Democratizing AI
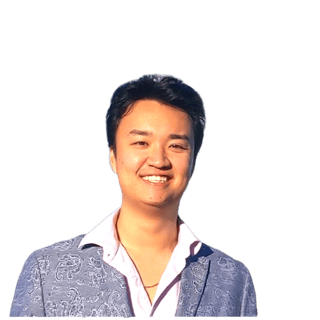
Yujian Tang is a Developer Advocate at Zilliz. He has a background as a software engineer working on AutoML at Amazon. Yujian studied Computer Science, Statistics, and Neuroscience with research papers published to conferences including IEEE Big Data. He enjoys drinking bubble tea, spending time with family, and being near water.
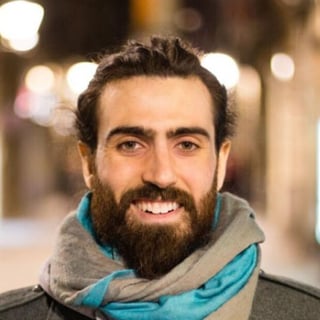
At the moment Demetrios is immersing himself in Machine Learning by interviewing experts from around the world in the weekly MLOps.community meetups. Demetrios is constantly learning and engaging in new activities to get uncomfortable and learn from his mistakes. He tries to bring creativity into every aspect of his life, whether that be analyzing the best paths forward, overcoming obstacles, or building lego houses with his daughter.
The popularity of ChatGPT has brought large language model (LLM) apps and their supporting technologies to the forefront. One of the supporting technologies is vector databases. Yujian shares how vector databases like Milvus are used in production and how they solve one of the biggest problems in LLM app building - data issues. They also discuss how Zilliz is democratizing vector databases through education, expanding access to technologies, and technical evangelism.
My name is Yujian Tang. Right now I'm a developer advocate at Zillis. I'm actually drinking coffee with milk right now, but when I go to coffee shops, my favorite thing to order is two shots of espresso with sparkling water over ice, and a little bit of cream. What? Caramel shots? Yeah, usually caramel shots.
Two shots of espresso over ice. With sparkling water. Yeah, with sparkling water. Before. Sparkling water In the coffee. Yeah, yeah, yeah. It's actually this Moda coffee. Yeah, it's really good. If you put a little bit of caramel syrup and like some like cream or like milk in there. It's, it's, oh my god, there's not a special name for that.
I don't know if there's a special name for it, but this is what I've been ordering for the last I saw it, I saw it at this place called Espresso Vivace or something like that in Seattle. And then I haven't seen it at the other places, but I go and usually if I, if I order it, like they'll have it like I don't think Starbucks does it, but some places, some mini like locally owned coffee shops here in Seattle, they have sparkling water and so they'll make it for you.
Hello. Hello everyone. Welcome back to the ML Lops Community podcast. I am your host Dimitri Os, and today I'm flying. So again, y'all are probably thinking that. People are starting to not like me, or I must not have any friends because I've been doing a few of these solo podcasts recently, and it is not true.
I'm just gonna refute it right now. I do have friends and I could ask them, but I've actually been liking doing this solo thing. I am gonna be straight with you right now because I always would have co-hosts come on because I was always insecure that I was not able to go sufficiently deep enough in my questioning to actually provide value to you, the listener.
However, now I tried it and I feel like I was all right. I feel like I could go deep and I know a few things now. I mean, let's be honest, it's been three years. I've been doing these at least twice a week for the last three years, so hopefully I picked up something along the way. But as always, if. You have strong thoughts on if I should be having co-hosts come so they can go even deeper than I'm able to go.
Please let me know in the comments or in the reviews or all that fun stuff. I love hearing from you all. It is a pleasure being able to do this. I have the most fun interviewing all of these great minds. And speaking of great minds, let's talk about our guest today, Eugene Tang, who currently is a developer advocate at Zli Zli.
You probably know because vector databases are blowing up their exploding on the scene right now. And Zli is the parent company that keeps Milus in check. Milus is one of the OG databases, vector databases out there, and they are built for scale. They are absolutely just beasts. You've got some really big companies that are using them and.
These companies, they, they got scale. All right, so let's, let's talk about that now. In our conversation today, we talked about vector databases. We talked about the democratization of AI in this L l M world and how vector databases play in that. And we also mentioned all different kinds of ideas around the best ways to fully utilize your vector database if you are using it in conjunction with a good old, large language model.
Now there is some very hot topics like chunking and what is it? Dense vector representations. Sparse vector representations. And so we go into that a little bit and we also just talk about zi Liz, and what they've been doing and how they have now. Vis light, and since we are releasing this today, there's also ZI Cloud.
So there's easy ways for you to get up and running with a Vector database. And if you wanna choose ZI or vis, it is very easy and I gotta give 'em a shout out because they were kind enough to sponsor this episode and because they're sponsoring this episode, we are able to continue to bring you some absolutely amazing content in the ML Lops community.
It helps us a ton for them to be sponsors because we get to do stuff like have meetups all around the world. Even if meetup.com is costing me an arm and a leg, seriously, if anybody knows any better alternative to meetup.com, please tell me. I've tried Luma, but you don't get the whole like notify people when you have an event.
I mean, meetup.com. Seriously. How do you sleep at night? That's what I wanna know. How do you sleep at night? Oh my God. Anyway, let's get into this conversation about vector databases before I fully derail it by talking about meetup.com. Anyway,
If you can just share this episode with one friend who you think would enjoy it. All right, let's get into it.
