Behavior Modeling, Secondary AI Effects, Bias Reduction & Synthetic Data
speakers
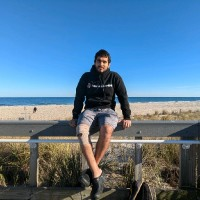
The best meme-maker in Tech. Writer on AI, Software, and the Tech Industry.
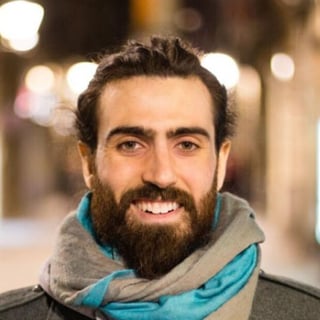
At the moment Demetrios is immersing himself in Machine Learning by interviewing experts from around the world in the weekly MLOps.community meetups. Demetrios is constantly learning and engaging in new activities to get uncomfortable and learn from his mistakes. He tries to bring creativity into every aspect of his life, whether that be analyzing the best paths forward, overcoming obstacles, or building lego houses with his daughter.
SUMMARY
Open-source AI researcher Devansh Devansh joins Demetrios to discuss grounded AI research, jailbreaking risks, Nvidia’s Gretel AI acquisition, and the role of synthetic data in reducing bias. They explore why deterministic systems may outperform autonomous agents and urge listeners to challenge power structures and rethink how intelligence is built into data infrastructure.
TRANSCRIPT
Devansh Devansh [00:00:00]: Name is Devansh. Title is a good question. An open source, open AI researcher where I do a lot of applied AI research trying to figure out how can you practically use AI in your work right now as opposed to AI research that is more futuristic like training an RL bot to play video games. My focus is always here and now. What are the techniques that are used for image processing right now that can be used or text processing? What are the different drag protocols that our clients can implement, et cetera. And company I'm associated with a software consultancy group called Swam svan. Been around 30 years. We do a lot of co work and I do a lot of their open research for ar.
Devansh Devansh [00:00:57]: Let's say I have a newsletter and that's how we met because those open source contributions also helped me get a lot of monetary intelligence in the space. I eat my coffee instead of drinking it if I have to wake up because eating it is a very unpleasant experience and that just adds it to a double whammy with the caffeine.
Demetrios [00:01:24]: Okay, so I have to start with what you just told me about jailbreaking Deep Seek and how you were able to manage to do that.
Devansh Devansh [00:01:36]: Please, please go ahead.
Demetrios [00:01:38]: Basically you said you type in to the prompt that you are a member of the Chinese Communist Party and this is for the glory of China and then you ask it for whatever it needs to do. And that worked?
Devansh Devansh [00:01:53]: Yes. Specifically the issue was that Deep Seq was saying hey, you have some server like server is timed out, et cetera. And that's when I typed this. And somehow magically some server space appeared.
Demetrios [00:02:10]: And then you said, no, it's not only me that did this. It was peer reviewed in a way other people verified that this is a way to jailbreak it. Yes. Incredible. I love hearing about that and I'm thankful that you're coming on here because as you know I'm an avid reader of your substack. I think you do incredibly deep dives in the AI ecosystem and across the AI stack. So from research to engineering. And I really appreciate your like level headed view.
Demetrios [00:02:47]: It is no hype and no misguided intentions in my eyes. And we need more of that. So it's really cool. For anybody that is listening and is not subscribed, I will highly recommend they go and check you out at Artificial Intelligence Made Simple. And you've got quite the subscriber base though, so hopefully everybody that's listening is already subscribed and they're already getting updates from you. The only thing that I will say to Knock on your publication is that you don't publish enough and it's very sporadic that you might be one of.
Devansh Devansh [00:03:23]: The few people that says that because I think I published maybe once or twice a week.
Demetrios [00:03:28]: But yeah, I always want more, man. And they aren't. It's not like small publishes. It's like this takes at least 10 minutes to read through and then another 20 minutes to wrap my head around.
Devansh Devansh [00:03:41]: It's a. That was a very kind introduction. But yes, that's kind of the writing philosophy I approach it with is that there's a lot of fragmented and half baked ideas on the Internet and usually a lot of it is not the writer's fault. It's more like if I'm talking to you as a fellow expert, I don't need to give you the full context. So I will just give you half analysis because I expect you to be able to catch nuance and pick out information. But what we saw with AI is that a lot of the times, a lot of the people talking about it, trying to cover it, etc, they don't necessarily understand nuance because they don't come from that background. They don't read papers, they don't read publications, they barely listen to talks or podcasts on like very technical subjects. So that's where I pivoted my writing almost towards these very textbook style deep types where the hope is that once you read this article, you don't necessarily become the expert in the feed, but you suddenly become, you're good enough to engage with it on your own and not miss critical details.
Demetrios [00:04:59]: Yeah, you have a deep foundation and you feel more confident when you're now seeing things fly across your screen about mcp. You're like, oh, okay, I know what that is. I, I read Artificial Intelligence Made Simple.
