Becoming an AI Evangelist
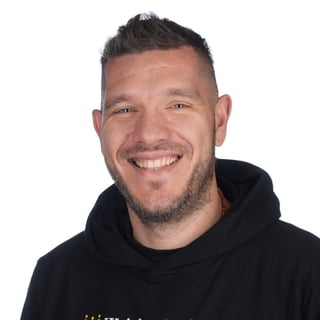
Alex Volkov is an AI Evangelist at Weights & Biases, celebrated for his expertise in clarifying the complexities of AI and advocating for its beneficial uses. He is the founder and host of ThursdAI, a weekly newsletter, and podcast that explores the latest in AI, its practical applications, open-source, and innovation. With a solid foundation as an AI startup founder and 20 years in full-stack software engineering, Alex offers a deep well of experience and insight into AI innovation.
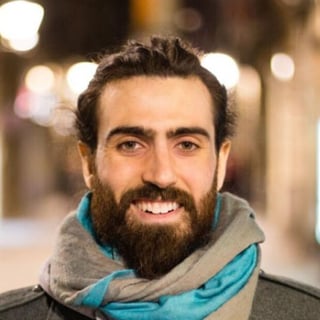
At the moment Demetrios is immersing himself in Machine Learning by interviewing experts from around the world in the weekly MLOps.community meetups. Demetrios is constantly learning and engaging in new activities to get uncomfortable and learn from his mistakes. He tries to bring creativity into every aspect of his life, whether that be analyzing the best paths forward, overcoming obstacles, or building lego houses with his daughter.
Follow Alex's journey into the world of AI, from being interested in running his first AI models to founding an AI startup, running a successful weekly AI news podcast & newsletter, and landing a job with Weights and Biases.
Alex Volkov [00:00:00]: Everyone. My name is Alex Volkov. I'm an AI evangelist with weights and biases. I'm also the host of the Thursday I weekly newsletter and podcast. And I don't drink coffee anymore for the past six months. But I do have caffeine. So this is my coffee. Basically either bomate or just black tea.
Demetrios [00:00:19]: It's another mlops community podcast. I am your host, Demetri Os. And what a show we've got today with Alex. He is absolutely kicking ass on Twitter. I love all the stuff he posts. Not gonna lie. I don't spend a lot of time on Twitter, but when I do, I see his posts and I smile. He's also got a weekly show that's happening on the Twitter spaces.
Demetrios [00:00:41]: I guess that's what the cool kids are doing these days. They've got their Twitter spaces. And he is one of those cool kids bringing on incredible experts and talking about all of the newest updates that are happening in the AI space. And today we got into so much in our conversation, everything from podcast nerdery on our gear and what microphones we're using and how we travel with it and whatnot. All the way to evaluation, multimodal, and even a bit of agent talk. Autonomous agents are, as you may know from listening to other podcasts with me, something that I think are complete bullshit. But he was not giving me the runaround. He came with his feet on the ground.
Demetrios [00:01:26]: And I appreciate the answers and I appreciate his viewpoint on agents, which TLDR is just they're still not there yet, but they're getting better. So don't expect like they're not going to be or they're going to stay where they're at forever. The other thing on this I want to mention, we got deep down and dirty when it comes to evaluating systems, evaluating your llms, evaluating everything. You need to be thinking about a little bit of a hack week that he did and how they are evaluating these different products that they did at their hack week. And it makes me want to let you know that we are currently running a little bit of an evaluation survey. That is right. I broke down the past evaluation survey. I looked at it all and I wrote a blog post on it.
Demetrios [00:02:25]: But now time has passed and it is high time for us to do another survey, figure out what are people doing in this space, how are they going through it? And we're not just doing it on our own this time. I found an incredible partner bend AI to do this with. And it's not by any means a vendor or anything it is someone that actually knows how to do surveys. And so that helps a lot, because if you know me, I've been in the dark when it comes to how to formulate the surveys, how to extract the data. I've been leaning on a lot of the community to help me extract all the insights. And now I've got some trusted friends at Bendai to help me with that, and it's much needed. So I encourage you, if you are doing anything with LLMs or not even, I would love if you would take the five minutes, fill out this valuation survey that we're running and we will give you access to the final product. We're going to make an insane report that is going to probably cost me a few gray hairs.
Demetrios [00:03:45]: That's all. I'm leaving. All right, let's get into this conversation with Alex.
Alex Volkov [00:03:48]: Here we go. You.
Demetrios [00:03:54]: So, dude, tell me about what you've been up to for the last like what, ten years, twelve years, 15 years? You've been doing this for a while.
Alex Volkov [00:04:03]: 1015.
Demetrios [00:04:05]: Yeah. Give me the breakdown real fast, because I think you came on my radar relatively recently and it was through your antics on Twitter, bitching about Apple Pros or Apple vision pros and stuff like that.
Alex Volkov [00:04:21]: I'm a Twitter native. I'm an ex native. I think that's a big part of how I came to be kind of in this profession, but generally in everything that I did before. And maybe we'll talk about this later, but it's a big part of early adoption as well, of technology. I saw Twitter and once I kind of got to the professional thing of it, I was living in another place. And I quickly realized that I can get the upper hand on everybody else around me professionally just by knowing what they don't know yet by the newest stuff. And that's kind of how I grew up, just professionally. I'm a Ukrainian in origin.
Alex Volkov [00:04:59]: I lived in Israel for most of my life, most of my adult life, and so know grew up as a software engineer in so most of my career has been in software development, mostly on the front end side. And then I kind of became like a full stack. Everybody tries to do everything, so full stack. I used to call myself the fullest of full stack, which was foolish, but I used to do like product and design as well, including front end and back end. So a very generalist approach. Nothing ML related. This whole world was so far from me. I'm self audit.
Alex Volkov [00:05:35]: I'm really bad at math. I think I'm number deficient or something. So math is not perfect for ML. And so the visual stuff pulled me more than everything else, but I love just to create and code. And the stuff that you probably saw me through is I worked for a decade at one specific startup. I started there very early on, so I did pretty much everything. I did the front end, then full stack, then I led teams that became director, product director of this, director of marketing. And then I basically got tired of it, and I moved here.
Alex Volkov [00:06:10]: I live in Denver, so I moved into this office work remote just before COVID So that worked out. And then after maybe eight or nine years, I got so bored, I literally hit all the possible ceilings and did all the lateral moves that are possible. And at some point, I was like, okay, I need to do some stuff. So I looked around about, like, okay, what's interesting, what's new, what's cool? Crypto was my thing for a while. For about three months. I went and see. Went to see if that was a whole thing while web three was exploding. I'm here in Denver.
Alex Volkov [00:06:42]: Eth is here. And then I kind of stumbled onto Dally from OpenAI. And back then, I already kind of used copilot, and dally just blew me the hell away because I looked at this and I was like, I can't draw, and I wish I would be able to draw. And now it seems ridiculous to talk about this in 2024. Oh, you know, Dally one or Dali two? I think it was the image quality. The physician was not that great. But to me, this showed that AI is not only this autocomplete. Back then, we didn't have llms.
Alex Volkov [00:07:16]: It was literal autocomplete in GPT. But it's also something that can add to me a power that I don't have. And Dally started my deep dive into AI, which I can talk about separately. I was working with Dali, and then stable diffusion was starting to come up in the discord in the beta. And as they released stable diffusion, released Dally exactly like that. They started charging money. So you used to get from, I don't know, an impossibly free tier in Dali that you can do everything and you have to iterate with this to like, hey, I'm going to start charging you. And then also stabilizer, like, hey, here's a free thing.