Oh, man. What is this? You tried a hundred different snacks in the last two years.
So. Wait, what are we talking snacks? Like chips or like guacamole? Is that a snack? Hummus? Like single packaged, like goods that are like packaged for like single, single, single person, like consumption, kind of like a, like Justin's like peanut butter cups, huh? Yeah. Yeah. Like those things.
Or like the Kauai, co k k O I A, I don't even know how to pronounce it. Like the, the drinks or energy balls. Yeah, yeah, yeah, yeah. Or like the, there's like these like coca truffles that have some sort of coca, lavender honey stuff in them. They're like pretty, oh my god. It sounds amazing.
Yeah, they're, they're actually, they're, they're, okay. There's, did you record on TikTok your response too? So you remember this recording me eating these I have it on the account somewhere. I just I just got too lazy to, to continue doing it. Cause I was like, okay, like I just can't be on so many social media platforms and oh I got a new phone.
I don't have TikTok on my new phone. Cause I was like, all right, I don't want to be on TikTok. So I'm just, just getting rid, get rid of it. Yeah. It can definitely take up a lot of your time. So you're working as Liz right now, your developer advocate.
I mean, you've been doing all kinds of cool stuff with vector databases. Tell me a little bit more about your trajectory and how you got here. Yeah, I guess, I guess I can just start kind of at the beginning, or after I graduated college, I was working at Amazon for a while. I worked on the auto ML team, so I did I built like this, like the infrastructure side for machine learning models.
And then I left Amazon to kind of start working in startups. I started a couple of my own, like companies. The first one that I started, I like created this app. It was like around like some sort of like data aggregational kind of stuff. I got a few thousand users on it, but I never really figured out how to monetize it. Yeah. So I, I shut it down and that was when I started working in startups. And I've, I've been to, I've bounced to a couple different startups kind of doing this kind of developer advocate kind of stuff.
This is my first role as like an official developer advocate. Before it was like, like full stack engineer or some, some stuff, stuff like that. And then or like technical consultant or, something like that. Basically like I, I decided at one point that I kind of enjoyed this kind of content creation stuff and I started like writing my own blogs.
So last year I wrote a blog that got like 200 some thousand views last year. Nice. And then yeah, I, I, someone reached out to me as I was like shutting down like my second company cuz you know, I was like, okay, I figured out how to monetize this one, but no, like product market fit, so, you're not really making, you're making like a few hundred dollars a month.
So I was like, all right, I can go do something else. Yeah, that's hard. And then they were like, Hey, there's this company who's working in this really cool space. And I looked into vector databases cause I'd heard about vector databases before. But I, I never really knew, knew a lot about it.
And then as I poked into it, I was like, oh, this is like something that I think really has a lot of potential to be like an integral part of Stacks. Especially for, well, especially for the large language model applications. Um mm-hmm. And then kind of just seeing like the other applications of it for anything that it requires semantic similarity.
It's just like a very easy way to kind of, be searching through those documents and stuff. And so I was like, this would be a really cool experience. And I met the team and I was like, wow, these people are all really smart. And then I, they were showing me the architecture and I was like, taking a look at this.
And I was like, oh, this is like super cool. And so I was really excited to to just kind of like get on board and here we are. Those two companies that you started? The first one was around data aggregation, was the other one around data in some way too. It was around it was a natural language processing api.
Oh. It was like a tech space, like processing api. So I was already like in the space of doing like this kind of like tech space kind of like stuff with like natural language processing. And so it made a lot of sense to me when like vector databases were explained in the context of semantic search.
Mm-hmm. Yeah. You had been stewing in it for the past couple years and probably felt a bit of the pain that Vector databases can solve and, and solve for. Yeah. So let's talk about that a little bit for the uninitiated. Vector databases have blown up in the past, I would say six months. Basically since chat G P T came on the scene, they've been the unsung hero of this whole generative AI wave.
Why is that? I really think that I mean, like prior to this, it's not like vector databases weren't being used. They were being used for some of the more traditional use cases, like the semantic search stuff and or, or like image search or, product recommendations.
But kind of the, the wave of LLMs has really brought up vector databases into more of a mainstream kind of topic, I guess. And the, the real reason I think for that is just because there's so many data problems with LLMs. LLMs are, so, we'll take GPT three th or 3.5 whatever, for example, right?
It's only trained up until a certain time. So it's only trained until September of 2021. So, if you, in 2023, you want to be using an L l M app and your backend is like G P T three or 3.5 or something like that. You're only getting access to. DA data that is almost like a year and a half outta date now.
And the only way that you can kind of inject, you can, the only way you can work with these with modern data is that you inject the data. And the other thing is for like enterprises, they want data that use, they want to be able to interact with their data. And so they need some way to, to do, to do that, basically you need some way to inject this data layer.
And that's really where Vector databases have been really popular is that they allow you to, to inject them up-to-date data, inject your domain data and if you are, work, if you're interacting with chat G BT or G B T, whatever, a lot LLMs a lot, it's gonna be costly. And there's going to be some things that are FAQ kind of stuff.
Especially if you're working on like a large system. Let's say you're at a call center. You're gonna have customers who are gonna be asking some of the same questions. And so, if you have some sort of chat bot and a lot of customers are asking the same question, you're gonna want to cash some of those queries so that you can return back your answers without having to ping the LLM again.