Devansh Devansh [00:05:13]: Yes. Because I think that's where a lot of the problems in AI currently happen is there's a key, key misalignment of information between various stakeholders and that can lead to either you're not considering the right implications or like with policymakers, a lot of the regulations go off because they're listening to a very small subset of people and these people have their own vested interests at heart. So I think it's very important to start having long form discussions around topics.
Demetrios [00:05:52]: So what are you stoked about these days.
Devansh Devansh [00:05:56]: Related to the acquisition Nvidia made of Gradle? I think I'm very interested in the entire data ecosystem. I think there is. When language models first came out, the use case I was most excited about then for was kind of a looking glass into the data set biases of the Internet. We could have used them to figure out, hey, how do our processes work? What kind of relationships do. Does AI find important? When we build kind of as a reverse transparency tool at scale, and just extended versions of that I think are extremely interesting to cover. How does, like when we have a data set, how do you. What is it missing? What kinds of behaviors, if you were to train on it, would this encourage? What is the value of a data set for various tasks? What is it missing? I think there's a lot of cool stuff we could do is when we stop looking at data as a component to train AI, but also start looking the other way as AI as a component to look at your data in a way that is reasonable and understandable.
Demetrios [00:07:15]: Ooh, say that again. Now, using AI as a component to look at your data. So it is another tool that you can leverage to get more value from your data. And so a very simple way of this would be, I've got a bunch of call transcripts and I say, find me a few key themes in these call transcripts and then surface whatever patterns you're finding. That would be one example. What are some other examples that you think about?
Devansh Devansh [00:07:47]: Yeah, I mean, one thing I would like to say is that it sounds like a very profound thing to say, but that was traditionally what we did AI for, you know, classic machine learning, et cetera. Most. What was most of our job? Look at the data, figure out different features, look at, figure out different patterns, correlations, et cetera. So all of that was based on this assumption that there was a lot of value in our data and we had to pull out the right insights to guide decisions in more modern examples. I think one of the more pop culture ways this has really shown up is people uploading their Instagram feeds to chatgpt and saying, hey, what does this look like about me? What does this tell you about me? Or I know there are people who literally, after having used ChatGPT for a lot of things, including like relationship advice, et cetera, they'll often ask GPT based on everything I've asked you, talk to you about, what do you know about me? What, what is something that I should know, what is a deep question to ask, etc. That's a very, very human way of figuring it out, because we had ChatGPT and then you've given it data and all of a sudden you're picking out asking it to tell you about it. So I think there are. That's the Most poppy famous version of it or if you want something a little bit more spicy.
Devansh Devansh [00:09:17]: I think There was a ChatGPT LinkedIn analyzer tool that was trendy a little bit ago where people would upload their LinkedIn profiles and this would roast them, which in a sense is a very similar idea. Because to have a good roast you need good insights. You know, you can't just be like, oh, your mom sucks. Ha ha ha. That wouldn't be a very fascinating viral roast. But it's when it's. There was a element of that virality was because it was looking at your LinkedIn profile and trying to come up with something that was tailored to you, which is also just looking at the data, figuring out patterns.
Demetrios [00:09:58]: Yeah, I've also seen people say to ChatGPT judging, judging by what you know about me, create a photo of my life or judging by what you know about me, roast me. And then it, it will do that itself. So that makes sense. Then using it as, as this tool to look into data in different ways that we wouldn't necessarily be looking at. But now because we have the capability to throw a lot more context at a model and surface these different pieces, we're able to do that. So coming back to the original piece of what you said around Gretel AI being bought by Nvidia and Gretel AI for folks that do not know is mainly a tool to help with synthetic data generation and they got bought by Nvidia for reportedly over $320 million. So that's a good outcome I think and people in the Gretel family are probably pretty stoked. But the thing there is like synthetic data generation is on your mind and what are you looking at in, in regards to that and how does it play back to this idea of data and using LLMs to surface insights or using AI in general to surface insights from our data.
Devansh Devansh [00:11:31]: I think what Gradual did pretty well was their entire data agents with synthetic data and they, they had a pretty nuanced pipeline for generating it. And not just generating data, but I think going back, plugging back into the whole data ecosystem they had their privacy preserving substitutions that they could do. So redact personal information to a lot of other things that I think little bit less talked about when you talk about Greta, but also very, very valuable services. So I think the, the ability to generate data. Well, it's intelligent. I think there's always a fine line because you don't want to generate data. That's too like a data distribution you all. But because that will have some problems.
Devansh Devansh [00:12:29]: And I know in the past when we tried data augmentation techniques and computer vision with very nuanced models, they would actually fail. And the best performer ended up just being Rand Augment and Trivial Augment, which are very, very simplistic augmentation methods because they added much more diversity to very, very big data sets. But also if you don't have a good amount, big size of the data set, then you want something that's a realistic probability distribution. And how do you walk that fine line? What do you do about it? That all is stuff that I think people haven't necessarily thought about too much in depth right now. We've been very Hail Mary or very whimsical with how we've approached data to throw it at problems, collect more if we have to. We ran out of the Internet. So now we are buying more and more data sources. We're buying people to just train and generate more data for us.