Alex Volkov [00:07:56]: And that free thing was a model, a weight file, and that's it. And barely some python code. And so that's where my kind of AI progress started. And then we can talk about how I got into AI evangelism from there.
Demetrios [00:08:09]: Incredible. And before we get there, what do you feel like burnt you out, you mentioned. And what were you looking for? Like doing all these lateral moves. What was it that you were looking for?
Alex Volkov [00:08:23]: I think now in retrospect. So since then, we can probably chat about this. About a year after I discovered AI, I also switched careers. I don't code anymore unless I really have to. I do some demos here and there. I code for fun, but I decided personally that the coding days for Alex is somebody who commits code and maintains code in production are done. And I noticed this after a while doing my own startup, specifically in that company. It was a fintech company, and we did like a very cool product, but nobody that I knew in the world that I'm in on x or whatever, nobody cared what we did.
Alex Volkov [00:09:05]: And you could talk about the cool stuff that we built, but it wasn't really very interesting. And I just love talking to people. I love the community building aspect of this, and there was no community to build around that thing specifically. And I think that I tried multiple things within the. So I did a bunch of hackathons internally, I did like a bunch of stuff. And I think when I moved remote, so a whole community piece of a workplace that exists in a workplace I think was missing for me. And so when I worked remote, I stayed there for a while still to keep it going. And I have a family, but after a while I was like, no, I need something that I enjoy in my life.
Alex Volkov [00:09:47]: And I just didn't have a lot of enjoyment. And then also they did a layoff. So the longest employees were the first to get hit super quick, but actually it was a blessing in disguise.
Demetrios [00:09:57]: So you went through the layoff. So you were trying to do community stuff at a company that basically had no need for community. You also were trying to find your footing and recognize what it was that you were really passionate about. It seems like you knew what you were passionate about, but at the place that you were at, it wasn't able to service those passions.
Alex Volkov [00:10:21]: There's no developer experience to be done because we didn't offer an API. And me coming up on X, most of the folks that I followed were, at least back then, folks who are developer experience at Google, on the Chrome team, I remember all of the names, Jack Archibald and all of those folks, and to me, those were the coolest positions possible. Those are the guys who go to give talks. And when I lived back in Israel, I loved giving talks on stage. I loved just like presenting and teaching people what I learned. And oftentimes this is my process of learning myself. And even back then, I just commit to a talk about a topic that I had no idea about. And I promised myself that by the time that this talk is ready, I'm going to be the person, the expert on stage.
Alex Volkov [00:11:06]: And a lot of this came from, literally from just being able to be on Twitter, learn super quick about a very new niche concept. I just didn't find a way to turn this into my actual career. And so I don't love managing, and I got stuck into this management route where I had to make sure people executing, et cetera, that wasn't my vibe. So I scaled back a little bit to individual contributor, and still it wasn't like what I actually wanted to do. And after they laid me off, or I guess after the laid off happened, it wasn't like a specific personal thing for me. It was a blessing in disguise. I was already super tapped out, nine plus years, all the upsides for stock equity, whatever way in the past, I decided to embark on a solo journey of my own to open the startup. And this was around the time where AI was happening very strongly.
Alex Volkov [00:12:01]: OpenAI released Whisper, and this was a few months after stability fusion released. So stable diffusion gave me the kind of the understanding, okay, here's a weight file. Here's how you kind of prod this brain to do what you want. And then Whisper came out. I was like, oh, this is so cool, let me try whisper real quick. And so I tried whisper real quick. As I was building already a startup around AI art and stable diffusion, I tried whisper, and the first thing that came to mind was, hey, I can use this for translation. It's not only ASR, it's not only automatic speech recognition.
Alex Volkov [00:12:32]: Whisper also does decent translation. If you just say, hey, that person is actually talking English and not Ukrainian, whatever, there's a translate flag. And so I used Whisper to put subtitles on a video from Ukraine. I'm ukrainian in origin, right? So everything's happening in Ukraine at the time. I'm ukrainian origin. Something happened there that's very interesting. And I don't speak Ukrainian. I'm from the russian speaking area of Ukraine, so I don't very natively speak Ukraine.
Alex Volkov [00:13:00]: I really was interested like, oh, I can do this with Whisper. And so I built like a super quick thing that translates videos and put subtitles and put it on x, and that blew up. That blew up. And I was like, oh, okay. This took me like 5 hours to set up, to put the Python notebook, to do ffmpeg, to stitch subtitles. Whatever, but this can happen automatically. And so I went into a cave even though I already had like a startup thing brewing. And I, for a weekend, spent time in that cave and came out of it with a bot on X that auto translates videos.
Alex Volkov [00:13:38]: So X is know a lot of text, but also started to show a lot of videos from different worlds, especially around the war in Ukraine. I built a bot that just replies with a translation. And I think I was the first one to do this automatically and that became my startup for the next, I want to say seven months. Putting that aside, looks super good. We can talk about the ML ops of this. I had to scale this. I had to put the models in production, had to monitor do, I had to learn another full stack on top of my already full stack. And that was fun.
Alex Volkov [00:14:09]: But I think it's behind me. We can talk about it.
Demetrios [00:14:11]: But is that when you went to the local meetup in Denver?
Alex Volkov [00:14:14]: Yeah, I think I went there a little bit after. So in Denver specifically, I met with the Mlaps folks in Denver. Shout out, I think Bianca and Phil. I want to. And, yeah, I forgot the names. I'm not really good at names on the spot. I'll try to remember them. And I went there because we also have a local meetup here in Denver because one of the biggest parts of being a remote worker is I missed a community in real life, in physical life, I should say.
Alex Volkov [00:14:48]: And we started our own AI meetup, and some of them came. And then Claire as well, I think Claire showed up on our meetup and said, hey, tomorrow we're doing our own thing. Mlops. You should come. And so I came like a day after a day to the meetup. So it was super fun.
Demetrios [00:15:03]: Yeah, probably Pete too, I imagine.
Alex Volkov [00:15:05]: Pete. Yes, Pete. Pete. I feel it. But definitely Pete. He comes to ours as well. We should combine forces, man. We should.
Demetrios [00:15:13]: Exactly. The whole team there. What is super cool for me to watch the Denver community grow is that the first meetups, I think, had like five people and then there was seven people and then there was like ten people and then there was like 50 people and boom, there is 100 people.
Alex Volkov [00:15:33]: And now they're sold out. Yeah. And now they're cool events.
Demetrios [00:15:37]: It's one of those things where you get to see that it takes on a mind of its own and the dedication from the local organizers to keep going with it and keep figuring out ways to make it better. We've been having these local organizer meetings where we share, oh, in Berlin, they did have, I'm part of the chapter right. So, well, kind of. I guess it's a bit of a stretch these days to say that I'm part of it. I used to help organize it more, but now I just drop in to the events occasionally. And what they do, which I think is super fun, is that we will get together and at the beginning of the events to encourage people to break out of their shell and meet other people. We'll have trivia games. And so we say, all right, everybody, you get put into a team depending on when your birthday month is.
Demetrios [00:16:34]: So if you're born in May, you're going to be with everybody else that's born in May and that's your team. If you're born in June, it doesn't matter the year, it's just the month, right? And then you get together and there's a trivia quiz and the person who, or the team that wins the trivia quiz gets like bose speakers or whatnot. And so we make sure to have some cool prizes. But at the end of the day, it's so cool to see the people interacting on a totally, I can go there with no friends and come out with a group of comrades, as you could say.