Yeah. So these are like the three main use cases that I, I see factor databases being used in for LLMs, and I think that's why they become super popular is just because if you're building an L LM app at scale, there's almost no way that you're not using it. Vector database. Yeah. Yeah. And this is something fascinating that I think about a lot that trade off of when you use a Vector database and when you just try to stuff everything into the prompt and how you look at that, because I know that anthropics.
Context. Oh yeah. As you're, you're able to throw so much more into the prompt and you just raised, the great issue is that, hey, it's not only the stuff that you're putting into the l l M that you're grabbing from the Vector database, but also when it comes out of it, you can go and save it in there and cash it in.
Therefore, the next time that you ask that same question. Right. But how do you see that? Because I think that's something that is coming up more and more okay, where's the role of the vector database? If we can just put millions and millions of documents into a prompt eventually. Yeah, that's, I mean, that's a good, that's a, that's a good question, but I, I think the main thing there is do you really want to be expending a hundred thousand tokens every time you talk to philanthropic?
Or every time you talk to Claude? I, I don't know. I think it's pretty can you do that? Yes. But I think it's a cost issue and. For this LLMs, I mean, vector databases is, helps solve part of that issue. But what you really need also are other tools or frameworks around it.
Some of these AI like agent building frameworks like Auto g, bt, or LAMA Index or Lane Chain, are really offering some tooling around that to make sure that your prompts are reasonable or that you're only sending like the right amount of info to your prompts. So I was working with Lane Chain recently, and Lane Chain has this thing where they limit the number of tokens that you send to, to the prompt to kind of keep your costs down.
But I, I think that, the main, my main kind of thought around that is just, you probably don't want to be using a hundred thousand tokens every time you talk to Claude or you're gonna only talk to it like three times a month. Right? Yeah. You're gonna have to have a damn good reason for talking to Claude if you're gonna be doing that.
Yeah, it makes complete sense. It does seem like costs can skyrocket if you are hitting that limit. Continuously and Right. So there is there is something interesting too that you were mentioning before is how it's almost like you have these large language models and we talked with Neils at Cohere a while back Sure.
And he was mentioning how difficult it is if you want to make a large language model, understand something from the present, like someone died. Yeah. And you have to show them so many different. Examples of this person dying. To update the knowledge that a large language model has every time the world around us changes. And it feels like that's why Vector databases can be slapped on top and you can say, all right, cool.
And let's, let's look at what we have in the vector database first, and then we can go and query the large language model. And one area that I'd love to explore with you, and I'd love to hear what you've been seeing, is these newer models that are coming out that have been O open sourced and they're smaller.
Okay. It feels like you can lean on a vector database a lot more than we originally thought. Mm-hmm. Have you been seeing that, or how have you been like noticing the performance and as opposed to I mean, obviously GPT four is a beast in its own and it does its own thing and it's very hard to find something.
In the open source world right now that is as good as GT four, right? Yeah. But I feel like what is going to start happening more and more is this idea of, hey, smaller models leaning on vector databases more. And we're not asking it these crazy complex things. We're just asking it these things that we know it can do.
Right. Yeah, I think that at the heart of the, at the heart of machine learning models in general, and like, why is it so hard to retrain one of these models? Why do you have to show so many examples? Is that, large language models are still neural nets they're all statistical models, right? They're all using some sort of, they're initialized with some sort of weights.
They're given this data and the data really, this is why, they're called 'em training neural networks, right? Like adapts to the data. And so when a neural network, for example, I think the, the you, the, the point that you brought up about having to show a lot of examples of the queen is dead is like a interesting point because we as humans, like we hear oh the, the queen of England, she like, she's passed.
We kind of immediately are like, okay, she's gone. I think that's because I guess this is a bit more philosophical, but like humans kind of have this like inherent built in maybe feeling or knowledge of like time and like death and machine learning models don't. Really, I don't think they fully grasp the human mortality.
Yeah. And so we as humans, we hear that and we're like, okay, that's fine. But you tell the language model and it's a, it's a statistical model. So it says, well, based on my data, here's 3 million points that say the queen is alive and here's one that says the queen is dead. So I think the queen is alive.
And like the whole like fine tuning thing, this is the problem with fine tuning is you, if something like that is a major update or, if if Microsoft buys open AI or some, some like major update like that it's gonna need a lot of examples. And that's why the whole like vector database thing is, is interesting, right?
It's like why you have, you can have this kind of like data cache, data store, that just sits in between the, the user and the the L l M. And really what you use the LM for is just understanding what the user is saying. To parse the question mm-hmm. And to reformat the query or reformat the response in a way that makes sense to the user in like a conversational kind of like sense, right?
You want it to be like a chat bot. Mm-hmm. So I wouldn't be surprised if really what we end up seeing as like the l l m stack is just that we can, we will have some sort of framework that'll, it's like very easy to switch out these language models because, is chatt G B T three a lot better than Chatt g BT two?
Yes. Is Chad G b t four a lot better than Chatt g BT three? Yes. But is it something that we can really tell the difference of when it comes to most like enterprise use cases? I don't think so. I think that like for if you have a chatbot that you want to just chat naturally with, maybe chat BT four outflow chat, bt three by a lot.
But in, in terms of like for like most, enterprise scale use cases, What are people gonna be doing? They're gonna be asking questions about some of the data. They just want it to be able to formulate some sort of chatbot type response that makes sense. Like full sentences, paragraphs, and, 3.5 and three can really do this and Akuna can do this and like llama can can do this.
