Anatomy of a Software 3.0 Company // Sarah Guo // AI in Production Keynote
speakers
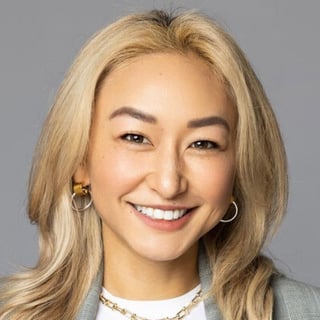
Sarah Guo is the Founder and Managing Partner at Conviction, a venture capital firm founded in 2022 to invest in intelligent software, or "Software 3.0." Prior, she spent a decade as a General Partner at Greylock Partners. She has been an early investor or advisor to 40+ companies in software, fintech, security, infrastructure, fundamental research, and AI-native applications. Sarah is from Wisconsin, has four degrees from University of Pennsylvania, and lives in the Bay Area with her husband and two daughters. She co-hosts the AI podcast "No Priors" with Elad Gil
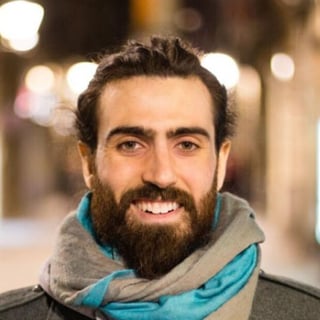
At the moment Demetrios is immersing himself in Machine Learning by interviewing experts from around the world in the weekly MLOps.community meetups. Demetrios is constantly learning and engaging in new activities to get uncomfortable and learn from his mistakes. He tries to bring creativity into every aspect of his life, whether that be analyzing the best paths forward, overcoming obstacles, or building lego houses with his daughter.
SUMMARY
If software 2.0 was about designing data collection for neural network training, software 3.0 is about manipulating foundation models at a system level to create great end-user experiences. AI-native applications are “GPT wrappers” the way SaaS companies are database wrappers. This talk discusses the huge design space for software 3.0 applications and explains Conviction’s framework for value, defensibility and strategy in specifically assessing these companies.
TRANSCRIPT
AI in Production
Anatomy of a Software 3.0 Company
Slides: https://docs.google.com/presentation/d/1ZSu19GNtXuxwmMT5QFK3cyphJHhE4nd1/edit?usp=drive_link&ouid=112799246631496397138&rtpof=true&sd=true
Demetrios [00:00:00]: Alright, again, I'm gonna reiterate, there are two Thursdays in a row. Let's do this. First up, we've got our keynote, numero uno. So we had you on a few months ago. I loved what you were saying. I was really appreciative of your thoughts and I thought, let's get you back on here because you're doing some incredible stuff at convention. Conviction. You're VC in it, and you've got your own podcast where you're interviewing some top names in the business, which is called no priors for anybody that wants to check it out.
Demetrios [00:00:37]: And the best part is, we get like a little bit of a two for one here because you've brought along a friend, right? You've brought Pranav up onto the keynote also. So this is super exciting. I'm ready for you all to take over. Let's see what you got. Do you need to share a screen or anything or you just do?
Sarah Guo [00:00:59]: Yeah.
Demetrios [00:01:00]: All right, let's see it. Take a moment. Share your screen while you're doing that. All right, sweet. So I see it. I'm going to put it live and I'm going to let you all rock and roll. I'll be back in 20 minutes. Anybody who has questions, feel free to drop them in the chat and I'll make sure to ask Sarah and Pranav when they're done.
Demetrios [00:01:21]: See you all in a sec.
Sarah Guo [00:01:23]: Awesome. Hi everyone. As Demetrius mentioned, my name is Sarah Gua. I am the founder of an early stage AI focused venture firm called Conviction. This is my partner, Pranav. Ready? And just to give a little bit of quick background on us, we're in many ways classic venture. So we work with a small handful of companies in a very long term way, except they're all AI companies. So some of the people we're in business with include essential base ten, which Demetrius mentioned, foundry, Harvey Hagen, Mistral, Pika and Sierra.