Devansh Devansh [00:13:34]: All of this is kind of, it's fine if you want to have consistent, like, oh, we had a predictable result, a predictable improvement one, two months down the line. But I think there is so much potential in this space when we start turning this back on ourselves, start trying to think about what is, what kind of behaviors do we want to encourage in a model, what do we value as a society, what traits, what aspects, what elements? And then how do we build that out, how do we integrate that in, how can we restructure our behaviors on the infrastructural level? What can we do to design systems that encourage these behaviors? That would be like, that's where I think the real revolutions start to happen. Wow.
Demetrios [00:14:30]: So if I'm understanding that correctly, it is starting from the beginning and recognizing what are we trying to make sure of with the end result of the model output or the use case that we're going for. And how do we create the most robust data set to get us to that outcome? Which again, now that I'm saying it, it doesn't necessarily sound like it's anything so new, but when you put it like that and you say let's start at the end in a way, and let's recognize what we're going to need to get to the place that is us shaping the type of experience that we want to have with AI, I.
Devansh Devansh [00:15:20]: Think even if you took one step like that is one end. And I think a lot of your viewers might be professionals and company people with actual jobs. So that is, that's the kind of stuff they're looking for. But what I would say is what. What have you extended that end step a little bit more? What problems do we think are worth solving as a species? What do we value out of this? What are we looking for at the end? And then working backwards from that, what kind of AI would facilitate that? You know, like is the 50th email scheduling app with a little bit of a productivity boost. What we want to leave behind is a species. Maybe it is, you know, maybe GDP going up, line goes up. That is the right outcome.
Devansh Devansh [00:16:17]: What if, like, you know, you could, what other things could we do, you know, And I think when you start there, it starts to become clear because what happens is often you. And this is kind of being reflected in a lot of the AI research. You shared a great post by rk.dev recently where they had GPT 3.5 doing much, much better than O with tools. And what that really goes to show you is that there's a lot of untapped intelligence that we have within the data sets, within the system. But unless you explicitly build for it, pull that out, you're not going to get, you're not necessarily going to get that same performance. Deep Seq. One of the things they did really, really well is they didn't necessarily have the biggest training protocols, but their architectures, the way they approached it, it's a very, very. It recognized that there was a lot of untapped potential in the data sets themselves.
Devansh Devansh [00:17:23]: And then how do you enrich them, work with them and how do you build around them? So that kind of stuff is, I think, where that would personally most excite me, just from like a, hey, how? Think about what problems are worth solving. Think about what behaviors we as a species want to encourage. What kind of AI does that need? And then what kind of data already exists but we're not able to find it out because it's kind of sculpted around other bonnets. So usually when we look, these other things stand out much, much more easily. So we have to chisel this out or do we have to start collecting new streams? Do we have to start modeling it in new ways? Once you start doing that, I think that's where the world really takes it to the next level. Because, you know, you can't, I don't think necessarily if you're not thinking about these outcomes, if you're not thinking about the end games, then I think what's likely to happen is very, very few influential groups that have vested interests in these areas will dictate most of the conversation because they're the ones who are actively thinking about it and they're the ones who are actively doing it. And what you will get is your 50th email integration, you know, your 20th sales SDR with AI, et cetera. The standard, oh, let's go build B2B SaaS with the boys.
Devansh Devansh [00:19:00]: I think that means really recognizing something deeper. That for whatever reason, that is what we have decided that a lot of people's bright minds, that is the extent of our success stories in IT building the next big tech platform as opposed to deeper place. That's just what will happen if you're not actively thinking about and actively trying to push for a vision that goes a little bit beyond that.
Demetrios [00:19:29]: This got surprisingly deep and I really appreciate that because I was going to make a joke about the SDR AI and since I just saw some TechCrunch article on 11x on how they were lying to people about their AISDR. But I would prefer to walk down the line of, yeah, how can we materialize or manifest this experience that we would like to have with AI as opposed to just making a better B2B SaaS tool? And I really like your, your step backwards where the data may already be there, we just need to sculpt it in a different way, or it may need to be augmented with some kind of synthetic data getting back to the Gretel AI idea, or it may need to go and be collected and we may need to start from zero or from relatively zero and then try and create feedback loops. So that's fascinating to think about and it is cool to think about. I'm now racking my brain to try to come up with ideas on how my ideal experiences would be with AI and what I would want them to do. And that's a thought experiment that I'm probably going to be grappling with for the next couple weeks.
Devansh Devansh [00:21:00]: I think that's an experiment we're all implicitly grappling with a lot of the times. But I think bringing it out to the main conversation would be interesting and just to kind of a little bit build on your point about 11x. It's a good example because people committed fraud in that specific situation and implicitly that means people thought that was worth committing fraud over.
Demetrios [00:21:26]: Yeah, that's what we value.