And I think most of the, the open source models have reached the point where if you get them right, if you use them correctly, they do pretty much match like three or 3.5. And there's been a lot of research papers around this. Um mm-hmm.
But one thing to say here is that also it is difficult to judge the, how good a large language model is because we only have, there's no quantitative like metrics for it. It's it's all qualitative. It's all oh, I'm reading this sentence here. Does this make sense to me? Like that's, that's, that's pretty much all there is to it.
So I think that like the. I think that there will be more like small language models.
But it is interesting to see there's a lot of this new like, language model development and I, I really do think that having a effective database and the LM stack is gonna be pretty pretty integral. Like anybody who's kind of building this stuff into the future is probably gonna be using some sort of vector story.
Well, so breakdown how that works. What does it actually look like in practice when you're using a Vector store? You mentioned how, cuz my understanding is that you can actually just, no, you break down how it works. I don't want to tell you, but my understanding is, Okay. So let's say so I'm just gonna I'll just cover like the architecture of OSS Chat, which is like one of our like, like demo apps that we use that lets you chat with open source documents.
So basically what you do is, if you wanna build one of these apps is you go and you grab all the documents that you need, and then you pipe the documents you need and you as you pipe them into a vector database in this interesting manner. So you, you, you break them down, you chunk them, obviously, and what you do is you actually send the documents to chat G B T or una or a llama or whatever, Bard, whatever, open source whatever LM you want.
Yeah. And what do you do is you tell the l lm like, here's some documentation. What are some questions that I could ask about it? Or something like that. That might not be the exact prompt, but it would be something like that. So now you have the question space and you have the answer space. And what you do is you store both of these in together.
And so each entry into their vector database is gonna include a question and an answer. And now you have a way to query for the questions instead of saying okay, so if I'm a user and I only have the docs and I wanna say something like, ca can you show me how I don't know x, y, z?
How does Torch vision work? And I wanna ask Pi Torch about it. It's gonna be very difficult for it. So maybe I wanna say how do I build a C Nnn in, in with, with Torch Vision, using x, y, Z modules it's gonna be very hard for the vector representation of just the answers to give you a good response.
So you have to store the question space as well as the answer space. And so first, once these, once we, once these documents are sent to chat BT and it generates these questions, These embed, these entries are put into the vector database that includes the embeddings for the questions and the answers.
And then when the user comes, the user says, I have this question. Show me how I can build a convolutional network with PyTorch. Then what it does is it says, okay, well, outta these questions, which one of these questions was generated from a, which one of these documents generated a question that responds to this?
And it finds like the closest question, and then it says okay, well, this, here's this tutorial on how you can generate a convolutional known network and then it outputs all the code for you. So the, the thing is that you have to kind of store both the question and the answer space, and you just use it as like a, you use the Vector store as your first place to go query.
So if you, so one of the things that is, that you'll also see is if you ask a question about pie torch, let's say we're talking. So the way OSS chat works is it can. Predict which op, which open source library you're asking about. But if you only have PyTorch documents and you ask a question about, I don't know hugging face or whatever, it's not gonna be able to give you a correct response and it'll default back to chat g bt and we'll, it'll see what it says, but most of the time that's up to you as like a user in, in, in your production use case, whether or not you want to default back to the LM or if you just wanna say, we don't have that information in our data.
Yeah. So one of these use cases that you mentioned before with the enterprise and mm-hmm. I think it's becoming more and more popular, is the idea of we have a knowledge base and we have everything in a million different places, but we can pipe all of that into a vector database and we can have it on hand so that if people want to query different questions about.
How this project is going, or how this product was created, or who was involved with it, et cetera, et cetera. That can happen. And the way I understand it is you pipe all of that data from all these disparate sources into the Vector database, and then you are, you're chunking it out. You're sending it to your large language model of choice, asking for the questions that can be asked around that.
You throw that back into the Vector database. But at some point, and if I understand correctly, you also are going to get these questions that haven't been asked. So then let's say there's, there's a question that I, I want to know, was John working on this project when Henry was not on the team yet?
Right. And that seems potentially it doesn't need large language models to do, especially because they could hallucinate that really easily and just tell you whatever. So how have you been seeing those kind of situations where, I have a question, a very specific question. I go into the Vector database, the question hasn't been asked yet.
And then what do you do from there? And then how do you like maintain the validity of those answers? Yeah. So the actual so the Vector database doesn't actually, you don't actually have to store questions that have been asked. You actually ask chat BT or whatever, re whatever l l m, you have to come up with a set of questions.
And so I think that, in that case, perhaps the LM has not come up with a question, was John working on this project before Henry had joined the team? But you know, once you have one of the things about. Well, I can only say that VIS has this, I don't know I don't know other databases that well, so I can't say exactly what they have or what they don't have.
But VIS does allow you to do this kind of metadata search around okay, let's say we have a filter and you wanna say, I only want information about this project from these dates. Then you can pass that in and then it would be up to, whether or not the LM can parse that correctly.
And that's something that is kind of, that, that is like a more up to the user's implementation and how the, how the user is able to implement that or whether or not that is able to be answered easily or whether or not you're gonna get some sort of more generic response. But I would say that, like you would just have to think about some of the filters that you may need to apply on your data and allow.
The l l m to kind of like know this kind of stuff. It is very unlikely that you would see some, so like for example, with that question exactly, it's very unlikely that you would see something like this team, this was done when Henry had not yet joined the team. But it's possible that you see something maybe in the docs, and maybe this means that, you don't have to do the filtering.
