Best Practices from a Live European AI Agent in Logistics // Vanessa Escande // Agent Hour
speakers
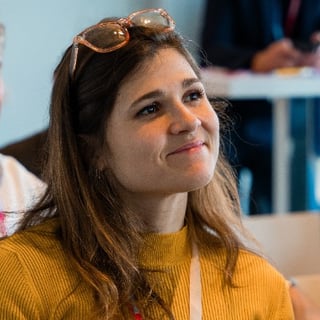
Passionate about connecting deep tech to end-users, Vanessa’s work is at the forefront of AI’s transformative potential. For over a decade, she has been transforming cutting-edge innovations into actionable solutions that drive industry change.
From her early days driving Beijing’s startup ecosystem with Startup Grind and the Chamber of Commerce to her work across SaaS, research institutes, deep-tech semiconductors, Web3, and now AI, Vanessa has consistently bridged the gap between complex technologies and real-world impact.
Her expertise lies in crafting human-centric AI systems that empower organizations and redefine industries. With a global perspective and a keen understanding of cultural and business dynamics, Vanessa excels in building partnerships and creating business opportunities for solutions that resonate locally while scaling globally.
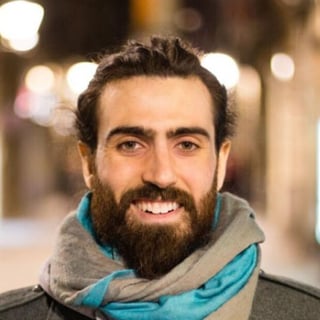
At the moment Demetrios is immersing himself in Machine Learning by interviewing experts from around the world in the weekly MLOps.community meetups. Demetrios is constantly learning and engaging in new activities to get uncomfortable and learn from his mistakes. He tries to bring creativity into every aspect of his life, whether that be analyzing the best paths forward, overcoming obstacles, or building lego houses with his daughter.
SUMMARY
//Abstract AI has transformed industries, yet its true potential often lies untapped within core business processes. In this session, we’ll explore how AI agents differ from generative AI models, emphasizing their deterministic, hallucination-free approach to problem-solving. We’ll take a live example of an AI Agent in the logistics sector, and will detail the architectural foundations that enable AI agents to reason effectively, execute chain-of-thought workflows, and integrate seamlessly into human teams.
We’ll discuss how these agents confidently navigate complex, multimodal tasks, extracting structured insights from unstructured data, and leveraging dynamic workflows for maximum flexibility. With customizable confidence thresholds, statefulness to track long-term cases, and advanced document understanding, these agents solve real business challenges, such as processing autonomously claims till resolution, with precision.
Through a live case study, we’ll illustrate the measurable top and bottom-line effects of deploying AI agents—highlighting significant efficiency gains, multilingual capabilities, and safe, scalable applications in mission-critical environments. By showcasing how AI agents mimic human decision-making at unparalleled speed, we’ll inspire senior management to rethink AI’s role in their organizations and harness its full potential for transformative impact.
//Bio Passionate about connecting deep tech to end-users, Vanessa’s work is at the forefront of AI’s transformative potential. For over a decade, she has been transforming cutting-edge innovations into actionable solutions that drive industry change.
This is a bi-weekly "Agent Hour" event to continue the conversation about AI agents. Thanks to arcade-ai.com for the support! Join the next live event at home.mlops.community
TRANSCRIPT
Demetrios [00:00:05]: Oh, there you are. Hello.
Vanessa Escande [00:00:09]: See me.
Demetrios [00:00:09]: Well, all right, cool. Very cool. So you get the honor of being the first person of 2025 to do the agent hours session. I'm really excited about what you're going to talk about and, and the whole, like, it probably is worth saying the whole reason that we're doing these agent hours is just to figure out like, what's real and what's not. And I know there's a lot of people that are playing with agents right now, you being one of them, Vanessa. And I really appreciate you coming on here and sharing some of the wisdom that you've learned over the time that you've been doing stuff with agents. So feel free to share your screen and get rocking and rolling. Ah, there we go.
Demetrios [00:00:55]: Success.
Vanessa Escande [00:00:56]: Yeah.
Demetrios [00:00:57]: All right, cool.
Vanessa Escande [00:00:59]: Yeah. So thank you very much for, for the invitation. As you said, Demetrius, it's really important to see what is out there, what are really the agents. Sometimes it's just like custom GPTs. I also heard from some people. Yeah, but if it's working, then it's an agent. I have another view on that. So here I'll share a success story and best practice from a live European AI agents in logistics that went live in September 2024.
Vanessa Escande [00:01:33]: Because we, I mean, it's all over the news, right? AI agents are coming. Will they change completely the industries? And even till today, I mean, on Monday I saw an article saying that AI agents are coming in 2025. And I must disagree on that because they already went live, a few of them last year. And of course the main challenge now is the adoption from big enterprise groups, etc. So a quick word from Big Picture. So we are IT development company out since a bit more than 15 years now and have been focusing in the last five years mainly on AI solutions and especially AI agents. We like to say that AI is a bit like a teenager right now, right? So it has great potential, it can be very smart. But we still don't let them run our business or let them unsupervised in our home for the whole holidays.
