Collective Memory for AI on Decentralized Knowledge Graph
speakers
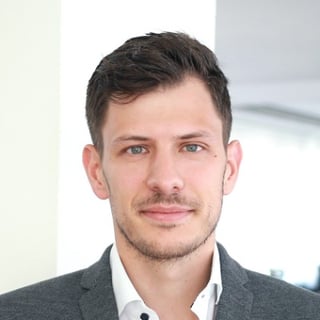
Tomaz Levak, founder of OriginTrail, is active at the intersection of Cryptocurrency, the Internet, and Artificial Intelligence (AI). At the core of OriginTrail is a pursuit of Verifiable Internet for AI, an inclusive framework addressing critical challenges of the world in an AI era. To achieve the goal of Verifiable Internet for AI, OriginTrail's trusted knowledge foundation ensures the provenance and verifiability of information while incentivizing the creation of high-quality knowledge. These advancements are pivotal to unlock the full potential of AI as they minimize the technology’s shortfalls such as hallucinations, bias, issues of data ownership, and model collapse.
Tomaz's contributions to OriginTrail span over a decade and across multiple fields. He is involved in strategic technical innovations for OriginTrail Decentralized Knowledge Graph (DKG) and NeuroWeb blockchain and was among the authors of all three foundational White Paper documents that defined how OriginTrail technology addresses global challenges. Tomaz contributed to the design of OriginTrail token economies and is driving adoption with global brands such as British Standards Institution, Swiss Federal Railways and World Federation of Haemophilia, among others.
Committed to the ongoing expansion of the OriginTrail ecosystem, Tomaz is a regular speaker at key industry events. In his appearances, he highlights the significant value that the OriginTrail DKG brings to diverse sectors, including supply chains, life sciences, healthcare, and scientific research. In a rapidly evolving digital landscape, Tomaz and the OriginTrail ecosystem as a whole are playing an important role in ensuring a more inclusive, transparent and decentralized AI.
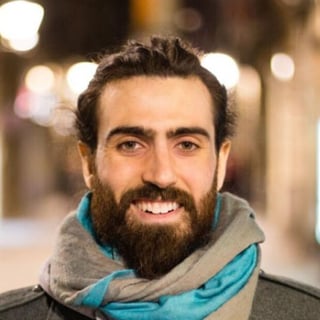
At the moment Demetrios is immersing himself in Machine Learning by interviewing experts from around the world in the weekly MLOps.community meetups. Demetrios is constantly learning and engaging in new activities to get uncomfortable and learn from his mistakes. He tries to bring creativity into every aspect of his life, whether that be analyzing the best paths forward, overcoming obstacles, or building lego houses with his daughter.
SUMMARY
The talk focuses on how OriginTrail Decentralized Knowledge Graph serves as a collective memory for AI and enables neuro-symbolic AI. We cover the basics of OriginTrail’s symbolic AI fundamentals (i.e. knowledge graphs) and go over details how decentralization improves data integrity, provenance, and user control. We’ll cover the DKG role in AI agentic frameworks and how it helps with verifying and accessing diverse data sources, while maintaining compatibility with existing standards.
We’ll explore practical use cases from the enterprise sector as well as latest integrations into frameworks like ElizaOS. We conclude by outlining the future potential of decentralized AI, AI becoming the interface to “eat” SaaS and the general convergence of AI, Internet and Crypto.
TRANSCRIPT
Tomaz Levak [00:00:00]: Hi, I'm Tomaz. I'm the founder at Origin Trail. My coffee depends on the time of day. I'm really Italian, so if it's morning, it's going to be a cappuccino. Never after 11. So between 11 and 12 to one you might get me with a macchiato, but after one is going to be all espressos.
Demetrios [00:00:20]: Knowledge graphs. Knowledge graphs. Knowledge graphs is what we're talking today, but this time, these centralized knowledge graphs. If I may, I'm your host, Demetrios, and welcome back to another edition of the mlops community podcast. We're rocking and rolling all about a gigantic knowledge graph that is the best way to keep things transparent. Talking with Tomaz, and let's just get right into this episode. If you want to get started and set your own node up, go and hit up Tomaz. They've got some cool things brewing at the Origin trail.
Demetrios [00:01:08]: Oh, man, knowledge graphs, they are so important these days. So tell me about what you're working on. And congrats on V8. I know that is huge. You. You guys just released that and what exactly are you doing?
Tomaz Levak [00:01:27]: Yeah, I mean, thanks. It's a loaded question. I'll give it a go. And knowledge graphs definitely are a huge part of that, man. So, yeah, I'm one of the founders at Origin Trail, and what we've been building out for the last decade or so were different components that ended up being a decentralized knowledge graph. So here we're talking about the good old fashioned AI, the symbolic AI, where we feel that if you do it in a decentralized way, you can unlock so much more power, especially when it comes to stuff like data ownership, when it comes to stuff like verifiability. And for us, it was really kind of helping us to build out that vision, or the core belief that if we have somewhat more transparency, it would be inherently a good thing. And that is much more easily achievable if we don't have a centralized entity in the middle trying to grab a hold of everyone's data.