That maybe if you have something in the documentation that's team members who worked on this project were, John, Barbara, Sam, I don't know. And then, the, what the doc could, what the language model could understand from that is to query these documents and look for ones where Henry is not mentioned in the team name.
But yes, I mean, like that's a, that's a, that's a tough, that's a tough question, right? That's like something where if you are building the LLM app, that's kind of up to you to decide how you want to handle these things. Where The user may ask a question that the l l m doesn't know how to parse and that hasn't, hasn't been asked.
Yeah, I get it. And the, I guess the other big question for me, always it comes back to when, how can we set up some kind of process or rules or whatever to where we don't need to use the large language model unless we absolutely need to. Because I think it's always going to be a better scenario if we don't, considering that it can go off the rails, or if we can get the information just directly from the database.
Yeah. It's going to be faster and cheaper and all of that. So like how can you set up those, potentially just those rules. I imagine it's not gonna be hard coded. Maybe there's actually people, there's, there's. Researchers out there that are creating papers on this very topic, but I wonder about that a ton.
How to set up rules around using vector databases and like an LLM app as okay. Yeah. I mean, it's really like how to make sure that you don't overuse the LLMs. Mm. Because you don't always need them, right? Yeah. Yeah. I mean, I think that, well the way that the way I, I kind of see that working is just then you would, you would say okay, we only want, if, we, we want you to query the vector database first.
And if the answer is not within a certain distance, and so, okay, this is where we get kind of get into like vector embeddings, right? So vector embeddings are these numerical representations of some sort of data. And they're usually taken from the second to last layer of your, your model.
And one of the things that, the way that vectors are indexed is based on what the vector embedding actually is. And then there's two ways, there's multiple ways to measure distance, but the two ways that we do it are inner product, which is basically cosign similarity. Or L two norm, which is the, the L two norm with two vectors.
I would say, you would basically say, here's a query. Are you finding anything within a certain dis you'd say to the vector database, are you finding something within a certain distance of this? And that distance metric is a parameter for the user to tune for their user to understand like what makes two queries too far apart.
Because what you can really do, what you normally do with this is you say, gimme the top three results, or gimme the top five results, or gimme the, top whatever results. And then you look at those results and you say these results are. Like this result is the right one and maybe it's the second result that's returned.
Maybe it's the first one. Usually we just say the first, we just assume the first result, the closest one is gonna be the right one. But if you have like certain things where you want to say, if the vector doesn't, if the vector, it's cuz the vector database is never going to not find a solution as long as there is a vector in the vector database.
If there's only one vector, it will return that vector. Every time you'll, you have have to set some like parameter that says if the vector is this far away, then then it doesn't count, then it's not close enough to our, our query vector. And that is actually up to how well you know the data. What do you mean by that?
How well you know the data? So if you, so if you wanna say there's a certain vector distance that is too far from the vectors, the vector query, you can kind of like look at some of the distances between your vectors and understand okay, these two vectors are. Let's say a thousand units apart.
And that is too far to be, to be related cuz maybe one of them is, is like
apples are pollinated by birch trees in the Pacific Northwest. And the other one is like Kobe beef is raised in Japan. I, I, or maybe the other one's you just came up with this off the top of your head. That was incredible. I love the creativity there. Oh, thanks. Yeah, I mean these are good though.
So they are kind of similar. They are kind, yeah. That's why I was saying, okay, maybe the second one should be something like, the Northern California is better than Southern California. Yeah. Maybe, maybe the other one should be something like, the derivative of E of X is always E of X.
Okay, there you go. Why you have two completely unrelated topics and you say, this is where I draw the line. But you could also draw the line at the food one you say one is about, beef, one is about apples. These are two, two, unrelated to, to to say that this is a correct result. As a vegetarian you have to kinda know what your data looks like and know like how far apart is too unrelated for you as the, the querier.
Yeah. I see. So as the query, you are the one, and correct me if I'm wrong, you are the one that is setting up these, these rules on what it returns. If it is not far or if it's too far, then you're gonna say, don't return that because it doesn't make any sense. Right, right. Well, I guess not necessarily. It might not necessarily be the person who's running the queries who sets that up.
It's probably the person who's setting up the, the app and I'm just assuming that these are the two of the same people, but that's not always the case. In fact, I guess that's usually not the case. But you know, the person who's setting up the app should understand like what, understand that at a, at a very basic level.
Yeah. Okay. And so then when it is too far thes, when you say go to the large language model Highly oversimplifying this, aren't I? I mean, yes, but yeah. Yeah. I mean, yeah, that's basically what it is. It's if it's too far, you say go to language model or you say return, like we don't know uhhuh. I see.
All right. So one thing that I was thinking about too, as you were mentioning all of this good stuff is how the vector databases, like they're great for certain use cases as we've been mentioning, but they also are maybe not great for other use cases. And so what, when would you advise someone not to use a Vector database?
Yeah, I think that if you have something where your primary differentiator is something that is, can be stored in like key value pairs, then you don't need a vector database. And in fact, I think that if you. Are working with data that isn't, that doesn't need any semantic similarity. You don't need a vector database.
So for example let's say you're working with you're labeling cans, and you want to say this can is lime flavored sparkling water. Okay. So now you have two kinds of key value pairs, right? Flavor, lime, water type sparkling. And then you want to filter based off of, am I looking for sparkling water?
