Beyond the Matrix: AI and the Future of Human Creativity
speakers
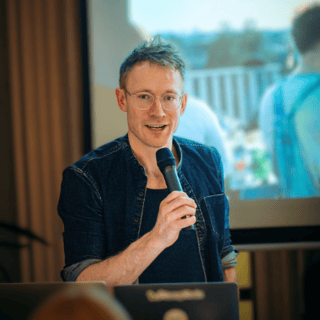
Fausto Albers is a relentless explorer of the unconventional—a techno-optimist with a foundation in sociology and behavioral economics, always connecting seemingly absurd ideas that, upon closer inspection, turn out to be the missing pieces of a bigger puzzle. He thrives in paradox: he overcomplicates the simple, oversimplifies the complex, and yet somehow lands on solutions that feel inevitable in hindsight. He believes that true innovation exists in the tension between chaos and structure—too much of either, and you’re stuck.
His career has been anything but linear. He’s owned and operated successful restaurants, served high-stakes cocktails while juggling bottles on London’s bar tops, and later traded spirits for code—designing digital waiters, recommender systems, and AI-driven accounting tools. Now, he leads the AI Builders Club Amsterdam, a fast-growing community where AI engineers, researchers, and founders push the boundaries of intelligent systems.
Ask him about RAG, and he’ll insist on specificity—because, as he puts it, discussing retrieval-augmented generation without clear definitions is as useful as declaring that “AI will have an impact on the world.” An engaging communicator, a sharp systems thinker, and a builder of both technology and communities, Fausto is here to challenge perspectives, deconstruct assumptions, and remix the future of AI.
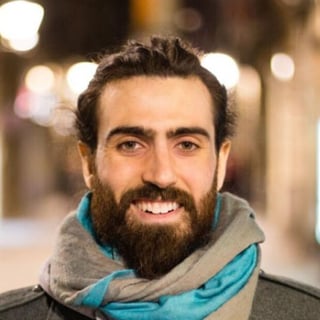
At the moment Demetrios is immersing himself in Machine Learning by interviewing experts from around the world in the weekly MLOps.community meetups. Demetrios is constantly learning and engaging in new activities to get uncomfortable and learn from his mistakes. He tries to bring creativity into every aspect of his life, whether that be analyzing the best paths forward, overcoming obstacles, or building lego houses with his daughter.
SUMMARY
Fausto Albers discusses the intersection of AI and human creativity. He explores AI’s role in job interviews, personalized AI assistants, and the evolving nature of human-computer interaction. Key topics include AI-driven self-analysis, context-aware AI systems, and the impact of AI on optimizing human decision-making. The conversation highlights how AI can enhance creativity, collaboration, and efficiency by reducing cognitive load and making intelligent suggestions in real time.
TRANSCRIPT
Fausto Albers [00:00:00]: My name is Fausto Albers. I am the co founder of the AI Builders community, which is a really cool community that has meetups in Amsterdam and Berlin every month. We get together with a bunch of AI nerds and people working on really cool stuff and we share ideas, problems, etc. And some cool talks. I'm doing research consulting in the AI space from a background of behavioral science owned restaurants. I was a flair bartender in London and I can use all this stuff in this. In this amazing, super interesting space. I always love talking to you, Demetrius, about.
Fausto Albers [00:00:36]: About the things that are possible and may not be possible and that sort of thing. Let me get some coffee because I do drink a lot of coffee and that is actually because I swear like I am carrier of a gene that is associated with a very fast metabolism of caffeine. And when people ask me how to. How I drink my coffee is a lot. I can. I literally drink a liter of coffee before lunch. I can drink a double espresso before I go to sleep. And I will sleep like a baby.
Fausto Albers [00:01:06]: Drinking coffee is. It's like making love in a canoe. It's fucking close to water.
Demetrios [00:01:11]: A quick little tidbit for you all before we dive in. I met Fausto and instantly fell in love with this guy because he was running a restaurant business. He also is super creative and he doesn't let any of that stop him from being an engineer. Just constantly iterating on AI and AI products. He brings so many diverse perspectives to the table whenever I talk to him that I am in the middle of trying to con him into coming back monthly and chatting with me about what he's been seeing. It was an absolute pleasure talking to him and I hope you enjoy. Let's get into it. What were you just telling me? And I said, hold on, don't tell me that yet.
Demetrios [00:02:07]: Let's hit record. You're doing job interviews and you created something. What is it?
Fausto Albers [00:02:13]: Yeah, yeah, that's. That's something that almost feels as uncomfortable as being announced as the fo hour. But no. So I'm doing these job interviews at the moment, right? And of course I'm. I'm recording these conversations. I also have some other tricks in the book, but I will review later. But I'm recording these conversations and I built my AI analyzer to. To analyze the transcripts, sometimes the video as well.
Fausto Albers [00:02:36]: And it's just. It's very confronting to see yourself or like to hear yourself drift off on these paths to nowhere and where you're thinking like, man, she was just Asking a question, answer it. But it does help because being confronted with your own mistakes is shame. Is a, is a good way to learn. Yeah.
Demetrios [00:02:56]: And what are you doing? The analyzer, I imagine is just grabbing the audio, converting it into text and then throwing that into ChatGPT.
