AI's Next Frontier
speakers
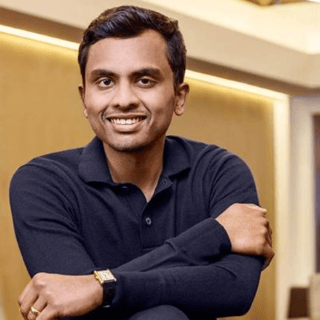
Aditya Naganath joined Kleiner Perkins’ investment team in 2022 with a focus on artificial intelligence, enterprise software applications, infrastructure and security. Prior to joining Kleiner Perkins, Aditya was a product manager at Google focusing on growth initiatives for the next billion users team. He previously was a technical lead at Palantir Technologies and formerly held software engineering roles at Twitter and Nextdoor, where he was a Kleiner Perkins fellow. Aditya earned a patent during his time at Twitter for a technical analytics product he co-created.
Originally from Mumbai India, Aditya graduated magna cum laude from Columbia University with a bachelor’s degree in Computer Science, and an MBA from Stanford University. Outside of work, you can find him playing guitar with a hard rock band, competing in chess or on the squash courts, and fostering puppies. He is also an avid poker player.
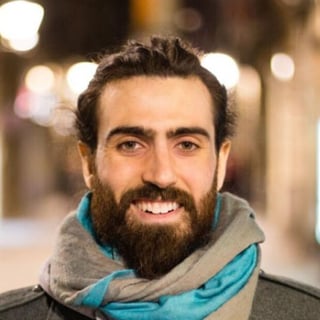
At the moment Demetrios is immersing himself in Machine Learning by interviewing experts from around the world in the weekly MLOps.community meetups. Demetrios is constantly learning and engaging in new activities to get uncomfortable and learn from his mistakes. He tries to bring creativity into every aspect of his life, whether that be analyzing the best paths forward, overcoming obstacles, or building lego houses with his daughter.
SUMMARY
LLMs have ushered in an unmistakable supercycle in the world of technology. The low-hanging use cases have largely been picked off. The next frontier will be AI coworkers who sit alongside knowledge workers, doing work side by side. At the infrastructure level, one of the most important primitives invented by man - the data center, is being fundamentally rethought in this new wave.
TRANSCRIPT
Aditya Naganath [00:00:00]: Aditya Naganath. I am a principal at Kleiner Perkins and I love cappuccinos.
Demetrios [00:00:07]: Folks, welcome back to the ML Ops community podcast. As always, I am your host, Demetrios and we are in for a wild ride in this conversation. As you may have seen, occasionally I like to bring on some VCs to hear what they think about the current state of the market and the space. This is one of those times I got to talk with Aditya about how he sees MLOps tooling, LLM Ops tooling, if you want to call it that, infrastructure and AI infrastructure, and what the difference is between those two because I needed a little bit of clarification on that and oh, so much more where he's excited about investing and where he's not excited about investing. He made me rethink some of my previous assumptions and I hope some of the stuff he said is wrong for the sake of my portfolio companies. But you know what? He made some convincing arguments. So let me know what you think in the comments or give me some feedback. Tell me if you are seeing the same thing that he is seeing or if you're seeing something totally different.
Demetrios [00:01:31]: Let's get into it. And as always, if you enjoy this episode, share it with one friend. We are in a field that is very busy and you more than anyone know that because you are investing in this field. How does it feel to be an investor in the AI space these days? Man, it's gotta be wild.
Aditya Naganath [00:01:58]: Thanks for having me, Demetrios. It is indeed wild. It is crazy out there. And the reason for that is we just see so many kind of ideas emerging and at the same time so many different startups tackling those ideas. For any one interesting idea, you have 10 or 12 pretty solid teams taking a crack at it. And you know, our job is to, is to pick ones and the question is, how do you do that?
Demetrios [00:02:23]: I hear people talk a lot about, oh well, it's gotta be the team. It's gotta be the team. Do you have any other insights that you tend to lean on or biases, dare I say it?
Aditya Naganath [00:02:35]: Yeah, I think, I think beyond the team, I wanted to see some semblance of a novel approach. Right. And ultimately the question is, what are you going to do to rise above the quote unquote noise floor? And I think there are people who are just like, hey, this is a pretty consensus opportunity and we want to go for it. That's not that exciting. I want to see someone actually put some thought into look like there are 12 other pretty solid folks going after this. But here's why I actually think we have a chance. And it kind of points to the details and points to say, having put in more thought into the actual underpinnings of a successful product.
Demetrios [00:03:18]: And I can imagine a lot of times that has to do with go to market motions.
Aditya Naganath [00:03:25]: Go to market for sure. Right. Like, you know, if you think about at the end of the day what it takes or it's a great team that has a great product that has very good go to market. Right. So in that sense it's not rocket science, but you know, good go to market follows a great product. And then so to me, it's still about what is going to be that. That insight that drives a really novel approach that yields a great product which will then yield a great go to market.
Demetrios [00:03:52]: Yeah, it feels like one way to bet on a team or have a little bit more success rate is if you know that the founders or the team has some kind of advantage on that go to market and their motion. Whether that's like the founder has a lot of distribution or is well followed or has a community or has been very active in communities, that type of thing just feels like, oh, there's an unfair advantage here. And so you can't count that out.
