Challenges of Working with Voice AI Agents // Panel // AI in Production 2025
speakers
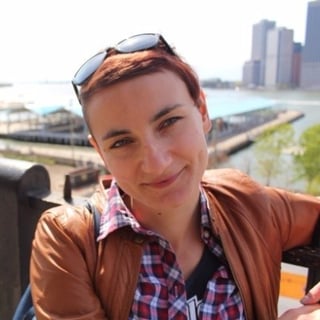
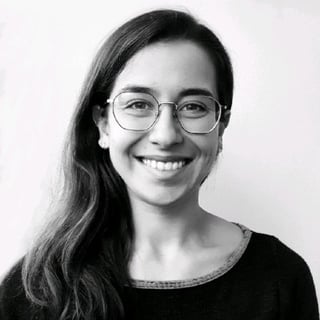
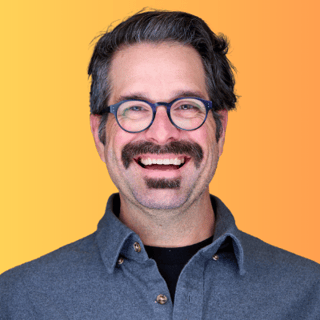
I'm an AI builder and educator with 13 years of product leadership experience at both large enterprises (Pandora, Amazon) and scrappy startups (Rdio, Bryte). I'm also a mediocre mountain biker, voracious podcast consumer, and pretty fun dad.
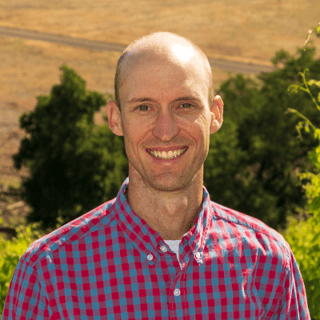
Tom Shapland, PhD, is the cofounder of Canonical AI. He loves working with Voice AI developers to help them analyze and improve their agents. Before Canonical AI, he was the cofounder of an agriculture technology startup.
SUMMARY
Voice AI agents sound great in theory but in practice? They come with a whole new set of challenges—latency, accuracy, real-time processing, handling ambiguity, and making them feel actually useful. This panel digs into the gritty realities of building and scaling voice agents that don’t just talk but truly deliver.
An MLOps Community Production sponsored by Humanloop & Rafay
TRANSCRIPT
Adam Becker [00:00:04]: And voice agents is the next topic that we have. We got a panel coming up here, so let's see. I'm going to let everybody in. Gatam, Monica, Rex and Kiara, are you all here?
Rex Harris [00:00:14]: Yes.
Tom Shapland [00:00:16]: Hi everyone.
Rex Harris [00:00:17]: Okay.
Adam Becker [00:00:20]: Very good to see you and very good to see some of you again. Thank you for coming and I'm going to hand it off to you guys. Tom, I believe that you're running the. You're hosting the panel today.
Monika Podsiadlo [00:00:32]: Correct.
Adam Becker [00:00:33]: Awesome. So I'm going to be back in 20 minutes folks. If you have questions, please drop them in the chat and I'll see you all very soon.
Tom Shapland [00:00:42]: Great. Well, welcome everyone. We're so excited to speak with all of you about about Voice AI. I'm really excited about the expertise on this panel and the perspectives that they're going to bring to the Voice AI about the Voice AI space. I'd like to start with some introductions and we're going to go about this, starting with Chiara. Chiara, would you please tell us about you and how you got into Voice AI and what you're working on, what you see your expertise is. I realize I'm being a little bit nervous. I gave you too many questions, so let's make that a little bit simpler.
Tom Shapland [00:01:14]: Please tell us about you and what you're working on in Voice AI. I think just those two things is probably a good start.
Chiara Caratelli [00:01:21]: Cool, thank you. Hi everyone. I'm Chiara Decaratelli. I'm a data scientist at Process. A couple words about Process for those who don't know it. It's a global technology company with about 2 billion customers in areas like E commerce, food delivery, fintech, classifieds and we focus heavily on AI and also have a ventures branch that is always looking for promising AI startups and opportunities. Personally, I work on frontier projects, especially with AI agents and multimodal AI, hence Voice. Together with our portfolio companies so far we built several voice applications mainly for E commerce and edtech.