Alex Volkov [00:17:10]: I think this piece in my kind of position at that startup, the fintech startup, was sorely missing for me. I was missing that, exactly. I love building communities. I did this throughout everything, including within the startup, as I moved countries, as I kind of moved cultures, essentially. I still kept doing this. And I think that's a big piece of what you guys doing with mlaps, like everywhere, local meetups and everything. That's what we do. That's how we met with the MLAps community, because they came to ours and then I came to theirs.
Alex Volkov [00:17:41]: And a lot of the people are pretty much given the same talks, talking about the same things Claire talked about arise, for example. And that's something that is in ML ops but also fits the AI meetup that the tinkerers do. And I think one of the coolest things that I've noticed in our meetups is we had a month after month conversation where a month after somebody came up and started talking about their process and then something that, a light bulb moment that he had during the previous meetup that somebody else talked about the approach to AI tinkering and that other person, Matt, was in the room and the guy was on stage with Sam and he's like, oh yeah, that's the, like, yes, this is exactly what we're here for. These osmosis learning thing doesn't happen necessarily online, it does on x, but not everybody's as native to x as I am. But definitely that's the community part that we want. That was super cool. And then we had beer, all of us who drank.
Demetrios [00:18:41]: Yeah, you make it a good time, it's like guaranteed that you're going to go there, you're going to enjoy yourself and then learn something too. And honestly, after whatever, two years of being stuck inside, everybody just wants to go and be around people again. Right. The talks, in my eyes, are an excuse to just hang out with people and have.
Alex Volkov [00:19:02]: That's true. Sometimes there's too much talking and not enough comparison to person talking.
Demetrios [00:19:09]: Yeah, 100%. And you see that, too, with all of the different in person events, like conferences that are popping up again. I understand there's a value in that connection. I also am huge, obviously, I'm huge on virtual stuff. We're doing all these virtual conferences, and I think that has its value because you can get people that are in different parts of the world and they can jump in. You don't have to be in Denver to get the knowledge from the experts in Denver. Right. It's just kind of like the pressure's on me, the organizer, to make sure to go out there and get everything sorted.
Demetrios [00:19:53]: So these experts want to come and talk and they want to share the value, and then we make sure that the talks are good. So talk to me about the transition that you went through. You mentioned how, yeah, you stopped in Covid and then you had your own stuff. You started working on AI. You had your own for seven months. You were doing all kinds of ML op stuff. I imagine you learned quite a bit in a short amount of time.
Alex Volkov [00:20:22]: So I'm sitting in Denver, no friends who are into AI, one friend who's. It's more of a dialogue between me and him and not a conversation where I talk about the stuff that he's very interested. But it's not like we're brainstorming. Right. And a very good friend of mine and I'm very happy for the opportunity to be able to be vocal as well and not just like in front of a screen, because sometimes you just need to bounce ideas off of folks. But not. Yeah, rubber duck. Exactly.
Alex Volkov [00:20:53]: But not for debugging specifically. It was just for like, hey, this cool thing happened. That cool thing happened. I can connect the whisper model, I can connect with the translation model, and I can put up a pipeline. I can do evaluations on production. I can do all these cool things. And he's like, yeah, but he's not a technical person at all. And that was very helpful.
Alex Volkov [00:21:10]: And I just turned to my community online. And so there's maybe an additional thing where I got lost on x for a while during 2016 to 2020 ish in the politics area and my whole feed became just like awful, just awful and not fun. And I kept scrolling and engagement farming got to me and everything. And as I was starting the startup, I decided, hey, I'm going to clean this out. I want only AI stuff. And as I'm learning, I think you touched on a very interesting thing. As I was learning, my process of learning is speaking about the stuff that I learned. I learned the best publicly.
Alex Volkov [00:21:46]: When I use x as my kind of notetaking app or brain dumping app, I notice that the content that performs the best is the content I just put out for myself to be able to find it later. And I used to do it with a blog a long time ago. And that's the content that resonates with people, not the performative stuff, not the like, hey, here's seven tricks and tips and whatever. Just the content is like, hey, I just found out this cool thing. I just put that on production and this worked and this didn't work. That content started showing up. And so I just noticed that I'm building this community on x and slowly getting followed by folks for also discovering this. As I was building this, Chat GPT came out.
Alex Volkov [00:22:24]: I literally started, I want to say October with whisper stuff and I was building, building and then Chat GPT came out. I could not ignore what's going on because I used GPT-3 before the auto conclusion stuff. It was night and day. Something about the memory retention in the product. It was night and day and to me it wasn't the night and day in the UI stuff. Obviously everybody's now on the chat bot is incredible. You talk to it, it gives you so much. To me, the night and day was because I used these tools before to do a bunch of stuff.
Alex Volkov [00:22:55]: But now I wanted that as an API. I wanted their memory management in context. I wanted the ability to handle a conversation, the ability to role play like all these things. So I actually came out with a bunch of side projects and I got sidetracked from my startup just for a little bit because I had to make sure that I know what's going on with this new technology. So I did a bunch of stuff that went kind of viral, learned a bunch, learned about prompting, about sandwich prompting. I came up with a bunch of techniques and I love the new stuff. We just talked about the vision pro I just bought on release. My best existence as a person is on that frontier that we all got something new as humanity, and I get to discover this first and talk about this publicly.
Alex Volkov [00:23:43]: And when this whole thing with JDBT came out, I started noticing that, slowly started noticing that while I'm building my startup, it's an AI startup. It's very exciting. I'm kind of way more excited to share than to build, to share what I built than to build. And I think that slowly rotated. Like, after a couple of months, I went down to the hole again. I built the whole UI and the payment system and everything. I released my product to the world. It's called Targum Video.
Alex Volkov [00:24:08]: It's still live. People still use this. And I just noticed that as I released it, I was like, okay, how do I market this? Okay, I released it. I'm a solo entrepreneur. I don't have employees. I didn't get fundraised. How do I market this? Oh, I keep doing what I'm doing publicly. I keep talking about the stuff that I'm doing.
Alex Volkov [00:24:26]: I slowly started noticing that talking about AI and innovation and cool stuff is taking over. The excitement about the product itself, the excitement about building more features, the excitement about delivering value to customers, all these things. And that's where I started the audio stuff. So X or Twitter, formerly known as Twitter, some of us still refer to it as Twitter. There's a x spaces thing, which is kind of like clubhouse for folks who are not Twitter natives. Basically, a radio show with an audience live presented the radio show with an audience, and I hopped on one with my friend Travis, also a tinker in AI, when GPT four came out. So I remember that date. March 14, 2022.
Alex Volkov [00:25:16]: March 14, 2023 is GPT four comes out, and we've all been waiting, and it's incredible. And we hopped on the Twitter space of somebody else's that ended, and we were like, we were so excited about talking about this, like, hey, dude, let's just open a space of our own, keep the conversation going. And we had, like, a nice vibe, and so we started with this, and that happened on a Thursday, and next Thursday we came on again, and next Thursday we came on again, and next Thursday we came on again. And then at some point, Travis started his own startup. So he's like, hey, Alex, I don't have time for this. You go. And so I kept going, and I have been doing this every Thursday since then, I think more than 70 times. And around the summer, I noticed that, okay, the startup is no longer of an interest to me.
Alex Volkov [00:26:00]: Like, honestly, this was a one person thing. It still runs. It works. I haven't added any features for the past six months. I apologize to the customers out there. I just noticed that preparing that weekly conversation, learning throughout the whole week about the new stuff, talking about this, preparing, it became a show. It became a show with segments, with repeating customers, with guests, with entertainers, is way more fun to me than everything else. And around the summer, people told me like, hey, you're doing a live thing.