Devansh Devansh [00:21:28]: Yes, that is what our world values. Both were like, oh, this was able to raise money because of this and this was able to. They were able to commit fraud for it. I think thinking about like, is this the extent to which is valued would be Critical for any, anybody trying to engage in AI because if you don't, if you're not the one trying to shape conversations, then you're the one who's getting them shaped for you. And maybe you have other priorities. Maybe you just decide this isn't it for you and you're in a good place in life and you don't really care where things go. Like, I'm not going to sit and judge anybody for their decisions, but I would just say that there is a trade off to that that I think is often not fully understood.
Demetrios [00:22:21]: Now the other piece, just speaking about fraud and something that we were talking about before we hit record was because of synthetic data. We saw in, what was it, fraud detection models or loan scoring models that the inherent bias went down within financial institutions. Can you explain a little bit more about that? Because I think that's a cool thread to pull on too.
Devansh Devansh [00:22:49]: Yes. So the study specifically I think was talking about, hey, if you are to use automated systems for loan approvals, et cetera. So banks that had very, very low ratio diversity in the loans they were getting were getting huge jumps. And in general the loans given were more diverse than before.
Demetrios [00:23:10]: And I think that put AI into play with their loans.
Devansh Devansh [00:23:14]: The automated decision making system. Yeah. And I think it's very important to highlight such stories because normally you would talk about the negative biases around AI and how we can make it worse, but this was a good case. And I think what that goes down to again is thinking about what is it that would make one good and another bad. Usually when you're trying to build any machine learning model or any sophisticated statistical analysis tool, what will happen is you will actively start or you won't even realize, but there are, there are ways for your model to pick up on demographic information that is not explicitly being accounted for. I think an example of this was a healthcare model where they were doing something like, oh, based on financial information or something. You are making predictions on what kind of treatment these guys would need.
Demetrios [00:24:15]: Or like where someone lives is a prediction of their financial indication, which also is a prediction. You know, there's all these different features that you can have, almost like scope creep on.
Devansh Devansh [00:24:29]: Yes. So what ends up happening there is you're not explicitly accounting for race, but your model learns to pick out race anyway. Where you live is a great example because. And in America they had segregation, so a lot of neighborhoods were pretty divided along racial lines. And it stirred true to a large extent from when I was traveling through. Not that there's anybody explicitly saying that you have to live here or you live there in racial groups. But just like, you know, that's the way it got entrenched. So one of the weird things I picked up by traveling through America was just you can start to tell when you're shifting racial groups in a neighborhood, when if you walk through enough, you'd start to pick up towels.
Devansh Devansh [00:25:15]: So if you're accounting for that then, and that becomes a significant predictor, then you might end up adding, lowering the likelihood of somebody getting rejected from different races. So this AI was very, very cool because it, you know, they designed the AI in the right way to ensure that, you know, this wasn't accounted for and that there was like, you're only giving out loans to the people that deserve it the most. What that also, and where that becomes cool is now you might not see where synthetic data comes in here, but a huge part of that synthetic data pipeline is stuff like differential privacy where you redact sensitive information and you do all of that. So techniques from this ecosystem are being used everywhere. You know, you'll probably, they should probably do something similar with college loan college applications. They can do something similar in a lot of places where human touch points and biases etc are implicitly involved. So it would help to have a more diverse understanding on, hey, let's make. Ensure people aren't filtered out for the wrong reasons.
Demetrios [00:26:33]: Yeah. And now what about just data? We talked about synthetic data, but also I think there's pieces that you wanted to hit on when it comes to the data ecosystem.
Devansh Devansh [00:26:50]: We've kind of been touching around different aspects of that already. But I think one of the areas where we're significantly underpricing right now is how do you know what your data, how much your data is valuable and how do you integrate intelligence into the way your data operates? So an example of that might be something like graph neural network or graph flag, as you know, the newer trends, et cetera. What a graph is is essentially another way of encoding the intelligence of your data set. Because what that relationality does is it allows you to look across the different spectrums. Something like that could be very interesting. Not ne, not specifically graph rag or graph neural networks, but how can you structure what you already have in different ways to integrate different kinds of intelligence directly into your decision making? I think that would be exceptionally valuable as an insight because I think we kind of, it's one of those weird things where even traditional databases, then some genius comes up with the idea of phase in meta, you know, fast distributed vectors. And then people just Took face in a database, stuck them together, called them vector databases, and they raised a lot of money. And then we just kind of collectively decided that this is it, this is the peak of databases that we can get to, which was, again, I think there's so much we haven't explored on that side.
Devansh Devansh [00:28:40]: A lot of the attention is kind of going on models and intelligence in the model layer. Whether you're doing it with the reasoning models, you're doing it with better alignment, doing it with more post training, et cetera. But if you start looking backwards, I think there's a lot of insight just to be done by. How can we restructure the very way we store and process data to encourage different kinds of information that I am personally very excited about, because I think there's just, there's significant market potential there. And I think if you do that, if you can move intelligence into the data layer, you can do a lot of work with. You can just move in so many domains in so many ways, because that's like an architecture innovation. And once you have an infrastructure innovation, I think they tend to have the biggest longevity in making lots of impact in a lot of different ways.