Am I looking for still water? Am I looking for lime or grapefruit or apple or whatever? You wanna say that's like something where there's no need for a vector database. Like just go to a key value store. And also it wouldn't really fit a vector database that well because with, with, with text you actually want the text to be, well, I guess it depends on the, the.
The model that you use to generate your vector embeddings. But most models that we use to generate vector embeddings are trained on written text in the form of like sentences or paragraphs. And so if you have something like, flavor, colon, lime, comma, water, colon, still, it won't understand that as well as if you said something like this is a can of lime flavored sparkling water.
So if you have your data in a different form than complete sentences, a, it's gonna be, I don't think there's a lot of good models for finding the right embeddings. And then B, even if you do find the embeddings and you sort 'em in back to database, you're wasting your time. You could have just used a key value store.
Mm-hmm. Yeah. It's not the right tool for the task. Right, right, right. It's not the right tool for that task. Yeah. So one other thing that I hear people talking about quite a bit is elastic search. And elastic search feels like it could be used for this, but not quite. Have you seen people talking about that and like, how do you navigate, Hey, should I be using Elasticsearch?
Do I need to bring on a vector database? Can I just get away with what I've got? Yeah. I've seen people talking about this as well. But to, to be honest, I don't have a lot of experience working with Elasticsearch. So I'm, I'm not entirely sure. Yeah, I, I can't, I can't really say. All right, no worries then.
So let's go into this idea of where you have been seeing generative AI apps. What are some common pitfalls that you have been seeing? So, So let's go into this idea of what different pitfalls you have been seeing in generative AI apps are. Well, given the space where I work, most of the pitfalls I see in generative AI apps are just like data.
Mm-hmm. And like I said, like I, I like, most of them, what I just see is that they don't have the right data. Or what I've seen a lot on Stack Overflow recently has been people like saying things like, so this one's actually I've seen on Stack Overflow and Reddit is someone was like, Hey, I have the CSV data.
And I I, I vectorized and I put in a Vector database and, oh, actually someone came to vis office hours with this question too. They were like, I'm having, I have this data and I vectorized it and I put it in Vector database and it's not returning the right responses to me. What's up with that?
What's going on? And then, so we took a look. We, we we took a look into their, into their into their code with them and into the, the data as well. And what we found is that, for example, like I was saying earlier, your CSV data, it's not stored in full, full sentence like format.
And so you see you have these problems, like it's got a bunch of special characters in it slash r slash n, slash t slash you, these, these, whatever. And then also there's a bunch of commas that just separate different independent clauses. And so when you have something like that, it's difficult to vectorize for machine learning models in general.
And then it doesn't work well in, in, in a Vector database or any, any kind of database really. So one thing I see is people kind of like have dirty data, yeah. Or they don't have the right data to insert or, one thing that I, I think is, is interesting that I haven't seen a lot of people solving is really like, how do we.
How do we make sure that our data in our vector database is continuously up to date as well? And like you can build a pipeline for that. You can build a batch and insert for that. You can do event based processing for that. But there's a bunch of there's, there's a bunch of, of kind of people who are just saying what I see a lot of built in the LM app space right now generated AI apps is like people who are just like, oh, like here's chat.
So I saw this one, it was like chatt for your PDF documents where they throw a PDF into an ocr and then they, vectorize that, and I've seen this multiple times. I don't know what they're doing under, underneath the hood. I don't know if they're, if they're getting the, the, the question space and then having you question the que question space or if they're only storing the answer space.
But you know, I see a lot of things that are just like, here's how you query your own documents. Or for example, like auto G B T that one was like super popular and. I have not, I've met one person who's been like, oh, I was actually able to get this to, to do something like great, to do something useful for me.
Someone said that they got it to build like a very simple like react react site that like had a spinner on it. And I was like, oh, okay, that's cool. This is like the first story of anybody saying that they've done something with auto G B T that's worked for them. I try to get auto G B T to do some research for me and I was like, find me news from the latest month, the last month of ai.
And it went back to 2022 and I was like, this is not really, not really what I need. Little suspect. Yeah, yeah, yeah, yeah. Right. A joke that I thought was hilarious and I don't think anybody else laughed, but it was along the lines of yeah. So I asked Otto g p t to cook me a carrot cake and next thing somebody from TaskRabbit is knocking on my door with the latest D V D.
From Space Jam because like what is going on up? Yeah, yeah, yeah. It says here, I'm gonna do this. A rabbit. Yeah. Bugs Bunny is in Space Jam and then you've got Bugs Bunny likes carrots and all. It just went way off the rails and Task Rabbit and all that. So yeah, I think I've heard a lot of people echo that sentiment, but again, we digress.
I didn't mean to derail you on this idea. I think the, the greater theme here is, and it just goes back to something that we say time and time out on this podcast, is you gotta be intimate with your data. Yeah. And you gotta just go and know it. The, the really top performers, they can know tho that data so well, and then get those insights from it because they're just so.
Very familiar with what is going on in the data. Yep, yep. Knowing the data is definitely, I mean, I feel like anybody who's worked at ML for long enough kind of co comes around, everybody is oh, these model, like, when you first start, you're like, oh, these models are like, so cool.
We gotta get like the right model. And then as you kind of get into it, you're like, oh, wait you know what, what, what was the data that we trained this model with? And then you're like, oh, we didn't include anything about bananas. And so the model doesn't know anything about bananas and stuff like that.