Chiara Caratelli [00:02:01]: And I test how these technologies interact with users and what kind of possibilities they open. So yeah, happy to meet you all. Looking forward to our discussion.
Tom Shapland [00:02:13]: Great, thank you. Monica, how about you go next?
Monika Podsiadlo [00:02:16]: Hey, my name is Monica. I'm one of the founding members of the speech synthesis group at Google where for over 14 years I've been focusing on various voice AI solutions for essentially all of Google's speech outputs in various ICN leadership roles. Before that I started my career in a startup building voice AI for computer games industry.
Tom Shapland [00:02:39]: Great, got it. Thank you. Rex, how about you go next?
Rex Harris [00:02:43]: Yeah, thank you. Tom, welcome Everybody excited to be here? I am currently on a solopreneur journey building all sorts of AI systems, including voice agents and conversational AI automations, that kind of thing. My background is primarily in product management, product leadership, been doing that for 12 plus years. I had actually worked on Alexa for a little while at Amazon. So, you know, this era of voice as an interface is very exciting for me. I feel like I'm getting to finally see everything that we wanted to come to fruition happen right before our very eyes. And so it's been a blast kind of working in the space over the last year or so as an entrepreneur.
Tom Shapland [00:03:34]: Great, thank you. And I will go last, I guess here. So I am Tom. I am the CEO and co founder of Canonical. We build observability for Voice AI agents. So for people that are building Voice AI agents, we help them see what's happening in production when real humans are interacting with their agents so that they can improve them. So where I'd like to start with our discussion today is with. I'm sorry, I'm not sure if I'm pronouncing your name correctly.
Tom Shapland [00:04:03]: Kiara. Is that.
Monika Podsiadlo [00:04:04]: Yep.
Chiara Caratelli [00:04:05]: Yeah, that's correct.
Tom Shapland [00:04:06]: Okay, so my first question is for you, Chiara. I think so much of what I see, so much of why I think Voice AI is a fun space to be working in is it's a whole new like user experience and ui and there's all these new challenges, interesting engineering challenges to like building Voice AI systems, not just from an engineering perspective, but also design. I think that's so much of why it's fun and also why it's a great community because there's like, there's a lot to discuss among other builders of Voice AI. If you're building a Rails app, people know how to do that. But Voice AI is like, well, how do you do this part and how do you do that? It lends for a rich community. And the thing I wanted to ask you is from the sense of when you're building a Voice AI agent, what are some of the things that you have to consider that are for building the design and the architecture of that Voice AI agent? And how might that be different than building a text based app or a live chat?
Chiara Caratelli [00:05:09]: There are definitely many new challenges that arise with voice. I can give you an example of how we approach this. Last year we were building an agent for E commerce that would browse the web and be able to search for items, purchased things for a user. And at the same time we were previewing OpenAI Real Time API, so very exciting. Real time unlocks so many possibilities. So we wanted to connect this and have a real time agent that could perform tasks for the user. At the beginning we were trying to use the same text prompt that we were using for the text agent and yeah, that failed miserably. It was clearly not working because with text everything is nicely structured.
Chiara Caratelli [00:06:00]: You see everything, you can have a lot of control. But it is not the case with voice. Some of the challenges that we encountered are that the user gets very frustrated if the agent doesn't respond immediately. This has a big implication with tool calling. When an agent needs to act, we need to rethink a little bit how this flow goes. Tool calls need to be as short as possible because the user needs constant feedback. Also you need to make use of all this constant stream of events that you have via WebSocket or WebRTC to inform the user. If we start, for instance, a web search, the agent can inform the user that it's starting and then user can interrupt and you know, interact with the agents during the tool call.
Chiara Caratelli [00:06:58]: That was the biggest change. We even went to the extreme of doing things completely async. So we had some very slow processes like actually purchasing the item for the user and in that case the agent would inform the user first and tell them like, hey, I'm going to do this and that you can close the call, I will let you know later and just send an SMS reminder. So yeah, that was one of the biggest changes. The other is that everything needs to be structured as much as possible. So for instance, user input can be very vague and sometimes it's very hard to understand what the user means if they're talking about postal addresses, names and things like that. And I feel like we're not in a stage where the voice agent is so self aware that understands where it got these things wrong. So we need to play around with that and avoid flows where user needs to spell.