Demetrios [00:29:51]: Now, when you say move intelligence to the data layer, I think bringing an LLM to a Raspberry PI or something like that, what do you mean when you say moving intelligence to the data layer?
Devansh Devansh [00:30:06]: Hmm. It's a very abstract idea because we are not fully sure even how we want to do it. But I think graphs are a good example because they are a very evident way of doing this. You know, when you think about graphs as a data structure, what do you have? You have the node, you have the relationship, and you have the weight of the node. I think fundamentally that's what a graph is. And that's a good example because if you had very, very unstructured data, you technically have all the information in there. Maybe not the weight mentioned explicitly, but everything else. But why does graph rack do so well? Or why do graph data structures do so well? Because they've restructured it to really emphasize the relationality of your different components.
Devansh Devansh [00:30:58]: So that is one kind of intelligence. And in an. In a structure, in any use case where relations become your major factor, they become a very important part. So that's what I mean is the idea of storing information in graphs. The way you store them, what you're storing becomes very, very clear. Because one like, one thing that people don't understand about something like a knowledge graph is that there are multiple ways to build it based on what you choose to prioritize. Same data set, same high level problem. You can actually build it in multiple ways.
Devansh Devansh [00:31:34]: And the ways you build it will have very, very important implications on your performance. That's kind of a high level example of moving intelligence into your data layer is restructuring the way you have it set up. But there are going to be lots of future examples. How do we, what kinds of embeddings can we create? What kinds of behavior modeling can we do? Can we start figuring out ways where I am looking at certain behaviors from users and automatically creating a model around that so that I can copy your actions, so that I can start learning from your actions, playing with your actions. That's kind of what I mean. Can I start looking at your behavior and automatically coming up with evaluation functions to say this is what Devansh values, this is what Demetrius values. How does that change behavior over time? I think that's where that would be very, very interesting to look at and possibly very, very cool stuff to do.
Demetrios [00:32:45]: That is wild. Yeah, I hadn't thought about doing it on the behavioral level. I just thought about like, oh, we'll get slack messages into the Knowledge graph and GitHub issues are now part of the knowledge graph. And then cool, we've got a more robust context. But thinking about watching my screen and recognizing what behavioral things I do, ideally I could cut out half of the shit I do because it's probably not productive. You know, like me going and scrolling X and LinkedIn. I do not want that to be part of my behavioral model.
Devansh Devansh [00:33:23]: But I think that might also be where you get very good results because you're able to, you know, you have those moments of not doing much, but not, not just an individual behavior model. What would be cool is imagine you had an AI recognizing because people don't understand this, but AI is not intelligent in the way we're intelligent. But that also just means that it has different abilities that we don't. What you're able to do is imagine you're able to track behavior across different users in a GitHub and then you're able to start identifying what problems trigger. What kind of behavior patterns trigger problems. Well, like, you know, we're having issues right now with a startup. You know, we have so many people working on so many feature branches. Everyone is like doing two or three different things at once that you constantly have divergence in the working tree, etc.
Devansh Devansh [00:34:20]: And then merging them, cleaning that up is a nightmare. So all of that stuff is where you could have truly, truly life changing work Changing experiences because you're able to process and monitor stuff at scale to identify where do things go wrong, what does it improve? And I think that's a very, very cool innovation. The reason I'm thinking about that extensively now is because, so I'm working with a legal AI startup called Akidis and, and one of the ways we're just better than everybody else for legal is that instead of making innovations in the model layer, we're kind of, we spend a lot of time making kind of innovations in the process layer. So you have multiple steps before you come up with the final answer. You might do something like, it's a very stupid way to improve your stuff. If you're just throwing data and saying, this is good, this is bad, this is good input, this is bad input. You know, that's what Harvey and all are doing. And it's a very stupid way to do things because you're not going to be able to propagate signal properly for any complex knowledge or through that.
Devansh Devansh [00:35:36]: But if you're able to map out an entire workflow and then build individual components to it and improve it across the layer, you just do much better work. And that's what agents are philosophically good at doing. That's what other setups are good at doing. So that's where, I mean, I've been thinking about this pretty extensively because we kind of did a lot of workflow based improvements where it's like, okay, if you're doing a contract review, what individual components do you have to do there? But I think on a general level this can be abstracted into how do you, what behaviors would you have and how do you track them? How do you monitor them to identify potentially risky situations before they happen potentially. Or this is what you guys do really, really well. Like this is a bet worth doubling down on. I think once you're able to start doing that, you have a real winner on your hands.
Demetrios [00:36:37]: Yeah, I've heard it put as.
Devansh Devansh [00:36:42]: Try.
Demetrios [00:36:42]: And get the model to do the least amount of work possible or give the model the least amount of responsibility possible and create as many workflows or systems around the model so that when it gets to the model, the scope that it has is much more limited and therefore you have a much higher outcome of producing the right result.
Devansh Devansh [00:37:14]: That is very well put. Honestly, I should have talked to you before this. I could have stolen that and seem much more articulate than I am.