Aditya Naganath [00:04:27]: For sure. For sure. You know, any kind of distribution tailwinds that you can generate helps, but at the same time, you don't want to be one of these flash in the pan kind of companies that has, you know, steep revenue growth, but then realize that, hey, look, that again, the foundations were unstable and as a result, you know, two years out, three years out, that company has churned a bunch of customers or that growth is, you know, a shadow of what it was. Right. So there's no substitute for thoughtful product execution.
Demetrios [00:04:57]: And what about those companies like a Lenza where it's a fad and maybe in the hype, in the peak of the fad, you're trying to discern if this is lasting or if this is just a flash in the pan.
Aditya Naganath [00:05:13]: And first of all, I'll say consumer is hard. Right. And if you think about consumer in as a black box, it's all about user retention because at the end of the day, you're competing for people's time and attention. Right. And that's gotten so hard with obviously the Facebooks and all sorts of other apps of the world. So we pay a lot of attention to retention data.
Demetrios [00:05:38]: Yeah. So there is a. So consumer is a beast. I like how you put that. It is a black box. And when we're dealing with AI, so you get the double black box and two wrongs don't make it right. I can imagine how it's a bit of a crapshoot. You're going to the casino with some AI apps for consumers.
Demetrios [00:05:58]: But what I'm really interested in talking about with you is the MLOps space, the AI infrastructure space, and then the apps space or the application layer as some people call it. Because I want to know first and foremost, there was this Cambridge explosion that happened with machine learning platform tooling companies in let's say 2018 to 2021, 2022. And I would love to know what you need to see now from a company that was funded in that stage to make you a true believer.
Aditya Naganath [00:06:51]: Of course, you know, a great business is one that, that has a lot of revenue and is generating that revenue efficiently. But, but really what that points to is in the ML ops world in particular, were they able to show enough value to their buyer set such that that buyer set wanted to buy the solution versus build in house. And if you kind of think about the mlops landscape as a whole, again from the perspective of a venture investor, a lot of those companies haven't been great outcomes. Right? Like, you know, scale AI is one that obviously is a great outcome, but they were far left in terms of the value chain of an MLOps or ML engineer. Right. Like they handled labeling data that nobody will want to do in house. Right. But if you think about all of these companies that emerge with like, you know, AB testing your model, like evaluating your model and you know, being a feature engineering platform or what have you, the sad reality is that they couldn't really crack these larger enterprise contracts because those enterprises had teams that would build those solutions in house, tailored to their own needs.
Demetrios [00:07:58]: Do you feel like there is still too much custom white glove that needs to happen with the way that the each enterprise has architected their ML platform, you can't quite sell this standardized tool into the platform. So you haven't seen such a large takeoff like was anticipated in that 2018, 2020 era.
Aditya Naganath [00:08:30]: That's right. I still believe that. And you know, even if you are able to sell something into these enterprises, what I found is that you tend to capture a small portion of bud because you fit into a narrow sliver of that overall workflow or value chain. And big challenge for these companies has been to the dream rather for these companies has been to actually own a lot of that value chain. But again, because of the amount of customization that's been required. It's been really hard.
Demetrios [00:08:58]: How do you compare that with the data platform? Do you feel like? Because if in my eyes what I've seen is that there is a lot more growth there and it does feel like there are companies that have taken off. So I'm always questioning is it the maturity of the ML platforms or is it that ML is such a small TAM or such a small sliver and it is a very, as you mentioned, unique use case. But almost everyone is going to have a data platform, whether you're doing ML or you're doing analytics, whatever it is. So there's a, there's a larger TAM there.
Aditya Naganath [00:09:41]: Yeah, great question. I do think it's a larger tam. Right. And if you look at the companies that have really broken out, whether it's Databricks and Snowflake etc, they've really made their money off of compute or storage. And that's really anchored to this trend of data only exploding in the enterprise. Right. Structured and then you have unstructured data that's 4x as large as structured as structured data. And in the case of databricks, they sold a compute fabric.
Aditya Naganath [00:10:08]: Right. Ultimately, Spark was a better way to do computations over large swaths of data, essentially through this innovation called the rdd, which allowed you to do in memory computations and they monetized that. Right. So they were in the flow of computer and then with Snowflake it was, you know, hey, look again, we'll just give you a way more efficient warehouse on top of again, your growing swaths of data. So they anchored themselves to that trend. Now you've also had, to your point, six pretty successful companies that have, you know, existed in that value chain of, you know, data operations. Right. So DBT being a shining example where they've owned, you know, transformation.
Aditya Naganath [00:10:48]: But I would say the jury is out on how big DBT will be as a company. But it's still a much better outcome compared to a lot of these MLOps companies that have also tried to go after analogous workflows. So yeah, overall a bigger market because data was only exploding and people across the board needed to do interesting things with their data. While in the MLOps world, to me it feels as though these MLOps, these ML practitioners, they were doing a bunch of custom things with their models. But ultimately it's unclear what that, you know, what that secular trend was for a startup to go and anchor themselves against. Beyond, by the way, what scale AI did, which of course, you know, they were very smart in identifying that every model is Going to be powered again by surprise, surprise, labeled data.
Demetrios [00:11:37]: Yeah. So knowing that, and I appreciate these almost like cold buckets of water that you're pouring on me right now. And I wonder, being equipped with that vision, do you try to dig deeper into the new ways that compute and data slash storage are coming out? Because I know there's no shortage of companies that are trying to reinvent the ways that we're using compute or just take down spark, if we want to be blunt.
