Enterprise AI Governance: A Comprehensive Playbook
speaker
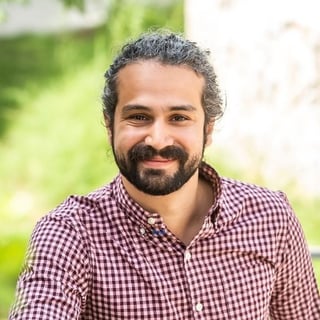
Ian Eisenberg is Head of AI Governance Research at Credo AI, where he advances best practices in AI governance and develops AI-based governance tools. He is also the founder of the AI Salon, an organization bringing together cross sections of society for conversations on the meaning and impact of AI. Ian believes safe AI requires systems-level approaches to make AI as effective and beneficial as possible. These approaches are necessarily multidisciplinary and draw on technical, social and regulatory advancements. His interest in AI started as a cognitive neuroscientist at Stanford, which developed into a focus on the sociotechnical challenges of AI technologies and reducing AI risk. Ian has been a researcher at Stanford, the NIH, Columbia and Brown University. He received his PhD from Stanford University, and BS from Brown University.
SUMMARY
This talk presents a comprehensive overview of enterprise AI governance, highlighting its importance, key components, and practical implementation stages. As AI systems become increasingly prevalent in business operations, organizations must establish robust governance frameworks to mitigate risks, ensure compliance, and foster responsible AI innovation. I define AI governance, and articulates its relationship to AI risks and a common set of emerging regulatory requirements. I then outline a three-stage approach to enterprise AI governance: organization-level governance, intake, and ongoing governance. At each stage I give examples of actions that support effective oversight, and articulate how they are actually operationalized in practice.
TRANSCRIPT
Slide deck: https://docs.google.com/presentation/d/1maujdaxxyky5OCDVzVcTcPNJLy5FpRMo9Nl5iqJwPTI/edit?usp=drive_link
Ian Eisenberg [00:00:10]: Hi, everyone. I'm Ian Eisenberg. I lead AI governance research at Credo AI. I'm just going to put a timer up so that I can kind of keep this on track. So Credo AI is an AI governance platform. So we've heard a lot today about kind of the ops, the technical ops that are needed, but they ultimately need to be connected to kind of human processes. And Credo AI builds a governance risk and compliance platform that allows organizations to kind of monitor their AI systems and manage their development, procurement and deployment. Kind of safe and efficient.
Ian Eisenberg [00:00:51]: And I'm going to take you through a playbook for what enterprise AI governance looks like. And by an enterprise today, I'm thinking about the organization who isn't necessarily developing foundation models, but is trying to figure out how to apply them, whether they're procuring external applications or they are kind of developing AI systems on top of them. So I'm going to divide the AI governance kind of journey into three stages, organizational level, governance intake, and what I'm calling just governance. The organizational level is going to set up the foundation for the enterprise as a whole, and the next two are going to be more use case specific. Governance is generally contextual, and so we'll spend most of our time there. But let's just quickly talk about what organizational governance looks like. So there are a number of components here. I'm just going to quickly go through them.
Ian Eisenberg [00:01:49]: Basically, organizations first start by identifying their principles. That's kind of like in 2017, all organizations came out with their ethical AI principles. That's a good first step. But after then you need to come up with a shared understanding of what is the AI lifecycle and how are we going to kind of come up with a governance framework? There are many different kind of conceptions of AI lifecycles. It's not actually that critical exactly how you do it, just that you've come up with a shared understanding that we're going to talk about like kind of the research phase, the development phase, the deployment, the post deployment monitoring, whatever it is, it's not so critical, just that you've set it up so that the rest of your governance approach can kind of connect to that. Comprehensive governance framework is basically what I'm going through today. Probably the most important thing is team responsibilities. You have defined someone to be kind of the DRI for governance.
Ian Eisenberg [00:02:41]: We like to call that like the AI governance custodian, but it doesn't really matter. Basically, AI, as we all know here, is rapidly changing the ways that we're going to integrate it into our organizations. The regulatory kind of context around and the ops that you're going to want to make use of to develop and deploy your AI systems effectively and safely are going to change. And that needs someone or some team that is responsible for that oversight. And then it's probably helpful have tooling that supports that governance itself. We often see enterprises kind of start in a kind of loose way, and then maybe they start to create spreadsheets, and then at some point they come to Credo AI, and they're like, okay, we're really looking for a tool. I think there's going to be a moment of problem here. Yeah, Credo AI is really this tool.