Alex Volkov [00:26:30]: Why don't you just put it on the podcast? Because I don't want to be live on Twitter when I listen to you. I want to drive to pick up my kids on a Sunday. And I was like, I don't want a podcast. Podcast. I don't like my voice. I don't like this. I don't like that. I was like, okay, at some point, I just did it.
Alex Volkov [00:26:45]: And now, now here we are. Basically. Let me just say where we are exactly. There's a weekly show called Thursday AI because we talk about AI every Thursday that I host with a bunch of friends of mine who are experts in different fields where we cover everything that's interesting in the world of AI. For the past week, mostly focused on open source and llms, but also we talk about vision, we talk about new papers that are exciting, that come out. And it's been a while, time to build that community as well, because that's now a huge community that I did not expect to happen.
Demetrios [00:27:23]: Yeah. And I think that is how I stumbled across what you were doing. And it's incredible to see the dedication that you've had. I know we talked about it before. Like, I hit record. We talked about the travel gear and how you almost are like, oh, I don't want to travel right now because I got to do this Thursday, every Thursday. And so break down, like, over the, whatever, the past, let's say, month or two, what are some huge things, maybe papers that have stuck out to you or big news that has come across and you're like, well, this could lead to, if we extrapolate this out a little bit, where can we lead to with this?
Alex Volkov [00:28:02]: Definitely what happens is, and I think you've touched on a very interesting point, is because I get to do this every week and because I only focus on what's new that week, I'm noticing that we talk about the new stuff, not necessarily only papers. I do try to be very practical about, okay, which open source model just released, which performance optimizing thing happens that makes this whole thing go better. And because I have this record, a weekly record, I'm able to look back during the summer and say, okay, we thought that this is going to happen. And now we're here during winter of 2024, beginning of 2024, which of the things that we kind of suggested might happen, happens. And I think several trends are showing up, and it's very interesting to be able to see which ones were predicted or not. And multimodality is, to me, one of the biggest ones. Agentry is another big one, and I think multimodality 2024 is going to be remembered the year of multimodality. What I mean by multimodality is Llms as a concept.
Alex Volkov [00:29:08]: They're naturally just spitting out kind of next token predictions. That's how they're built. But the architecture behind them, transformers architecture, can be applied to multiple other things, like envision, like in whisper, for example, ASR field. So NLP and more and more folks realize, and I think GPT four started actually being the first announced multimodal model, or a VLM visual language model, where they combine language, or NLP understanding with visual understanding as well. And I've been on this almost every week since GBD four came out. I was like, hey, we need this also on the open source, because that's how we humans talk. Even on x, let's say, example, I take a screenshot most. I know that the most engaging tweets, whatever, they're like the visual ones, with video, with a screenshot, not only text, but also because these models have a better world understanding what happens in our environment when they have proverbial eyes, let's say.
Alex Volkov [00:30:14]: And it's harder to train those models. It's harder to evaluate those models. The evaluation sets for kind of the combined things are not necessarily exist. But there's a very interesting talk that Ilya Sotker, who was a chief scientist in OpenAI, actually don't know his status there right now, but he was like one of the co founders. He talked with Jensen from Nvidia about how if you build this model with the concept of eyes, basically with visual understanding, you get to the same concepts that the large language models get to, like, what's the concept of red? But quicker because the model can see. And I think since that point, I was very interested in how visual multimodal models perform. And multimodality. I'll just say this one thing, and then we can talk about this.
Alex Volkov [00:31:06]: Multimodality often gets referred to as, okay, there's only two modalities. There's text and there's video, or text and images, for example. Video is a whole different beast that we can talk about, but it's so much more than this. Meta has released a paper and I think it's not a full model, but like an example model that has six modalities in it, text, images or video. They also have Imus, which is motion units, so they can understand from video motion. So if a trains comes in the model natively understand know distance and motion units, et cetera. And if you think about this, you want to embody AI at some point in robots that walk around, they have to understand motion as well. It's not like they're going to take a picture every second.
Alex Volkov [00:31:51]: Okay, between this picture and that picture were changed and there is example of multimodality to go even crazier.
Demetrios [00:31:57]: All right, let's take a quick break from the episode and talk to you for a second about our sponsors of this show, Wandb, and what they've done with their newest LLM course. You can elevate your machine learning skills with weights and biases. Free course on training and fine tuning large language models, LLms as they're called out in the wild. You can learn from industry experts like Jonathan Frank from good old Mosaic ML, friend of the pod Weeang and Mark Seraphin. And while you're doing it, learning from them, you can deep dive into the LLM fundamentals, advanced training strategies like Lora and prompt tuning, all while getting your hands dirty and having some hands on applications. This engaging course offers 37 lessons and over 4 hours of videos and a certificate upon completion. Enrolled now to master llms and advanced techniques. Check out the link in the description to start your journey.
Demetrios [00:33:01]: And let's get back into the pod.
Alex Volkov [00:33:04]: I had the folks from prophetic, prophetic, I think, I believe they're called. They have a multimodal transformer architecture. The modalities are not text or vision at all. The modalities are inputs from an EEG signal and fMRI signals, right? So functional magnetic resonance brain scan signals, basically an EEG electro something. And they use this to turn the output of their transformer architecture into locations in your brain where a super, highly precise ultrasound can hit to invoke a specific state in your brain for lucid dreaming completely. That's an AI system that takes in brainwaves and outputs directions, right. And that type of multimodality is also very interesting to me. So definitely that's an area of extreme excitement in 2024 so the fascinating thing.
Demetrios [00:33:55]: About multimodality, besides claims of invoked lucid dreaming, because who doesn't want the easy way of lucid dreaming? I don't know if you've tried. Have you ever tried lucid dreaming?
Alex Volkov [00:34:08]: Yes, I've been pursuing lucid dreaming most of my life. I still do 12345 and try to poke a finger through my hand to see if I'm in reality or not. I do reality checks every day. Yes.
Demetrios [00:34:17]: Is that the one? So I tried to turn off and on lights because that's another one that you could do. So I'm guessing then, if you do that you've been through a lucid dream?
Alex Volkov [00:34:30]: I think I got like a once or twice. Even though I've been pursuing, I'm not really a great lucid dreamer. Well, there you go.
Demetrios [00:34:39]: Prophetic is perfect for you, right?
Alex Volkov [00:34:41]: Yeah, that's why I trust technology to get me there eventually.
Demetrios [00:34:46]: Yeah. But on the other hand, when it comes to multimodality, one thing that I think about is multimodal rags and the difficulties there and all the challenges that need to be. Like, there's so many hurdles, I feel like when it comes to how we do those. I'm by no means an expert in the field at all when it comes to multimodal rags, but I have heard people talk about why what we have, the current tooling and what is out there right now is not up to standards, especially if you're getting into video or if you're getting into audio. And you can think of, I guess one way that you can think about it is looking through long movies and being able to grab context, not from just the transcript, but also what is in the scene in the movie. Right. But that's really hard if you are taking a screenshot every frame and then you're actually using that for your rag. So there are those pieces.
Demetrios [00:35:52]: I do wonder how that will change over. As you're saying, hey, multimodal is 2024. It's the year for multimodal in every aspect. Not just lucid dreaming, but in multimodal rags too.
Alex Volkov [00:36:05]: That was definitely an extreme example of showcasing how modalities are not only image and text, as most of the people will get to think about multimodalities. FMRI inputs as a modality in addition to text, for example, or facial structure inputs as in addition to webcam feed in addition to what you say, and intonation, for example, also could be modalities. I just want to highlight that multimodality as the most practical one is probably imagery in text, what GPT has for us, right? You could consider audio modality as well. But honestly, until they detect intonations in my voice, until that point happens and it doesn't happen, I haven't seen that happen yet. Audio modality is just text modality because of ASR. And LLP just translates everything I say into text, right? So it's text and imagery, not even video for most of it. I think the next jump, like you said, is, okay, how do we understand from frame to frame? Because we humans, we don't experience 24 frames a second. We experience.