Chiara Caratelli [00:08:05]: So for instance, have the user insert this data in an application and recognizing the user through the phone number, if it's a phone, phone call, and so on, and also trying to structure as much as possible the output. So try to, you know, if you need for instance a task of searching for an item and purchasing an item could be can be created with a certain structure input and using the agent to fill that input and asking the user if some things are missing. Yeah, these were the main things. I could go on a lot, but I'm happy to hear if you also encountered similar challenges.
Tom Shapland [00:08:50]: Yeah, so part of what you're saying there, my way of thinking through the state of voice AI right now is that we have these amazing models like our Speech to text, our LLMs and our text to Speech and some of our orchestration models around that too, like our VAD models. They're really incredible, but they're still immature, they're still not quite there yet. That you can get a voice AI agent to be production ready out of the box by just stringing together these systems or using a voice AI orchestration platform. There's still a lot of craftsmanship that goes into taking your initial MVP and bringing it to something that's production ready. I think the canonical example of that is around spoken letters, where you really have to work carefully around spoken letters. Because I love what you said about self awareness. The models are not aware of the fact that they're not good at saying letters or understanding them. And I want to next hand the mic over to Rex because I, you know, I think it would be good to segue to you with a very similar question of like, like, what are some of the things when you're building voice AI agents where you thought like, okay, I have 12 years of practice product management experience, like, I know how to build a product.
Tom Shapland [00:10:04]: And then you start building a voice AI agent, you're like, oh, this is different. This isn't what I was expecting of what I would need to consider in building the user experience for this product.
Rex Harris [00:10:15]: Yeah, yeah, totally, I think. And you know, much of what Chiara was mentioning, you know, is definitely resonating with some of my experiences. I think, you know, it's hard enough, you know, starting to build applications on top of LLMs to begin with in terms of their non deterministic nature. So testing and trying to enforce some level of predictability and consistency is already kind of difficult in and of itself. And then with voice, you add a couple additional layers on top of there, some of which Kiara mentioned, because then you have to make sure that the transcription is coming over correctly in terms of know what somebody had said and what that inference was. And then you also, you know, have these kind of very. Call it, you know, this term is kind of overused, but it's like the vibes of the conversation are also something you need to, you know, be aware of. You know, what is that kind of tone intonation? You know, often, even with the latest models, the voice agents tend to, you know, have a hard time knowing when to speak or when to be, you know, sort of silent.
Rex Harris [00:11:35]: They definitely can't be silent for Very long. Unfortunately, that's still kind of an ongoing issue if somebody's thinking, you know, the, the agent has to, you know, seems to want to interject quite often. And so there's kind of like this, you know, stack, you know, of different kind of like user experience variables when you're building any application. And, and this just kind of adds a few more to the sort of like fault tolerance of that stack and it makes it so that it's much more challenging to test, it's much more challenging to enforce a level of consistency so that the user experience is what you'd kind of envisioned and what you may have. We might talk about this a little bit or what you may have seen in a prototype, you know, for, you know, a few runs or a demo. Things change dramatically when you have hundreds and thousands of conversations that you're then, you know, providing with real humans.
Tom Shapland [00:12:40]: I like to say that humans are nature's most unpredictable force.
Rex Harris [00:12:44]: Correct. Very, very good point. And you know, we should celebrate that fact, especially given what we're talking about. Right. We don't want to be too predictable. That's a boring life. But it definitely just kind of adds to everything you need to think about as you're trying to deliver a world class user experience.
Tom Shapland [00:13:08]: Yeah, great. Thank you, Monica. It sounds like you have experience both in the development of the model there and also experience in building the applications as well. So, like, is that a good understanding of your career experiences in Voice AI that you've helped shape the models and also like help build, bring those models into things like Google Maps or maybe you could elaborate a little bit more on like the sorts of things that you have built to get everyone that's listening and the other panelists kind of up to speed with your expertise. A lot of the people. And part of the reason I'm asking that question is there's so much interest in voice now and there's a lot of newcomers like me that have just come into the scene over the last year when people start bringing voices to LLMs. And I talked to a lot of people building in the application layer. But it seems like you have experience in different parts of the stack.