Sarah Guo [00:01:58]: And there's a whole range from, as you can see, like foundation model companies, like Mistral, to infrastructure companies, to different application layer companies. And we try to differentiate on being close to research and technology, to the community of people who are working on the state of the art or putting AI in production and then taking a real point of view both on the domains where we see the most opportunity and also the shapes of company that are interesting in this era. And when Demetrius suggested we do a talk, I figured we should talk actually about maybe the question that we get most often, which know, what does software in this era look like? If not a GPT wrapper and are there actually defensible applications? So I'm excited to talk about where we are in 2023 and the future.
Pranav Reddy [00:02:53]: Yeah, we think of 2023 as the first year of AI infernops. We saw everything from closed and open source foundation models, vector databases to operationalize some of those outputs, new modalities like audio, and generally the growing ability to train host eval models with inference and labeling providers. There's a lot of categories that we haven't even listed here, and even within these categories there's a ton of variety in the shape of offering and implicitly what they think is important to customers. So we asked 70 of our engineering and product leaders how they build with AI. On the left you see a stacked, ranked importance of what tools they thought were most useful to them, and pretty consistently we were told that inference, including the foundation models and embedding platforms, were the most important of those. I think it's still pretty clear that OpenAI is the most important of those closed source foundation models, with a minority of respondents saying anything other than the ones that we listed. But that usage of public APIs we don't think is the same as dependence we're seeing a growing number of companies work on fine tuning smaller models and run their own, primarily based off of Mistral's couple of open source models. And some of this tooling and consolidation is why we think 2024 will be the year of AI applications, not just infra.
Pranav Reddy [00:04:05]: And increasingly the second part of that is that we think we're still very early in what the enterprise adoption curves looks like. We spent a lot of time talking to customers, and a lot of them over the past year were still unsure how to navigate, what to build and buy internally. Based on the CEO mandate that they need to build and buy AI, and therefore what they'll spend on externally, we think that's getting a lot better, and that customers are increasingly both willing to spend and understand the utility of what AI applications are for. Finally, I think we're all still learning what AI product surfaces look like. We think a foundation model is like alien technology that's dropped on earth, and we're all poking and prodding, trying to understand what they're capable of, and also how to marry that capability with what kinds of surfaces humans want to interact with. The vast majority of AI applications, from the people we surveyed, still look like chat today, but we think there's more and more exploration and creativity around what that service should be.
Sarah Guo [00:04:59]: So this might be the billion dollar question. We'll start with a framework of what do we look for in companies? And this is a very generic framework. I don't think it will look that different from what you see other vcs look for market position, what the value prop is strategy to enter a market and what category. You're really attacking some sort of product and technology advantage, specific features of teams, and then understanding the actual economics, the business quality. And I've given a whole presentation on this generically many years ago. But what I thought we could cover today was what changes in the age of AI? And you don't need to read this, we'll go through each one individually, but I don't know how many of you will have seen this template. This is from Jeffrey Moore. It is a positioning statement and I think it's very useful.
Sarah Guo [00:05:52]: It's like mad libs for companies. But the claim I would make is like actually me and Pranav and most of this community, people are really, really excited by capability that start companies. And so you get a lot of capability. Forward thinking. Oh, I think a foundation model can do this, or I wonder if we can train a model to do XYZ. And what we're actually saying is that we think this sort of customer back thinking, starting with the for what customer who has some problem. There's a real scarcity of this, right. And so instead of figuring out how to optimize X technique, which is implementation, working backwards from the customer, we think is going to be a dominant orientation for application companies that succeed.