And it's interesting to kind of see that, and the same way that it's I mean, it's kind of like how people are as well. You kind of see this like Anth, anthrop, anthropomorph, lms, acting like people, because they don't have like the, the, they, they don't have the data. And if you don't give them the right, the data in the right form, it's just like people, if you don't, if you don't give them the right data in the right form, they don't understand.
And so, it's, it's very interesting to kind of like see that, and it's very interesting to see that as like a problem that we're, we're kind of like facing right now. A hundred percent. Yeah. So, dude, talk to me about vis, I know that you all have a ton of cool stuff going on, and there's vis, which is the open source project, right?
Right, right. And then you have Liz, which is the beefy Enterprise version, or is it like a managed vis? And then there's also Vis Light, and I know you all just, I think somebody, was it Frank? He presented at an ML Lops Community Meetup in San Francisco and it was all about G P T Cash. So y'all are doing a ton of stuff.
And then there's, there's the Liz Cloud too, right? Yeah. What's, there's, there's a ton going on there. Break it all down for me and what you're seeing people using each one for. Yeah. So, I'm, I mostly use VIS light vis, so there's vis, which is the open source factor database. And the great thing about, I think the really, really cool thing about Mil Ovis for me is that it operates really well at scale.
And when I say at scale, I mean like, if you're working with 500 million vectors, kind of like scale. Yeah. And there's some huge players that are using VIS too. I know that I've seen like the, who uses novis and there's, it's that kind of scale's who Yeah. Like Walmart and, and eBay and, they're, they're just, they've got huge amounts of data.
And I think that one of the things that when they, when they first showed me the architecture diagram for Novis, it looked pretty complicated. And it kind of is, there's three different kinds of like notes that you spin up. There's a query node, a data node, an index note, and they all handle different things.
And the query node handles how you query the data. The data handles actually, what, what's going on with the data. Yeah. And in, and then in S3 or whatever persistent stories you use, there's all these like segments. So VIS has these things called five segments, and they grow up to 512 megabytes.
And then once they're 512 megabytes, they're sealed off. They're not touched anymore. They're just stored in persistent memory. And one of the, I think, I think one of the reasons why VIS works so well at scale is because of these segments. And it's really interesting to kind of like see how it works is let's say you have one terabyte of data.
You don't want to re-index all of your data every time you add something to it. Right? And one of the nice things, and also if you search one terabyte of data, that's just the amount of calculations you're gonna have to pour 'em is really high. Yeah. So when you have that splitt of 512 megabyte segments, what happens is actually we parallelize each search for the segments so that it's actually possible to basically have you're, you're basically getting like a.
A much, a much, you get much faster queries on large amounts of data and it kind of, as it scales, your performance gets better and better relative to having single block chunks of data. So I think that's one thing that's really cool about it. Vis it itself is usually run, if you are running vis in like an enterprise, you're probably gonna be running it on some sort of server, some sort of sets of servers that you can kind of like horizontally and vertically scale.
And so if you wanna run it locally, usually people use like Helm or Docker composed something like that. But the nice thing about vis light, and this is what I think is actually really cool, is it kind of like, does this job of, democratizing vector databases by making it more accessible and so I wrote a piece recently about vector databases and how, we're democratizing vector databases and like the, the three like pillars that I have for that is like you provide like educational material that actually teaches people about, what are vectors, what are vector indexes what is the importance of, using the right vectors and stuff like that.
And then you have another pillar of, making, making it accessible. Cuz vector databases used to be, well they're pretty complex in general. And so they used to be things that were pretty much available only to large players like enterprise people. And now with things like mill to light, you can run it directly in your notebook.
You don't even have to be like a software dev. You don't have to understand you don't have to understand like docker or like Kubernetes or anything like that. You don't even have to know what an image is. You can just say PIP install vis default server dot start. And you've got a vector notebook.
You've got a vector database right in your notebook. And so now like data scientists, data analyst people who could actually, be using VIT databases. Now have access to this. Right. So I think that's cool. And then the last thing is obviously like the evangelism aspect where you just go and you like tell people like, Hey, this is cool technology, you should go check it out.
Right. But so yeah, I think that know the mil slide thing is really cool. I write a lot of Jupiter notebooks. I use a lot of just, I just, it's, it's easy to kind of like get these mini projects up and running, like llama llama index or like lane chain or anything else like that can, you can run in your vector notebook and then you can in your Jupyter Notebook and you can throw it on CoLab and it's like easily accessible to the public.
So I work a lot with VIS light. Initially when I, when I first onboarded, I worked with vis like the standalone vis on a server version. Yeah. Which is fine, you just, you have Docker and then you spin it up. But vis light is just really easy to use and it's just really nice to have.
And then Zills zills Cloud Recently has released this like free feature where you now have free Zillows cloud up to half a million, I think vectors. And it's, it's kind of like managed novis. Like you don't have to worry about scaling you don't have to worry about, hosting it on whatever host you're using e C two or, you don't have to worry about spinning up and spinning down pods and stuff like that.
So that's kind of what Zillow is, that's what Zillow cloud is. G P T Cash was like a project that we built that actually came from OSS Chat, which is the one that allows you to, talk with the open source software. G P T Cash was the caching layer for that. That was like, oh, someone's asking a lot about the same questions about Pie Torch.
Like people keep asking about torch vision or people keep asking about torch audio. Now let's just cache some of this as well so we don't have to ping about that. So G B T cash was kind of like that thing that was just like, Hey, we're, we're cashing out a bunch of queries, like your FAQ and you can save a bunch of money doing this and here's how you do it.