Alex Volkov [00:37:04]: I think our refresh rate is higher than that. There's two things here in terms of difficulties, right? One of them is the actual scene to scene understanding and scene boundaries and cuts and all these different things, and streaming audio, enough hardware to be able to actually get those frames. So everybody knows that, okay, transformers have this problem called quadratic attention problem. They're incredible because of their attention. Attention is all you need is the paper that came out with all this. However, the quadratic scaling with this attention, the more tokens you give, the harder it is to run inference, the more imagery you add, way quicker that the attention grows, right? And if you consider that every image from a video is also like, I don't know, 24 frames a second, you start getting into serious, let's say, ML of problem. Just before the even transformers problem of how do you even store all these things? There's a bunch of stuff that video included. So shout out to folks at twelve labs that came to one of our AI tinkers meetup in Denver.
Alex Volkov [00:38:08]: One of them is in Denver and showed us kind of the future. They're working on video embedding and the video foundational model that understands video specifically. So they have a video modality built into their model and video embeddings built in. So hopefully folks, check this out. Definitely very interesting. And the other problem that you've touched on, very interesting problem even in text right now, is evaluations. How do we know that the model that was trained is actually better than the model was trained yesterday? It's literally a competition now, like a competitor's game of releasing a better model. And it's better by like 1.7% on the MMLU evaluation stack.
Alex Volkov [00:38:46]: And here's where weights and biases comes in. The company that eventually hired me because of the podcast, so now Thursday I is part of weights and biases. Evaluations as part of the building of the model is very important. So there's like the open evaluation stack on hugging face. If folks follow that there's like a very specific MMLU and GSM, four K and AK and math and all these things. But also the problem you touch on is this gets maybe an order of magnitude more complex into multimodality. Because how do you then evaluate, how do you evaluate text and imagery? How do you evaluate, if we get to video, how do you run evaluations of what the person saw and then said, and if there's an intonation in addition to this, if the model reacts differently based on if I'm angry or sad, how do you evaluate all these? And I think that it's fine for a technology to change, evolve. And then also the supporting tools, like the ML ops tool, tracking tools, like with ambassadors, they have to catch up to technology because it moves.
Alex Volkov [00:39:46]: It doesn't wait for anyone. But definitely there's going to be challenges in multimodal evaluation sets, multimodal understanding of, okay, how do we actually know what's good or not?
Demetrios [00:39:55]: It's funny you mentioned that, because we did a survey on evaluation last year, at the end of last year in the MlOps community, and one thing that is absolutely clear from that survey is when it comes to evaluation, you kind of have ways that you evaluate depending on your use case. So if you're doing translation, you use one evaluation set. If you're doing summarization, you use another evaluation set. And that's basically the standard right now, which doesn't feel like it's the most robust at all. It's like, this is a little bit janky.
Alex Volkov [00:40:33]: It's very interesting because that keeps happening also in the open source LLM world that I track very closely week to week. So I'm very fortunate to host from week to week folks who build or fine tune the open source LLM models. And that's kind of the bread and butter of Thursday. We talk about kind of the progresses in llms, and a bunch of those evaluations, like you said, are task specific, or at least the instruction specific. And what happens is we are building generative models. Most of the people's goals here is agI, artificial general intelligence, whatever that means. Your definitions aside, the main concept there is generality, the ability of this model to do this thing and that thing and that thing. So often we see in the open source world, like this model beats GPT four, GPT-3 on this specific task.
Alex Volkov [00:41:26]: That model beat GPT four on this specific task, and that's incredible. But GPT four is like this general model that can do all of these tasks and still perform at the top. But from the evaluation side, all of these evaluations are also on a specific task, evaluation said word, error rate, captioning for video and imagery, et cetera. And the problem is, we also need general evaluations. We need evaluations that tell us how overall the models are doing. I think a lot of those are now, for example, average of all the scores, average of all the specific tasks and valuations. And one shout out, I think, is to Lmsys. If folks are not familiar with LmSys, they're the folks who fine tuned Vikuna, either vicuna or alpaca.
Alex Volkov [00:42:14]: I always forget which one. But they also, since then, released, like, a bunch of very interesting things. One of them is their leaderboard, the LmSys leaderboard, where they actually measure an Elo score or rank based on conversations that people have with these models blindly with like two models, face to face. People ask a prompt, and I suggest, everybody here goes and tries this out and contributes to this data set. And they just, humans just prefer, okay, this answer is better. That answer is better. And I think one of the coolest evaluations that came out of this world, of a digital, crazy world that we live in, is the vibe check. Like the Internet vibe check.
Alex Volkov [00:42:49]: So you go to Adamsys and there's like the human vibe check of what they thought was more helpful. There's not a number there. They just put the number there. But also, if you go to Reddit, there's like a whole subreddit of people who try things for specific purposes. Some of them are building wifus, but they just say, okay, this model performs better in roleplay than this model. And so we get this very weird vibe checky understanding that a mistral, for example, is performing better than Yi from h zero, whatever they're called. And so we get this wipe check, but nobody knows really why. And that's kind of incredible to me as well.
Demetrios [00:43:26]: Yeah. And the other piece that I think is interesting to note when it comes to evaluation, besides the vibes and how that is now a thing, is that you have to look at evaluating in so many different ways. Right? Are you evaluating the training of the model? Are you evaluating the system or are you evaluating the output? Right. And then if you're using rags, are you evaluating the retriever and how you're evaluating all these things and each piece of the system or the greater system all plays into it. So it's not just like, oh, let's look at the model for this, whatever I'm asking it to. Write me a poem in the style of Bob Dylan. It's like, what is the use case? What kind of model? The size of it, the speed of it, the accuracy. All of that can come in, like, how many tokens it spits out, how well does it listen to you? What's the toxicity? All these different pieces need to play a part, especially if you're trying to use something in production, right? It's not just like, oh, let's go and see what this model can do.
Demetrios [00:44:41]: And that was another piece that jumped out at me because, funny enough, timing is perfect on this because I'm writing the takeaways from the survey, even though we did the survey like three months ago, now, four months ago, I'm writing the takeaway blog and just looking through all these answers and I think like over 120, 30 people filled it out. And so you get to see what people are saying and how they're evaluating and what is important to them. Basically, everybody's like, truthfulness, hallucinations. I want to make sure to mitigate that as much as possible. So accuracy hallucinations are big ones, and then cost is another huge one, like evaluating the models based on cost. And that's where the open source stuff comes in and it's huge. And so looking at all that, and the reason I forgot to mention, the reason I'm trying to do this is because of the fact that we're going to run this survey again and see what's changed. It's only been three or four months, but a whole lot has changed in the evaluation work, in the evaluation space.
Demetrios [00:45:47]: Right. And now we know much more. And if people are doing multimodal rags, how are they evaluating it? Like you said, there's incredible details that you need to be thinking about. And how do you know that the model says okay, or the output says, yeah, there was a red dress in this scene of this movie and it extrapolates out from that. But how can you double check that? That's really hard. And so I know that there's a lot of things happening as far as, and there's a great paper, I don't know if you've read this one on mitigating hallucinations. I'll throw it in the show notes and send it over to you too. But it just talks about all the ways up until now that people have tried to make sure that the models don't hallucinate.