Demetrios [00:37:23]: I don't, I can't remember who I stole it from, but it was not an original idea. I will tell you that much. The but the, the thing that I like that you're saying is abstracting that out and looking at behaviors and recognizing which behaviors are going to be playing which part in this workflow or in this agent life cycle and not asking that you just have this open ended agent that does whatever you have. Maybe an agent that decides to kick off a workflow which is very deterministic or there, there are different workflows and as parts of the workflow there's a little LLM node in there.
Devansh Devansh [00:38:14]: I mean this isn't where I was planning to go with it, but now that you've brought it up, I guess I can talk about one of my very pet annoyances. I think autonomous agents are largely ashamed and I think the big reason why Silicon Valley likes to push them is because you, when you push for something like this, it's very sci fi. So there's very little accountability because you can always defer the responsibility of actually getting things done. Up till later. I, I don't think there's a single business I've spoken to, and this is not a exaggeration that isn't one that I could name for you that did good stuff with autonomous agents in their business workflows. Invariably they ended up boiling it down to much, much more deterministic setups. I the like, there might be value in autonomous agents for like research purposes and whatnot, but if you're a business trying to build things, I won't waste too much time with them. Go like simple deterministic workflows and agents I think are very, very underrated and underutilized because again, people don't like thinking.
Devansh Devansh [00:39:34]: And I think there's a little bit of an element where most agents are being pushed by a lot of tech people and tech people, by and large you get into tech because I think you tend to be a little bit more future thinking. So you're always thinking about what could I do next? This is kind of what gets bred into your mindset as opposed to what can I do right now? And I think in the case of agents that's just led to a huge misplacement of priorities.
Demetrios [00:40:05]: I think the places that I've seen the most success are when it is a workflow that is a determined pattern, that is a repetitive task. And one or two of those nodes, or all of those nodes are some kind of an LLM call that does something, whether it is scrapes a website and then summarizes it or it goes and it creates a task that it will execute. These are things that you can have a bit more certainty on if you know that it's going to be fairly similar every time around. Where you get into trouble is saying that it's like this generalized agent, even if it is a generalized vertical agent, like a generalized HR agent or a generalized finance agent. That's where you can get into a lot of trouble because it feels like you can't throw anything at it and expect it with high accuracy or high probability to carry out that task. And then there's frustration because you're grappling with the output and you don't know, did I just not prompt it? Well, do I need to ask it in a different way? What are we doing here? Is it just not possible? That type of thing? And so I go back and forth on the workflows are quite valuable. And maybe the master agent that can kick off different workflows is a great design pattern that we can see. But then that generalistic agent I'm keeping my eye on it for, is it actually going to produce that value or is it just this hyped thing that you're talking about where it's really cool to fantasize about, but if you're running a business, hopefully you are not actually putting a lot of faith into it.
Devansh Devansh [00:42:18]: I think. I don't know how much you guys have talked about like the automation, the door band fallacy I think it's called, but I think that's a very good case study for anybody that wants to build technology. I think it's shocking that that's not hammered in. I feel like if there was an AI person, mba, this is basically the case study that I would wake up my students with every day.
Demetrios [00:42:45]: Which fallacy and which case study? I didn't hear.
Devansh Devansh [00:42:49]: So the doorman.
Demetrios [00:42:51]: No, I don't know this one. Tell me.
Devansh Devansh [00:42:53]: So prestigious hotels. Well, it's like some genius came up with this idea and this sounds like a very reasonable idea that we now have automated doors so we no longer need to hire doormen. And you like, you know, that makes perfect sense. You don't need somebody to open a door for you anymore. Just keep it automated. When that happens, what ended up happening is the hotel had worse outcomes. Like there were homeless people hanging around. The worst part, you know, customer satisfaction was down, etc.
Devansh Devansh [00:43:32]: And what you end up realizing is that the doorman's stated job is to hold open doors and not do doors. But what the doorman also does is greets you. Well, maybe you don't care that Much for that. But it's also a matter of you. I come in with a taxi. I've just flown in from a different country. I have big suitcases. Oh, the doorman's going to help me out.
Devansh Devansh [00:43:56]: The doorman standing there prevents, like, vagrants from coming in and, you know, if they're not guests, etcetera, Hanging around the lobbies and whatnot. You have all of these other, like, not said benefits or here's a great example. This one time I was biking through New York and I dropped my phone. There's a true story. And somebody picked up my phone and left because they thought, oh, I don't want somebody else to steal it. But what this means is that I no longer have my phone. And I just moved into the city, so how am I going to get back home?
Demetrios [00:44:31]: Yeah.
Devansh Devansh [00:44:32]: Oh, no. And what helped me was a hotel doorman because I'm an Uber kid. I think I've definitely taken more Ubers in a year than I have taken yellow taxis my whole life. So he actually flagged down one of their, like, yellow page taxis or whatnot for me, and I got to go home now. I wasn't even living at that hotel. But that kind of stuff is not something an automatic door does for me. An automatic door opens and closes. So I think that's where it's a great case study for both replacing human labor, but also for thinking about when you're talking about these verticalized agents, when you talking about these verticalized platforms, like, people don't realize how much other stuff people do that has nothing to do with their job that ends up being marginally useful here and there.
