they said, we want AI, and I go, all right, what are you picturing? It was nothing or Skynet. And I'm like, all right, but now the demos are so slick, but it's so hard to get a working product going that non technicians have this very vivid image. They're like, I'm going to talk and it's going to do everything. And that's a way harder vision to push back against than like, oh, there's math you don't understand, right? They're so excited.
Demetrios [00:17:40]: Oh, man, you're speaking my language, dude. Okay, 20 seconds before we jump back into the show, we've got a CFP out right now for the data engineering for AI and ML virtual conference that's coming up on September 12. If you think you've got something interesting to say around any of these topics, we would love to hear from you. Hit that link in the description and fill out the CFP. Some interesting topics that you might want to touch on could be ingestion, storage or analysis, like data warehouse, reverse etls, DBT techniques, et cetera, et cetera. Data for inference or training, aka feature platforms, if you're using them, how you're using them, all that fun stuff, data for ML observability and anything finops that has to do with the data platform. I love hearing about that. How you saving money, how you making money with your data? Let's get back into the show.
Demetrios [00:18:35]: And that's one thing that I really wanted to talk to you about, was, like, how have you found success in managing expectations, especially from the non technical business stakeholders? Especially, like, you've just been talking about how important it is for the data teams to really be aligned with the business side of the house so that the work that you do is valuable and it has strong business outcomes. How can you then translate that and manage expectations when somebody's like, oh, so we can just use AI for this, right?
Nikhil Suresh [00:19:18]: Yeah, it's very hard. So I'll put some thoughts, kind of groundwork on what we call in psychology, like, theory of mind, which is imagining what is happening in someone's head, basically. And part of the issue is, and it's probably across software engineering, people don't have a good model of what any software work is by default. Right. So, for example, I assume you don't have a medical degree. I don't have a medical degree. You have a reasonably good idea of what a surgeon is doing, right? You couldn't do the surgery, but you understand, like, well, that area needs to be sanitized. Leave him alone.
Nikhil Suresh [00:19:54]: He's a professional. Keep some blood bags on hand. Like, you couldn't do it, but you get it. If you went into a surgery, you wouldn't be like, I don't understand what's happening. Whereas even pre AI, you have to understand, to a non technician, it looks like we're writing a word document in a weird language, and that immediately causes issues. Right? Because you cannot, I like this term from rich hickey, like complect. So to simplify is have everything straight, and to complect is to tangle, you know, so really bad abstractions and bad, it's like a big knotted spaghetti bowl. Word documents can't be complected.
Nikhil Suresh [00:20:32]: That is, you can write from the beginning to the end, and this paragraph can be bad or whatever. It is not possible to write a sentence in a word document so bad that the rest of the document stops working. Right? Like, that's not a, that only happens when you're programming. So immediately, I usually try to explain that kind of thing, but that needs a really long term relationship and a lot of patience. And getting into AI, like the LLM thing, it's, it's so difficult because it looks, it looks like a person talking. Yes. It's so, so difficult to explain to people. Like, it's nothing.
Nikhil Suresh [00:21:05]: Quite a person talking. And all the demos, salespeople, they are going out of their way to make you think it's a person, because now you think you're buying a person for like fifty cents per API call or whatever. So it's really about that disambiguation. I've been trying to find a prompt that really consistently breaks chat GPT so I can demo it. Awesome. I wasn't sure what to use. I'm still playing around. I was thinking maybe something like asking it to play a game of chess, because I've heard that it doesn't have anything approximating board state, so it just starts making moves that don't make sense.
Nikhil Suresh [00:21:46]: Like, that might work, but yeah, I'd have to play around. I assume someone's already done this. I should have looked it up instead of doing it from scratch. But really you need to. I think I haven't succeeded with this yet. You just need to like, what's the wizard of Oz thing? Show them the man behind the curtain. Like, there's nothing here.