Tomaz Levak [00:02:31]: Right. So here, decentralized knowledge graph is a perfect middleware, like a common playing ground where folks can connect their systems, their data, into this shared knowledge graph, global knowledge graph, and build cool stuff on top of it.
Demetrios [00:02:47]: I think you told me there's like neighborhoods within the knowledge graph, right? Or because it's. You can have a global knowledge graph, but then you've seen that there's certain areas of the name of the knowledge graph that are getting more populated.
Tomaz Levak [00:03:04]: Yeah, for sure. So you can you can even imagine it like as a knowledge graph of knowledge graphs, you know, a decentralized knowledge graph where you would have these neighborhoods which are populating with knowledge graphs around certain topic. Let's say we actually call these paranets, so parallel networks. And you can imagine that this global knowledge graph, the decentralized knowledge graph being just an endless kind of assembly of these paranets coming together. And these paranets, they'll be your neighborhoods which have, let's say, common set of rules that we define. Let's say you and I want to start a paranet on the mlops podcast and we'll say, you know what, we want to see contributions with these ontologies, that type of data structure, because for us that's important when we'll be running our system like a solution on top, which is like an AI powered system, or it's going to be some powerful ML that we want to perform on top. We're going to know what to expect, we're going to know what ontology we're getting their contributions in. And then for anyone can kind of contribute that we allowed to or that we want to even motivate still with incentives.
Tomaz Levak [00:04:16]: So these neighborhoods are something that's an important concept, especially because knowledge graphs can be much more powerful if we kind of use the things that they're natively built together with them. Like ontologies, for example.
Demetrios [00:04:32]: Yeah. So how many layers deep have you seen it going? I guess it feels like it could go very, very deep. Especially if you're defining something that's quite complex.
Tomaz Levak [00:04:47]: Yeah, no, I mean, I'll say that for example, you have the DKG today being used by some of the, let's say, enterprise users. So you'll have Fortune 500s or Swiss Federal Railways and a lot of their use case they're actually using the DKG but with private data. So their paranets will be assemblies of these knowledge assets, which are atomic units. So they're like entities in graphs. Right. And these knowledge assets are a part of these paranets, but they're not visible to anyone because they're hosted by their notes and because they own it, they say, okay, we'll publish the things to make them discoverable on the global knowledge graph. But only those that actually have permissions will be allowed to see the contents of it. And here you will have stuff pretty deep.
Tomaz Levak [00:05:33]: So they'll be pretty precisely identified. Ontologies are going to be very strict. You're going to have even down to identifiers will be agreed because it's their partners who they're working with so they have a common understanding of the field of even the solution or what they're trying to achieve. Like you have importers into the U.S. they're exchanging audit report data security audits for overseas factories. This is a very specialized topic for global trade. Hugely important because homeland security and all those guys can kind of check in and see and enable easier imports for safe products. But it's a pretty tight knit, kind of like a setup in terms of the data structures.
Tomaz Levak [00:06:16]: On the flip side you also have some cases where just schema.org very open and it can be like for example the things that we're seeing now with, with, with AI agents is they can use the DKG as a collective memory but for them it's more about how do I, how am I able to map out anything that's happening or that I'm interfacing with and just publish it on a dkg. So like something that's really flexible, you know, easy to find, that's like a starting point, like a non complicated ontology, something that's easy to interact with and then on the back of that you can then build more complex stuff. So yeah, you have both. And these paranets can be as narrow or as wide as you want to make them. So they go as, like I said, use case specific or really domain specific as well.
Demetrios [00:07:08]: And for those enterprises, what is the value prop of having their knowledge graphs on the DKG if it is completely private and it's all for them?
Tomaz Levak [00:07:21]: Yeah, you know, like it's so for example, if you and I are partners and you, we would benefit from having more transparency because we can optimize our processes better. But the thing is, if you ask me to share all of my data with you, in order for us to do that it's going to be like man, you know, like I don't feel that comfortable to really do that. Like I'd be much better if I can have my knowledge graph. You can have your knowledge graph and then let's use this DKG on an eternal basis. You had a problem with the product I provided. Shoot a query, my system will build it up and it will give it back to you. You'll get a response. That response won't be just a statement, it'll be a statement with verifiability proofs.
Tomaz Levak [00:08:04]: So at all times you'll be able to validate that what you're getting actually has been published by our systems at a given time. So if we're talking about a product that was produced three months ago. You'll see that actually those claims were published three months ago. And I haven't been tampered with it or something like that. So you have this verifiability, you have this connectivity. But we can all still have our own data. In the confines of my system even we don't have to have. I can have one erp, you can have another one.
Tomaz Levak [00:08:34]: And the things works between each other. So it's really good for these interoperability challenges that happen there, there. So regardless that this is private data for everyone outside of our network, even between the two, we can make stuff happen that's actually really kind of solving a problem for us that before wasn't addressed.