Aditya Naganath [00:12:19]: Totally. So I presume you're, you're, you're alluding to this whole AI wave, right? And the reality is that they have introduced two new workloads of node, right, LLM training and inference. And what's happened is you can, you can think of the infrastructure layers being bifurcated across LLM Ops companies, right? And these are the companies of the same ilk as these ML Ops companies that preceded. And then you have companies that are true infrastructure across, you know, compute storage. And we're now seeing emerging class of networking companies. So what I've found is that, you know, the compute layer has been quite interesting to invest in. And in fact, you know, we at Kleiner Perkins invested in a company called Together AI because it turns out that, you know, on both obviously LLM training and inference, there's a ton of demand, right, from these models. And so anyone who can figure out how to, you know, serve that compute at a cheaper rate and with lower latency has a chance at being very valuable.
Aditya Naganath [00:13:20]: They essentially again monetize through compute. Then there's a company called Vast Data which is finding themselves in the, in the data centers themselves and essentially being the hub for, you know, again, petabytes scale of training data. And they have done very well as a result. The jury is still out, I would say, on the LM Ops companies, right? And you have a ton of companies going after, say, evaluations and you know, we're seeing companies try to be, you know, data curation platforms and we'll see like, you know, I, you know, we haven't invested in that layer. And again, I think the big question is, you know, will there be one that is able to break the, you know, build versus buy mold and, and also, you know, be able to rise above all of these other competitors that look pretty identical to, to each other.
Demetrios [00:14:08]: Talk to me more about the LLM OPS paradigm and how you have sat out up until now. You're saying we'll take the wait and see approach because probably it, a lot of things look familiar from the ML Ops is It again that you're looking at a small tam. Because when I look at LLM workloads and this, the AI infrastructure, I 100% agree with you. Where there's been the Cambrian Explosion, it's a lot of similar tools and that's not very inspiring. But I do feel like you have a much broader user base because you are not only getting the ML practitioners, you're also getting a lot of software developers who now are playing with AI APIs and they can go out and they can put a few API calls together and now they're an AI engineer, which in the MLOps era you didn't have that you were looking at a much smaller subset of the engineering world.
Aditya Naganath [00:15:24]: Yeah, when I actually think about ML Ops, yes you had your machine learning engineers who were the guys behind a lot of the interesting models of that, of that world, but you also had software developers as well who were able to write Python and make a call to XGBOOST and get some interesting outputs. Right? And yeah, so in the LM OPS world what I think has happened is you have, you know, people coming up the maturity curve, right? And so again LLMs are a black box. That's what's different. They're non deterministic. And so compared to, you know, some of the typical machine learning models there is, you know, a question around reliability and how do we work with the outputs that these things provide. Which if you're a bull, you can make the case that you will need scaffolding tools to work with these things. And then the question is will people want to build those tools in house or just buy off the shelf? Because again, all they care about is the use case and the problem they're solving at the same time. A I would say the question is what is the scope to differentiate yourself.
Aditya Naganath [00:16:36]: Right. Because let's take again this, this really hot category of LLM evaluations, right? A lot of teams have identified this pretty, you know, obvious problem that hey, LLMs are not always reliable. Their outputs will, will many times drift against your key objective and sometimes it's not even knowable why they drifted against this key objective. So can we first start with you know, just giving you observability and then over time worker work our way into helping you fine tune reprompt your model to be better. The question to me then is what is someone going to do that's markly different from the 12 or 15 other companies going after this problem? And then I think the second thing to keep in mind is look a couple of years from now, people are going to be that much more aware of how to work with these things. There's going to be that much more knowledge disseminated around LMS that engineers will read and get smarter about. And then so when they do, you could still have a situation where people build in house because they have custom needs. Right? So I think that's my concern with that overall market opportunity.
Aditya Naganath [00:17:42]: It doesn't feel like. It doesn't feel like it's provably big yet. And on top of that, it feels like it's getting chopped up in many different pieces.
Demetrios [00:17:52]: So now I have to ask, why is together different? Because it does feel like together is in that space and, and we say together because you guys invested in them. But we can also throw out just so that we're not completely biased. I hope you allow me to humor you. There's a few other tools that are in the same space, right? Like I would consider modal part of that space and base 10 and maybe beam Cloud, maybe Lepton and Run Pod. Do you consider them?
Aditya Naganath [00:18:26]: Yeah, yeah, absolutely. Run Pod fireworks, Right? So you're absolutely right. There's. There are a ton of fireworks.
Demetrios [00:18:32]: I forgot about them.
Aditya Naganath [00:18:33]: Yeah, ton of folks who are, who are also operating at that compute layer. Right. Which a. I would still demarcate as separate versus the LLM Ops category. So that's number one because I think of them as actually core providers of, of infrastructure in some sense. Right. They are ultimately providing compute, while to me LLM Ops is more about, hey, are you providing some tooling that kind of works around the LLM so it feels one layer lower to me.
Demetrios [00:19:07]: Okay, so hold on, let me run this back to you because I think this is the first time that I've thought about it that way. You can use AI and not have a AI infrastructure company like these LLM OPS tools that you're talking about, but you can't use AI and not have a gpu.
Aditya Naganath [00:19:25]: That's right. That's right.