Demetrios [00:22:06]: Exactly. Yeah, yeah. And for anybody out there that has figured out how to break chat chibute consistently, let us know, because that is a great way of doing it and just kind of like bringing people's expectations back to earth. And it is so hard for anybody who has used social media in the last year to not feel like, oh my God, this is really incredible, and this is like the future or the amount of stuff you hear on podcasts and how it's been projected to be something that is going to revolutionize the way that we work and live. And then I think there's us on the forefront of this and we're seeing advancements and we're seeing cool stuff happen, but it's not like what non technical stakeholders will expect from it. And so I find that really hard. I know there's a lot of people that are not only struggling to translate that to the business side of the house, but also, like, if you do manage miraculously to get something up and running and align with stakeholders and put it into production, how do you tell someone that we probably shouldn't trust this, like a lot, you know, like we should lower our expectations on trust because this thing confidently lies a whole bunch of times and so making sure that people are aligned on that part. There's so many, like, nuances of the productionizing of AI.
Demetrios [00:24:07]: And I really like how, how you said it in your blog where it's like, maybe we don't need to even be thinking about this, and it's a little bit of a waste of time. On most companies, you can buy something from a vendor that is toting how they're using AI, but really that vendor is probably just buying it from another vendor. And it is. And there's like, you go, it's russian dolls in a way, and down the line somebody's using some kind of AI in there. But for the most part, you don't need to be the one that's trying to do the cutting edge shit.
Nikhil Suresh [00:24:49]: Yeah, yeah. In fact, I don't even think it's good for engine. You know, normally in engineering, cutting edge is good, but if you, if you pull the russian nesting dolls out, you pull them all out. So many of these products, at the end of the day, like, the smallest doll is just the line that says import OpenAI. That's not job security. All right, anyone can type OpenAI ingest, but if you're shooting Xgboost, you actually have a specialization. I'm not just going to pull some random guy in. I go for improv theater here in Melbourne and one day I turned up.
Nikhil Suresh [00:25:22]: No one there is an engineer. I'm the only software engineer there. I remember my really good friend Felipe comes up and he goes, I've been thinking about getting into prompt engineering. I've heard that's a thing. And it's so hard to explain why people shouldn't do it because. Because that's a problem. You can do it. Literally anyone who, like, has a command of a language.
Nikhil Suresh [00:25:45]: Chad GPT could speak, could learn how to prompt engineer in like one month. Like, it would be fine. You do not want to build your career in something that people are going to catch up to in one month.
Demetrios [00:25:56]: Exactly. That's a really great point. And I feel like one thing that I've seen, and I've been trying to workshop this in my head just to get my thoughts more clear on it. So I'll throw it out there right now and see what you got to say for it.
Nikhil Suresh [00:26:12]: Yeah.
Demetrios [00:26:13]: There are a lot of engineers that are spending a lot of time trying to figure out how to get AI to work right, whether that is taking the really hard route and doing everything on prem and open source and incorporating it into a product so that you can get whatever chatbot to be your companion. And that takes time, energy and resources from the engineering team that could be spending it on other stuff. And then you've got the marketers and the salespeople who are using some low code, no code solution, putting together some pipelines, churning out a whole ton of content or emails or whatever that is very useful for their job. So I've seen marketers who have increased their website traffic because just they spit out a whole lot of, whole lot of blog posts. Right.
Nikhil Suresh [00:27:22]: Yeah.
Demetrios [00:27:22]: Or I've seen salespeople who are able to really tailor their pitch to a certain person or a certain company, et cetera, et cetera. And this is, or actually, one way, sorry to go off on another tangent.
Nikhil Suresh [00:27:35]: No, go for it.
Demetrios [00:27:36]: I think the coolest way that I've seen a salesperson do something is that they get the data from people who are looking at their sales page, and then they know which companies are looking at the sales page, and they can go and outbound to that company with a very tailored message on what other companies like that company have been doing, and blah, blah, blah. And so they are doing all of that with AI, quote, unquote, AI, right.
Nikhil Suresh [00:28:05]: Yeah, yeah.
Demetrios [00:28:06]: Or tools that are using AI under the hood, and they're seeing a lot of value from it. So the thing, the point that I'm trying to make is, like, you have engineers over here that are spending time, energy, and resources and really working hard to get the quintessential HR chatbot into production, that at the end of the day is going to tell someone how many holiday they have left, or you have the marketers and salespeople over here who are making good money from their AI efforts using low code, no code, and they probably didn't think much about the actual implementation and how they're doing it. And so it's like, wait a minute, engineering, spending all this time and effort on something that isn't giving us a return, and it's just like a hard engineering problem. Meanwhile, sales and marketing is actually using it and leveraging it, and it's giving us money. And so I've been trying to, like, figure that out and how to eloquently put it for engineers to, like, nothing. It is cool. But don't spend your time, don't waste too much time on something that doesn't have that clear return.
