Sign in or Join the community to continue
Data Selection for Data-Centric AI: Data Quality Over Quantity
Posted Oct 10, 2021 | Views 622
Share
speaker
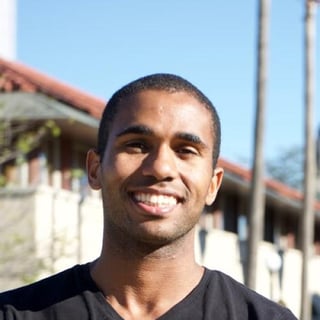
Cody Coleman
CEO @ Coactive
+ Read More
SUMMARY
+ Read More
Watch More
Driving ML Data Quality with Data Contracts
Posted Nov 29, 2022 | Views 2.6K
# ML Data
# Data Contracts
# GoCardless
Data-Centric AI Means Centralizing Training Data
Posted Nov 10, 2021 | Views 600
# Computer Vision
# Presentation
# Health Care
# v7labs.com
Solving the Last Mile Problem of Foundation Models with Data-Centric AI
Posted Apr 18, 2023 | Views 2.1K
# LLM in Production
# Foundation Models
# Data-centric AI
# Snorkel.ai
# Rungalileo.io
# Wandb.ai
# Tecton.ai
# Petuum.com
# mckinsey.com/quantumblack
# Wallaroo.ai
# Union.ai
# Redis.com
# Alphasignal.ai
# Bigbraindaily.com
# Turningpost.com