Demetrios [00:19:27]: I see. Yeah. All right, all right. It makes a lot of sense now why those folks are in their own category as opposed to all the rest like the evaluation or the orchestration tools.
Aditya Naganath [00:19:37]: That's right. It's. It's just about the layer of abstraction that, that the, that you're operating at. So coming back to your question, right, There's a ton of demand for compute, right? And that's because on one end you have people who are training their own models and that takes a ton of compute. And then once these models are trained, you obviously have them being slotted into applications and then running inferences as a result, which is again, compute on a gpu. So the claim that or the work that folks like Together are doing is, hey, you know, we will provide some really, really interesting pieces of software that optimizes the utilization of these large scale GPU clusters, which then allows you to be, you know, at the kind of the apex of this, you know, cost and latency curve or Frontier. And if you mentioned certain other companies, right. And some of these companies, what they're doing is they are acquiring GPUs and then kind of passing them through to the end user without much of those optimizations built in via software.
Aditya Naganath [00:20:40]: So I think that's one thing to note, right. Like ultimately, if you again, look at the work that companies like Together are doing, they're doing deep research right, across training and inference. You know, for example, Together has a chief scientist named Tri Dao and he's the inventor of this thing called Flash Attention. And it's basically a way to train LLMs more efficiently. Right. So he's commercializing this into Together's platform. Then, you know, on the inference side, you can do some pretty nifty things with speculative decoding and what have you. And ultimately what this boils down to is can you offer Compute more cost effectively without compromising latency and still have pretty good economics.
Aditya Naganath [00:21:16]: I think that's the key piece to understand here.
Demetrios [00:21:19]: So. All right, I can see that now. Let's go one level up, maybe two levels. If we're coming from the GPU infrastructure area, then we go, we bypass the whole LLM ops area and we go up to the application layer. And I know that you've had some excitement around there and it feels like that's where things are happening. We just got done with the agents in production virtual conference. There was a ton of energy around it, like a ton. It feels like it is the new thing.
Demetrios [00:21:58]: Everyone's learning together. We had some great people that were sharing their knowledge, but nobody is an expert in this, right? Nobody's been doing it for more than a year. And even the team that we did it with, Prosys, they started their journey with agents, which really was in the beginning it was just a rag. And that started two years ago. And it was about a year ago that they really started going with the agents. But that's one area of what feels like the application layer and how people are using that. I know it feels like one aspect that has been very clearly defined to have business value when it comes to Agents is the customer support. Have you been seeing other areas where it's obvious that's going to be an AI application area and we need to bet big on it?
Aditya Naganath [00:23:00]: Absolutely. So the application layer, first of all has been the most exciting to us at this moment in time as investors at Kleiner Perkins. And to directly answer your question, software development has emerged at this pretty obvious application of LLMs. Right. And for now it's this autocomplete use case which is how do we essentially provide useful suggestions to developers within their IDE that allows them to move a lot faster. And you know, it's a pretty beautiful use case if you think about it, especially in the context of being a wedge. Because on one end you had, first of all, GitHub Copilot educate the market back in 2022 about why this is even useful. Right.
Aditya Naganath [00:23:46]: Which was very, very helpful to a bunch of startups that emerged in 23 that haven't had to pay that tax as a result, and they've grown very quickly. But the other things to note are one, it's a use case where the LLM will get it right, let's say 30% of the time. And when it gets it right, it's a very helpful thing for a software developer. They can move a lot faster. But if the suggestion is wrong in that 70%, it's not devastating. The developer just doesn't tab complete and then they try to figure it out on their own. And so as a result, you have this use case where there's a pre provable productivity gain with at the same time, you know, tolerance for error. Right.
Aditya Naganath [00:24:29]: So every CIO has now decided that they need to equip their software developers with some kind of coding accelerator and that's opened up a huge market. Right. As a result, I, you know, so we have a company called Codium in our portfolio that's actually leading the charge here.
Demetrios [00:24:45]: Yeah, Varun, that's right. Came on here and that was, I think almost a year ago. And after we stopped recording, he was like, yeah, man, we used to be in the GPU space, but we got out of it and it's all for the better right now. We're doing really well.
Aditya Naganath [00:25:05]: That's right. You know, he is operating in, I would argue, like the poster child market for this first wave of application companies. It's one of those markets where you can talk to any enterprise, any CIO within that enterprise. No one's going to disagree that they need one of these things. Right. So we're very, very privileged to be in business with someone like Varun who's building Codium. And then I would say the other market is enterprise search. Right.
Aditya Naganath [00:25:30]: And so we are in business with a company called Glean, that's leading the charge here. And that's another interesting case study where, you know, Glean, first of all, the team is phenomenal. The CEO Arvind, he was one of the co founders of a company called Rubrik. They have one of the most technically stacked teams out there. When ChatGPT came out in 22, it was obviously the watershed moment for AI. And I would say what was interesting about it again was that a lot of CIOs were like, hey, I want this on top of my own data. So it ended up being like a, again, market education phenomena where OpenAI was pouring all these resources into making ChatGPT a consumer product. You had these CIOs who obviously were fiddling around with ChatGPT saying hey, it would be great if we had this on top of our, our own data.