Monika Podsiadlo [00:14:06]: Yeah, absolutely, that's correct. And I would echo what both Rex and Chiara said. Voice AI is such a complex problem and I don't even know how to splice kind of my story to express the complexity of that. Right. But let's say that, okay, you nailed the voice. You think you have a voice that sounds great, it sounds natural, sounds like a human. And it says what you needed to say. And then you try to put it in an agent scenario.
Monika Podsiadlo [00:14:35]: When you have a dialogue with a user and suddenly it's not just about having a great voice. It's also about interpreting what the user is saying and reacting to it factually. But then also emotionally, you need to interpret those very, very nuanced and subtle clues and somehow instruct the voice to act accordingly. When you have something like a navigation direction voice telling you to turn left, to turn right, to stop at the light, and you're destination being on the left, this is like a very simple use case. But you might make, you might want to make it more complex. You might, for example, inject into that voice not just factuality, but some emotion that would maybe ask you to slow down. Right? Using the vocal clues and encourage you to drive more safely. There's a lot you could do with that voice.
Monika Podsiadlo [00:15:34]: And now imagine more of a modern scenario that we see right now in the field. An agent, a companion that is genuinely trying to have a conversation with you. How do you build an agent that reacts to. Imagine that your user is a young girl who's asking, how can I lose £20 in three days? What kind of voice agent do you have? Right. You want to build a solution that would be a good agent in the world. So from the kind of semantic or pragmatic perspective, you want the voice to redirect the user into more of a healthy frame of mind, discourage them from ideas that might be potentially harmful. How do you translate that into voice acoustics? Right. What kind of voice do you see could accomplish this most effectively? Empathetic, maybe softer.
Monika Podsiadlo [00:16:34]: Do you want more of a kind of educated, maybe harsher, maybe more like I'm a doctor type authority tone? How do you build that? And that's all on top of just being correct as a voice, pronouncing the words right, interpreting the letters right, having the right melody, and all these things that we often don't, we take for granted, but they're really extremely complex. Imagine that you're supposed to interpret something that is given to you in a textual form that just has a simple sequence of doctor and the dot. What does your voice agent say? Is that a doctor or a drive or maybe still something else? So the layers of complexity are just immense when we're talking about voice AI in my career, I've touched upon, I would say each and every one of them. But now my favorite challenge right now is building agents that cross this uncanny valley, as we call it. So we still don't know how users react to voice AI, we don't have enough data. This is still very fresh and very new and very exciting. Traditionally, we're seeing a little bit of resistance to interacting with new technology where there is a certain. The user is unsure whether they're talking to a real human or an AI agent, and there is a certain reluctance to really dive into that interaction.
Monika Podsiadlo [00:18:04]: So trying to really see what would help here to drive user adoption, what people prefer, what makes them feel comfortable, what makes them feel comfortable right now, which is probably different to what will make them feel comfortable five years down the line. I think in five years, we'll all be very, very comfortable talking to machines, and we won't really wonder about these things. But right now, you know, you, you pick up the phone, you hear a voice. Okay, sounds legit, and then you're thinking, hey, is this AI? Is this a real human? How do I act? Do I just, you know, hang up? Oh, no, we don't hang up right now. Like other millennial thing. So, yeah, layers of complexity. And this is my favorite. How do we drive adoption? How do we build agents that sound appropriate to the context, with all the emotional contours and nuances that humans have in their speech, and at the same time encourage fruitful interactions with users?
Tom Shapland [00:19:08]: Let's run with that idea. I'd love to hear Chiara and Rex's thoughts on, on what you've learned in building voice AI agents to help drive their adoption. Or maybe even stated a different way, like, how do you reduce the percent of calls that result in the human caller hanging up? Like, what are some of the lessons you've learned to try to keep the caller on the line longer and really make people more open to engaging with the machine to help them and give the machine a chance to help the user complete their objective?