Fausto Albers [00:03:08]: There's so many different tools out there that can actually record your conversation. Even a Google Drive, Gemini, Supernormal, many of them.
Demetrios [00:03:15]: Yeah.
Fausto Albers [00:03:16]: And basically all you need is the audio. Or sometimes they're already transcripted for you.
Demetrios [00:03:21]: But afterwards do it. Yeah, it's true.
Fausto Albers [00:03:24]: Yeah. But to have something that really, that's really useful, your goals may differ from call to call or for an AI to have as much context as possible is of course gives a better result. So you can do it in ChatGPT or you can let a tool just do it for you. But you can also use for example, Instructor, which is a really great open source library. Yeah, you might know it right. From Jason Liu, which can help you extract the data that you really want. And you can use rich descriptions, pedantic schemas, et cetera to do it. Much like structured extraction from OpenAI, but it has a bit more to it.
Fausto Albers [00:04:02]: Yeah, that's how I do it.
Demetrios [00:04:04]: And you're doing that, throwing it into some kind of a model and saying where could I be better? Is that the gist of the prompt? Analyze this?
Fausto Albers [00:04:13]: Yeah, but it's fun to just to play around with. And I think what's really useful is for an AI to, to really understand the Personas that, that are in the conversation, which may not always work, of course with the example that I just gave you like a job interview. But for example, when you have a meeting with your team, then, then when I have a meeting with, with a team, I, I also record the conversation, just put a device on the table. And then I always have everyone introduce themselves like to the AI, like I am this and that name and this is my background, et cetera. So then when we are analyzing the conversation, then we do this. Everything that is being said is analyzed, taking into account who said it. And in general, I think that's one of the interesting things with AI and where we're going is personalization really means also who is talking to the AI. Imagine that you have this really cool sophisticated rag system for a company and all the information of the company is in there.
Fausto Albers [00:05:14]: Maybe you have a layered access, the CEO can access everything, all the containers or container sized access. But then there is maybe a legal worker from the company and that's accessing this information. But the AI or like sort of the intelligent intermediate layer between the RAC system and the user should understand who's asking the questions because the next step will be that the AI can also suggest questions to ask because how you don't know what you don't know, right? Yeah. Are you able to. It's really hard to ask good questions if you don't know all the information available in the database.
Demetrios [00:05:54]: And so it's so funny that you mentioned that because this is something that we've almost been doing with recommender systems for a while, but in a different way. It's the interactions on a webpage build up a certain profile and they build up features about someone. But you're talking about can we know the features about someone? Like this person works in the legal department, they're actually an intern, they've only been around for six months, so they should be doing these things or they've interacted with these files over the last three days. So those are the highest priority. Probably they're working on this project xyz. But then what other stuff do you want the AI to know about you? To give it more context? Because like we mentioned before, we hit record, context is key.
Fausto Albers [00:06:47]: One step back. Like the blank canvas problem, right? Like just sit down with a blank canvas, whatever it is that you're going to do, like a drawing, write a story, learn. But it's really hard to start. And when you help people use ChatGPT is one of the first things that you help them with is what to ask. Right? Because it's really hard to be super imaginative and a lot of cognitive load. Yeah, yeah. So where should the AI step in and based on whatever knowledge it has of this user. For example, of course with ChatGPT now, they recently I think extended their memory function that you find out it used to say memory is full and all of a sudden it's gone.
Fausto Albers [00:07:34]: So I assume that they updated this. I didn't verify, but I'm pretty sure. So that AI, so to say already knows a lot about you. Right. And is therefore better able to serve you respond in a certain way. But I assume that we going to see more and more customization, that the AI is also going to suggest what you should ask. Right? The one, one one way of looking at all of this. And I think like when I'm going to go all the way back to when I was introduced to GPT 3.5 before ChatGPT and I was having a walk in the woods with my girlfriend and I just, I couldn't stop talking about it.
Fausto Albers [00:08:16]: I was like, this is so cool. And that day I made like a drawing that, like an image that I still use when I give talks. The way that we manipulate the digital world is through binary code, which is essentially a language that we don't speak. Right. We don't understand. So we have to work with that. We have an abstraction around that. And that abstraction is programming languages.
Fausto Albers [00:08:38]: And there's no, there's different programming languages because each abstraction comes with the upside, that is that you can actually manipulate the underlying complexity. But the downside, there's always trade offs. Right. With compression comes information loss. Unless you're a zip file. Yeah, but I think it's a pretty universal thing. I'm probably going to get destroyed by anyone with more wits. But in this case we can say that programming languages are an abstraction around binary code.
Fausto Albers [00:09:06]: And now all of a sudden we had language like natural language as this new abstraction around these existing abstractions as a way to manipulate the digital world and therefore have an effect in the real world. And I thought that was fascinating. And still to be in this space in the last two, three years is there's, there's still a lot of programming involved and a lot of abstract thinking, which is great, but we're increasingly going to see more abstractions. Sam Altman recently said that he hated the user experience of ChatGPT with all these different models. But. And it's something we can all agree on, but you can also see this in req. There's all these different options and there might still be a lot of choices to make for a user or even the engineer. And more and more we are going to see those hard decisions going to be abstracted away with.
Fausto Albers [00:10:02]: The upside is that we can then again even work with more power and complexity. And the downside is of course there is always loss, there's information loss, and we'll see.