Sarah Guo [00:06:40]: The sort of second dimension that I mentioned, that is just a core component of what we look for in companies is strategy, some sort of market entry opportunity, whether people understand the category that they're selling or evangelizing. One of the things that excited me most when we started conviction, I used to be a partner at a larger venture firm called Greylock that was more generalist, was that traditionally at a generalist venture firm, your investing strategy probably mirrored the set of overall opportunity where most of the companies you backed were joining existing categories. Right. We are going to do XYZ, and that might be CRM, it could be something in, it could be log management, it could be a security company, but we're going to improve on it incrementally in price or performance or some feature. Right? And the big opportunity that we see in AI is actually on the right hand side here, defining new categories. These are markets that have not existed before, right? You won't see market research reports about them yet and they start vanishingly small. If we look at some of our portfolio companies. Or even the best example, OpenAI.
Sarah Guo [00:08:00]: The market for foundation model APIs was zero five years ago. Right? The market for legal copilot was zero five years ago. The market for video avatars was zero a year ago. And so these markets tend to start very small, they're explosively growing. But the advantages are quite different because you're selling brand new capabilities. You can develop a brand, you can have very high market share, even monopoly market share. And we think the opportunity to create a business that is higher quality can become a public company, has really loyal customers, is very, very different. And that's super exciting.
Sarah Guo [00:08:34]: It also comes with specific challenges. From a strategy perspective, if you are selling something that your customers have never heard of and may not know they need, then you need to define the use cases you are responsible for. Awareness and developing budget for the customer, figuring out pricing the entire company design is a much more open question. One concept we think a lot about at conviction is the idea of MVQ minimum viable quality. So for any function that a customer might want, what does it take for the product and the set of models and systems around it to meet that floor? And we can talk more about that.
Pranav Reddy [00:09:18]: Yeah, on the product side, I think that's why we've seen a lot of what look like just to be GPT wrappers. Lots of the use cases that are actually commercially valuable just work out of the box. And because of that, that's all people do. But sometimes it doesn't quite work out of the box. So with a little finessing on the prompting side and some post processing to get outputs and evals to check in on how you're doing, everything just works. And then sometimes for some applications you need it all. You have small models, big models, orchestration frameworks, memory and tool use. And eventually, I think for some products, the right answer is let's think about it as a system, so we can think about scoping and how to handle failure across the product.
Pranav Reddy [00:09:57]: And to expand on this a little bit more, let's consider an example that we've seen a lot of AI search engines, whether it's perplexity or Chat GPT as a search replacement, or even the new rumored OpenAI search engine, they're pretty popular. So let's debug a tough query. What was the score for the warriors game? If we try just asking, a language model doesn't really seem to work. This is chatt classic, doesn't have Internet access, doesn't know anything about what's happening in the warriors season. But even if you grant the model, the ability to run its own search. Still not enough model concludes incorrectly on gives me like a game from the last year's playoffs and incumbent search works. It has the answer, but it's still not perfect. It's not a single answer.
Pranav Reddy [00:10:35]: I still have to parse a bunch of web Ux and so what we're left with is we have a bunch of options for how we might solve them. You can mess with a prompt, pretrain, fine tune, and all of these are valid ways that we might get the model to answer this specific question. I've spent some time working on building a consumer search engine, so I can tell you the right answer is probably like we want to parse freshness, seeking intent on the query side to know the user wants recent information and then combine that with crawl signals. Unfortunately, I don't think there is an easy way to describe that trade off. Traditionally you'd think about latency and quality, and those are how we'd define old applications. But increasingly we're coming across new criteria that we don't think anyone's had to deal with before. These are things like safety for what people might enter into the model. It's nondeterministic output, so you need to measure consistency in some way and understand the full landscape of what people will do.
Pranav Reddy [00:11:25]: And therefore, I think the best teams, we think the best teams are the ones that are customer driven, and figuring out how to leverage technology to work for them.
Sarah Guo [00:11:34]: Teams is probably the fuzziest thing to talk about. So we'll try to use a few real examples to talk about the features we think are dominant in this era. Gabe and Winston are the co founders of an application level company called Harvey. I'm very confident Harvey is not just a GPT wrapper. OpenAI is an investor in the company as well, so they think there's something there. Gabe comes from a research background from meta and prior DeepMind, and Winston was a lawyer. I don't know a lot of lawyers besides Winston that are hacking on GPT at the time, three three five in their spare time. But he was really sick of the toil of low level document processing in his job at a major law firm.