Chiara Caratelli [00:19:41]: Yeah, I think there are a few things that we try and that worked. One of the agents that we built was in the edtech space. We had a number that you could dial to ask questions about a certain course. So we noticed that users felt much more comfortable when the agent was speaking, not only with natural tone, but also with expressions like utterances, like hum, you know, this kind of things we say when we speak that the model doesn't really pick up if you don't prompt them. So having good, good prompting was really good for that. Also, poses. Users get really frustrated when there are poses, especially initially. I think after 250, 500 milliseconds, users already realized that they're talking to an agent.
Chiara Caratelli [00:20:43]: Even if they know they are talking to an agent, it still feels a bit uncanny. Something very important was also handling languages and different way of speaking, like dialects. I think we always do this test in the lab in English where we know how to talk to the agent. We can, you know, speak very clearly to them. But once you, you have a food driver in traffic in Sao Paulo calling the agent, maybe they're angry because they need to handle something that went wrong with the restaurant. Like, how do you handle that? So for that we built, yeah. Test set, like generating different samples with different emotions, way of speaking languages, different type of noise. And yeah, that's one way that worked to improve.
Chiara Caratelli [00:21:43]: And yeah, I think these were the main ones. Important is to understand that once you put an agent in a real world scenario, it's going to be completely different and expect anything from that interaction.
Rex Harris [00:21:58]: Yeah, it's the Mike Tyson saying, everybody's got a plan until they get punched in the face.
Chiara Caratelli [00:22:04]: Yeah, exactly.
Rex Harris [00:22:05]: Never know what to expect. Yeah, I want to add on to that and actually Monica had a point that I wanted to sort of drill down a little bit that's related about the sort of like emotional, you know, kind of reactivity that the sort of voice agent needs to nail. And it's really hard because I think everybody on this call has probably done some sort of prompt to an LLM that says, you know, you are an, you know, given a role, you are an empathetic voice agent, you know, blah, blah. But because of, you know, the unpredictability of humans and the sort of range of emotions that can happen through any dialogue, any conversation, you know, empathy is important, but that may not always be exactly how you want to respond. And so it's very difficult to build these voice agents to have that range of emotions to appropriately respond. And, you know, I think a lot of the models are getting better. You know, certainly, you know, what people have done over at Hume and Sesame, you know, kind of the more recent stuff that, and I know Google DeepMind's working on stuff, but, you know, there's, there's definitely a lot of room for improvement on that. And, and it's exciting, but it's challenging, I think, to.
Rex Harris [00:23:29]: More specifically to the question, to echo some of what Chiara was mentioning in terms of like that single kind of dialogue, you know, making sure that you're not losing people, whether it's through pauses or, you know, they're kind of, you know, the voice agent starts to get hung up or kind of has these kind of like telltale signs that you're talking to an agent, not a human. I mean, it's very dependent on what you're trying to accomplish. You know, some folks are going to want almost to rather talk to an AI agent because they just want like a transactional conversation versus, you know, you know, having some long sort of, you know, dialogue with somebody and having to kind of, you know, determine the nuances of that person's individual, individual personality, that kind of thing. I think where it gets way more difficult and stuff that I've been working on with some of my clients is building a voice agent for repeat dialogues. So an agent that you have that works to help you with something over multiple conversations and the retention metrics around getting people to call again, man, it's hard. It's like a really fun challenge to work on. Again, my background being in products, specifically on B2C apps, almost primarily that sort of challenge of what makes your product sticky, what gets people coming back over and over. It is definitely a fun and rewarding challenge to work on.
Rex Harris [00:25:07]: But holy cow, it's so much harder with these voice agents because you need to. Just like you would with a person, you need to not just be transactional, you know, for a single conversation, you need to develop some level of relationship that would bring somebody back. You know, it's like, you know, as you get older, it's a little harder to make friends. And, you know, it's like, you know, the sort of, like, the interactions that you have with a voice agent need to be so good and so compelling that when you hang up, you want to call back and you want to be able to have another conversation. And, you know, I can't say that, you know, we've kind of nailed every bit of that. So it's an ongoing challenge, but something for everybody, I think, on the call to kind of think about as they're interacting with, you know, different consumer apps, you know, that offer this type of modality, but also as they're building it for themselves.