Aditya Naganath [00:26:20]: You know, Glean had already built a bunch of these connectors to applications and data sources and were very quickly able to launch their own assistant that was privacy aware. And you know, it's been lights out from there. So that's been another use case that's emerged as pretty consensus at this point where every enterprise feels like they need it. And then we have seen, you know, certain other use cases start to emerge and be very interesting. Right. So we're in business with a company called Synthesia. They provide avatars for the enterprise and we can talk about that. We have some companies in stealth that I would say I'm very excited about because they're using LLMs in a smart way in conjunction with well thought out product to, you know, go after workflows that were previously like inaccessible, where you could have only owned say 70% of that workflow.
Aditya Naganath [00:27:09]: But now again, by infusing the product with alarms, you're able to go after the entirety of it because some of those workflows involved, you know, reasoning over unstructured data. And now you finally can. Right. So that's, that's what I'm excited about, at least from this first wave. But as I'm sure we'll get into, this is only a stepping stone for what's to come. Right. You know, the whole dream for this AI movement is can you get these things to be able to reason and well enough such that they can resemble, resemble a human being and as a result be a coworker versus a co pilot.
Demetrios [00:27:44]: Yeah. And I want to get into that coworker versus co pilot because I think that's a great way of putting the current versus the future scenarios. And. But first I'll, I'll address a few of these points on just starting with Codium. And when Varun came on here, he was the first person to talk about how much easier of a problem evaluation is when it comes to code versus when it comes to unstructured data of language. And he's like, if it runs, then you're kind of good. It may not be the most efficient, it may not be the best, but it still runs. So if, if it compiles and you're happy, then the other piece that I always think about when it comes to the coding tools, and especially the AI coding tools, is a segment of the evaluation metrics.
Demetrios [00:28:42]: If I were running it right, I would be a hundred percent looking at how much of the code that I suggested is still in production after three months or six months, because that's how you can know like the quality of this spaghetti code or non spaghetti code that you're spitting out. Then moving on. Let me just remember what else you were talking about. Was the next one that, oh, Glean completely took over the market. So the funny thing for me is that is it's almost like there's Glean or there is, you're doing it yourself. And I know there's probably a ton of other tools in there, but what has been so amazing to me is how Glean took everything and just ran with it. And every time you hear about them, they're raising another hundred, $200 million. And so it's outrageous.
Demetrios [00:29:40]: But the chat with your data is the quintessential hello world for AI. So the fact that Glean has had so much success, when going back to what we were talking about earlier, how hard is the problem that you're dealing with? You would think that it's not that hard because everybody is creating this chat with your data. But then you recognize that chat with your data when you have a few PDFs versus chat with your enterprise data and across all of these different sources, that and making sure that the right people have the right access to the data. And then you are constantly updating this access and constantly updating the data that's inside, that's where things get a little bit more difficult. And so I understand why Glean took the market on that one.
Aditya Naganath [00:30:33]: Yep, that's a great summary. That's a great summary.
Demetrios [00:30:37]: So the companies that are infusing it, I also Wonder about how you feel when it comes to the no code, low code with AI and LLMs, because I've seen quite a few use cases where you get someone that is on the sales team or that is in HR or they're on the marketing team and they're able to create immense value for themselves because they spend a weekend or they spend maybe two weeks playing around with one of these low code, no code tools, recognizing that they can automate, like you said, 70% by themselves with their own workflow that they like and they're able to just hit the ground running.
Aditya Naganath [00:31:23]: That's right, yeah. We, one of the beautiful things about this movement is that, you know, LLMs again are, are a higher form of abstraction. And you know, before if you had to unbundle them, you'd have probably had to write code to sort of simulate the outputs of what you're getting today with LLMs. But today all that is now has now been pushed into the weights of these models, which basically means that non technical folks can do a lot more. Right. Because you have LLMs that have, that get you pretty far along. So as a result they're able to build and construct, you know, bespoke applications, bespoke workflows without any help of a developer, without needing to be technical. Because LLMs now get them pretty far along.
Demetrios [00:32:12]: Yeah, I do see different people wanting to take some of these low code, no code solutions or features or whatever they create and then infuse it into the product that they're working on. And that's where you get this gigantic divide. Because you go from a low code, no code MVP type thing that works when you hit run and there's one or five people on the team and they're using it sparsely versus this is now a feature in your production application.
Aditya Naganath [00:32:44]: Right.
Demetrios [00:32:44]: And so that divide and that disconnect, I, I think is still not super close, but who knows where we'll be a little bit down the line. And the, the, the other thing that I was going to ask, just when you said LLMs and it brought up in my mind, how are you feeling about LLM providers in general?
Aditya Naganath [00:33:14]: I would say it's just like an interesting battleground there. Right. So you know, on one end, you know, you have OpenAI and anthropic that are pushing the limits of research and coming up with all sorts of new, you know, methodologies from the way they handle data to the way, you know, obviously the algorithms that they're using under the hood. And then, you know, I think they also even want to go down to the data center level and kind of own the optimization of compute and networking, even at that layer. But then you have, you know, the matters of the world that are, that are using a bunch of the free cash flow that their core business generates to outdo these guys. Right? And so it's very interesting to see. And so far, you know, by all benchmarks, OpenAI and Anthropic are still ahead of the open source ecosystem. But that gap is only closed with every epoch of model released.