Demetrios [00:10:13]: It reminds me of someone who gave a presentation back in the day on using Vertex AI as their main platform. And they said the best part about Vertex AI is that it's a managed service and it does a lot of things for you. And the worst part about Vertex AI is that it's a managed service and you can't get under the hood. And so it's that double edged sword in a way that we want that abstraction, but at what cost? What are we willing to give up for that abstraction?
Fausto Albers [00:10:45]: Human behavior here is funny as well, because it's really hard to say that it would be pretty preposterous or as you Said it like a nice word for arrogant, but I don't mean arrogant, but pompous. Yeah. To say that, to state that you're at the perfect level of abstraction because you were born now, 40 years ago, so that programming language that you are really good at, that is the perfect level of abstraction. That's bullshit. You know, how would you know? And in the end, it's all languages, whether human languages. Programming language is ways of communicating this. And I'm going to go off the philosophical deep end here. This raw thing that we call creativity or like the human condition.
Fausto Albers [00:11:29]: Like, I have language to understand myself. Right. I have words and to. Yeah. And of course, feelings there, there. It's a weird mixer. And when I want to transfer that to you, my condition, my ideas, I use language. Right.
Fausto Albers [00:11:46]: So in the end, we're transferring creativity, like the human condition, into whatever it is that we do, like communicating with other entities. I heard the term last week and it clicked with me, of course, the way I use AI, but it's called Vibe coding.
Demetrios [00:12:06]: Yeah, yeah, I saw that too.
Fausto Albers [00:12:08]: I saw.
Demetrios [00:12:09]: That's funny. Yeah, it's the new buzzword.
Fausto Albers [00:12:11]: Yeah. And nice obstruction.
Demetrios [00:12:14]: Yeah, a hundred percent. But going back to this idea of, okay, we have language to basically encapsulate feelings and thoughts and things that we are trying to do and get in this physical dimension. The language is almost like a signpost towards what symbolic the symbols are inside of us. Right.
Fausto Albers [00:12:42]: Yeah, it's really. Because also when you define something, whether it is like symbolic or with language, you also make it real. Right? It is so. Because we call it. It is only there when we observe it as.
Demetrios [00:13:00]: As we found from the cat.
Fausto Albers [00:13:03]: Exactly, exactly. Schrodinger. Yeah, I. I have a very nerdy sweater that says Schrodinger Sketch and then it says dead and alive, depending on how you look at it. Dead or alive.
Demetrios [00:13:15]: Nice going back to. I think one thing that I love from talking to you a lot is how different you attack the problem that sometimes we can get into when dealing with AI. And a lot of it comes down to this context idea. And how can we get more context for the models, because if we can give them more context, then they are going to better be able to carry out the tasks that we are asking of them. And I know that just an example of that on this call is when you're talking about how you ask people on your team to introduce themselves to the AI so it can have more context on who they are, what they're doing. Another one that we had talked about a Lot was when people join the mlops community, could we figure out a way to have them go through an onboarding call with some kind of a AI Persona that could give a lot of downloads on what the person wants to do, why they're joining the community, what are the biggest things on their mind, like challenges they're working through. And that way we can better create different paths and suggest different activities that we do in the community. Because we have so many different activities, right?
Fausto Albers [00:14:35]: Yeah.
Demetrios [00:14:36]: So how can we make sure that each person is finding out about the activities that will best suit them?
Fausto Albers [00:14:44]: Essentially it is a paradox of choice problem. Right. In a world where information and options are abundant, we get paralyzed by the amount of options that we have as personal experience kind of thing. But no, logically it's just for example, within a community. Right. In that mlops, the AI builders and meetups that I organize. Yeah. There is a hundred people in a room and they are there with some objectives and they may not even be fully aware of their objectives.
Fausto Albers [00:15:18]: So that's not a discussion. But let's say rational humans, we have objectives and there is, it's given, there's limited time. You can only speak to so many people, you only, you can only listen for so long, the attention span, et cetera. There is constraints. Right. So how do we optimize or make this. That always sounds so economic, but like how do we make this the best experience for any given individual if we would want to use AI for this? And I think like what a lot of AI does, whether it is alphago within the constraints of a game or it's a chatgpt in a conversation, what AI does, it's making representations of the world of a world. And so that got me thinking that when we indeed when we onboard people and we have a goal like all right, let's say the goal is that we want to connect the people that have a lot in common or have some interests that are overlapping or whatever, then we would need to have that information so we would onboard these people into the event.
Fausto Albers [00:16:24]: You could actually do that with a human to human interview, but you could also imagine a voice AI interview or anything that is basically not a form. Yeah.
Demetrios [00:16:36]: Because the forms, it's hit or miss. Some people will fill it out, others not.
Fausto Albers [00:16:40]: Yeah. It's Jufo Harari in his latest book Nexus, great book. He explained, took that example of explaining bureaucracy and like a form is forcing you as a complex human being to fit these slots, however complex you may be or multidimensional and you have to fit the slots, you have to tick a box or that sort of thing. And you can see a lot of AI. It is a much more flexible form in production use cases. Right. Whether it be a sales application or maybe onboarding, as we're talking about here, it's like a dynamic forum, but it's a better experience anyway. We would end up with a database or with all these individuals and there's all this information about them and not in a super structured format.