Demetrios [00:46:32]: And so it's not exactly evaluation, but it's like, hey, what can we do so that we can be a little bit more confident? Things are working and then how do.
Alex Volkov [00:46:42]: You actually evaluate that you were able to mitigate hallucinations? I want to add some fresh learnings from this from actually my crew at wet and bias, the growth ML team. I literally went live yesterday with part of the team. And then the second part is next Monday. I can send you the link to the show notes as well, where our team was tasked to build, because we build the tools, but we also need to actually experience the rag, kind of see what new, what changed, how do people evaluate. We also need to listen and build and build intuition around these tools ourselves, just to know what is the best product to deliver to customers. And our team back in December got a week off different other tasks to just work on build week projects or hackwick projects that we said all of them around LLM use cases, all of them around like hey, let's build an actual thing for the company and just to see what are the pain points, what are the pain points to solve? And so here's kind of the outline. I'm going to give some alpha to your listeners what happened in those conversations. So we had one person who built a rag bot, basically a retrieval called wantbot, where if folks go to a discord or slack, and I think now it's part of the GPT store as well, they can just talk to a weights and biases documentation and all the help and the code and everything, and this bot will give them answers.
Alex Volkov [00:48:07]: So that's been up on production for a while, and that's way more mature than the weak build bots that we were able to do, right? And so he joined this build week and he kept enhancing the performance. Everybody else started pretty much from scratch. Here's the learnings. It's fairly easy to build something that looks like, oh yeah, I download all my data from documentation. I built like a retrieval button top of this. I did chunking with Langchain or llama index, and I embedded this with this embedding that embedding whatever. And see, you ask it a thing and it works. It's fairly easy to get to that point.
Alex Volkov [00:48:44]: Here's a few very interestingly problematic points. First of all, you have to figure out what use case you're solving for, actually whether or not you'll have users that will send you evaluations and say, hey, this works and doesn't work. Second of all, most people don't build in even these things, not even to mention like storing everything to be able to run this again. Folks should have considered built this in. If it's a slack bot build the buttons, whatever, store that information. It's very crucial for you to ask your users whether something worked or not. Third is educate your users into actually using those buttons, into actually saying what you need for which use case and whether or not it worked, because that will be your data set going forward, then once you have this bot in production. So I just literally am comparing the mature bot that people actually work, and we have users for, and kind of the buildweek projects, they were cool.
Alex Volkov [00:49:36]: But here's kind of their next steps, figuring out what happens with the data set when it changes. So everybody talks about rag, but not a lot of people talk about ingestion pipeline. What happens when your documentation changes? It changes often, like sdks change. We all know the problem with llms, where there's a cutoff line of knowledge and doesn't know everything above it. So you have to augment this with Rag. So the mature bot, the want bot, has an ingestion pipeline that runs automatically every week. And now we're getting into ML ops, right? Like you have to set this up. There's, Crohn's involved, there's like a bunch of stuff.
Alex Volkov [00:50:09]: And the person, Bharat incredible, he gets a report of how much changed which ingestion, because we have documentation, we have translations, we have GitHub code, we have a bunch of stuff that this bot learns. And the more, the more it learns, the more important it is to make sure that you keep up, because it's not only about hallucinations anymore at some point, it's also about not giving the right answer just because you didn't update your data set against what's actually running, especially when there's like multiple people in an organization. So ingestion pipeline is very interesting because nobody builds an ingestion pipeline when they just hack on llama index. And the other side of this is automatic evaluations post this thing. And so very interesting is that suddenly we use llms to evaluate llms. So after a data set has been built, after you get your users to do thumbs up, thumbs down, after you get enough of examples of what your users would want, you now have enough to actually start building an evaluation pipeline that's automatic, that keeps running. And that's incredible, because that unlocks this next thing where iterations are suddenly possible. What are iterations? For example, you can switch from an open GPT four model, for example, to a local mistral model, and then see your evaluations are running.
Alex Volkov [00:51:26]: Is it better, is it worse? Is it significantly better? Significantly worse. One evaluation has to be about speed, infrared speed, because the more prompts you get, the more ragninder you get. At some point your user is just sitting and waiting and it's really hard to measure that. But for many users, that's a pain point where they would stop using this completely. They would just go and look it up. Right? Most documentation places have search, so at some point it's diminishing returns. So also evaluate your speed to user inference. And I think another thing that is possible to evaluate automatically is embeddings.
Alex Volkov [00:52:00]: Not many people consider the embeddings model themselves. They're not fine tuning the embeddings model, for example, which is possible. They're not doing re ranking, for example, which is also possible and significantly better at some cases. And so now once you have automatic evaluations running, we are able to now switch embedding systems. So OpenAI, a week ago, two weeks ago, came up with a completely revamped embeddings. They moved from Ada two to text embedding, light or small. And text embedding large and large is like significantly performant. Small is very performant.
Alex Volkov [00:52:33]: Both of them are cheaper. I love how everything gets cheaper. But then how do you know? Do you just switch and hope that OpenAI gave you the best tool? So we now have the automatic evaluations on the more mature bot that now we can actually replace run for a week and say, okay, yeah, that's actually kind of faster and better, less hallucinations.
Demetrios [00:52:52]: So cool. And there's a great point that you just kind of skipped over, but if you're not automatically ingesting, then it could be the right answer that you're getting for the documentation. But it could be the right answer from two weeks ago. And since things have been updated and now it's not the right answer, but it was, but now it's not so evaluating that. It's also huge that you're talking about these embedding models too. And there's a lot of cool stuff happening out there in the embedding model space. There's a lot of open source embedding models that you can use. There's a lot of just different.
Alex Volkov [00:53:30]: I want to shout out a few, if you don't mind, because just recently, this is what I do, right, so now we're moving into the Thursday I podcast because I specifically have a thing about embeddings. Our friends from Nomic AI, the folks behind Atlas, if you're familiar with atlas, is a way to visualize embedding.
Demetrios [00:53:45]: They're going to be talking at the next conference.
Alex Volkov [00:53:47]: Oh yeah. Awesome. So nomic folks released a fully open source nomic embedding end to end, including the training set and everything. And that's incredibly awesome. The more open source things are, the more you're able to take on the training and the fine tuning of embedding model, something that people don't often consider. People consider fine tuning the GBD, for example, LLM. But you can fine tune embeddings on specific tasks. For example, another shout out is Gina.
Alex Volkov [00:54:14]: Gina AI released the Gina embeddings v two. And recently they released a fine tune on code, for example. And when you fine tune embeddings on code, you get better code examples rather than text examples. Because if you consider what embeddings are, usually they came from the world of just text embedding and understanding, not necessarily code. Code does a different thing. But now we're getting into the world where like Microsoft trained m five, which is an embedding model on top of Mistral, which makes no logical sense besides the theoretical fun for academics or academic sense, but it's super slow to run. You have to run like in 14gb, VRAM, whatever this mistral model. But if you consider what embeddings are, they have a brain in there that understands the concept of what you're trying to embed.
Alex Volkov [00:55:01]: And mistral is like this very open source, very nice brain. So they use that to train and that obviously outperforms everything else. But again, on the problem of evaluations, outperforms everything else on benchmarks. But you have to consider how much hardware and ram and speed to embed. You have to evaluate those things again. So embeddings, this incredibly vast area that people are now starting to get to towards, hey, we can fine tune for this specific task. We can fine tune for Q and A. Literally.
Alex Volkov [00:55:33]: You can fine tune an embedding model for specific q and a retrieval tasks. You can find them for code tasks. So definitely people sleeping on this, but it's coming. This area is now opening up. If we're talking about trends for 2024, I think embeddings is another interesting trend.