Aditya Naganath [00:34:07]: And again, like coming back to Together, Together is anchored on the fundamental belief that open source models will be good enough for the enterprise, such that enterprises will use them at large and then we'll need to find a way to serve them efficiently and as a result use platforms together. So it's a very interesting battleground. And you know, again, like, as we know, models are the fastest depreciating asset. You pour all this money into them and they're impressive for a couple of months and then someone else comes out with something that's better or better according to a certain type of benchmarks, and then you can ask the question, hey, like, was that investment worth it? What's interesting about OpenAI strategy is that they, they've been smart about it. They've, they've been integrating their models into ChatGPT, right? And ultimately and monetizing via subscriptions, which is, you know, higher quality revenue than API calls. And that is a good strategy because at least, you know, you can, you can make the ROI case for model training and for having to, you know, essentially burn more cash on bigger and bigger models. But there is this open question as to will the scaling laws hold and then what happens if they don't? And you know, how exposed are the model provider companies if they don't hold?
Demetrios [00:35:27]: Exactly. That's where I'm always wondering is, oh, we're going to hit that point of diminishing returns. And then I like the idea of, yeah, they're exposed. Now you find out that when the tide goes out, who's not wearing their clothes.
Aditya Naganath [00:35:44]: Exactly. And it's a scary thought for these companies, right? Because again, all of their competitive advantage thus far has been from being able to provide these models with, you know, billions and trillions of parameters. But, you know, for, if we're reaching this saturation point for reasoning, right, then it's scary. Right. And again, OpenAI has been smart by at least having this kind of brand value and distribution with ChatGPT that will still allow them to be a sustainable business. I Think anthropic needs to think about what their angle here is. But you know, to me, I think the two interesting questions are. One, you know, is this test, time compute, approach the next frontier, or do we actually just need to have something that goes beyond the transformer? The transformer has been very successful.
Aditya Naganath [00:36:32]: Right. And we stretched it to its limitations. But do we need something new?
Demetrios [00:36:38]: Which has been what I've been thinking a lot about and in the same camp, but you're almost expecting like a potentially like a once a lifetime event to happen because, yeah, we need to go beyond the transformer. That's probably, I imagine when deep learning was all the rage in the 70s and then the 90s and the 2000s, early 2000s, there was that same kind of idea. And it happens at least decades apart. So thinking that, all right, this is going to happen before these companies get exposed is a really wishful thinking in my eyes. And, and I also wanted to just mention that there are these other companies out there like the Mistrals and the Coheres, and I really wonder what going to happen to them. Right. If the Anthropics and open AIs are going to get burnt, then where is coherent Mistral in the conversation, if at all?
Aditya Naganath [00:37:49]: Totally. Yeah, I think that's, that's a big, it's a big question for them and their investors. And you know, they were always playing with fire a little bit by trying to essentially outdo these guys at, from a model competence standpoint. And you know, it's always been hard for these independent providers to do these, these models and they may have, you know, their moment in the sun for a couple of minutes, but, but it's quickly washed away. So I'd be worried if I were them.
Demetrios [00:38:18]: Yeah, yeah, yeah. So, all right, getting into the idea of co pilots versus coworkers, what are your main thoughts or assumptions on how that's going to play out?
Aditya Naganath [00:38:31]: Yeah, look again, I think coming back to this point about reasoning, it's about how do we, how do we cross the bridge from what we have today to, you know, the next level of reasoning? Because ultimately, again, this whole AI wave is exciting if we can stretch the limits of reasoning and get these things to act like human beings and do productive knowledge work as a result. And to me, a couple of just underpinning concepts that I've been thinking about is, you know, how do you, how do you essentially leverage things like reinforcement learning? Right. And how do you leverage techniques like, you know, Monte Carlo tree search and what have you that have been successful in things like Alphazero for chess playing as an example and kind of bring that to these models to get them to be able to be self aware, reflect, intelligently, prune a search space, right? That to me is the next frontier of reasoning. And I think, I think we need to solve this reliably to unlock these co worker products, right? And if you can unlock these coworker products, then you can start pricing as a fraction of, you know, wage spend, which is then when the markets become really big. Because again, if we look at these, these really exciting AI companies today, today they're great and in some cases they've created new categories out of dust. But they're SaaS companies, right? And that's fine. Nothing wrong with SaaS. But you know, for all the investment that's pouring into AI companies, if what we're getting out of it are SaaS companies with pretty known multiples, it's not going to end well for a lot of investors.
Aditya Naganath [00:40:08]: So you actually need these coworker products to start hitting the market to justify the investment because that's when they start tapping into a much, much bigger pool of dollars which is essentially spend on labor.
Demetrios [00:40:21]: So a bit of a tangent from what you just said that has constantly been going on in my mind is if you think about agent companies right now, it used to be back in the day when you had engagement and you had people that were using your product, that was a very good thing. But now because of the way that the pricing structures are, if someone is using your product, the more that they use it, that cuts into your profit margins. And I saw this very clearly when we were doing the agents in production conference and they, the guys from the OLX team were showing me their new agent, OLX Magic. And they were giving me this demo and I thought it was hilarious because I said okay, now I'm thinking about buying a new car. Let's. And they said yeah, yeah, we can do that here, watch. New car. You what kind of car? And I told them Toyota hybrid.