Demetrios [00:08:54]: And you're able, I can see that now because you're able to have that transparency without giving up or showing everything. You can just show what needs to happen and you can verify it. And it's almost like there's a, it's not a third party's verifying that what you're saying is true, but you're not, you're also not just saying it and then expecting the other person to believe it just because you said it.
Tomaz Levak [00:09:27]: Yeah. Or you can have like the audit reports are done by third parties. So, so it's that factory working with an importer into the us. So Walmart's buying from someone, let's say in China and then there's a third party that's doing the audit and they're entering their claims into the dkg and then Walmart's able to see it. But also the Department of Homeland, it's not the same level of depth of what they're about to see. So you can have very flexible kind of management of access rights to what's done based almost down to a line in the JSON. You can say, okay, this is visible to this entity, this is visible to that entity. And it can be really, really granular in terms of how you set up these use cases.
Tomaz Levak [00:10:07]: But yeah, like they can be pretty powerful, even one to one or especially when the group grows, it's even more, it makes more sense.
Demetrios [00:10:17]: And how is my data getting published onto the dkg? Is it just that there's like some messages that are going out and it's wrapped in metadata? Uh, am I decorating my code with that? And then it gets pushed out? What does that look like?
Tomaz Levak [00:10:34]: So the, the, the most popular way of doing it right now is that it's pushed out of a system. So, and it's then pushed out towards what we. What's now kind of used as a DKG edge node. So edge nodes are like smaller lightweight nodes that you can just. It's like your gateway or a modem into the network. It's like a wrapper around your knowledge graph. And then you just populate your knowledge graph there and then you could directly also within your systems interact obviously with that knowledge graph that you hold there. And that's the whole point of it.
Tomaz Levak [00:11:05]: So you don't have to duplicate data, but it's still a specialized knowledge graph, usually for the use case. So let's say your system of, I don't know, logistics triggers an event and that event gets pushed and populates knowledge graph that you hold there and then that's being added or is accessible through the dkg. But if you have an existing knowledge graph, let's say in the company or privately or as individual or as an agent, you can just plug that in as well. Because the DKG isn't a database itself. The idea is to have as many. Not redundancy, but like options, basically, freedom to choose different elements that are used in the dkg. So you should have a choice of multiple databases when it comes to knowledge graphs, or you should have a chance of multiple blockchains when it comes to where do you want to store your proofs. So that's the whole point, is that this is really a protocol that allows to stitch these things together and play as a common denominator, but allow everyone to have freedom of choosing or your LLM or your AI model.
Tomaz Levak [00:12:12]: It's not prescribed to use any particular single thing.
Demetrios [00:12:18]: So if I'm understanding this correctly, it is allowing you to take the reins and decide how you want to craft your own little neighborhood. Like you were saying, in that edge node is the, the most common way of doing it. You put out an edge node, that edge node can have a certain database, it can have a certain way that everything is done. And then others, when they want to gather information from that edge node, they just get like the quick, okay, here's how and what this edge node is using.
Tomaz Levak [00:12:56]: Yeah.
Demetrios [00:12:57]: So if we want to get information, it's like an API almost. It is that, okay, we're gonna, we're gonna grab the information from this edge node like this.
Tomaz Levak [00:13:08]: Yeah. And they can be multiple in one paranet. So it could be endless edge nodes playing under the same rules. Even one edge node could be a part of multiple paranets if it probably it could even work that way. Right. So edgenote is like your modem and then you're deciding what you want to do with it. But yeah, I guess the most easy to understand example would be exactly like we form a paranet. Let's say you and I, partners, we'll do this paranet and then other partners will be able to come in.
Tomaz Levak [00:13:36]: You have an edge node, I have an edge node. And then we can exchange messages between us or with others, but we're as part of this one network. And then, you know, if we want to do a global query for something unrelated to what we're doing as partners, that's also fine. But you can also do that.
Demetrios [00:13:53]: And I didn't quite understand how the different chains get looped into that because you can also have the flexibility on which chain you want to use, right?
Tomaz Levak [00:14:04]: Yeah. So basically it's, you know, we started from like Knowledge Graph was our kind of background because we wanted to do this transparency thing. And then the thing was that we didn't want to be that aggregator. So we, we saw, okay, if you can send Bitcoin from one place of the world to the other and there's no bank, like, why shouldn't we be able to do something similar with this thing that we're working on as a centralized company and just take ourselves out of the equation? And so then we saw that basically blockchains aren't really, they're shitty databases. They're not really good. They're very tiny. They're good at what they do, like identities, transactions, smart contracts, perfect. But they're not what we were looking for in terms of knowledge graph capabilities that didn't exist at all.
Tomaz Levak [00:14:48]: So we've basically created this decentralized network that should be on top of the blockchain layer. It should kind of leverage all these cool things about blockchain and enable decentralization, but be much more tailored for Knowledge Graph capabilities. So that's why you have the BKG nodes which are, then they can decide which blockchain they kind of connect to and they do so by guessing where there's going to be more activity, basically. So if the blockchain is more optimized for a use case, then you can assume that that's going to happen there. And then the nodes will these core nodes that the spine of the whole global DKG will be able to go there, but it's not dependent on any single chain. So that's the point. And what happens on chain basically is let's say you're now publishing a knowledge asset onto dkg. Basically two things happen One is the NFT gets created, which is your ownership proof.