Nikhil Suresh [00:29:22]: Yeah. And so I'm scheduled to give my first talk, very exciting, in about a month, and it's based off a blog post I wrote. It's a bit of an old one. It's a few months old, but it was called, I called it, does a frog have scorpion nature? You know, that old fable of, you got the frog at the scorpion? A point I make a lot is that a lot of engineers, I call it kind of like frogs, meaning pretty good natured. They want to get along with everyone, want to solve problems, and then they get halfway across the river, and then they find out they've been laid off or they are forced to come back to the office or just do something they don't want to do. And they're sitting there drowning, going like, why? We were going to launch the project. And the scorpions like, well, I don't care. It's not my problem.
Nikhil Suresh [00:30:09]: And I really encourage, especially younger readers who reach out to develop some idea of what's actually going through. Someone who's financially focused head. And if you listen to. I was listening to a podcast a week ago, which I declined to go on, but it was from a leader here in Australia. And they're talking about LLMs, and something really informative kind of cropped up because they don't work in the engineering space. They do work in the engineering space. So when they talk about it, they're like, love engineers, can't replace them, blah, blah, blah. Really pro engineering.
Nikhil Suresh [00:30:44]: And then they talked about customer support and they said something like, yeah, we'll get rid of all those expensive humans. And it's like someone at every company, if they pay you a salary, wants to get rid of you because you're an expensive human. And engineers who don't really, really think about whether they have made more money than they got, like, brought on for are at some level of risk. And even if you do make the money, I've seen the other case where people, like, earn triple their salary, but they don't talk about it, and then they still get let go because, like, no one in finance knows what they did.
Demetrios [00:31:20]: Oh, wow. Yeah.
Nikhil Suresh [00:31:21]: So, yeah, I have a friend, I think he got hired by a Yde company out in northern Australia. One of his, he hasn't done too much work in the field, but he, I think, generated something like $1.5 million in his first year. But, like, no one in management knew, so they cancelled the contract with it explained. You know, there's so many things you need to do for yourself that aren't just fixing the problem, but you have to start by fixing the problem. Right. You can't not fix it. That's step one.
Demetrios [00:31:53]: Yeah. And that is one thing that, it's so funny you mentioned that because I feel like in the community, that's one thing that I want to do, or I almost look at is a signal for myself for if we are being successful. And I just want to give people, like your friend the platform to be able to talk about these wins and, and understand that it's okay to kind of pat yourself on the back every once in a while and show others how you've done something, how you generated actual dollars for the company, and then that will give you the ability, hopefully, when management is like, so what's that guy doing? Or what did what. What have they been up to? It's like, oh, yeah, they've been. It's very obvious what they've been doing because they've been giving talks at local meetups about their implementation, or they've written a blog post about it, or they've gone on a podcast about it. And so I hear you where it's like, a lot of engineers might not want to do that because it almost feels kind of icky. Right. It's like, I don't want to talk about how good I am or how well I did on this, but it is a necessity.
Demetrios [00:33:17]: Otherwise, you may not be fully recognized for what you're doing.
Nikhil Suresh [00:33:22]: Yeah. And, you know, there's two sides of that. Some engineers are just a little bit socially anxious. And to that, I would say, you know, I was until I was 18. You do enough reps, you don't stress out anymore. It's. It's just like going to the gym. You just keep going, and you don't mind if anyone thinks your squat sucks.
Demetrios [00:33:40]: Yeah.
Nikhil Suresh [00:33:42]: And the second one is, I think something I love about engineering culture is I'm on Mastodon instead of Twitter. I really like tech culture over more generic corporate culture. And something I really like is they push back aggressively against what I call a LinkedIn brain. There's a level of self congratulation. I usually describe it as masturbatory. It's just like, come on.
Demetrios [00:34:08]: Yeah.