Demetrios [00:41:20]: So we typed that in and it was based in Poland, so it was in Polish slotskis and forgive the pronunciation for anybody that's based in Poland because I love calling them Schlatzkis. Just go with it. All right? So then I said, well can you. How much is that in euros? And they said, oh yeah, yeah, we can ask that. So they ask we get the prices in euros. And then I said okay, we'll now ask how can I convert a Polish license plate to a German one because I'm in Germany. And they go, no, no, no, we have guardrails on that. You can't just use this, like chat gbt, man, give me a break.
Demetrios [00:42:00]: And I'm like, but that's related to my question. Like, if I'm going to buy a car in Poland, I want to know all these things. So they said, well, yeah, but you got to go to some other provider for that. We can't just be basically giving you this for free. And so that was a huge click in my mind on. When you're building these products, you can't let the user do everything that they want or you don't have that ability per se. Because if you're rolling it out to millions of users and then each user is doing on use seven or eight calls to a model, that can add up really quickly.
Aditya Naganath [00:42:43]: It's a great point. It's totally a great point. It's, it's like every inference is essentially, you know, cogs, right?
Demetrios [00:42:50]: You hurt, you squ. Oh, they're asking another question. Oh, it's so, in a way you're like, yes, I want them to use it. And they told me they have a metric that if it's two or three, it doesn't do anything to conversion. And if it's after 7, it also doesn't do anything to conversion because it's just somebody that's playing around with it and trying to see what it can get. But from that three to seven, that's the sweet spot. And they see conversion really went up, so it made it worth it for them. But I am constantly thinking about these seven plus where maybe you do want to know all these answers and, or that you have all these questions.
Demetrios [00:43:32]: But they, they are trying to think about ways to cut you off from after you've asked seven questions. They're like, yeah, actually this is down for the moment.
Aditya Naganath [00:43:42]: Yeah, it's a, it's a great point. And it kind of, if you walk that backwards, it actually points to how under optimized we are at the data center level. Right? Because ultimately these applications are paying attax to the model providers in the form of API calls. Why are those model providers charging what they are? It's because they have their own underlying costs with how much it took to train the model and serve it. Right. And then it points to, okay, then how is model training happening under the hood? It's so under optimized, right? Like you essentially have, you're burning a lot of compute, right? And you know, at the end of the day, the networks, at least at the moment, in terms of the way they're designed are pretty under optimized for these kinds of workloads and that's only pushing up costs. Right. So we, and then GPUs are expensive because of the whole supply demand imbalance.
Aditya Naganath [00:44:31]: And we just need a lot of pieces to come together at many different layers. Right. Like a, I would say we need like, you know, some challengers to Nvidia's dominance, that we can bring overall prices of compute down. We need network fabrics to be a lot more efficient. Right. So that LM training costs go down. And then you just need to be able to have these, you know, chips get ever more powerful so that you can rely on fewer of them, which again will push LM costs down and that trickles them all the way up to lower costs for, for a model to be served.
Demetrios [00:45:06]: Yeah, and lower price reliable like the GPUs are so unreliable. And I think my favorite way of hearing this was when Todd Underwood gave a talk at the AI Quality conference we did, and he said you can look at a GPU the wrong way and it'll go offline. You can walk around a data center and because of the way that your feet are hitting the ground, you just blew out a whole cluster right there. And obviously he's making a joke, but it shows you how weak these are. And if you read any of the blogs, you can see how GPUs going offline is going to happen no matter what. And folks are just trying to figure out how they can deal with it and quickly get them back online or minimize the blast radius when it goes offline so that they can switch those training jobs quickly to other pools.
Aditya Naganath [00:46:01]: So true. And it's, it's, you can imagine how complex this problem is at scale. Right. When you have, you know, you're trying to train the biggest models that are distributed across thousands of GPUs. And you know, you, you first of all are trying to get max out the utilization, the flops in particular from these GPUs. And if it goes down, then it can actually impact entire training runs. Right. You, you, you really can't afford for them to go down.
Aditya Naganath [00:46:26]: So. Yeah, and that, that by the way, contributes to higher costs. Right. So the, it, to me it feels like, you know, trading costs are like, you know, 3, 4x what they, what they could be if we had more reliability of GPUs.
Demetrios [00:46:41]: Well, knowing all of these inefficiencies in the training and the GPUs, does that not make you want to Go and try and invest at that level or is it because there's only a handful of model trainers? It's like, yeah, I don't know if it's worth it.
Aditya Naganath [00:46:59]: I do think investing close to the data center is interesting, right? And again, I think venture investors who've put money into a company like Vast have done well or they're pretty happy. And then again, you know, people who've invested in some of these compute providers have done well. So there's something there. I actually find networking pretty interesting. I actually feel like for the first time in a long time there, there's a clear why now to invest in a networking company, right? Like the way, you know, at least the hyperscalers are approaching LLM training is they're literally provide provisioning separate networks just for LLM training. But even still, that's inefficient, right? Like the hardware could be a lot better than what it is today. There's a lot of talk about, for example, whether network interface cards should be smart. There is a lot you could do in terms of how you provision a network and essentially how do you get it to be a lot higher throughput, higher bandwidth.
Aditya Naganath [00:47:56]: So there's something interesting there for sure. Go to market will be very tricky for companies operating at this layer. And I think that's what we have to factor in as investors.
Demetrios [00:48:06]: Why?