So yeah, that's pretty much like what, what VIS and, and Zillow have been up to. I'm really excited to see like a bunch of like new releases on Zillow cloud as well. People in the vis slack ask me for things like, oh, is there, dynamic indexing like, or dynamic schemas can we change up things?
And it's like now, like you can, you can just throw in like a J S O N as your schema and it'll, it'll accept that you don't have to go and create your own, like right now there's like a field schema, a collection schema, and then like collection. Then you have to put the field schemas into the collections schemas into the collections.
And then it's oh, actually you don't have to do that anymore. And then there's like role-based access control, which people have been ping about on vis at least like three times or four times in the last couple weeks. Everyone loves that, especially once you start to get into actual enterprise use cases.
That's like a go no go type thing. Yeah. We've basically, we had someone who like literally came to us and was like, we've decided to go with Novis because you guys are the first one to implement, or Zillow, because you guys are the first one to implement role-based access controls. And I was like, oh, very interesting.
This is like not something that, you know, as like a, as like someone who doesn't necessarily have to work with enterprise data all the time. This is like an interesting like point, it's, it's, you got that SOC two compliance also? I don't, I don't know. I think so. I don't know.
I just, but this is like just something that people came up to me and they were talking to me about in, in the Slack and I was like, huh, okay. That's cool. Yeah. That's interesting. It's not something that I would've directly related or thought about. Yeah, it's. Huge for some companies. I mean, it me, it, it's a showstopper if you don't have that because Yeah, yeah, yeah.
I remember when I was at Amazon, like the whole I am policy was was was just, there was just a lot. You had to do a lot with the whole I am thing. So Yeah. Yeah. That data access, man, that's a whole nother beast in this. And I've heard horror stories too, about how somebody got a job and then six months later they still didn't have access to the data that they were supposed to be working on.
And it was like, that's, yeah, that's, that's, I mean, that's just, yeah, it's terrible practice. It's also really relatable. Like I've been at I've, I've worked at places where it's just we want you to build this thing that requires you to train it on the data. And then I was like, okay. Where's the data?
Well, software devs don't have access to the production data. Data. And I was like, what do you, can I, can I get access? What do you want me to do? I'm, I'm not really sure. Yeah. So yeah, I see that that's like a thing at like mid-size companies, like big companies. It's very it's kind of weird. That's more of a, I feel like that's more of like a cultural, like work culture, like company culture kind of thing than, than anything else.
Though I'm not really sure like how we could go about fixing these kinds of things. Yeah, there's definitely people trying, I mean, I think that's what the whole data mesh crew is yelling about. Oh. And we're talking how oh, you gotta own your data, data products and treat your data like it's a product and you service the producers and the con consumers, all that.
So the producers service the consumers or whatever. That's my limited understanding of it, just from talking to the different evangelists in the data mesh area. But I do know that there's. There's some pain around that. Yeah. And the bigger the company is, the the worse it is. So, dude, just a quick side note on G P T Cash, what, is this something that you can just slap on or do I need to be running vis with it?
I think you just slap it on I mean, it uses vis okay. In the background use zi. Okay. But yeah, you, you would need if you're gonna run a standalone, you would need like a, a standalone server to ping. Okay, cool. Yeah. Yeah. Cool. Yeah. Yeah. So this is all super, super cool stuff, man. And I'm wondering, like anything else you wanna mention before we jump?
What's what's new in your world that we didn't get to talk about? I just wanna say like we're seeing a lot, we're in new, in another like AI renaissance, for lack of better words. And I'm very excited to, to, to be part of it. I'm very excited to see more people get access to this kind of stuff.
I'm hoping that we are able to, provide like the educational material that people need to get started with this. And yeah, that's, that's pretty much it. I'm, I'm pretty, I'm pretty happy about this. Unlike a totally unrelated note, very happy to have the ML Lops Seattle community getting started as well.
Oh yeah, we gotta give a shout out to the Seattle community. You all did a meetup? Yes. A week ago, I think. Yes. And so, and yeah, it's, it's growing and it's picking up steam, I would say. So I am very happy to see that happening too. And you're leading the charge on that one? Yeah, yeah, yeah. I'm, I'm very interested.
I, I, I love the community stuff. I love getting my hands into the technical stuff and building stuff, but I also love just seeing people come together and Talk about things they're interested in, especially when it's ai and I've been a, I've been a nerd about this for a long time now, so I'm very happy to see other, other people talking about it.
Yes. Awesome. So also you mentioned that you're doing office hours, right? Yes. In the Mil Vista community. When is that and what's, what's the deal there? Oh, yeah. So we have these office hours with me and Philip. They're every Tuesday at 1:30 PM Pacific time. They're primarily, the questions are primarily being answered by Philip to be honest.
I'm just there to kind of like facilitate and answer like some basic stuff. But a lot of the times people will ask questions that are much more like, how do you use Mil Vista at an enterprise scale? And then Philip has a lot more experience than I do with that. So he'll answer oh this is probably blah, blah, blah.
This is probably blah, blah, blah. And you'll see kind of like, we see a few people come every week, usually. Mm, three or four usually nice. Yeah, usually about three or four people. I don't think I've seen more than like seven. Awesome. Yeah. So they get some quality time with you all to get, answer all their questions, their heart's desire.
We'll leave a link to those office hours in the show notes in case anyone wants to drop by and chat with you. And I think that's it for today, man. Thanks for coming on here and talking with me. Okay, awesome. Sounds good to meet trs. It was great to chat.