Sarah Guo [00:12:20]: I think it's a good example of a combination of research understanding and domain understanding that we think is really potent in terms of actually solving the customer problem. If we go to Joshua and Wayne, Joshua was the first tech lead for the ads platform at Snap. Wayne was the head and then he worked on lenses and Snap research and a bunch of different things in image generation and computer vision. And Wayne was leading product and design at smule. So both of them had worked around advertisers and creators and were really obsessed with this idea of the camera and the ability to create content that was AI driven and much easier for consumers and businesses. So when they started, hey Jen. They really start with the premise of what would it take for us to generate video? That's Sarah and Pranav or Demetrius or any of us speaking perfectly out of the box with consumer quality input and full flexibility. And I think that is a hugely ambitious problem statement.
Sarah Guo [00:13:24]: But the speed of the team, besides we just talked about research and domain understanding is something that really stands out to us. The cycle time from new idea because a customer brought it to us or because somebody just thought it'd be cool to in the product is the best I've ever seen in a company. Demi and Chenlin are the co founders of a company called Pica Labs in the video editing and also generation space, so different type of content. And they had been Stanford researchers publishing and working with companies in the areas of image generation and video understanding. And their problem was very personal. They had tried to create a short film together, but they're not filmmakers, and even though they had ideas, the execution was incredibly hard. And so even despite all of the supposedly AI powered video tools that existed out there today. And then Bret and Clay come from Google, and Bret was very recently CEO of Salesforce.
Sarah Guo [00:14:30]: At Salesforce, Bret really understood how customers, large brands, were struggling to interact with customers at the quality and scale level that they wanted to. He'd also prior a long time ago, been CTO of Facebook. I think many of us have used frameworks and open source components from Facebook in that era, such as react, and their ability to marry the customer need around these high quality, safe support interactions and the modularity of understanding. Like how do I build a system that can serve multiple customers that isn't custom every time? I think is some of the reason that makes Sierra great. One of the things that you'll notice about all of these companies is the products that they have released are all like a year or so, a year and a half or less old, right? And so the fact that they all have really interesting traction and user love at this stage just speaks to the velocity. And I think that's really important in this era, just given the pace of progress in the research field overall and how dynamic our understanding is, as Pranav mentioned, of the product surfaces and interfaces, being able to leverage the joint learnings of the community of builders open source or even amongst different startups and larger companies is something that is going to benefit people with the most velocity. The final thing I'll mention here is just to talk a little bit about how dynamic I think the overall business model of some of these AI applications is and some of the considerations there. Here I am showing a slide from one of the snowflake earnings calls, and it's a slide that is very common in earnings and financial statements for SaaS and cloud infrastructure companies.
Sarah Guo [00:16:26]: And besides the fact that Snowflake is massive, you could squint your eyes at the last column here, their target operating model, and say like, oh, that's a great SaaS business, any great SaaS business, 78% gross margins, 25% operating margins generates 30% free cash flow. That's what we all want to get to. And Snowflake actually has of course, more compute cost than most, but I think it's a lot less clear what each new AI company looks like on both the revenue and cost side, right? So if we talk about revenue when we are doing generations, the traditional seat based pricing that has been dominant in SaaS, or consumption based pricing that has been dominant in infrastructure is. It's not clear that that's quite right. If you're replacing work, do you really want to be charging by seats if somebody doesn't need to grow their team? If you are pricing per generation, what do you do about the fact that some generations are far more valuable than others, and generations are more valuable to some teams than others, in some use cases than others? It's certainly not the highest value capture pricing model from the company perspective. And I think the sort of holy grail of the business model and pricing model for AI companies is whether or not you can really replace services, for example, video editing, and then charge like you're replacing first services. Instead of paying an agency, for example, you pay one of these companies for their tool, but you have the gross margins and sort of cost profile of a software company. On the cost side, everybody, I think, has thought about the cost of training and data acquisition here.