Demetrios [00:55:48]: Yeah.
Alex Volkov [00:55:51]: Sorry, just one last sentence. Including multimodal embeddings as well, because embeddings now are not no longer about just text. You can embed images, you can embed videos, you can embed concepts, you can embed users preferences, you can embed like a bunch of stuff. And all of these you have to embed in order to retrieve from, right? So the multimodal embeddings are also up and coming and a very big deal as well.
Demetrios [00:56:14]: Yeah. With the burp models out there, you can basically fine tune an embedding model for like 200 or $500. And if you know what you're doing, that's really cheap and that gets you off to a really good start. Right? And hopefully if you're doing that, then later on down the line you don't have to go and fine tune other models that much. Maybe some off the shelf model will work good enough for you in what you're trying to do and what you're talking about here. Everything that you're saying just reminds me of how much of a trade off each piece of this is, right? Like if you're evaluating each one of these and you're trying to figure out what you want to do, you're basically looking at like, okay, what are the pillars of what I'm trying to do? Do I want the most accurate thing? Do I want the fastest thing? Can they be accurate and fast together? How much longer is it going to take me to build that? All of those different design decisions come into play and I'm sure you think about that quite a bit, just with the product background that you have and how that all will look at the system. Again, it goes back to that. Looking at it as a full system, should we add the thumbs up, thumbs down? Should we ask users to actually give us robust feedback? And how do we make sure that they give us robust feedback? What does that even look like? And then what do we do with that robust feedback once we have it? And so all of these different pieces, you got to kick them around in your head before you actually have something that you can confidently be like.
Demetrios [00:57:54]: This is about as good as it can be right now, right?
Alex Volkov [00:57:59]: One consideration to take into account is how it looks for the user and who is the user, right? So obviously llms came in and chat bots are like the number one thing, like chat bots. Chat bots by almost necessity mean real time interaction with the user who waits. And because everybody get used to the best chat bots around, GPT four. And now Gemini Ultra is going to release very soon. Those are huge companies. They run those on extreme, extreme scale, compute, where you get instance like a response like super quick so your users may get used to something like this. And here you are trying to run your own open source model on production, let's say. And so you have to take into consideration the use case.
Alex Volkov [00:58:44]: And I'll give you another example from the build week that we did. And by the way folks that are listening who work at companies, this is incredibly important thing to do for your own developers as well. Just give them like a week to play and try out things. The learnings that you get from not building to production necessarily, but just like going to hackathon or playing around, the learnings that you'll get will carry you through actual production deployments significantly. So here's another example. Thomas built a translation bot, and that's on GitHub. So it sits there. GitHub actions waits for a new pull request to come in with a new documentation update or a change, et cetera, and just like uses lms to translate that.
Alex Volkov [00:59:25]: So if you think about okay, one example is a chat bot that writes copy for ads. Somebody in marketing needs that answer right now. Otherwise they're going to go to GPT four and just shove everything they have in the context and just go there. And basically the diminishing returns will play in GPT four's favor here. Documentation is essentially our thing that if you don't do automatically, user will have to go and copy. And remember, like automation here is a benefit, but documentation doesn't have to be real time. The user does not sit in front of the answer and wait. And so what this allows you to do is now use the trade off calculation that you just mentioned in favor of a higher quality.
Alex Volkov [01:00:06]: Maybe you run the translation once and you run this again, maybe you do two or three prounds, maybe you do different thing because the user doesn't necessarily wait. Then suddenly your calculation changes, your cost calculation could change because of that. Maybe you go for a cheaper model that's slower your time to inference. Maybe response time is not the only thing. Maybe also evaluations are different in that case as well, because I definitely encourage folks to consider the user and how this use case is getting deployed at the end to definitely roll back and say okay, what are we optimizing for? Optimizing for inference speed or optimizing for hallucination removal because those, like you said, there's a bunch of papers retrieval or re ranking or all these tools, tree of thought, like all these things that take three of thought, chain of thought, they take more money in form of more tokens and more prompts that you run, but they can lower significantly hallucinations as well. So that's one consideration also to take into account in the back and forth between features.
Demetrios [01:01:12]: Yeah, you mentioned the LLM as a judge type thing, and that is something that I feel like is super hot these days. But it is another trade off because now you're making another call, you're making another API call, and you got to pay for that. Right? But the other thing that I was going to say about all of these different trade offs that you're looking at and what the end user experience is, I like to talk about something that Sahil Jain, I think, spoke about. He's a product [email protected]. And he said that for them, it's important to always be as fast as possible. Right. But you can't always be fast, because these models just aren't always fast. And so what they've been trying to do when it comes to optimizing for speed is he talked about the two metrics that they would look at.
Demetrios [01:02:11]: One is the actual time it takes to get the answer, and then the other is the perceived time that it takes. So what they're trying to do is build in these little tricks while you're waiting to make the perceived time go down. And so maybe that's. You take a quiz while you're waiting, or you get some kind.
Alex Volkov [01:02:31]: I have an example of this that I built, if you want to hear.
Demetrios [01:02:34]: What'd you.
Alex Volkov [01:02:35]: Yeah, yeah, of course I built a built. Okay. I created the custom GPT on the GPT store, and it went kind of viral as well. It's called visual weather GPT. So basically you provide like, denver or whatever, Seattle, and it will generate a dally image. You will go to bing, browse the weather for that area, and then generate a Dali image using a bunch of prompting and star prompting for that weather that day. So that's pretty cool, right? You get like a super custom art for your thing. And that went viral.
Alex Volkov [01:03:06]: And now I was singing there and I was like, yo, this is slow. Dally is kind of slow. Browsing is kind of slow users waiting. What's fast? Spinning tokens is like super fast. So I came up with a thing where it spits out a poem about the weather, and it spits out like four paragraphs of a poem, and then it starts generating the image. So by the time the image starts generating, the user sits there and reads the poem. By the time they read the poem, boom, the image generated.
Demetrios [01:03:29]: Nice.
Alex Volkov [01:03:29]: Nothing changed in performance. If anything, I made it slower. But essentially for the user, the experience is better. So definitely agreed. Perceived. It's like that airport story, I don't know if you know, that people complained that they waited long for luggage, and so the airport routed the people around, like a long way to walk for ten minutes, and they walked basically in circle ten minutes. By the time they get to the luggage thing, luggage was there. Nobody complained anymore.
Alex Volkov [01:03:54]: So it's kind of like that.
Demetrios [01:03:55]: But these aren't new challenges, right? I think about one of the stories I heard with Instagram when it was first coming up and how they would make the uploading time of your photos be so fast, and what they would do is you would take your photo, put it on the app, upload it, but you wouldn't actually post it yet, and you would have to write the caption. And so while you were writing the caption, they were already uploading the photo, which made it seem like everything was so much faster, because then when you actually did post it, it was either already posting in milliseconds, or it was like a second that you had to wait. And so it just makes me think about how we can look to the past for inspiration on this. It's not like it's necessarily the first time that we've encountered challenges where it's very laggy and it takes a while to do something because the tech isn't quite there yet.
Alex Volkov [01:04:59]: So I would say, if anything, this is maybe a sign of maturity of this field that we kind of all stumbled on. Many people did ML for a while, and then chat GBT came out a year and two months ago and completely upended everything. This is the maturity of building these tools into production that we're getting to the same user centric kind of issues, ux issues that have been there for a while, even in these tools. I think that's a good thing. That's generally like a step forward in a direction. We're like, okay, there are people who've been building these type of solutions for these type of problems. It doesn't matter whether the backbone is LLM or the backbone is something else. I think we're getting towards like, okay, now we're no longer in the play phase.