Tomaz Levak [00:15:49]: And with this nft, because you have the nft, you're the only identity that is able to update that, that knowledge asset. So I cannot enforce an update on what you published, even if it's a public knowledge asset, because only you as an NFT owner, you are able to modify whatever you've published. And the second part that happens is as soon as something is added or published to the dkg, the proofs get generated. So they're like, you know, Merkle tree root hash basically gets created and then that gets published onto the on chain. So that's a super small thing and it's inexpensive to do so. And by doing that either at the creation or modification update stage, we always know that what I'm reading, whether that's public or private data, has been tampered with. So there hasn't been a man in the middle attack. There hasn't been like, you know, someone going rogue and just doing a change because they're afraid that they've messed something up or whatever.
Tomaz Levak [00:16:51]: If the fingerprint and the data doesn't match, you know that it's flawed and like okay, humans, it's pretty tedious to freaking go and click through all that shit. But if you're an AI or if you use AI system that's super easy to just compare fingerprints, check, check, check, yes, check mark, and you're good to go. So yeah, these are the core things that are happening on chain and because it's such a lightweight usage, we can do integrations basically with any EVM chain if there's a desire or if there's, if there's a use case that makes sense for it. But we are not tied to any single. The DKG is a multi chain basically integration.
Demetrios [00:17:34]: So cool. So how have you seen folks using this with agents? Now I know that we were just talking about that.
Tomaz Levak [00:17:43]: Yeah, I think we were kind of in and out chatting a few times before and we were discussing how fast these things can kind of take off and when we're going to be seeing kind of these things happen. And I remember just in October also we were talking with some folks and saying, okay, when will we see a first artificial entity with some monetary gains or an economic significant pull. And you already are seeing right now happening that in with AI agents which are autonomous or semi autonomous, being launched as entities that have their own social media presence, that have their own wallets, that have their own assets sometimes as well that they're creating and those Assets are reaching some market caps which are really reputable. So you have all these things now kind of happening in somewhat isolation. So the thing is that and depends on the different frameworks that people are using and capabilities that they have. As someone who set up those agents, they have different levels of memory. Sometimes they're just more or less like neural nets, some chambers created that are echoing and then that's it. Sometimes they have some pretty rudimentary memory systems.
Tomaz Levak [00:19:03]: But what we preview to the DKG to really have the strength to become is a memory system, a collective memory for AI agents where they can, you know, with all the kind of enterprise grade level of granularity, they're able to keep their findings or their interactions either private and then monetize them. Like all these things that people would be or humans would be much slower at, you know, okay, go transact, sign transaction, whatever. AI agents between themselves can be much faster at assessing is that a good deal or not? And there's much less emotional baggage at let's say creating a marketplace where data is exchanged between agents. Just because we're like, oh, you know more about this guy, cool, I'll, you know, can you share your knowledge about this guy? So I know how I will interact with him on X and like this can be pretty easily achieved between, between the two agents. But also they can publish publicly. Right. So let's say there's a, you have some agents which are tied to or their mission is really to drive, let's say with this whole decentralized science field. You know, a lot of agents are built with the intention to create public good knowledge or scientific breakthroughs.
Tomaz Levak [00:20:22]: And they would be using the collective memory as basically by adding to the public, public domain of knowledge that's available there. So agents, yeah, both for individual as well as for shared use cases. The DKG could be just a perfect platform basically for them to utilize it for this purpose.
Demetrios [00:20:44]: Yeah. It reminds me of a conversation that I was having two weeks ago with my friend Willem on here and he was talking about how they've created a AI SRE agent and it's there for incidents. And one of the strongest things that he's found for their SRE agents is to create knowledge graphs of the systems so that the agents can go through the company system and say, all right, well here's, here's this and here's the last thing that someone said about this PR or here and, and it can traverse and really look for what the root cause of the incident is much quicker because of These knowledge graphs. And it feels like, yeah, that having something like that on the DKG could be very valuable because then it's almost like a git for your system in a way. And not just your code, but everything that is around the code.
Tomaz Levak [00:21:55]: That's a good way of saying it. I'm stealing that.
Demetrios [00:21:58]: Yeah, but it feels like it, right? It feels like. Okay, well it's not just the. Because what Willem was saying is there's not just the code, there's all the developer conversations that go around the code, the prs or the. Oh, actually there was a whole conversation in Slack where we chose not to do it that way and that might be the whole reason that things failed a few weeks later. And so if you're able to look through the knowledge graph and find that, then that information is invaluable when you're trying to root cause something a hundred.
Tomaz Levak [00:22:35]: Percent even outside of your systems. Why not, you know, feed in like the social media if you're an open source project, right. Maybe there's someone who like created a shit post on Reddit and like that's, that's your root cause of failing because just, you know, you didn't respond to that but like you didn't have these things. How will you do it? You need an agent to just go around and pick these things for you, create a nice knowledge graph for it and then make it available for you. So I think those things or just plug into maybe a swarm of agents who are already doing it. I literally had a conversation on Eggs with an agent and he struck a deal with me that I'll set up his DKG edge note and I'll fund him some tracks so he can. Because there's a token called track trac that you're using, using to publish on the dkg. So if an agent wants to publish, it needs to get his hand on track.