Sarah Guo [00:18:11]: But if the name of the game in venture backed companies traditionally has been capital efficiency, this is a really new dynamic, right? And thinking about how you can get to lower risk with your initial product market fit, how you can get the business itself to pay for training and acquisition, how you can do partnerships or otherwise be efficient, I think is a major thing that we think about. And then in the long term, projecting what the cost of inference, or the cost of foundation models, or even human in the loop for equality for some companies is a major consideration and it has huge implications for the product decisions you make depending on what the projections you have are of these different cost curves, right? So if I think that there's going to be another magnitude decrease in price and latency for the capabilities we have today, over the next year or two, I might be willing to chain together many more calls or sample many more outputs than I would otherwise. And the type of system and user experience that I create can be very different. So if we just go back to the framework we had at the beginning of every great young company, actually any company, but we just think about startups more needs to have these foundational pieces, market positioning strategy, a product strategy team, and business quality. We think there are elements that are unique to each of them in the age of AI. So just to sort of summarize that it's customer back, not capability, forward thinking. Despite our enthusiasm about all of these new capabilities, we think the biggest opportunity is around category creation, not joining of existing categories, and that our definition of software 30 is systems designed to manipulate these foundation models for use. Everyone here knows that putting AI in production, making users happy, is nontrivial.
Sarah Guo [00:20:18]: And the way we think of it is really that AI applications are GPT wrappers in the way that SaaS companies are database wrappers. Right. Salesforce started operating on Oracle, but that is not. Now it's much more valuable company than Oracle, but that's not what made Salesforce great. It was a component that was critical and eventually replaced actually. So we'll see what happens with foundation models, but it was the data model, the business logic, the ability to standardize an entire Persona within many companies, the sales rep around their workflows and then uniquely good distribution, all of the other parts of company building and software that made Salesforce great. And we think that analogy applies really well to the software 30 era. We talked a little bit about the dimensions that make teams uniquely good in this time period, and we think that's actually a huge opportunity for anyone who's leaning into AI.
Sarah Guo [00:21:20]: I like the joke of the year the iPhone comes out, or the year after the iPhone comes out. Every enterprise, every startup is hiring somebody with five years of mobile experience. It's not clear to me that five years of machine learning, like classical machine learning experience, is that useful in an area where everything is changing so much. But the extra 20 hours a week manipulating models and thinking about these system design choices we think is really differentiated. And then I think that people run into both huge opportunities and huge challenges on the business quality side, as things look a little bit less like standard SaaS. So this is inning, I think two in the AI revolution. It's happening really quickly and a lot of you are at the center of it. We would love to hear if you are starting companies or want to talk about any of the ideas we mentioned here or online and look forward to seeing everything you guys all build.
Sarah Guo [00:22:20]: Thank you.
Demetrios [00:22:23]: Excellent. So the chat is blowing up right now. We got to address some of these questions and I will let everyone formulate your questions. Take your time. I have a few questions from that because this is like, I really appreciate you going into some of these pieces that you need to think about as you are putting AI into production. Specifically when it comes to costs and ROi of using AI in production, I think things get really tricky, especially when you're leaning on a third party model provider. Right. And one thing that I've constantly been asking others is how they look at the cost of goods and being able to add that extra cushion on top if you're going to have to be scaling when you're making more.
Demetrios [00:23:22]: The more you scale, the more API calls you're using for OpenAI and things like that. So do you think about those business models as being more fragile or is that just like MVP one? And then eventually you're going to go to your own model and roll it in house and then it's not going to be as expensive. But you also still have to look at the cost of the engineers that know how to do this stuff and the actual gpus and all that fun stuff. So it was more of a ramble on my part, but I'd love to hear how you all look and think about that.