Ian Eisenberg [00:03:32]: We're trying to make it easy to go through the rest of the stages that I'm going to take you through. Okay, so we're at this organizational level, let's go through the rest of the enterprise workflow, and this is going to happen for every AI system. And I'll just say right now, an important aspect of a good AI governance workflow is that it is kind of contextual and proportional. A lot of people, when they first hear about AI governance or governance in general, they imagine an incredibly heavy oversight that slows down kind of development and innovation. And that's really not the appropriate lens. The appropriate lens is that you figure out the level of oversight as quickly as possible that can apply to that AI system. So we're going to divide things into what I'm going to call AI use case intake. And then again, the governance in each of these we can be divided into different stages, which I'm going to take us through.
Ian Eisenberg [00:04:29]: Okay, so intake, first step, ingestion. This is probably the most important step for most enterprises today. This is where people actually are. Ingestion is what AI systems am I actually making use of as an organization? Is there one place where I can understand those different systems? Do I have a kind of portal that makes it easy for my employees to kind of register them? That's not very burdensome. I'm not asking a million questions, but I'm asking the questions I need. You can imagine other aspects of ingestion might be the bottom up discovery of AI. Use cases as your employees discover new ways to make use of chat, GPT or procurement. The important point is that, you know, ultimately you need to get to a registry.
Ian Eisenberg [00:05:19]: You need to get one place where you can understand how am I using AI at my organization, both because it's relevant for understanding kind of risks and things that we're going to be talking about that are kind of traditional governance, but also like the value, where should you be devoting your time, the impacts. And this registry here, this is a screenshot from the AI platform. You know, ideally will bring to the fore the most important aspects that are relevant for your AI system. So that's definitely like risks, alerts, the impact, the actual use of it. And while here I'm showing what we're calling an AI use case registry, we're finding what you can find in Pareto AI is there are many different entities that need their registry. A registry of models like GPT, four of applications like ChatGPt, and use cases like using chat GPT for a particular area, and recognizing that kind of interactive nature of these different entities. We kind of just heard a talk about this kind of entity. Resolution allows information to flow more easily between these two.
Ian Eisenberg [00:06:27]: You can understand your governance for an application within the scope of uses and then have that information flow. Okay, so we've talked about intake. Next step, initial risk evaluation. This step is the main goal of it is actually triage. You're trying to set a label that allows you to then later on apply the appropriate oversight that you need. And so it's critical that it's like, you know, you're not making errors in either way, right. You don't want to be calling low risk systems high risk, then you're going to be kind of wasting your governance resources or high risk systems low risk. And that way that's probably worse.
Ian Eisenberg [00:07:06]: Then you're going to be having these systems kind of move on without any oversight. We generally think that qualitative labels are probably the best right now. Kind of like high medium low. That also is in correspondence with some of the emerging regulations both in Colorado and the EU AI act, which use this kind of qualitative labeling, high medium low. So while I'm talking about it right now as a part of your enterprise government's workflow triage, it's sometimes actually like necessary for the compliance requirements. And there might be actual rules about what this AI system should be labeled as. It could be helpful to have a risk taxonomy. So the high medium low, that's a very coarse grain way of thinking about risks.
Ian Eisenberg [00:07:48]: When you think about the use case, you can, it's helpful to have some organization to make you think about risk from many different perspectives at once. And the use case itself can have different risk scenarios identified and that will downstream affect your governance. And again, Credo AI supports you here by helping you surface, recommend risks, these kind of things depending on your appetite to understand risk next stage of intake, compliance requirements. Of course, GRC stands for governance risk and compliance. Compliance is important and will of course, increasingly become important for AI systems. Now, what compliance requirements apply to your use case is difficult to understand, especially in a changing ecosystem. It's dependent on the use case, your region, etcetera. But at credo AI, we kind of think it's useful to think about a common ground set of requirements, like what seemed to be, at least for now, the emerging consensus.
Ian Eisenberg [00:08:46]: That happens again and again, because if you can think about those, your kind of governance approach will be more evergreen, right. It will be applicable in different areas. And then you can just use this step to kind of understand the kind of nuance of the edge of. So the common ground requirements that we kind of have identified are registering inventory in AI use cases going back to the intake, the registry isn't just good practice. It probably will be regulatory. You know, a regulatory requirement AI system test. We've heard a lot here about evaluation. Evaluation is critical in human oversight.