Aditya Naganath [00:48:07]: Because you have, you first of all have. On one end, you're selling to a pretty narrow customer set, that's one. The second is you do have existing players, right? You have, you know, the Broadcoms, you have the Aristos, you have even to some extent, I guess like a Cisco that are trying to move into this space. You have some of these guys that are also doing their own work, right, Like Azure. And I even know Facebook is kind of like investing in innovating themselves at this layer. So then what is it that you're going to do as the quote unquote wedge, right, that all these guys buy from? And then what does that get you? I think that's. Those are two interesting questions to answer.
Demetrios [00:48:49]: But do you think there are credible solutions out there that are able to increase the throughput? Because I, I can imagine if you did have something that you could just show, hey, look, 2x in the throughput here, you're golden.
Aditya Naganath [00:49:07]: Yeah, I think people are working on this. I wouldn't say there's some, there's a silver bullet just yet, right? Because you can show this at like, you know, subscale, but can you prove it at that? Kind of scale. I think that's hundreds of thousands of GPUs. That's right.
Demetrios [00:49:22]: Is a whole different beast than just connecting two GPUs that are right next to each other together.
Aditya Naganath [00:49:27]: That's right, yeah. Yeah, I could see that Nvidia itself is doing a lot of work here. Right. So you have to overcome that beast as well. Like as we know, like when a box ships, it's typically got four to eight GPUs that are interconnected via this thing called NVLink. Right. So then you're. So you're not going to really beat that.
Aditya Naganath [00:49:46]: Then you have to probably play in this kind of like internode space. Right. And how do you make communications across these nodes for LM workloads? A lot more efficient.
Demetrios [00:49:57]: Yeah. And you need to just go after folks that have lots of nodes, so you're instantly looking at a really long sales cycle. You're looking at, how are you going to get someone to commit to using you? It. I can see the go to market would just be really difficult. So it makes it hard to want to invest in something like that. And how much can you saturate a market like that? If I wouldn't know, because I am in no means, by no means an expert in this, but I would think like after you've sold to OpenAI and Meta and Anthropic, maybe you're kind of good. Then, all right, you can go after Xai, you can go after Mistral, and then who else? Or maybe there is that. Well, once it becomes a standard, everyone's going to use it because it's better.
Demetrios [00:50:54]: So it doesn't matter if you have five GPUs or if you have 5,000.
Aditya Naganath [00:51:00]: Yeah. And look, I think if you can overcome some of these challenges that we just talked about, you know, I would say, like, yes, while a lot of investors will say customer concentration is bad, like there are successful companies we can look at that have immense customer concentration.
Demetrios [00:51:20]: But they're doing great.
Aditya Naganath [00:51:21]: But they're doing great. You know, Nvidia is not. Nvidia arguably is a company with a lot of customer concentration. Right. So I think the question is, how do you kind of overcome some of those hurdles? And if that results in you having, you know, a few handful of customers that are paying you an insane amount of money, that's not bad. And then, by the way, you know, inference ends up, in my opinion, being the big opportunity because, you know, ultimately every business is going to have LLM applications, as we know. And, you know, inference is just going to be such a pervasive workload, you know, many, many orders of mantu bigger than training. So if what you built is gives you the foundation to go after inference in some shape or form, then by all means, yeah.
Demetrios [00:52:06]: So we had a sponsor. We have a sponsor of a big company, big cloud company that I won't say exactly who it is, just to keep them unnamed. And I was talking to them about why they wanted to sponsor us and they were saying, yeah, well, we have a lot of people doing AI on our cloud and we want to know more about what they're doing and we want to get closer to them. So I asked, oh, yeah, really? We have so many people that are training models. Is that what's going on? And they said, no, no, no. The majority of the money is being made on inference here. Don't get it twisted. This is where things are happening.
Demetrios [00:52:49]: The training is great, we love it, we're not going to turn them away. But the inference is where the real cash is being made.
Aditya Naganath [00:52:56]: That's right. And, you know, I think with this whole test time compute paradigm at least seeming interesting, I would say the jury's out so far in terms of how effective it can be. But, you know, if that paradigm continues to yield exciting results, then. But no doubt inference is going to be, you know, way, way, way bigger than training. It's going to eclipse training as we know it.
Demetrios [00:53:17]: Yeah, yeah. It's fascinating, man. Well, before we jump, anything that you are super excited about something maybe that you just recently invested in or that you're looking to invest in, the types of companies we talked about the application layer, we talked about customer success or the coding. You kind of got tools that you already invested in there. So if somebody's doing stuff in that space, I don't recommend that they reach out to you. If somebody's doing stuff in what space should they reach out to you?
Aditya Naganath [00:53:49]: Yeah, I'm really excited by people that are trying to push the frontier of what agents are capable of. And again, comes back to what we talked about. Can you build a application that is able to truly automate a useful unit of work? Right. And so just to give you some examples, right, like there are companies that are trying to, you know, automate the work of security operations analysts. There are companies that are trying to, you know, automate the work of BPOs. There are companies that are trying to automate the work of site reliability engineers. These are just like really interesting potential applications where the underlying technology is. Is very hard to build because you have to, again, get these things to be able to reason reliably over, you know, large swaths of data, multimodal data sets.
Aditya Naganath [00:54:38]: And anyone who can crack that will find themselves in a. In a pretty big market. So, you know, people who are thinking along these lines, trying to push the frontier of reasoning for agents where there's a pretty tangible, you know, value proposition at the end of the day. I'm very excited to meet those people.