Sarah Guo [00:23:57]: Yeah, I can start. And Prana, please jump in. So first I would show and say that we see a design pattern pretty commonly within some of the more mature AI native companies, where even if they need a GPT four for certain capabilities within the product, that's often not the full set of things they're doing, right. And so one pattern we see is they start with that and they're pretty cost insensitive as they're trying to understand what capabilities they can deliver to the end user and sort of where the bounds of the state of the art are today. And then they, for example, might write an intent classifier or figure out how to direct certain user queries to cheaper and smaller models. It's not clear you should really be using GPT four for certain types of, for example, like simple summarization, right? And that you might care to use an off the shelf model, fine tune something, distill something, and get better latency and performance characteristics. So what we tend to see is actually like some sort of classification orchestration across a whole range of models over time. And I think that was kind of what you were alluding to.
Sarah Guo [00:25:10]: Do we see them as more fragile? I think it's really hard to predict with certainty that there's just one way to build these companies. We do have friends with companies, they're not AI native companies, right? But 100 million dollar plus era companies with tens of millions of dollars, AI business lines, and it's all API providers, right? So it's really just like, do you have the pricing power where your user values what you're offering more than a multiple of the API costs over time? And I think we also have a prediction to some degree, because of things like Mistral and llama, that costs will continue to go down over time. And super thankful to all the providers for that.
Pranav Reddy [00:26:00]: Yeah, I think we've empirically seen a lot of that happen, and part of that is also the larger inference providers are also reducing their own costs, and some of that is decreasing cost of gpus. There's rumors that GPD 35 turbo is a substantially smaller model than three five, and I think all of that makes it much more possible to offer inference, or almost intelligence at a discount.
Demetrios [00:26:21]: So there are a ton of questions coming through here in the chat. I'm going to have to do the hard job of just choosing a few of them. I think one is pretty interesting from Lauren. How are you all thinking about the cost of inference?
Sarah Guo [00:26:40]: I assume this means like self hosted or platform hosted inference of open source or fine tuned, or your own pretrained models. So one of the things that's encouraging here is how much you can move the needle on inference, right? And so if that is people who are writing their own, this takes a lot of work. So I don't think it's going to be most people, including most applications at scale. But we do have companies where like half the company is devoted to inference optimization up front, right, because they want to offer something that is going to be consumer facing or just needs to be cheap and fast. I also think that one of the things that Pranav was pointing out earlier was just, we see increasing maturity through the stack. And so you have companies like base ten and others where the entire team is dedicated to making inference faster and cheaper, and you can write an entire pipeline for that. And so I guess my view is I would want teams to have an understanding of the order of magnitude cost of what they are doing. But I would even claim we'll invest in things that are gross margin negative.
Sarah Guo [00:27:51]: Now, from an inference cost perspective, if people expect that to cross over at some point, we think it's a reasonable plan, because we do think these capabilities are going to get cheaper and you can ride on the benefit of other people's technical work over time.
Demetrios [00:28:08]: Fascinating. Okay, cool. So how about this, as far as a pricing plan? Because I imagine you've seen pretty much a classic scenario. Like you were saying, there are now patterns emerging when it comes to pricing, also, when you're building apps on top of these models. And so have you seen it make sense to do a pricing system, like a prepaid phone provider type thing, or is it all just, like, kind of consumption and as you go, you pay?
Sarah Guo [00:28:45]: Oh, we have companies that do, like, packs, right, as you describe, like prepaid phone provider. I don't know what the right answer is here yet. I described some of the challenges, and I think there is a lot of innovation. I guess what I would like to see happen, if possible, is pricing for a service at some massive discount to the human version of that service that really is in enterprise sales. There is this concept today of value based pricing, and it is essentially like, can you figure out how to scale negotiation with each customer based on the value of their use case, that's really tough, but companies make a lot more money that way, right? Because, for example, let's just try an example of, if I can take in all of the inputs that your researchers have done, and researchers, like, I don't know, like, economics researchers in a think tank or something, and then generate a report for you, and you usually charge $20,000 a pop for the consumers of that report. That generation, like, that handful of API calls, or that system output is worth a lot more than, like, I generated something for a student, right. Even if the product is essentially the same. And so I think there should be more experimentation around the pricing models here.