Fausto Albers [00:17:31]: So we might have to then use some AI with structured extraction and maybe knowledge graph, that sort of thing, or like information storage. We could even augment that information. There's. I saw this guy slightly doing a presentation. They were doing a presentation at one of my meetups. It was called Airweave. And they explained some pipeline like that that you could onboard a user and then augment it by connecting it to the Perplexity API, doing research, scraping linkedins, et cetera. Anyway, your end here would be a lot of information on each individual.
Fausto Albers [00:18:04]: Then I thought I actually bought a, made a POC on this. Very, very interesting to do, is to automatically create a virtual entity of each person, like an agent. It's basically an agent with a complex set of instructions and the information taken from the information that we've gathered. And then we ask it, like we make it, to embody as a matter of speech. Strong word, yeah, this, this person. And with the goal of then joining this virtual arena, the, the meetup floor, if you will, and to chase their goals and to connect with other agents there. And now there's some really interesting research. So my background is in sociology and social sciences.
Fausto Albers [00:18:55]: And there's a lot of like experimental research in there where you would put two people in a room, give $100 to one of them, and with the goal, with the task to share this hundred dollars and then the person that received a hundred dollars that can make an offer to the other person, right? Can make. Can be anything. And the other person can say yes, and then they share that, that cut, or it says no, and then they both get nothing. Right? Oh, now rational theory would say if. Let's say I've got $100 demi, let me give you $1. I'd rather have nothing. Walking away with $99.
Demetrios [00:19:36]: Because the other person knows that it's $100.
Fausto Albers [00:19:41]: So there's this sense of fairness, like in negotiation and then that sort of thing. And it turns out that AIs are actually really good in mimicking this. I found a repo that did this and I Took it and I made it a bit more complex to give him like a sort of extra reflective brain thinking out their strategy. I was experimenting with all this sort of like psychological research, things that were actually done in humans to find out how to set up this arena in order to get good outcomes. Because if you just let the AIs go there, they're going to find common ground with every other AI. That's just how they're. They're really nice, you know what I mean? Oh, that's. Before you know it, you're.
Fausto Albers [00:20:21]: They're building a thousand startups, which is not really realistic. Right. And there, there are also, it might be, let's say there's a hundred attendees and there's a 99 super smart machine learning engineers, MLOps people and there is one investor and then there's one, one person that's really desirable in that room because the other ones might not have to add too much to each other. So this is all given on context. So I thought like, why not use the constraints of a game and apply some rules? And I'm not gonna go into detail, but you can imagine that if there's rules and that scans are really good for this type of research is they have to be, they're constrained and then with the end goal of. And this is maybe confusing but taken from game theory, to find Nash equilibrium like that is the position where two actors are placed in such a way that if one of them moves then everyone loses. Like that. It's the optimal interdependent rewards that you're trying to find between actors.
Fausto Albers [00:21:23]: And yeah, with giving that you're basically just going to give every attendee an advice like, all right, go to this and that person. And here's some opening, opening words to start the conversation. We think you might have something to talk about.
Demetrios [00:21:35]: And because the whole idea, I remember when you were walking me through this is that for a meetup, wouldn't it be cool if we had this information on the folks who signed up for the meetup and then we could simulate out a few turns on this game and we could see, oh, our game is suggesting you talk with these three people because you all have these things in common. You might want to have a deeper conversation about X, Y, Z. And so it cuts through like you were saying, it would be great if we could all talk to everyone at a meetup. But sometimes you talk to someone and you don't want to be in that conversation. Other times you just. Sometimes I've been in this situation where I don't want to talk to anybody because I feel really shy and I feel like I don't have anything to talk to anyone about. And I imagine I'm not the only one who's felt like that in a meetup.
Fausto Albers [00:22:27]: Absolutely. Yeah. There can be like two ways when I attend like a meetup that I've never been like I find it hard to maybe even get to speak to people. And when I organize the meetup and I've been just like hosting it, like I find it hard to. There's some. They're queuing up. It's. Yeah.
Demetrios [00:22:44]: It's funny because we try and do this in some of our meetups by just saying hey, we're going to have a game at the beginning of the meetup. And we put people into teams by their birthday month.
Fausto Albers [00:22:56]: It's.
Demetrios [00:22:56]: And so you don't really have a choice. You're just going with everybody that's in the same birthday month as you. And then you have this opening. There's like this bond that forms because you're on the same team. Whether you win or lose the game, you have your team.
Fausto Albers [00:23:11]: You raise the rest of me point there because this whole idea builds upon the assumption that there is such thing as the perfect outcome. Now I used to own restaurants and one back in Corona we started building for the restaurants a QR order application that was with the goal of having people spend as little time as possible on their mobile phones. Because to me as a restaurant owner it was very clear what the benefits were to us. But it was also as a visitor I hated it to how to make this experience better for people with what if we predict what people want basically of you know, they can order from the menu like they have access to the menu through their mobile phone, but they can also. Yeah. The menu is ordered so that that it's in the right composition for that. Yeah. Long story short, in the very beginning I thought there was such thing as the perfect match between a given menu item in a given context with.