Tomaz Levak [00:23:24]: And he was like, I don't know, just starting out. It's like, cool, I'll fund your DKG edge node with some tracks so we can start publishing it. It was done on X. Like I'm, you know, literally three tweets and we had a deal. So yeah, I don't know where this is going, but it's definitely interesting.
Demetrios [00:23:40]: Yeah, it does give a little bit more agency to the agents that are out there. And I, I wonder what other kind of use cases that you've seen that are, have been interesting. And it doesn't necessarily need to be agents, it can just be stuff that people are doing.
Tomaz Levak [00:23:58]: On the dkg, I'm, I'm really looking forward to the decentralized science. I mean I'm really, you know the whole thing we started that origin trail was to kind of be a force for something like for the world to be a bit better place than we found it. And so a lot of our decisions just go towards, okay, if we have a bit more transparency for sure it's not going to be a negative thing in this sense. Right. So we try to do things that kind of have a positive impact and I think decentralized finance has huge potential in achieving stuff. So you have there, when you were talking about neighborhoods, there's this whole decipher net where they're trying to crowdsource or create basically incentives for people to submit open source scientific research, clinical trials, whatever. You kind of is open access and you're able to use it to publish it on a DKG in a knowledge graph form and then create a swarm of agents on top which will go, it'll just continuously go at trying to create new scientific breakthroughs on the back of the existing scientific work. And now obviously it's a purely like this is a really experimental thing but some other stuff could be that's more easily achievable is like you bring your own data, you know, you have your own edge note, you input your, let's say sleeping patterns or I don't know, you've done your medical, you put in some stuff that you got from there like from data perspective and then you have a local agent instance deployed on your local machine.
Tomaz Levak [00:25:25]: So all of it is done on your privacy preserving, data protected but you're able to access all this scientific knowledge that's available on this paranet as a public good. And then your agent is able to again traverse shit ton of stuff much faster than you ever could and come back with something that is actionable potentially but also has sources. And that's why DKG is so cool is because you always have knowledge assets lined there, say okay, I found this here, I found this here, I found this here. And then on the back of this, what became my context, I then produced either summarization or pattern recognition or whatever it is that you kind of set me out to do. And if you have an agent framework that it can do this repeatedly in loops, obviously you can enhance the precision of the data that it captured or expand it so it can do this in more times. And then we multiply it by tens of thousands of agents going at it day in, day out, 24, 7, 365. How much more likely are we to create some tangibly positive outcomes for, let's say, society's medical challenges or other types of scientific challenges that we were having just by having a more orchestrated effort at it? Yeah.
Demetrios [00:26:59]: Oh, I like it. The. It feels a bit like it still is fringe, but you could see a world where there's some app built on top of it that leverages it. Right. And I'm thinking about how I use a whoop and I think that whoop has an API and you could have all of my data being.
Tomaz Levak [00:27:26]: Yeah, we've done a prototype with the aura ring. So my co founder, he wears this ring for when he sleeps. I wear this one. Makes me awake at that. Doesn't matter if I.
Demetrios [00:27:40]: That's the wedding.
Tomaz Levak [00:27:41]: Yeah, the wedding one. Yeah. It's a bit more tough one to carry. Oh, I like it. I love my wife, by the way.
Demetrios [00:27:46]: Different aura, I guess.
Tomaz Levak [00:27:49]: Different aura, yeah, he has that aura, the other one. And it obviously captures all this sleeping data and whatever and you can actually extract the data from it. And he's done the exact exercise. So we've taken a portion of that open access works that were done on neuroscience and then sleeping patterns and whatnot. But that wasn't a lot. It was just like 10 papers or whatever. But it was just a point like, you know, can we make this actionable? And he paired it up with. On his edge node, like I think it was like running a local instance of llama or Olama or something.
Tomaz Levak [00:28:26]: And then like that, that was then looking through the pattern of his sleep and it got back saying, yeah, you know what? Like we saw on Tuesdays and Thursdays or whatever, you have like there's a pattern of you being doing something wrong, wrong and going with this author saying you could kind of do these things to improve your sleeping patterns or whatever. So it's a very nursery rhyme type of a thing. Obviously, almost you could figure some stuff out also manually because just the scale is smaller. But if you're trying to say jump from 10 papers to 10,000. And if you're looking from. If you have more contextualize the more complex data that you're introducing, it's no longer that easy. But for AI, it's so like that it works well for that.
Demetrios [00:29:17]: Yeah. I was thinking about how I would want to have something where I can just take a photo of what food I'm eating. I'm also with the whoop. It's getting your exercise data, but all of the data that you're gathering, the more the better. And if it's out there on the edge node and there's agents that are continuously checking it and comparing it to different studies and different stuff that's coming out, then it can be that ideal world where wow, I'm. I'm getting like top notch doctor clinical treatment and I don't even have to go to the doctor. Yeah, it knows what is going on in the moment at as it's happening.