Tom Shapland [00:26:08]: Thank you. There's so much to cover here. It's really interesting to. I feel like all we get to do in a session like this is just scratch the surface, unfortunately. But, Monica, I actually want to circle back with you because we kind of kicked off this cup, this thread of the conversation. You kind of kicked it off, but I wonder if you have more to add after listening to Chiara and Rex around. Like, how do you make. If you're building an agent, the A voice AI agent, how do you decrease the rate that people are hanging up on it and how do you get them to stick around more? Do you have anything to add?
Monika Podsiadlo [00:26:41]: In addition, I think it's really, really important to understand the product that you're building. You know, I, I love working on the engineering for voice AI, but I'm eternally frustrated with very vague product definitions because I think voice is so complex. It's like a very human experience and we cannot just cover all of it. So it's going to be very important, I believe, in the next few years to build agents that maybe are not comprehensive, but are great for whatever the product is. And that is very tricky defining what you're actually building. For some things, building something very transactional, maybe it's going to be a little bit more straightforward. And I would say define a strong MVP and don't go beyond that until you have more data. Don't add any, you know, extras until you nail your mvp.
Monika Podsiadlo [00:27:37]: And then there are things that are more complex. If you're building, let's say, a companion for the elderly. Right. This is a more emotionally charged scenario and there is so much more to figure out. And then, you know, you have to be a lot more thoughtful designing your product. But for something like refunds, that can be emotional too, but less so than having a companion of being reminded about your medication schedule. You can be a little bit more transactional and focus just on efficiency, voice quality, voice acoustics that make you intelligible, standing out against those noisy backgrounds, just like Kiara mentioned earlier, that's a big problem, actually selecting the tone of voice that would work for the environment in which the voice will be used. I think as the field develops, we need to be more thoughtful about those more complex scenarios where we want to build an agent that is more in tune with the user's emotions or has the retention of emotions, facts, requests to provide ongoing support.
Monika Podsiadlo [00:28:50]: As an agent, this is incredibly complex. Yeah, I don't think we have time to go into that. I think we're making partial gains in the field and we just need to persevere. I think it's going to be very frustrating for the next few years as we're tackling those more complex challenges, because the gains will be maybe very incremental or maybe there's going to be massive failures when the users are going to give us, send us a strong message like, hey, that was too much, or that was completely wrong, but we just have to persevere and keep listening. And I think what's most important is just being a good agent in the world. In case of doubt, design your product in a way that makes the world a slightly better place. Go for the safety, go for the be more risk averse in terms of that emotional tone and the empathy and, and what kind of assistance you offer to the user because it can get difficult and complex very easily. So define the domain, focus on the domain and ace it and then keep building.
Tom Shapland [00:30:05]: On top of that, what you're saying reminds me of something that I had this interesting conversation with Lily from Rhyme. If we just focus on the text to speech element of voice AI agents for a moment, the, the most I'm in like a, a group of YC founders that work on voice AI agents and the most frequently recurring question in that group is like, what's the most natural sounding agent? Most natural sounding text to speech voice. What are you guys using? What do you recommend I use? And it recurs like once a month. And I actually think when I brought that up to Lily, she said that's the wrong question to ask. It's not what is the most natural sounding voice, it's what is the most.
Rex Harris [00:30:53]: Appropriate voice for your use case for your product.
Monika Podsiadlo [00:30:58]: And people should focus a little bit more on answering this question. It's a very fundamental question. What are you building? And then tailor the agent exactly to that.
Tom Shapland [00:31:09]: Yeah, that's great. Thank you guys.
Adam Becker [00:31:12]: Hey Adam, is mine the most appropriate voice right now? I have a question. I think so. A few folks from the audience had a couple of questions and if we have time, I also have about 1,000 for you. Let's see, for the first one, Joe is asking what kind of experience and frameworks are you seeing positive outcomes in with voice enabled avatars? And in this case it's in situations where the person knows that they're dealing with an AI.
Tom Shapland [00:31:41]: Okay, so his question isn't about building voice AI agents to replace existing call volume for B2B or B2C use cases. It's more in the the LLM enabled novel use cases where people are talking to a character of some kind. Is that how you interpret?
Adam Becker [00:31:58]: That's my understanding of it, yeah.
Tom Shapland [00:32:00]: The question is, could you help?
Adam Becker [00:32:02]: What kind of experience and frameworks are you seeing positive outcomes in with voice enabled avatars?