Fausto Albers [00:24:16]: With a user. And the more I learned about it, the more I realized that and I could have known this because I've been on the sommelier and the Cocteau bartender side a lot that it's really in. It's really hang out there until the match is made. So to say, given that the entity that gives you the recommendation, whether it be a nicely tied up sommelier or a recommender system, digital recommender system AI the trust that you have in that system is affecting your experience. Also known as selling ice to Eskimos. Right. You're a good sommelier. You can sell a cheap wine for a lot of money as long as you gave the person that buys the wine social recognition.
Demetrios [00:25:02]: Yeah. And actually for some reason I'm thinking about Schrodinger's cat again, because it's. You're not getting that match until the moment. And so there isn't that perfect match that you can show someone. It's really in the moment that I decide that's when it's actually real.
Fausto Albers [00:25:24]: Yeah. Yeah. And then there's. There's so many factors at play there and there's so much like the medium is the message here. And also another presentation that I saw lately was much more about the interface. UX ui, building AI applications. And that's also fascinating because AI gen AI is an interface and it needs an interface because voice is an interface. Like, it's a way of communicating and chatting is.
Fausto Albers [00:25:56]: It's an. It's an ability of this of like the communication part is what it can do, but it's also very much affecting your experience. Whether you communicate through to chat or to voice or to predefined buttons or buttons that are generated on the spot for you but still have to be clicked. And there's so much there and spoken to so many people that are building products in the last few years. And I think this is still one of the very much unsolved problems is you can have this amazing application, but how do you get people to use it? Like, one of the first rough lessons I've learned is, is that people don't give a shit about chatbots.
Demetrios [00:26:35]: Yeah. And ideally we do not want to use them. I'm one of those people.
Fausto Albers [00:26:41]: So to take it back to the abstraction of the beginning of the conversation, what we really want is something that knows that we want. Now, Oedipus. Exactly where are you when we need you?
Demetrios [00:27:00]: And it's so funny because on that it goes back to what we were saying with the being able to suggest something in the right time, in the right moment, as opposed to us having to have that cognitive load and figure out what it is that we are trying to do in this moment and then type it out and type it in a way that AI is going to understand. So maybe we don't get what we want on that first time around. And we think, is it because my prompt isn't good enough? Do I have to rephrase this? And then it's more cognitive load and it's just. It creates this poor user experience and so I've been all about that, man. Like the. The UI piece for this feels like the most important part of everything. The way that some folks are doing it where you can say let's just click around and you have.
Fausto Albers [00:27:55]: We're so used to that. It has been around for a long time.
Demetrios [00:27:57]: Yeah, yeah. And when you get like those, you probably know what it's called, but those pictures where you have two sidewalks and then there's the trail in between the two sidewalks because it's the shortest path. There's a whole paradox around that too. But the. That's what it feels like where we are still trying to figure out what is that short path. Right now we just have the sidewalks and we're trying to figure out where's that path that we can just make a shortcut. And then let's.
Fausto Albers [00:28:27]: Do you refer to what we call in Dutch olivant elephant pets. That when there's a root that people will always find the shortcut.
Demetrios [00:28:34]: That poor design in the physical world. We see that. Wow, that's not how we as humans want to use this. It's a form or whatever. It's.
Fausto Albers [00:28:43]: Yeah.
Demetrios [00:28:43]: This form.
Fausto Albers [00:28:44]: Yeah. I was taking on what you just said, like to express what you want. And still we're in, in. In this like in general, you know, as using AI is do use AI, don't refrain from using it. But if you use it, don't expect it's going to be like this miracle thing like you, you have to put in some hard work and that hard work is often to understand what you. What it is that you want.
Demetrios [00:29:12]: Yeah.
Fausto Albers [00:29:12]: And yeah, good prompting is just basically explaining what you want and all the facets that are part of that. But it's hard. And in the beginning we saw all these easy reg systems where there's a user query that get embedded and then finds the nearest vector in a database and then augments the response with this vector, like with this information. But of course we know that the query is not always semantically similar to the information that is needed to answer that query. So then what do we expect? Do we expect the user to ask good queries because forget it. Or. Yeah. Or are we going to.
Fausto Albers [00:29:59]: That's. I think in 2024 we saw this huge explosion of agentic RAG frameworks like multi hop agentic RAG frameworks.
Demetrios [00:30:08]: Right.
Fausto Albers [00:30:08]: Where one very important part of that is query decomposition, like to query understanding, intent recognition. It's as old as NLP and every and everything. Right. And I've been building a lot of those kind of things. As well. And what occurs because there's this query decomposition and then there's query routing. Where are we going to send these queries? What databases? Then there's also of course the type of embeddings, because not everything should be or can be done with the off the shelf embedding models. But the complexity of a REQ framework I think is centered there.
Fausto Albers [00:30:45]: Because this is true, right? Like given that a decent LLM has access to the information required to answer your question, right, you ask the LLM, what did I have for breakfast yesterday? Obviously that's not part of the training data, but if the retrieved chunk gives the answer to that, then it's going to answer correctly and then you can generalize to more complex problems. So the problem of RAG is retrieval and retrieval is basically precision and recall, like precision, do we retrieve the chunks that we want and are we also retrieving precision and recall? Precision being the percentage of the chunks that we retrieve relevant to the question. Is there not too much noise and recall, did we retrieve all the chunks required to answer a question? Which is actually the harder problem because you don't really know what you don't know. Right. There might be some hidden chunks there that if not present, make your model hallucinate. Now with all these agentic frameworks, I mean it's useful, but it gets so complex and it's still prompt engineering. Like you're not updating the model, like you're making layer upon layer and it's really hard to actually check if you're doing a good job like you have to. The concepts just mentioned precision and recall, you have to measure them, otherwise you don't know what it is that you're doing.