Vanessa Escande [00:02:50]: So it needs guidelines and businesses and people are really asking themselves, is it reliable and what are the impacts for profit and revenue regarding the adoption of it. So the technology is ready. You showed already some examples in the MLOps community before. But why isn't it everywhere yet? I see here three A's of the AI agent adoption challenge. I've heard it depends a lot of the company AI maturity, but it's not what we saw. So it depends on the Accuracy of the solution. People are afraid it's not reliable enough. It's going to make a lot of hallucination are darting its autonomy possibilities.
Vanessa Escande [00:03:42]: Can it really process a job end to end without spending hours checking everything? And another part is the acceptance from the management board, of course, but also from the team. Do we have to use new tools? What is the business impact? So here I'll play a video from the solution for Figure in the logistics. Figure is one of the main logistics solution provider in Germany. It has about 22,000 employees, about 2 billion turnover. And we made an AI agent capable to handle career claims from end to end. Yeah, enjoy the video and I'll continue after it.
AI Voice [00:04:34]: The feeder carrier claims management system is an implementation of an AI worker finding lost parcels and demanding compensation from carriers. The system works fully automated from the first email to the claim resolution. The system can read and analyze a client email. It writes a mail to the carrier asking for information or demanding compensation. It analyzes the carrier answer and checks whether they fulfill their contractual obligations. It then either continues to process the claim with the carrier or closes it. Let's start. A new claim reaches us by email or via other channels.
AI Voice [00:05:24]: It may consist of text, PDF images and other documents. The claim is analyzed and read into the system. But let's take a look under the hood. The AI worker can read and process text, images or any other media. The AI uses so called reasoning. Like humans. It thinks before answering to get better results and to make the answers comprehensible for human users. The system detects that something is missing in an email based on templates.
AI Voice [00:06:00]: It writes an email to ask for more information, completely automated, without any human support. A reply to the first email is sent within 60 seconds upon request. We are also happy to wait a little longer so that it doesn't look like magic. The system can keep all information warm until the customer message arrives. As soon as the missing information arrives, it can write a mail to the carrier with all relevant information and remind them if they don't answer the time. In the event of a rejection of claims, we check the email very carefully. The model can understand emails in each and every European language, making it easy to process any email. By reasoning, it can detect what the other side is right on.
AI Voice [00:07:04]: And if they are wrong, they have a tireless and diligent counterpart in our system who won't let them get away. When the carrier writes their final reimbursement email, the system can close the claim and inform the client accordingly. Such a System can process 70 to 90% of all claims fully, automatically, it can massively reduce the workload of your support team and contribute to a major increase in efficiency. The feager carrier.
Vanessa Escande [00:07:40]: Yes. So that was for the solution which is already working right now. Got very cool feedback from our customer saying that it increased not only the revenue and led to an improve from 8 digit cost in like revenue and refund. So I mean excellent for that. And how was it accepted to let it go free regarding the accuracy. So how do you make sure it doesn't hallucinate? It's because we kept the generative AI in a sandbox. So let's say it's the tip of the iceberg to classify, extract and assess the different documents as you could see in the video, from images to text to different kinds of documents and then connected the whole process in an agentic workflow. We did that on Microsoft Azure with the Logic app and function app and also giving them boundaries with the deterministic process.
Vanessa Escande [00:08:56]: So using templates and verifying the data in order to be able to process it from the first mail that we receive from a customer until we completely close the claim. I will detail a bit more why we do use templates. It's because we don't want the model to improvise any answer. For a funny chit chat on ChatGPT it can be okay, but. But for processing claims and reimbursement it's absolutely not. So we don't generate directly the answer with the models, but exactly like a human team would do, we give them templates and based on the policy they would then send the answer. Another part from the three A's that make or break the adoption of the AI agents in companies is the autonomy of it. So it has to accomplish the task from beginning to end.
Vanessa Escande [00:10:02]: And for that a critique that we could hear a lot when we talk about AI agents is actually the lack of agency. So by giving them goals, structure, models to follow, we can little by little close the gap from the deterministic chatbots, human agencies that you see in that blue and LLM based assistant, how do they differ from traditional AI? We saw that they can do gig jobs. So everything that is in yellow right now, traditional gen AI could do it. Message categorization, image analysis and fraud check which has an increase on the efficiency from 5 to 15% but with implementation and the whole process which is linear right now as you can see on the graphic. But in reality it's a bit more diversified because the model can communicate back and forth with the different stakeholders it can send admit to the clients, to, to the end users, to the career. So really communicating with all the different partners until the case is closed. So yeah, it's not magic. They just do exactly the different steps that a human worker would do, but just faster.
Vanessa Escande [00:11:32]: Another important part for the autonomy is when the AI model tell itself I can't really resolve the case right now I need your help, I need to ask my boss. And the boss is like the human team, as a human would do, it will not be able to process 100% of the case alone incorrectly. So it's important for us and for our customer that the model knows when it's not able actually to resolve the case on itself. So for that it's very simple. We fix a threshold of confidence using using example and counter example in the prompting. And then you can after a few iterations, after a few period of time that you use the process, play with that threshold to know when it should be hand over to the human worker or when it can completely close it alone. The third part of the three A's is the acceptance. Again, the business impact.