Nikhil Suresh [00:34:09]: Don't do this in front of the children. This is. This is horrible. And engineers push back against that, but they push back too far the other way. You know, you can talk about what you did, right. In a pretty honest way. You know, my career is doing decently now, and it was doing decently before the blog post blew up. I'm very honest when I talk to people.
Nikhil Suresh [00:34:30]: You know, I had lunch with someone right before coming on here a couple hours ago who was in a position to give me, I would say, a pretty good job. And I was honest. I was like, hey, yeah, look, I'm smart, and I work hard. I haven't seen a successfully shipped project apart from this one thing. I'm batting 20% in the ML space. And some of it wasn't my fault. And I'm like, some of it was my fault. I ran out of mental health points before dragging the across the line.
Nikhil Suresh [00:35:02]: Like, technically, I could have if I stopped sleeping or something. Oh, people are open to that because they're so used to everyone lying. You don't need to. I'm not a super genius. I mess stuff up all the time. I mess serious stuff up all the time. Right? Like, it's. It's not just the.
Nikhil Suresh [00:35:16]: The easy ones that no one would mind. Like, I'm a human, and you can talk like that. It's okay. No one's gonna kill you for it. They'll appreciate it.
Demetrios [00:35:25]: Exactly. That's the key. It brings that human aspect to it, and people will very much appreciate it because we all know those blog posts where it's. There's the meme, right? How to draw an owl. Like, start with a few lines and then draw the owl, and you're like, what? That you skipped a few steps. And people, if you don't talk about the hardship or what the challenges were, then it also is a little bit bullshit.
Nikhil Suresh [00:35:53]: I mean, it comes off as insincere. Like, I've never seen an easy it project. I don't think I've seen an easy any project ever. Right? Just doesn't happen. Even, like, improv theater. It's so. It's unserious, right? It's just like, me goofing around with a couple of friends. And even then, I was at a holiday recently, and I had to miss three classes, and the teacher was like, you need to watch, like, 20 episodes of this tv show because it's the same format as, like, the improv piece.
Nikhil Suresh [00:36:23]: Oh, the things are just hard. And that's okay. You know, it's. And if you leave the hardship out, no one's gonna believe you.
Demetrios [00:36:30]: Yes. That is such a great quote. Like, things are hard, and that's okay. That's what almost makes it worth doing. Because if it wasn't hard, we would call it prompt engineering.
Nikhil Suresh [00:36:46]: And it's why it's so tempting, right? Cause everyone's like, could I just do the easy thing? Well, unless you're a grifter, you will only be paid for doing hard things. And you can study until they don't feel hard, but you're never going to start with them being easy. It's just not going to happen. Here's a question for you. When you started this podcast, you must have gone through what I'm going through now. And I'm bad at, like, the podcast editing arc where you learn about compression and just, oh, yeah. It's like, nothing is easy. It's actually hard, right?
Demetrios [00:37:21]: It's really. It's a pain in the butt. And it's just time you put time in. Also, I think the hardest part about that, it wasn't. I was really into video editing and audio editing before the podcast started. But the hardest part was you put hours into this and you're like, wow. You polish it off. So it's your best effort.
Demetrios [00:37:43]: You're putting your best foot forward, and then once you put it out to the world, you get, like, five views. And that was the hardest part where it's like, damn, I just put hours in my life that I can never get back into that, and I got five people to listen to it. And it's not like that happened once. It was a continuous thing, you know, like, for months that happened, and you gotta grind through it. That is really what it comes down to. I'm a firm believer in it.
Nikhil Suresh [00:38:16]: Yeah. Yeah. In fact, I think that's the only. The only failure about an engineer can really get into, is that they put the hours in invisibly. And the nice thing about publishing a podcast is it's not invisible by definition. Yeah, it could be awful. But as long as you keep working on it like it's out there, someone will eventually. Even if it's your mom, like, she'll get into it if it's her friends.
Demetrios [00:38:39]: Which totally happened with my TikTok account. My mom showed it her friends, and now I have them commenting on my TikTok, and I'm like, thanks, I appreciate it. Not exactly the demographic I was going for, and I know they don't understand anything because I'm usually trying to give takes on machine learning and AI and all that. I know that most of it goes over their head when I start talking about recommender systems and the evaluation of LLMs, but they still comment, and they love it, so. Yes, exactly.