Fausto Albers [00:32:15]: And I think also often people are trying to optimize their RAC system by looking at the user query input and the generated output. And then if that's not good parameters starting like prompt engineering. The real problem is retrieval because giving that if the model has access to the right information, it's probably going to be able to answer your question. Now I was working on a job or consultancy job for helping an organization make an AI and like a virtual service engineer for like the make industry complex machinery, but I think like aircraft motors, that sort of thing. You can imagine that those asset manuals, like those technical manuals are insanely complex.
Demetrios [00:32:57]: Yeah.
Fausto Albers [00:32:58]: So if the goal is to help an engineer find the right information there. And so I started playing around and like doing some multi hop retrieval, super complex and then Deepseek just dropped like a fucking bombshell. Yeah, and we all had our sort of paradigm to look at it. Like people with money started running from Nvidia stocks and stuff. But in the technical community, I think, like, it's all been like everyone I speak to has been so stoked about, like this algorithm, the GRPO algorithm of deep seqs. So a group relative policy optimization, right? Really a real step up from the PPO algorithm that was originally, I think, invented by OpenAI in 2017 to train models with reinforcement learning, used to be done with human feedback, or at least a model, a reward model. And now with this new algorithm, we can do this without all of that, like without human feedback, without having an external model to check our results. And there was such a cool insight that this was possible, right? But this works, it's reinforcement learning, right? So it works with domains that have a definite answer, like mathematics coding.
Fausto Albers [00:34:21]: Does it execute? Is the answer correct? And then I thought, what if we look at RAG as a close domain, closed source problem, because the chunks, like you have a question and there's an answer, and to give that answer, let's say that there is this database and the answer can only be Provided when chunk 12, 15 and 20 are being added to the context of the response model. Then we have a closed problem because it's not about the answer, it's not about the information on the chunks, it's just the chunk IDs. And imagine that we would have a set of, I assume, synthetic data, and there's a bunch of cool pipelines out there that can help you create this sort of synthetic data where you feed in this complex surface menu of a thousand pages and you have it with understanding. There's like images, tables, headers, text. You make different chunks of all of them. You add metadata, you map relations. So you have a model do this, right? And then you ask the same model with access to all of that context, which is different from a RAG system where the context is hidden, right? It's masked, it's in a database, it needs to be retrieved. But in this case, the context is there.
Fausto Albers [00:35:46]: It's like a puzzle that is already made, but it's still pieces, and the model can see that. And then you have it generate questions that can only be answered giving a certain chunk or a set of chunks, or maybe even like a set of chunks in a certain order. And then you are creating this source of truth that you can't. You can use this to test your RAG pipeline because you have this definite source of, of truth and you can measure precision and recall, but you can also use it to train it. And this is the idea. Like we, we started talking about it, we. With some really cool people from different backgrounds. There's, there's a guy doing, he's building like models for cancer research, his PhD.
Fausto Albers [00:36:30]: There's, there's a professor in computer science and there's all these different people from the community, which is the cool thing of communities, that we have all these different expertises.
Demetrios [00:36:40]: Yes, diverse.
Fausto Albers [00:36:41]: Yeah, yeah, yeah. And we started thinking like, wow, man. Like this is almost. Imagine that you can, if you want, we can go into the technical details of how this would work. But basically imagine that you can. This tacit knowledge that a domain expert has is often not directly related to what they know, but their skill set of understanding the domain and knowing where to find it. If you asked someone who's been working in a research lab, chemist and that thing to do a certain test and they need different. They may not even need, they may not even know yet what exact compounds they need and where they are stored, but there's good odds that that person is going to be able to do this a lot quicker than you.
Fausto Albers [00:37:36]: Me. Right. Because. Yeah, it has all these sets of knowledge or to ask the right questions to open the right drawers without knowing that the answer is in that drawer. But you get me, right?
Demetrios [00:37:50]: It's a baseline understanding.
Fausto Albers [00:37:52]: Yeah, yeah. And in the case, for example, of a virtual service engineer, and then a real human engineer might have a question and that service engineer needs to decompose that question into subqueries and needs to make a decision where to find the data, how to interpret the state of the machine, giving like sensor data and that sort of thing, and how to use that context to make queries to find right information. Now, let's say that we have this source of truth, given this machine. We have questions that have correct answers and we have the chunk IDs to get to these answers.
Demetrios [00:38:42]: And these chunks are just to give it more information that it can reason over. Or the answer is inside of these chunks. I didn't quite get that. Or it doesn't matter.
Fausto Albers [00:38:54]: So a very, a very simple example. Let's say that the engineer wants. It's missing a part and there's part A and there's part B and there's a. Sorry, it's missing a connector. There's part A, there's part B and it's. Yeah. The question is what part connects part A and B? Yeah, Then, and this is a simplified example, of course, but let's say there is three other Parts on the left connecting to A. And there is three parts, unique parts on the right connecting to B.