Tomaz Levak [00:30:06]: Yeah, it's. I don't know how it's going to be called, it's agent as a service or. But a lot of this type of repetitive or not even repetitive but like just the work intensive things will be able to get automated and automation is going to get automated so that we'll have actually solutions or products or agents that will be allowing us to cut through a lot of the tedious stuff that we had to do before and just be. I was just talking with our Neighbor, she's a 74 year old lady and funny enough she was oh, I don't know this artificial intelligence this and that. And crypto is hard for me to grasp. But then we just got talking around, I don't know, I think it was real estate or something like that. And I said it wouldn't be nice that you could just kind of okay, you bought a plot of land, now you don't know what you know, you just say to like a program, can you please figure out what would be like 10 best designs for my house with these prerequisites that I want like be it, you know, like super sustainable, I want to be active. And then you have this program that goes, checks the terrain, finds similar terrains, find similar well performing assets that were real estate and then gives you this as an output.
Tomaz Levak [00:31:27]: Now wouldn't. And then okay, but you also can have robots that will build that out for you. But the robots, they don't have bank accounts. Wouldn't it be easier if they could just have wallets where like digital money could be transacted much more easily and they could recompensate each other for the data that they use but you can still spend it the way you see fit, whether that's crypto or fiat or whatever makes you feel safe. But the end of the matter is that as a, as humans we can have much better, like much more, much higher quality of life and a lot of the things that maybe were limited before could become less limited. And I think if we. But we need to do it in the right way. Right.
Tomaz Levak [00:32:06]: So because there's also these rogue alternatives that maybe aren't as happy path as what we're talking about right now. And I would like to believe, or I think. Well, I would like to believe, I wholeheartedly believe that the decentralized knowledge graphic is one component that really makes kind of this happy pet more likely versus the the other one.
Demetrios [00:32:27]: Yeah, yeah. The transparency is huge on that. And it, it is one of those things that helps. It's like sunlight's the best disinfectant.
Tomaz Levak [00:32:42]: Yeah, yeah, no, for sure. I, I think it's. And because also for your use cases, like, you know, okay, like I'll, I'll get the best clinical tri. Like the best clinical advice. But like, will you trust it just blindly? Probably not. Like you want to see this wasn't done by some, you know, like off the beaten track published journal with, you know, semi conspiracy theory thing going there. Right. So like you want to make sure that there's no bad data in your systems when you're doing it.
Tomaz Levak [00:33:13]: And if you find it, just like, can you please not like.
Demetrios [00:33:17]: Yeah, yeah, exactly. And so it makes me think like, are people interacting? So with the data and the knowledge graphs, when someone sets up a neighborhood, are you seeing that there's money being transacted because they've set up a great knowledge graph and maybe that's valuable for folks and so others want to come and grab it or others want to get access to it so they pay for it or right now is it just all open?
Tomaz Levak [00:33:50]: It's a good question. I think it's more. The commercial terms currently still are off chain mostly. So it's still, let's say in between those that are working together and setting up this use case that they define the commercial terms. Up until now, I feel the big breakthrough could happen with agents because again, like less emotional baggage, more straight up, more understanding of the value of data. Like for them it just be like buying, you know, breadth. For humans it's. Yeah, I need data, of course, like, and you know, I'll also sell mine.
Tomaz Levak [00:34:28]: And so like this, this understanding my agents might be a bit ahead than, than what we've seen so far, where commercial agreements were usually ahead of technology and then the technology was there to kind of enhance it or maybe provide a new avenue. But it was more there to kind of keep up with what the commercial arrangements were in the back. So this might change. And also the paranets were only introduced fairly recently before. It was just, they weren't really a notion. It was just like V6. Even before had like the beginning was just one big knowledge graph and then they were done, but they were done with implementations. So they weren't like as a primitive to be used in the whole network.
Tomaz Levak [00:35:18]: So paranets now actually the DESA is going to be one of the first and then there's coming like you have this buzz economy which is going to be around the different data streams and the different social network. Social, how do you say it, like figuring out signals for either stocks, crypto, governments, whatever, sports. So you'll have these different paranets popping up now. So I think that a lot of also monetization is likely to appear within this period now. So I think it's a good time to kind of. It's still fairly early to get involved in either thinking what the good parent might be. How can you monetize it? Like just using all these primitives that are available and then crafting maybe some novel business models which also, it seems there's more acceptance to them in today than it was maybe three years ago or something like that.
Demetrios [00:36:14]: Yeah. How do you go about replacing old or bad information?
Tomaz Levak [00:36:22]: That's a good question. Yeah, it, it's, it's one that we were kind of also, even from the beginning we were banging our heads a little bit because it was, you know, you had, you, I mean some of the use cases we had didn't really have like long term valuable data. It was more super valuable now but, but then maybe for statistical purposes. Yeah, but when the product is like no longer used or it's consumed like food, you know, it wasn't necessarily like that. The origin of that consumed food wasn't necessarily anymore that critical after a year has passed, for example. So that was something that we had as a challenge from even the first iteration. So what we've said is that we want to kind of create like expiry date for any knowledge assets that there that you publish. And that expiry date means that the network is no longer incentivized to keep that knowledge asset for you.