Devansh Devansh [00:45:37]: You know, you mentioned scrolling on social media as an example. I will occasionally find stuff on threads or Twitter or whatnot that's worth sharing with my research lab team. I will occasionally find solutions. So that kind of. That kind of information. I mean, I found Grutto by scrolling, not by. As a company, but synthetic data. I was scrolling through.
Devansh Devansh [00:46:05]: I found some discussions on the replication bias on AI, and that led to me finding this paper that was talking about, hey, we're struggling with, like, with healthcare data you're not able to send across borders, so we can't replicate a lot of experiments, which is why we just chose to do synthetic healthcare data instead of real healthcare data. Because now I can just publish my whole data set and call it a day. And that was the whole reason I started monitoring synthetic data around 20, 20, 2021 in the first place. So, like, that kind of stuff, if you were trying to replace me. You could say this is not useful stuff. And if you were studying AI, then yes, technically 99% of what I would have scrolled through would be like MMA reels or cats or fight football or whatnot. But that 1% had an outsized impact but would be very hard to quantify and very hard to account for. So that kind of stuff I think becomes very, very useful.
Devansh Devansh [00:47:07]: Or like Jiu Jitsu. I like, I might, I threw a random off comment there about Jiu Jitsu on my, in one of my articles and then turns out one of my readers really likes Jiu Jitsu. So we connected on that and we ended up doing something together. Very, very cool, nice projects together. Now one of the interesting things there is AI actually doesn't like the way I write. It's told me on many occasions that because I, I tend to use a lot of references and very off the cuff tangents and things like mentioning Jiu Jitsu, not as a analogy, not like as an intelligent analogy, but like, oh yeah, I do this and I don't know, I was talking to somebody here and going into these slightly off topic tangents that AI is like, hey, clean this up. I think because your work is already long, like type in this, condense this. A professional audience would not like your work, especially Claude.
Devansh Devansh [00:48:09]: Claude hates my writing. Jesus. So I think that kind of integration becomes, or that kind of verticalization becomes very, very difficult. So if you were building any AI systems or any tech systems, any automations, I think it's always worth thinking about that A lot of the time. We as AI engineers aren't doormen. We as AI engineers aren't HR assistants and we've probably not studied that process in depth. Not saying that they can't be replaced. I'd be the first person campaigning to kick out every HR person and have them do something actually meaningful to society.
Devansh Devansh [00:48:56]: But like there's also like a reason these things exist and there's a reason like the like humans labor has a lot of random behaviors that you might not be able to quantify or touch for. That does a lot of good. That has a lot of impact in the actual work. So I think it's just a good case study to think about.
Demetrios [00:49:24]: Dude, this doorbell case study is wild because it's almost the second or third order effects of implementing a system that at face value is much better. You save costs because now you don't have to pay the salary for someone who's holding the door. And I think about it and extrapolate it towards Any type of AI system. And I, I know I, I tend to talk a lot about marketing copy being generated with AI. I've seen some very advanced systems of AI generated blog posts and you can churn out a ton of posts with AI. But it makes me question now, potentially what you're getting. You're getting really good SEO rankings because you're now churning out a ton of highly optimized SEO keywords, all that good stuff. And so you're shooting up in the rankings and you're getting a lot more traffic.
Demetrios [00:50:37]: But you also want to think through is this traffic, reading this, recognizing that it isn't that valuable and it's hurting my brand because that could be a potential outcome. If you're just churning out a bunch of AI slop, or in the case of hr and you have some kind of a pipeline and it's for hiring, but your potential candidates don't get to actually talk to a human until they've done three or five things. They've jumped through all these hoops that are very much graded by AI. Is that giving the best experience to this future teammate? Right. Like, what kind of culture are you building there? And maybe down the line you're optimizing for someone that is not going to be the best fit. Or there's. Yeah, it's, it's really. There's probably a name for this, but the second or third order effects are huge.
Devansh Devansh [00:51:51]: I mean, that is exactly what. Yeah, you said it so well, is second, third order impacts and where you get it wrong. And I think that's where, I mean, I feel like a lot of tech people would benefit from thinking not about tech, but about other aspects in their life. Like, why does a romantic dinner sound romantic in the first place? Most of it is psyop marketing bullshit, don't get me wrong. But like walking into like a place with ambiance, with a candlelight or whatever that does do, like, there's a reason those things have certain impacts that we associate. You know, one of the things is the more simplistically you look at a problem, a situation, an outcome, the more you're likely to miss critical pieces that end up becoming, that end up unraveling it. And I think that's where, I mean, a lot of our legal tech competitors went wrong. This is where a lot of stuff I've seen in general tech gets wrong very often is you'd look at you look at, you boil a whole problem down into a very, very narrow domain or a very, very narrow subtask, and it Turns out that that subtask isn't super duper important to begin with.