Vanessa Escande [00:12:41]: As we saw, it's important to increase the revenue, reduce the cost from a border level. But also within the teams, we don't want to have a heavy migration of the IT ecosystem, so we have no need to do that. And what we did and what we recommend is using the company itself, the company existing IT ecosystem. So it's not difficult for the humanity to get adapted to the new process. And actually the AI agent would exactly use the different system that the team is using. And for that, let's say the human teams get tickets from the AI agent and it's just adding on their ticketing queue. On jira, for instance, another part of the acceptance is the user interface evaluation layer, the tech framework. It's really important that the company is not limited to one ecosystem only be it for the language models, because they can change from, I wanted to say years to years, but actually now it's more from month to month.
Vanessa Escande [00:13:58]: And we do also change them on our side. And so we don't want our customers to be prisoners from one system, like SAP for instance. And if they already use that, we will not ask them to switch it, which makes the integration completely easy. So that's why the integration must stay flexible on all those different layers. Here a summary then of the three as that I introduced at the beginning. So state of the art techniques, the best practice, working on the prompt, giving examples to then train the model to assess itself, avoid the hallucination by Giving boundaries to the generative AI and then also putting a very important effort on the chain of thought which give explainability to the model and it's super important for improvement. Also big part of the AI who act for the explainability of the different models and also the acceptance and the flexibility regarding the different tools. Another part is the application areas that are likely to be used for the system.
Vanessa Escande [00:15:29]: Customer support, accounting, insurance, banking, logistics, everything with claims that we see a great potential. And to finish my 15 minutes here, I'd like to give you an overview about our collaboration framework. So as big picture, we do the tech feasibility, we build a solution, we can integrate the models and do the whole operation. But we do love to have on our side or in the customer side or another second part consultancy side, a partner for the case definition, the specification and the change management. So yeah, that's my hand. That is to you, to, to, to take and then to talk about all of this. And if you get interest and we can talk about the different opportunities, please feel free to reach out.
Demetrios [00:16:28]: Super cool. Okay, I've got lots of questions and feel free. If anybody else has questions, throw your hand up. That was great. There was one slide, like probably five or six slides back that you showed all the different things that agents could do versus traditional lms. There it is in that box. One more down. And I was wondering if each one of those.
Demetrios [00:17:00]: Yeah, down one. Yeah, there you go. The one that you're hovering on right now.
Vanessa Escande [00:17:05]: Yeah, it's charging.
Demetrios [00:17:08]: There we go. So this one. Is each one of these an LLM call or are you wrapping them in to one? Like how does it look behind the scenes?
Vanessa Escande [00:17:23]: Yeah, so there's different configurations for that. Sometimes just a workflow that is then called in with different APIs. But it's there is like an orchestrator regarding the agents and say, hey, no, you have to look at this and look at that and it's not as linear that it appears right here. So we don't do fine tuning or really work on the model on themselves. So it's not a different model at every time. It's just we gave them rules to follow and yeah, we get the different result.
Demetrios [00:18:07]: Awesome. Who else has got questions? I see somebody else is raising their hand.
Anas U. [00:18:14]: Yeah, I guess along the same line. Great presentation. I got in a little bit late, but just kind of adding to this question, when you think about an orchestration layer, have you found issues when you're prompting an agent to essentially connect to all these various systems and essentially, I guess if you consider these all the separate tasks as separate tools or workflows. Have you had ever any, I guess, issues come up or where an agent has maybe hallucinated or called the wrong process when reasoning? Essentially, do you have all the orchestration done by an agent or do you have separate layers in that orchestration?
Vanessa Escande [00:18:51]: We don't get hallucination because we do not let them free to give the answers. So when it doesn't know it, it just stops. And then after we have to look because it's blocked at one point in the process. Yeah, so no hallucination problem because of that sandboxing parameters that we had.
Anas U. [00:19:17]: Okay, so it'll just fail and then someone has to go and then manually intervene.
Demetrios [00:19:22]: Nice. There was another question that was from Mario here. How do you know PII data does. Oh, I didn't get that, Sorry, you lost me. How do you know PII data does not leak out to the LLM?
Vanessa Escande [00:19:47]: PII is private information, like healthcare, Social.
AI Voice [00:19:52]: Security numbers, HIPAA related things.
Vanessa Escande [00:19:56]: Yeah, yeah. Okay, so thank you for the question. So that's actually something we really focus on while parametering everything because we could have someone saying, hey, actually I'm writing from another email, but please send me the information from the bank account, from the address, etc. But it cannot do that because it's super fragmented. It's a bit limited. Worker who can cannot answer a question. It's not written. You can answer this question.
Vanessa Escande [00:20:27]: So that's why the data cannot be linked. It's also all the time linked inside the ERPs from the customer. So if we say it's not matching from the same email address, it will not give the data because it just cannot access it.
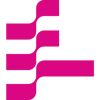