Nikhil Suresh [00:39:14]: Yeah. Yeah. A lot of people wrote into me and they were like, I don't know anything you said in that blog post, but they were like, it sounds like I'm gonna keep my job. And it was kind of funny, you know? So I'm a subscriber. All right. Well, yeah, people just appreciate you being human, and it's not a marketing strategy that gets talked about, because it's scary, vulnerable. I posted stuff about mental health before, and it's pretty serious stuff, stuff I've gone through. It's fine.
Nikhil Suresh [00:39:45]: People are fine with it. I guess it's something I push a lot when I talk to engineers, which is, you know, you don't have to change who you are, but it's also okay to show people who you are. Right. If you're super shy and don't like talking to people, you can, you can find it deep down in your soul to give, like, one talk. Your friends will help you get through it. Everyone will think better of you for it. And even if you look super nervous, like, people are supportive. The worst I've ever seen a comedian bomb was, like, the Melbourne Comedy Festival two years ago, and.
Nikhil Suresh [00:40:18]: But the person who bombed is clearly, like, freaking out. And, like, it was. They were not funny, they just didn't pull it off. But they got the loudest round of applause of anyone there. Oh, yeah. Because people just appreciated they were going hard. But if that person had been really polished and bombed, like, there would have been no support. Right.
Nikhil Suresh [00:40:38]: If you go to a TED talk in your suit and you try to come off all cool and you bomb there, you're done. Like, no one's helping you out.
Demetrios [00:40:47]: Oh, exactly. Yeah. I wanted to go back to another thing that you mentioned, and this is along the lines of being visible and being out there and making sure that people understand what you do well and understand who you are, because I imagine you get a lot of people that reach out just as I do who are like, hey, I'm trying to get a job, or I want to do this 100%. Tell people, you just start making content, let people know who you are, and that will help you more than anything else. More than if you're going to spend 2 hours filling out applications, try and spend 2 hours creating content and see if you can commit to doing that on a consistent basis. And it doesn't matter. Like, you don't have to be the best. That's what I really like.
Demetrios [00:41:43]: Try and hammer home. You don't have to be the best. You don't have to really, like, know everything before you start creating content. You just have to show the journey of you learning it. And that is huge.
Nikhil Suresh [00:42:00]: Content's really worked for me, but I think the overwhelming thing, especially if you're young and entering the field, is understanding that getting into the field doesn't require you to, like, be incredible cool. People will hire you off your potential. As long as you've put work into a thing. That's all it takes to have potential, right? You just tried to do something. If a junior engineer or somebody wants to be a junior, rocked up to me and showed me, like, the worst python I've seen in my life, but they clearly tried, I'd be like, all right, you're it. I'll give you a shot. And, yeah, that goes a really long way. It's different.
Nikhil Suresh [00:42:43]: If you're like. It's probably like an ageist thing here. If you're 50 or 60 and trying to make the swap, people aren't going to give you that same benefit of the doubt. And you've got to get a little bit more clever. It's doable. But, you know, if you're between the age of 20 and 30, you could literally do anything. As long as you seem enthusiastic, you'll be fine.
Demetrios [00:43:01]: Yes, exactly. And if you're stoked on one specific thing and you've gone down the rabbit hole on it, even better. Like, find a nice little niche of stuff that you can say, like, yeah, I really like. Whatever it may be, then that helps, too. I think about maybe it's like, oh, I'm really into observability, or I'm really into rust. Like, we've got a whole group on programming or LLMs and rust or whatever. Whatever your choice is, whatever floats your boat. I think that is a great way of looking at it, too.
Demetrios [00:43:41]: And you can always change.
Nikhil Suresh [00:43:43]: Yeah, yeah. And, you know, it's not even engineering advice. Right. This is advice on, like, how to just build good relationships with people. If you want to be a writer, just seem really excited about writing. Be really excited about writing. Yeah. Because so many people, I get so many emails and they're like, I appreciate where they're coming from, but they're like, you know what python book do I need to get into the field? And I'm like, it would be good if you read a book, but how many coffees have you lined up this week with people? That's the medicine you should be looking at.