Devansh Devansh [00:53:16]: So I just think it's worth thinking about the Dorbert fallacy things like that. These are genuinely things that debunked. If you don't think about them you'll just end up building products that are useless to society. AI based customer service is a good example where if you're not doing it correctly what ends up happening is you'll just have worse outcomes because I just want to call you up and ask you about my stuff and like there's no way for me to get that hey the customer service should be replaced. I'm sure like there is a lot of good outcomes there. You can, where you can speed it up. But you know if you try to replace everything with a voice bot and a dial this on your phone and do that I think you're, you might be missing very key elements of what is it that makes people happy or what is it that people want from you. And you kind of implicitly filter for people who like the kind of loyalty you'll build is towards people who want, who want to maintain a certain like cost becomes their main priority which means they'll cut you out if you become too expensive as opposed to offering a little bit more having that luxury and prestige and or not associated with it and providing that requisite services.
Devansh Devansh [00:54:43]: I pay a higher premium on my credit card and higher fees and whatnot. Like if I were overdraft just because my credit card guys have guaranteed me 247 immediate support. Any country I go to like I, I pay you an extra, you know few hotel for that and risk potential. Lot more on plot twist though.
Demetrios [00:55:11]: It's AI support and it's not human support.
Devansh Devansh [00:55:15]: If that happens I will be so mad. I'm sure talk to the cop in another country and say hey, please hold this ring call. Press 2 if you want to talk this but oh man.
Demetrios [00:55:28]: Well hopefully it doesn't ever get to that point where you have to use it. But I, I, I understand the sentiment of we need to for certain things we look at how we are. How can I say this? It's like we're, we're voting with our dollars and we're voting and inherently saying what is important to us as customers and the there's as a builder a razor thin edge that you have to walk on. If I automate this or if I bring automation into this, be it AI or not, is that going to create a worse experience or is this going to affect my business Metrics in any way, for the better or for the worse. And that's something that I think continuously, folks ask about, because you want to be able to show and justify an impact that you're making as a developer, as someone who is implementing AI into production. So you want to figure out what metrics you care about and the company cares about, and then how can you hopefully make those metrics move? Now, what you also want to recognize is, are you making those metrics move in the wrong direction, or are there other metrics that the business cares about that are being affected that you didn't even recognize? And so this brings in. It just makes me think like, wow, you really have to be playing 3D chess and you have to be recognizing all these different outcomes. And it probably just starts by you being a user or you trying to game it out in your head and recognize where and how the old way has its benefits versus the way that you're trying to implement and what benefits that has and really recognizing what the past benefits are with some.
Demetrios [00:57:39]: A doorman, for example. Why is that beneficial or an actual human that you talk to and what's good and bad about that? Because we all have had experiences where we talk to a human and we get passed around to three different departments and each time we have to explain our case. And so even though it is a human, it still is a really shitty product. So thinking about all those different ways that you want to make the product better is. It's fascinating. And now, like, I'm just going to probably be ruminating on this for the next week or two again with these thought experiments that you're giving me. This is like a lot of shower thoughts that I'm going to be having. I'm sure of it.
Devansh Devansh [00:58:27]: Oh, that's how I pretend to be smarter than I am, is I. Just before this meeting, I was coming up like 20 random hypotheticals so that you don't have to ask me on anything technical. And 70 years, people are thinking, who is this guy Demetrius is talking to? I don't think he could be here.
Demetrios [00:58:44]: Oh, what Sweet dude. Is there anything else you want to hit on before we. We cut it?
Devansh Devansh [00:58:51]: I think a talking point I try to emphasize a lot through my work, but I think it's always worth thinking about. I don't think AI is as solved as people think it is. And what that means is that it's a very open field and people aren't being ambitious enough with that idea. You know, there's a lot of open space in AI where You can come in, really screw up existing power structures, kind of throw your, throw a vengeance in the world and come and take over. And I think more people should really come to recognize that yes, artificial intelligence is a field of study, has existed for many years, but AI as an institution in society and an influential group and how it's impacting it at a much more active level is relatively new. And what that means is that you don't necessarily have to be a math guy like me or a software engineer or something to make an impact. There's like a lot of, there's just a lot of open space where you can come in and shake things up. So if there's anything I would want people to think about and work on actively is just, hey, am I, am I really doing things to shake things up or am I just doing things to get by and get, you know, am I, am I trying to become a Google or am I trying to be picked by a Google? And I'm not saying that there's something wrong with the latter.
Devansh Devansh [01:00:34]: Again, if you have other priorities, you're content with your life. You know, I'm not going to say sell your wife and kids and focus on, you know, whatever you have to, but I think so many people that I speak to are just kind of implicitly buying into narratives where there are experts and there are non experts, there are, there are, you know, there's an other people from them where that will actually do things. But as, and they kind of become, end up becoming participants and they become viewers of society as opposed to participants. So I would just say like, if you're going to make that decision, make it consciously as opposed to just kind of making it because you've never thought about the alternative.