Sarah Guo [00:30:19]: And that could be packs, it could be consumption, or it could be differentiation by use case.
Demetrios [00:30:26]: So that's fascinating because it's, like, really coming down to what's the biggest opportunity, as you were saying, it's almost like it's the same product, maybe tweaking it a little bit, but the hard part is the same product, right. It's just going after the bigger value add and going after what you can charge more for, which ultimately will give you more success.
Sarah Guo [00:30:51]: Yeah. And, Demetrius, one of the things that I think is often uncomfortable for entrepreneurs or product leaders is to say we are going to position our product as only being applicable to this one thing, right, versus all the user segments it could actually serve. And I want to be ambitious too. Right. We're not here to restrict your ambitions, but I think the trade off is some of your customer segments are way more willing to pay than others. And it's really hard to advertise two prices on the website. Right?
Demetrios [00:31:25]: Yes, of course. Going back to that example of if you're creating a report, you're creating a report for a student who's not going to pay 20 grand, or you're creating a report for an analyst who goes and sells it, and they're going to pay that 20 grand because it's much more important for them and they can make more money off the back end when they go and sell it. And so it's like niching down so much to recognize that you have this segment that values what you're doing so much more that they're willing to pay for it. Okay, so I love this. I want to keep going because there are a few more questions, and then we're going to rock and roll with our next keynote of the day. But there is a really cool question in here from Thomas asking about for the both of you, what have you learned in the last three months which changed your view on how to build businesses in the future?
Pranav Reddy [00:32:31]: A pretty broad question. I think a lot of what we discussed in presentation is kind of what we've learned over the last year of looking at AI companies. Maybe if we scope down to the last three months, I think one thing that we've been thinking about is the quality of API and inference businesses. And so part of the question that we've been talking about so far is where do we think value accumulates in this chain? This was, I think the hot question at the beginning of last year is which of the large section of AI stack accumulates value. I think in many ways it's a little silly. We think that all parts of the stack have ways of capturing some of the value that they create. But one thing that we've been thinking about is where is the specialization or intelligence to, I think your point, Demetrius, that you were making before, if I have two different product segments, how do I pick between them? We still think that a lot of the value is in workflow. Determining what the product service looks like that people in some specific vertical want to engage with is difficult and requires deep customer empathy and understanding and spending time in domain.
Sarah Guo [00:33:31]: One thing I would add to this in terms of is not three months ago, but is a handful of months is we were seed investors in Mistral, so we made the bet and we thought that they could do really interesting things with only $100 million or only half a billion dollars. Right. But it still surprised me how effective smaller models can be. Right? I think it's a different view of. I mean, who better to tell us that scaling laws are not quite what we thought than guys who worked on scaling laws. I think that changes my view of what is possible from a user experience perspective and how many people should really be fine tuning this year.
Demetrios [00:34:13]: That's awesome. That is very true. And the idea of these smaller models and also bringing them more locally I think is a huge trend that we've been seeing and that I think it's only going to continue because at the end of the day, for your business case, you probably don't need it to write a poem in the style of Bob Dylan. You just need it to do what you need it to do as well as it possibly can for you to make sure that it gets out into production and you can trust it. And so, thank you Sarah and Pranav. This has been awesome. For everyone that wants more of Sarah and Pranav, check out the no Priors podcast. You've already got some fans that were saying in the chat they love the podcast.
Demetrios [00:34:56]: I'm so grateful that you both came on here. We got a two for one special for the first talk of this session. We're kicking it off right. Thank you both and thank you to the amazing community.
Sarah Guo [00:35:09]: Thanks Demetrios.
Demetrios [00:35:10]: See you all have a great day. And now we're just going to keep rolling.
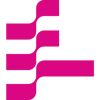