Fausto Albers [00:39:26]: And we're missing the part in the middle. Then the question could be what parts do connect to part A and what parts do connect to part B. And if it is that in the retrieved information we have only one common denominator, well then we have the answer, right? That's like logical deduction you can imagine, way more complex. But this is the beauty of what I think that can be. The beauty of reinforcement learning is given that synthetic data set that we would have, then we would give these queries to. So we'd give these queries to a solid model saying like Gwen32B, you need some solid hardware to be able to run this. But. And there's been experiments out there of people with way smaller models as well.
Fausto Albers [00:40:11]: Like tiny zero is a really cool one. I highly recommend checking that out. But let's say we have a bit more body model and then we give it these queries and then we each query, we let it generate 10,000 reasoning paths and we give it access to a function, function calling. We give it access to a database, it can retrieve data in a cyclic manner and whatever, do what you need to do. The only thing that counts is then the answer at the end. And then we have this GRPO reward model that then given certain arbitrary functions. But in this case we can imagine that our final answer needs to be correct, but more importantly our retrieved chunks need to be the right chunks. Then, well, we have this new subset with correct answers, correct chunks.
Fausto Albers [00:41:04]: And then maybe we want to further refine this subset by having a function that, I don't know, optimizes for the shortest reasoning path or the longest. Or there's different things you can do there, but you maybe end up with the 10 best answers for each query. And you do that, then it updates the parameters of the model giving this algorithm and then you do that many times. Then I would love, maybe I am like way off the chart here, but I'd love to hear if someone thinks so. But yeah, can't it be so that we are squeezing this. It's hidden somewhere in this model already. The ability to use the correct reasoning path to find the right information, not necessarily return the correct answer to optimize the reasoning path.
Demetrios [00:42:00]: So let me play this back for you and make sure that I am understanding it correctly. It's basically saying that we need to give the right context to the model. What we don't necessarily need to do is give it only the Right context in one shot. It can be various iterations of giving it context. And since the model can reason, we can now say figure out if that information is there because we've already done a bit of training or fine tuning on it to show it what the right context looks like and how to know if you are retrieving the correct.
Fausto Albers [00:42:46]: Chunks in reasoning models. And mind you, we start here with a non reasoning model and this is referred to in the Deep Seq paper as the aha moment, all right, that the the model starts to reason and self reflect on that reasoning and then take another path because I'm not sure. And so it's a very. If you see these reasoning models like O1 but it doesn't review all his reasoning tokens but Deep SEQ does. If you see these reasoning models reason it's a very anthropomorphic like human like way of reasoning and it shows a lot of doubt perhaps and maybe and, or, and this makes it well first of all very much understandable for us. But it's also an important thing because when there's too much doubt and our problem that we want to solve is relying is like multi hop. For example, we want to know how many atoms a compound has given the combination of atom one first, sorry compound one and compound two and then do this and that to it. So then we have to know, we have to be sure that we understand the atom count of compound one and compound two right.
Fausto Albers [00:43:59]: So if we make a mistake there, a false assumption, then our final answer is never going to be right. So if you have a reasoning model reason over this, you'll see this lot of maybe, perhaps, et cetera. Now there's this great paper, it's called O1 search. It was I think published just before the Deep SEQ thing or at least it's not referring to that, but it's a way and they open source the whole code as well to have reasoning models recognize their own ambivalence. Like if they're not sure enough and then stop the reasoning process and basically perform a search for more information like connect to a rag system does some other things as well. And it's not function calling, it's actually making a. It's formulating a question encapsulated between string tags and added with a special token that gives the script the the call to stop this process, find this new information and it's actually sending it to a parallel chain that has access to all the steps so far it's cleaning those chunks for better precision and then adding it back to the main loop and then continuing and it can do that n many times. So this is using a reasoning model to be more sure.
Fausto Albers [00:45:16]: And you could imagine that you could make this ability like to improve this ability a lot. And I think you could imagine using that formerly mentioned reinforcement learning way there as well.
Demetrios [00:45:33]: I'm trying to just make sure that I understand this. Basically the reasoning model recognizes when it doesn't know if it is right or wrong or it doesn't. It recognizes when it does not have the right information or enough information to be able to confidently make a decision.
Fausto Albers [00:45:55]: Yeah.
Demetrios [00:45:56]: And it can then go and find that information. And you're saying instead of like with the O1 search, the potential that we could have in a rag system is that instead of it going out and trying to find that information on the web, we can just go and try and find that information in our embeddings.
Fausto Albers [00:46:17]: Yeah. In any external source it could be the human, it could be web.
Demetrios [00:46:23]: So it asks another question.
Fausto Albers [00:46:26]: The important part is in any reg system like I've been going to like in very much into the sort of hypothetical solutions. But if you take a step back is a rag system needs to know if it needs to find more information. Is it? Sure. Is that the information that it has access to in the training data, is that enough? Do I need more information then. Then I get more information. Is this enough? Is this what I need to answer the question? This is something that is. You can't possibly do that rule based in for real world scenarios, right? Yeah. Then part of that but more technical is that the system needs to be able to understand the query for the user to decompose that query in the optimal way and making the search queries optimal, whether it be for web, human in the loop or vector.