Alex Volkov [01:05:38]: We're now in, hey, let's go to production. In production, there are users, and that phase is very interesting to me as well, that shift, dude.
Demetrios [01:05:46]: So talk to me about another, what I would consider topics that tends to blow up, especially on Twitter, which is agents. And how much experience do you have with agents? What are your feelings about them? And just give me the overall vibe check that you have on them.
Alex Volkov [01:06:06]: So a few things here from personal learnings, but also from talking with people who built a bunch of very cool, cool things. So maybe people remember Chat GPT blowing up, sorry, excuse me. Maybe people remember auto GPT blowing up on GitHub becoming the fastest growing GitHub project in stars, et cetera. I met with the auto GPT team, and during one of the conferences, they're very cool folks as well. And many people got excited about the idea and then fairly quickly learned that, okay, the grass is not that much greener in the agent world because of hallucinations, because of inability to execute tasks, for example. And then I met with a bunch of folks that did agents. I remember distinctly this one very important milestone in the agents world, where many folks went to a hackathon about building agents. And Andre Kapathi from OpenAir was there.
Alex Volkov [01:06:59]: And Andre Kapathi basically said this thing, he had like a seven minute talk, and he said, hey, if you go to OpenAI and you suggest like another transformer architecture, you suggest, like, state space, transformers, whatever you suggest, the RWKV people have tried this out. There's like phds in there. They wrote papers of why this works, doesn't work. They tried all the permutation with transformers, mixture of experts, like all these things. If you talk to them about agents, at least that was the case in the summer, they're fairly as new to this as you would. He was talking about this in hackathon. He said, basically, you guys are at the frontier of what we can do with these models, because the idea of running them in loop is also new to us. We just came up with GBD four.
Alex Volkov [01:07:40]: Before GBD four, it wasn't even possible. GBD three could not execute on tasks that it wrote for itself. It just didn't have as much. Sorry for the pun, agency. And now what happens is there's a bunch of these agent frameworks, and the latest that I know about, and I know people are getting excited about this crew AI. But this guy Joao Mora and I had draw on the podcast, actually. So if you want to check this out, I'd be happy to send you a link. We did a deep dive, drop it in the show note, and specifically why he chose to write his own framework, where like 17 other frameworks exist already.
Alex Volkov [01:08:15]: And we also had a chat with Killian Lucas about open interpreter, which is an agent on your Mac that actually executes code and runs it on your Mac. So kind of like a code interpreter, but like an interactive one. And the interview of Killian is also great. And what I notice is that we're getting better. And by we, I mean kind of llms are getting better in context generally, right? So, like, one trend of 2023 to me personally, was context length are exploding. GPT four started with four, and now we're at 128, and I had the folks from the rope scanning paper as well on. So context is getting solved, even though the quadratic invention still is a problem. And context is very important for these agents because you can only do so much with rag.
Alex Volkov [01:09:01]: Okay, you can go and save the memories and the task and everything in like a vector database, retrieve them at the time and say, okay, the user wanted this and that. At some point you need context. You need this model that runs your agent to remember, oh, you asked me a preference of yours to send your email in your tone seven days ago. And that's like on the top of the context, if at all. And so I think context grows and the more so auto GPT, when it came out, we only had like 4000 tokens in a context possible window. Now these tools like crew AI, they can send like books. So the great Gatsby, I think is around 70,000 tokens or so, right? Chad? GBT now supports 128 over the API, so you can literally send the Greg Gatsby's book like context. It would basically understand all of it.
Alex Volkov [01:09:46]: I think that's a crucial piece for agents as well. Another very important piece is evaluations, and that's also horrible in agents. If we talk about evaluations for a basic thing, for a rag, a very basic, like you go, you retrieve some stuff, you answer the user, maybe you do something else. Evaluations of whether or not the agent did what you needed to do, whether the task was completed successfully. That's significantly more difficult because you don't build them to be specifically task focused, you build them to be generic. And so there's a bunch of approaches to agents as well. The cool thing about crew AI, the guy that I talked to is he actually kind of came up with basically an assembly of agents, each with a specific task and a specific prompt. They're all going to assemble together like a team to do a thing.
Alex Volkov [01:10:32]: And that's pretty cool. That's why he called it crew AI, because in crew there's one person shouting directions. Everybody else like rowing. So he specifically has this approach where every agent is a specific task. And kind of like we said about evaluations, you can evaluate every agent with a specific task as well, and then in accordance of all of them together, they come up and do a thing. I also tried multi on, which is super cool. I've tried a bunch of them. I'm still waiting.
Alex Volkov [01:10:58]: I'm still waiting because I haven't yet been able to replace a core part of what I do, which is as I learn an x every week about the cool stuff in AI. I just drop a note and say, hey, I'm going to talk about this on my podcast. I'm going to cover this. I'm going to cover this every Wednesday before Thursday. I need to go on x, find all those replies, compile them into a thing to show notes. So I have the show notes. I wasn't able to find or build an agent that does this for me easily. And until I do, I'm still waiting on agents as like this panacea or promise in this AI world.
Demetrios [01:11:31]: You've got a clear use case, though. That's awesome.
Alex Volkov [01:11:33]: I have one, and it's like an evaluation basically, that I can test on.
Demetrios [01:11:37]: Yeah. Have you messed around with DSPI?
Alex Volkov [01:11:42]: So I've seen DSPI on my feed like everybody else. And this week we're going to talk with, like, I'm planning to learn a little bit before the conversation. I'm going to talk with Benjamin Clavy and Connor from the Wavy podcast because they dove into DSPI and had very interesting content about this. So actually, if you ask me next week I'm probably going to have more about DSPI, but I know for a fact that that's very interesting. And as people mature in these products, they understand that, okay, maybe prompting, like, you would talk to a human, that's not the thing that we want. Maybe we want to actually treat those machines as machines and to program them a little bit. I think that's the main idea from the spy.
Demetrios [01:12:25]: Excellent. Yeah. When you do play around with it, let me know. We talked to Omar a while back, and I'll drop that in the show notes, too, because I think you're going to like it a lot. And while you're doing a little bit of studying on it, you can listen to the episode that we did with him. And it is so exciting to see how much because it takes a little bit to understand what's going on, right. And I look at it as like you throw something at it and then it gets broken up and there's like all these prompts that kind of race to see which one is the best, but you don't have to go and you don't have to create all these prompts. It just automatically does that.
Demetrios [01:13:07]: Well, man, I've taken up a ton of your time. I appreciate you coming on here and chatting with me and us geeking out on everything from podcast gear to evaluation metrics. It's been a blast, and I love what you're doing with Thursday AI. This is great, dude. Hope to have you back.
Alex Volkov [01:13:26]: Thank you so much. Yeah. So folks are more than welcome to join our live streams. They're very interesting folks who build the stuff that we talk to often come and talk about the stuff they built. And I find that maybe one of the best parts of the community that I reported something that was released this week and oftentimes, at least in the smaller know, Jeff Dean from Google is not going to come probably. But we had folks from news research, we have folks in the open source community, we have folks for Gina embedding AI. We have like a bunch of very interesting people that talk about the actual things they built. And I find that incredible in the Thursday community.
Alex Volkov [01:14:00]: So definitely invite folks to check that out and obviously shout out to the coolest place to build your llms and track your LLM data. So. So definitely give us a follow.
Demetrios [01:14:12]: Well, this has been an absolute blast. And we'll leave all the show notes for everything. Or we'll leave everything in the show notes, I should say. Thanks so much, Alex.
Alex Volkov [01:14:25]: Cheers. Bye.