Sign in or Join the community to continue
Databricks Model Serving V2
Posted Sep 30, 2022 | Views 1.1K
# Databricks
# Deployment
# Real-time ML Models
Share
speakers
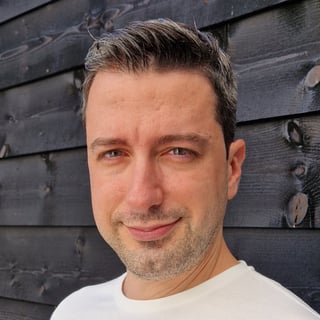
Rafael Pierre
Solutions Architect @ Databricks
+ Read More
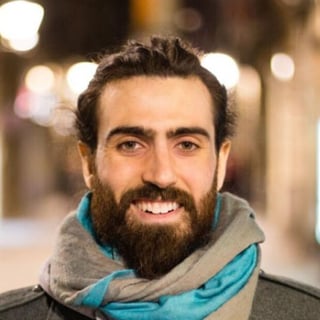
Demetrios Brinkmann
Chief Happiness Engineer @ MLOps Community
+ Read More
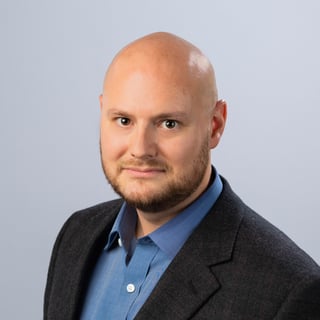
Ryan Russon
Manager, MLOps and Data Science @ Maven Wave Partners
+ Read More
SUMMARY
+ Read More
Watch More
Declarative MLOps - Streamlining Model Serving on Kubernetes
Posted Apr 18, 2023 | Views 716
# Declarative MLOps
# Streamlining Model Serving
# Kubernetes
Introducing DBRX: The Future of Language Models // [Exclusive] Databricks Roundtable
Posted Apr 12, 2024 | Views 1.1K
# LLMs
# DBRX
# Databricks
# Databricks.com