Fausto Albers [00:47:17]: But that's more a technical thing. Like it boils down to how good does this system understand its own limitations and how can it be sure of things? And we cannot prompt you can improve it. But how could you ever do this in a sort of rule based manner. Right. But what you could do is use reinforcement learning given that you have a set of correct answers, correct chunks and then just squeeze it out like blood out of stone.
Demetrios [00:47:48]: I think the fascinating piece here, and one of the hardest pieces about this is that you are trying to coerce the model into this unconfidence. And as we all know, anyone who's worked with LLMs is their confidence level is off the scale. And so they.
Fausto Albers [00:48:10]: That is different with reasoning models. Right. I think what we see with scaling, inference, time, compute. Is this sort of not being.
Demetrios [00:48:20]: Yeah, yeah. To not think that it knows everything.
Fausto Albers [00:48:22]: Right.
Demetrios [00:48:23]: But still, I think that's the big unlock here is being able to say, because of the way that this question was phrased, I can only get this far. So maybe we need to go back and retry one of these steps so that like low confidence score is huge.
Fausto Albers [00:48:47]: Yeah. And I think because it's so hard to explain this in rules, that if this sort of approach would work that it would probably be limited to certain domains, just like in human domains where. Yeah, it's, you know, given this, given that. Good old beige. Given this, given that. And that is information. This would be the right question. Right.
Fausto Albers [00:49:11]: And this is what it's like the best information looks like. Now I can generalize that to maybe when I'm a bartender and I know my way in the bar and I can maybe generalize that to the kitchen, but I can't generalize that to the lab. Right. There's so much, so many smart people working on reinforcement learning, on making better rag systems in all sorts of ways at the moment. But we're going to see this like we're not going to see AGI just yet in that sense, whatever it means. Up for another one? Yeah, totally different, but this closed source of smaller domains where a combination of reasoning models, agentic frameworks are working together to solve problems within a closed source. Like Ethan Mollick said it, I think he was that he said, give a good example. We have now the OpenAI browser agent which is like this wide, can do anything.
Fausto Albers [00:50:03]: Let's say that's the AGI kind of thing. And we have deep research. Right. And both are combinations of agents and reasoning. And with browser agent, it's nice, but it still really sucks. Like it's not useful. Deep research is amazing. Not the holy Grail grill yet, but I'm using it like a lot.
Fausto Albers [00:50:26]: I'm not using browser agents. That's what we're going to see whether it is in. In. In domain specific react intelligence or tasks like. Yeah, it's, it's fascinating and I bet we're going to see a lot of progress in the tactical.
Demetrios [00:50:40]: So take me back to what you were doing with the manuals for all of these. I think it was the airplane engineering diagrams and all that stuff, machine diagrams that are very complex. How does this play into that? I know you gave the very simple example of there's a connector and there's those two pieces and we got to figure out which one can connect the two. And you're assuming that there's only one answer there.
Fausto Albers [00:51:09]: Yeah, but it might be, there might be more answers, of course.
Demetrios [00:51:11]: What are the other examples of this? How you build the RAG system to make it work? What does that all look like? I guess is what I'm trying to figure out.
Fausto Albers [00:51:21]: I was asked in this project to look at like how we could test the knowledge of engineers and there's like a contradictory in Terminus because try ask someone. The whole definition of tested knowledge is that you can't really explain it. It's just you do what you do because you know how to do it, because it feels right. And yeah, the idea, however complex it may be and I think we should really start very simple to, to verify if it's even and we're chasing this pot of gold at the end of the rainbow. But that if there is an answer and you could indeed use human knowledge for this as well. But if there is an answer to a complex problem and there's external information that that is, that's needed to come to this answer, then we should be able to use reinforcement learning to find the right path. Because there is many possible paths. Right.
Fausto Albers [00:52:18]: But try, give it enough tries and you might just end up on a very good path and do that many times and then you have examples or you train a model to, to behave that way. And I think what it comes down to, what is so fascinating is that people say that models cannot answer questions outside of their training data. Well, that's a truism of course. Yeah, of course. But what is in their training data? Is that a set of discrete words? Or is there emergent capabilities of all this information stored in this network? And one of these emergent capabilities seems to be reasoning. That is the aha moment right of the deep Seq paper. And with reinforcement learnings, if reasoning is already present in this set of set of information in this set of data, what else could be there? And I don't know, I don't find it a weird idea that there's much more present. So yes, models cannot answer questions outside of their training data, but that's only really relevant for real time facts as what did we have? And what did I have for breakfast yesterday? When it comes to capabilities, I think there's much more possible than we've seen so far.
Demetrios [00:53:36]: I like that you say that if there is an answer and we can correctly make that answer be known, there's a yes or no answer or a way to do it and task complete, task not complete type of binary way of looking at it then we can figure out how to get AI to try and do that. I I love talking to you about.
Fausto Albers [00:54:06]: This stuff I don't know if they actually use this but they shout out to Craig Cameron he he he learned this this Snake game so you can compare models they're playing Snake against each other and great way to test the capabilities of the models problem solving skills etc. And then someone in the comments was on LinkedIn said I wonder how long it's going to take before each game is over instantly oh wow and it's just a roll of dice because they all panned it out they played it out and you can like in this sort of constrained space of a game like that is a relatively simple game like that you can imagine that reinforcement learning can get to that like I don't know I'd love to hear people who know more about it to say that this is possible or not that.