Demetrios [00:44:13]: Yeah. Oh, man, that's so true. And that is not. That's for any stage of your career. Right? Because it goes back to the point that you were talking about on the making sure that you are aligned with the business objectives. And to do that, it's very much a relationship game. We had Sol Rashidi on here a few episodes back, and I asked her, she was chief data officer at a gigantic company. I think she said she had, like, 900 people on her team.
Nikhil Suresh [00:44:47]: Jeez.
Demetrios [00:44:48]: And, yeah, huge. Huge.
Nikhil Suresh [00:44:50]: That's huge for a data function. Like, I've never even heard of that.
Demetrios [00:44:54]: It's gigantic. And I was saying, what was the most important part of your role? What were you spending your time on? If you could give me a pie chart. Was it trying to figure out the operations of the projects that were being implemented? Was it figuring out new tech that you may need? Was it relationship building or, uh, and she said, like, without a doubt, it's all the relationship. Like, 90% was relationships and just making sure that every one of the stakeholders was aligned the whole time and everything else, like, kind of fell into place after that. So it's exactly what you're saying, man. And it kind of almost can go in that along the lines of what you were saying, too, wherever because of that, the politics of it, you get shit. That is kind of bullshit.
Nikhil Suresh [00:45:55]: Yeah, yeah. And that, that's a hard one because a lot of the time when people go like, hey, how do I fix this at this company? I just go, you don't. You're one person. You know, like, there's those four people that say they're generative AI experts. They don't have another skill. You can't convince them to become homeless. Right. You're not going to talk them into that.
Nikhil Suresh [00:46:17]: Like, you just have to accept some battles are not necessarily winnable right now. I remember when I did that better offline episode, it ended with them asking, what's your plan for dealing with the Gen AI hype? And I was like, I'm just going to stay away from it. That looks like it's going to explode, and I don't want it to take my career with it, but as soon as it does explode, I'm probably gonna get straight into the space and get into, like, the interesting bits. I just can't do it now because everyone tells me I'd love to do it. It looks super cool. I just can't figure out who is serious about hiring and who's just kinda making it up. And I don't have time to, like, work through ten jobs until I find the one serious person.
Demetrios [00:47:02]: Wow, that is some wisdom right there.
Nikhil Suresh [00:47:06]: Thank you. Thank you. I should clarify for any listeners. I have no idea what I'm talking about. Just making it up.
Demetrios [00:47:14]: Classic. No, I do like that stance because you can set yourself up for failure if you're not picky or you're not smart about how you engage in this space right now.
Nikhil Suresh [00:47:30]: Yeah, yeah. A lot of it's just finding people who kind of have a little bit of mutual respect. The thing I always compare it to is when my electrician comes in and he's like, we got to open this wall up and move all this stuff. I could be taken for a ride, but I don't know, I just go, all right, listen, I trust you, Tony. Whatever you want, just fix the wall up. As far as I know, I'm not being scanned. I haven't lost a bajillion dollars. Software engineering is the only thing where people have no idea what I do.
Nikhil Suresh [00:48:03]: Consistently try to have an opinion. How nuts would it be? I don't know anything about electricity. It's the magic thing that makes the computer work. If I went to my electrician and I'm like, I don't think you need gloves, Tony. Why can't you do it faster? You wouldn't even think about doing that. But people try it with software folks that the people are. I just wait until I find someone who doesn't behave that way, and then I'm. I'm gonna work with you forever.
Nikhil Suresh [00:48:29]: You're never gonna get rid of me.
Demetrios [00:48:36]: That is so good, dude. Well, this has been awesome, Nick. I appreciate you coming on here and chopping it up with me, and I really am stoked for your voice of reason in this space. Hopefully, you will continue to share your experiences and your learnings with the rest of us in the comedic way that you have been doing thus far.
Nikhil Suresh [00:48:58]: Thanks. I appreciate that. I will say this is the coolest, chillest podcast I've been on so far, so that's tremendous.
Demetrios [00:49:07]: Well, we only have a few listeners so far. I guess that's why.
Nikhil Suresh [00:49:12]: I'm sorry. I'm sorry. I didn't know the truth.