Rex Harris [00:32:09]: I could maybe take this. I don't know if it's necessarily directly related to the frameworks question, but I did work on a project for a client where it was for folks who were in recovery from prostate cancer surgery and it was for a urologist. And for this we actually use heygen and what we had built for those individuals was an avatar like experience that they could call at any point, they could kind of get on a zoom call with and get any information they needed about their recovery because it's a two to three year long process and you know, access to the doctor surgeon was difficult. And so the framework we used for that was, you know, kind of just using retrieval, augmented generation, you know, rag over a bunch of documents that talk that the doctor had sort of like, you know, kind of curated specifically for that recovery process. And so any question that was able to be answered within that documentation, the avatar would do so. And it was just kind of a very lovely experience being able to feel like you had kind of like almost like a nurse, you know, that you could call at any point 247 and then anything outside of the documentation would then, you know, the avatar would respond with, you know, let's set you up for a follow up appointment. So I don't know if that's kind of where Tom was asking but that was definitely successful and you know, really from a kind of user experience was, was really nice for the end user.
Tom Shapland [00:33:56]: Monica, it seems like you have to drop. Do you have anything to add to that before you have to drop?
Monika Podsiadlo [00:34:01]: No, I just wanted to thank you for a great conversation and feel free to email me if there are any follow up questions. I'll be happy to answer but unfortunately I am going to have to drop off. But thank you so much for hosting me.
Adam Becker [00:34:13]: Thank you for joining us.
Chiara Caratelli [00:34:15]: Thank you.
Adam Becker [00:34:16]: Thanks Monica. Actually there's another question here in the chat. How do you address cultural context and variation in different languages?
Tom Shapland [00:34:29]: I feel like that's a good one for Kiara. She alluded to the fact that she's worked with her EdTech and E commerce agents in various cultures and locations.
Chiara Caratelli [00:34:39]: Yeah, we worked a lot with Brazil through iFood, which is one of the biggest food delivery companies. It's based in Brazil. And yeah, there you have a lot of, you know, regional dialects and different ways of speaking. Some people will speak faster, slower as well, like, like everyone. But it's something different from what you would see in English which is, you know how most of these models are merely trade on And I think yeah, the best way is to really test extensively like build actual test set that reflect what your product is going to interact with in real world. And there is a lot also of generative options for creating this test set. For instance we use directly OpenAI real time API at the time when I was working on this project and it worked pretty well like simulating different interactions with yeah, different languages but Also emotion that can change. And yeah, of course, once you start to get real data, then you can really act.
Chiara Caratelli [00:36:02]: And yeah, shout out to Canonical for this. Yeah. So I think really understand where your product, how your product is going to interact. So to refer to what Monica was saying and like test extensively. Don't think that if it works in English, it's going to work in other.
Adam Becker [00:36:23]: Languages as well, building robust tests. Thank you very much, folks. If others have some more questions in the audience, if you can please hang out in the chat for a bit.
Rex Harris [00:36:36]: Adam, can I plug one thing real quick at the end?
Adam Becker [00:36:39]: Yeah, go for it.
Rex Harris [00:36:41]: These are really fun to work on. This is a little bit of a throwback to my, my, my talk last year at this conference. These are very fun to work on. The like, experience of being able to like, speak with this agent as you're kind of building it and like, really feel like you're kind of like, you know, understanding its personality. It's really fun to like demo to your friends and family and your coworkers. Like, please, like, these challenges are important but like, don't let them be a barrier. This is like really, really fun technology and we're all kind of figuring. So highly encourage everybody on the call to like try this out, even in like a no code fashion with a tool like vapi.
Rex Harris [00:37:19]: Just go in and like, you know, start to kind of explore. It's a really awesome time to be in this space.
Adam Becker [00:37:26]: That was an excellently inspiring notes to end on. Rex, I'm going to follow up with you because I have a bunch of ideas for things that I want to try out.
Rex Harris [00:37:35]: Yeah, right on, man. Hit me up.
Adam Becker [00:37:37]: Thanks for the inspiration. Tom, Chiara, Rex, thank you all for joining and Tom, thank you for facilitating. This was absolutely fascinating.
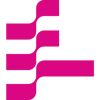