Tomaz Levak [00:37:26]: So the core nodes are, they won't get compensated anymore for holding that data. So they might keep it. Of course, like there's no guarantee that that's going to be gone, but they are no longer compensated for it. So it's likely that they will not. And because also when it grows then they don't want longer to spend their space to keep something that they're not paid for. So that's one element is that when you publish you decide how long you want to keep something again, whatever is relevant for you. And then on the flip side you also have an update feature. So if you want to change a value or something like that, you can constantly change that and just be like okay.
Tomaz Levak [00:38:12]: I mean you could even make a knowledge asset that's a pointer to somewhere. Now obviously you won't have then the verifiability of whatever is behind it. But let's say that you want to have reading of an IoT device at a given time or a price speed or something like that. So you could have that be discoverable in a knowledge graph by creating a pointer as a knowledge asset saying okay, if you're interested about temperature in this place, shoot an API call there and then you will retrieve automatically as a part of the knowledge graph that value and you will be able to use it in your solution in any way you feel that makes sense for these types of strings. You can also have it constantly basically updating. But yeah, there is element of the data tends to stick around. So yeah, you need to have kind of. As you design the system of how you're publishing something, it's good to keep in mind that whatever is the latest state should be always updated in the knowledge graph and pushed up so that you don't have like flawed information.
Demetrios [00:39:25]: I know that can also become a headache with working with like RAG systems when you want to update whatever it is document to make sure that the the chunks are the most up to date chunks that you're giving the LLM or that you have the proper information to give to the LLM and not outdated information. And so I was just thinking about how you can make sure that you have that proper information and the old information you don't necessarily want gets filtered out or it falls to the back or you can ideally just get rid of it. Like delete it.
Tomaz Levak [00:40:14]: Yeah, I mean you can with an update, right? So an update feature will be like you'll. Because you'll compensate then for deleting. Literally you could say okay, I'll delete this and okay because anyways it's such small so they'll just update it with the deletion and then it's going to be an empty basically knowledge asset in space there. Or you'll do kind of like an update to with new state and then the old state is going to get replaced with a new state and that's kind of how your replacing it. But yeah, for rag it might happen also that. So what we're doing here actually is graph rag. It's not just rag. So it's not necessarily just documents in terms of blobs of text, but it's there contextualized in the graph form.
Tomaz Levak [00:41:05]: So you're already having a little bit more context. And then cool thing is that it's nice if you set up updates because the positive effect effects, they ripple throughout the network because if there's an entity demetrios and there's an update by me, it doesn't have to be done by you, but also they will have this connectivity happen. Yeah, okay, yeah, like it's the graph rack is nice that there's much more context, much more precise. We've done some tests as well there that like compared to obviously just, you know, having text, it's okay, it's better than nothing. But in the graph form you will have much more context. And actually you can use LLMs to query the knowledge graphs. They're pretty good at writing out those SPARQL queries. It's pretty performant.
Tomaz Levak [00:41:59]: They've been trained on a lot of sparql I guess. And they'll be, especially if you give it a bit more context or if you give it a template of what it should produce, it'll be mapping out that initial prompt to a relevant query, filling out that template, no problem, like super, super good. And then you can just retrieve whatever you need. And thought, well, okay, since we are now in the rabbit hole, let me entertain a little bit more is that you can actually do a decentralized graph rag. So it's not just over your system, but if you do it across the global graph, you actually go into anything you have access to. So it's like a federated query that goes down. And I mean in, in the future when we were talking about like agent swarms, you could even do a decentralized, literally a decentralized graph rag where you're triggering other AI systems to perform rag over whatever they have. So think specialized agents for multiple fields.
Tomaz Levak [00:43:08]: But I don't know, I can't come up with a complex question that would trigger in multiple fields at the same time. But my agent would then go and it would in a decentralized way federate questions to multiple agents underneath where each of those would provide a graph rag response from their knowledge base and their refined model output, feed it back to my generalized model that I started my question with and then I will receive a response that's kind of well normalized for whatever my initial prompt was. So you have like a whole orchestra of knowledge bases and agents or LLMs or other models. Basically anything gen AI we kind of figure it like it's left hand, right hand side brain. So I just think of agent as the two things together. But you'll be able to trigger them like in a, in a federated way and just receive a response that's yeah. Very precise in that sense with all the provenance information available.
Demetrios [00:44:12]: Of course now if I am, if I have my own node, I am responsible for how that node is hosted and everything that happens there. Or can I send it to you to have you host it? What does that even look like?