Ian Eisenberg [00:09:21]: I think about this top row. It's like the set of things you're doing internally to manage your AI systems. Well, the second row, independent evaluation, AI impact assessment, no transparency. That's about external oversight of your systems. Like you're going to have to report out to someone about something or be audited by someone. This is not very mature right now, but is maturing. So starting to think about how you're going to be able to create reports is important. And then ongoing risk management.
Ian Eisenberg [00:09:50]: And what's nice about that is they map nicely to this system that I'm taking you through. So the compliance requirements, some of them are particular, like you need to evaluate for fairness for this hiring system. Like it might be very specific, but some are just like, you need to have this process in place and that actually like corresponds to where most enterprises are right now. Okay, so finishing up intake is defining a governance plan. So the whole point of intake is that you have identified your AE's system and you understand its requirements because you understand important context about it, its risks and its compliance requirements. Hopefully also its business benefit is also part of your intake. And the step is to say, okay, what am I going to do about it? What risk mitigating controls? What controls are required of me? What internal policies do I want to apply? Thats the definition of governance plan. And like I mentioned before, that doesnt mean that the governance plan needs to be heavy just because youre defining it.
Ian Eisenberg [00:10:51]: Thats just an intentionality. If your system is low risk, your governance plan might look a lot like what your ops already is. It might be, hey, we need to make sure that we evaluate the system for its business use and monitor it later. Hopefully that's just general practice for many ML teams. So it's not like an additional hurdle. It's like, do what you're normally doing, but you might have a medium risk system and you're like, this one needs privacy review. Let's bring in our privacy team. Or a higher risk, like maybe there's an executive committee.
Ian Eisenberg [00:11:24]: Like, there are different ways that you can approach this. But again, defining the governance plan is just being intentional about how you are reviewing the system and that can be automated. In fact, in free to AI, there are aspects where you can like make this much simpler. So here we have one view of what we call policy packs, sets of controls. And some of those policy packs up here are like regulatory or standard based, but other ones could be based within you as an organization. You might come up with some rule where you say, you know, if this system deals with PII, I want to apply my internal policy of PIi. So, you know, you. The governance plan in credo looks like identifying a set of policy packs and on another screen, identifying a risk mitigating plan for different risks and areas that you might identify.
Ian Eisenberg [00:12:15]: Okay, so we finished that. I'm gonna fly through the next bit because I have a little bit less to say about it right now. But this gather and evaluate evidence is actually a huge set of things that you could do. You defined your controls, now you need to gather evidence about whether you are meeting those controls. And that means doing a bunch of things we hear about here. Like this is the evaluation, this is ensuring security, this is doing your fairness assessments. This is post monitoring. This is like your experiment, tracking your data linkage.
Ian Eisenberg [00:12:48]: All of those could be a kind of control that you need to kind of gather and evaluate against. And who evaluates the AI governance custodian, right. This is a separate oversight function, and that's a big reason why you don't want to be applying it all the time. You want to be judicious about your use of governance kind of function. Functions, okay. Generating reports and dashboards is important both internally, you know, multi stakeholder alignment, these kind of things, tracking. Lots of people are into it, but it will be increasingly required externally. You need, you know, to have kind of societal oversight.
Ian Eisenberg [00:13:23]: You're reporting out to someone. And finally, there's like ongoing audits and monitoring. So these are, again, I think you're seeing like the mlops kind of brought in about this human oversight. So we finished going through these aspects, we're done. Right. That felt easy. But of course, for governance to work, it is integrated with your AI development. It's not a separate silo.
Ian Eisenberg [00:13:49]: So one aspect is your AI governance platform. Connecting with this is just one example of what that development could look like. Right. You've got your data governance, your model of data selection through prompt engineering, AI systems, all of these, both should be configured or potentially configured by governance and certainly ingested. And the other aspect that AI governance is important for is connecting your AI development to what I'm calling societal oversight. Basically, the regulatory standards and best practices need to connect, and that's difficult to do. And so a good AI governance platform will help you kind of take those requirements and make them easily available for your use case. And oftentimes maybe you don't actually have to do anything more, but if you do, you need to be tracking it.
Ian Eisenberg [00:14:38]: Okay, I'm going to skip this. AI governance is a journey. Different people are at different stages and that's it. Thank you for your time. Yeah, I don't have a QR code here, unfortunately, but you can go to Credo AI demo if you want to see more about Credo AI or come talk to me. Thanks. It.
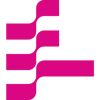