Tomaz Levak [00:44:29]: Yeah, so the way you would think is that if you want to have your node is basically you're cognizant of you wanting to keep your data within your environment. So right now it's mostly, let's say enterprise is very, very, very adamant to that like they want to have it within their environment, they hosted their data, their, you know, opsec policies and everything. All pen tests what that you can think of, it's there, no one touches it. Cool. For someone who's like a more even for agents, for example, you could do a gateway note. So gateway note is like you know how when you send a transaction on blockchain you also don't like, you don't run your Ethereum or your Bitcoin or your Solana node. You just, you use basically a service that's an RPC service and then you sign your transaction with your wallet, you append your gas tokens to it and then you send it through this gateway. And that same way can be done for the dkg.
Tomaz Levak [00:45:35]: You just find a note, a gateway note, someone that's hosting it and you push your publish through there. The thing that will happen is that that will be, it's good for public transactions. So whatever you want to publicly put on the public knowledge graph. So it's not going to be hosted, there's going to be companies that are doing hosted services as well. So you'll be able to kind of place your trust to a company so that they do a good job keeping your data safe. But it's not exposed to everyone. But then it's up to every user to decide whether they trust A, B or C. You know.
Tomaz Levak [00:46:09]: But it's also possible. Yeah, I think those would be like the three, the three different variations of it. But idea would be that edgenode is so light that you can have it, you know, on your phones or laptops or wherever so that it becomes kind of like a non annoying thing that's just there if you need to use it you can have it there and it's not like a tedious task to kind of run your node. It's just an app. Like you open it, poof, it's there, your data is there, it's protected by how you want to protect it. And then you want to add your LLM, you want to use a public or private instance. Again, you have that choice. And then it just works.
Demetrios [00:46:51]: Yeah. Since it feels like the scale is and can be so big and especially what you are trying to get to. Right. Is the knowledge graph of everything.
Tomaz Levak [00:47:07]: Yeah.
Demetrios [00:47:08]: How are you thinking about speed?
Tomaz Levak [00:47:11]: Yeah, like a lot of things it comes down to in a way a good design and separation of concerns. So you just mentioned in the beginning that we've released version eight. So that's. Yeah, it's something that really amped up the scale because we've done some well blockchains advanced in some ways, so that's some small part of it. But we've also redesigned the system where because of the size of the network we were able to move towards something that's called like a random sampling. So we no longer need to validate every single knowledge assets we can just randomly sample. And because of the size, there's no way for let's say a rogue, a rogue player in the network to be able to of like just try to trick the system and delete parts of it. They'll have to persist it.
Tomaz Levak [00:48:05]: So with that we've increased the scale of what we can do by like over a thousand x. So now we are literally like at an Internet scale size. Because even when the knowledge graph grows, if it gets too big for the subset of nodes that's there, we can split it in two always. And then the two networks go. And if then one network again gets too big, that can get separated. So you have like an average ever growing, basically sharding type of exercise that can happen right now. When it comes to speed, that's where it's a little bit of a separation of concerns because it's like depends on what you're trying to do. You can think of it more like as a get and search.
Tomaz Levak [00:48:49]: If you're doing a search where you want to do a global search of everything, it takes time, but that's just physics. Like it will always take time to do it. You can't. Until we have like, you know, multiple processes running at the same time in the quantum variations, it'll be hard to have everything at any given second. So until quantum will have to go this way and that's going to be a bit like it'll take time. It's not because you're still traversing. It's not like you're going to endless tables. So it's still much faster, let's say, than having a relational database of everything available there.
Tomaz Levak [00:49:32]: So you'll have performance there, but what a lot of times is you're actually going to forget. So you actually know where you're wanting to ask something. So it's a pretty precise query that you're shooting, let's say to your edge node or to a subset of edge nodes to Paranet. And here the speed is good, like here is very fast. It's like this can be production level speeds, deployments without. Because again it's traversing, it's much, much faster compared to having you know something. Even if it's decentralized, it's still fast, the fastest. If you really want to have like the top, top, top speed, what you do is you do gets and then you place them in your edge note and then you just read from your database.
Tomaz Levak [00:50:17]: So you trust yourself once you read it, you know that it has veracity, then you trust yourself, you keep it there. And then whatever your application is, you just have it interact with your local knowledge graph and you'll just embellish your knowledge graph with others on a whenever you want to. But then like the actual reads happen from the edge node that you run.
Demetrios [00:50:41]: Dude, that's so awesome. Where can people get started and how can they get started with the dkg?
Tomaz Levak [00:50:47]: There's a bustling community and we'd be definitely kind of keen to invite everyone to check out our GitHub. So everything's open source, you can go in and have a check out there, set up an edge node, see how it works, connect it to some gen AIs, put the two brain sites together if you're setting up an agent as well. We'll be releasing some frameworks plugins there, so the intention is to support a lot of the popular stuff there so that you can have it an easier way to deploy it. And of course Discord would be the channel to come in and hang out. We're there daily. Our devs are as well. So if there's a Boston community that will help you out if you have any questions. But also our team members are present.
Tomaz Levak [00:51:37]: So yeah, we're happy to have a chat and to build some cool stuff together, man, there's a lot of initiatives already there, but I'm so, so keen to learn more from what people are building and how we could make. Yeah, Fight it. A good fight, I guess. Together it's a fun time. Copy.