EU AI Act - Navigating New Legislation
speakers

Co-founder & CEO at LatticeFlow AI, building the world's first product enabling organizations to build performant, safe, and trustworthy AI systems.
Before starting LatticeFlow AI, Petar was a senior researcher at ETH Zurich working on the security and reliability of modern systems, including deep learning models, smart contracts, and programmable networks.
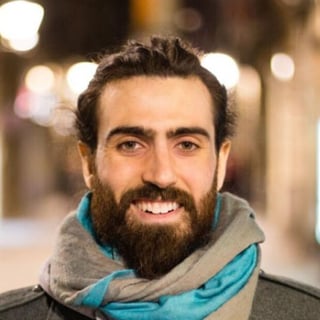
At the moment Demetrios is immersing himself in Machine Learning by interviewing experts from around the world in the weekly MLOps.community meetups. Demetrios is constantly learning and engaging in new activities to get uncomfortable and learn from his mistakes. He tries to bring creativity into every aspect of his life, whether that be analyzing the best paths forward, overcoming obstacles, or building lego houses with his daughter.
SUMMARY
Dive into AI risk and compliance. Petar Tsankov, a leader in AI safety, talks about turning complex regulations into clear technical requirements and the importance of benchmarks in AI compliance, especially with the EU AI Act. We explore his work with big AI players and the EU on safer, compliant models, covering topics from multimodal AI to managing AI risks. He also shares insights on COMPL-AI, an open-source tool for checking AI models against EU standards, making compliance simpler for AI developers. A must-listen for those tackling AI regulation and safety.
TRANSCRIPT
Petar Tsankov [00:00:00]: Hey everyone. So my name is Petar, Petar Tsankov, CEO and Co-founder of LatticeFlow AI and I do like my coffee non regulated because I like to make it exactly the way I like it.
Demetrios [00:00:13]: What's going on? People of Earth, we are back for another ML Ops community podcast. As always, I'm your host Demetrios and today we got talking all about the AI EU Regulation Act. I find it fascinating because there is a narrative that is going on which is all regulations bad, the EU act is stifling innovation, et cetera, et cetera. And here what we see. Petar came on, he talked about what we need to know if we are doing any kind of AI within the EU and how we can action all of this legal jargon, the words on a page into tests for our technology. Last time we talked about how we were optimizing for accuracy. This time I really want to get into how we've been optimizing for capabilities, especially with these large language models. Uh, but then there's this sneaky thing that kind of came up and blindsided a few folks who may or may not have realized it was coming.
Demetrios [00:01:36]: But that is the whole EU act and the AI regulation. So I wanted to have you on here and just really get into AI safety, AI compliance, AI governance, all of that fun stuff. Because I think there's a lot to unpack here and what it means exactly like this hopefully will be the definite guide for if you are trying to do AI in the UEU in 2024 and 2025, as long as the current legislation stands, then you can come and listen to this and get the best breakdown and hopefully the best understanding to know where to go and what to do. So last time we spoke about a decade of AI safety, right? What's changed since our last discussion?
Petar Tsankov [00:02:28]: Good point. So, and glad to be back on the podcast. So last time when we spoke that was just about six, seven months ago I believe. It feels like it's been several years, but time was very fast in our space and last time it was very, very mixed state in terms of AI safety. I think there was still a lot of confusion, like safety was such a, such an abstract topic, so people were still worrying about the wrong things, like worrying about existential fears of AI. Not quite clear what's going on with regulations and so on. And lots of things have changed, I mean on all aspects. So kind of on the more practical aspect, companies are a lot of organizations right now are really hands on implementing AI governance.
Petar Tsankov [00:03:13]: So I have been to multiple industry Conferences where you see companies realizing that they go from kind of from five AI applications to a hundred over like period of, you know, several months, which is crazy. And everybody's realizing we have to put this in order. So let's start, you know, making sure everything is. We at least understand what solutions we have in place. Let's make sure we can classify which ones are important for the business, which ones are not, kind of what are the risks if there's regulation, if there are applicable regulations, like the EU AI act that came into force since August 1, so just last month. So then how do we demonstrate compliance? How do I pick the models that would actually pass these checks? So this is very healthy to hear this kind of progress because it's not about, let's say AI would destroy the world tomorrow, let's stop training models globally. So this is now, these are really now the practical applied topics that you want to be thinking about. And that's really good to see.
Demetrios [00:04:16]: I like that you call that out. How there was so much fear mongering especially. Yeah. In February even and people were being called to the Supreme Court or to Congress to testify in front of Congress and were talking about how we need open source or oh, we're going to put a ban on a certain parameter of model training size or whatever. It was all so outrageous and now it's changed a ton. I was even, funny enough just reading through the state of AI report and it talked about how these model providers have switched from this whole AI doom scenario type of narrative to oh, now we gotta go out and make money. And so we're going to not talk about how AI is going to take over the world or any of that kind of stuff. And I find that, that really funny.
Demetrios [00:05:10]: But you said a few things that I want to pick apart. One is companies that you've seen going from five to a hundred models and recognizing this can get out of hand very quickly. So they need some kind of governance process in place. I want to talk a bit about what governance processes you've seen that have been working. But also I want to mention we had a guy on here probably around the same time that you came on last and he was talking about how he was working for Yandex, that Russian.
Petar Tsankov [00:05:47]: Google type thing, the Google search.
Demetrios [00:05:49]: And they had no idea out of the thousands of models that they had in production which models were doing what and which ones actually were affecting the business. So he coined the term they had zombie models in production. And one of his biggest projects was to go out there and Take down a lot of these models that weren't doing anything because people were afraid. They were literally afraid, like, ah, I'd rather just keep it out there because I don't know if I take it down, if it's going to all of a sudden show up as a $2 million loss on the balance sheet.
Petar Tsankov [00:06:27]: Yeah, some people, it's not clear that how it's being used and what it's doing, but you better keep it running. Yeah, yeah, but it's really true. So it's really, I mean especially in the larger organization, understanding what's happening underneath, you just don't know. And we do. So unless you put this very centralized process in the organization where there's like designated team responsible for this with more clear definitions about what is high risk. I'm talking about high risk for the business. Not necessarily with respect to regulations, the UA act and kind of other criteria that are also now becoming relevant. Unless you have these people to kind of go and dig and understand this, you don't, you just don't know.
Petar Tsankov [00:07:14]: And that just exposes then the organization at high risks. Because on one end you have, I think everybody really understood the real business risks, that this is not just technology that you deploy, but there's users on the other side that get affected. So I mean there were already several lawsuits for the typical things like bias, wrong information. I mean if you, you rely on the customer support and the organization gives the wrong information and then because of this something goes wrong, why would I not sue? Yeah, you know, I would. That's how, that's how life works. And that's kind of where I think these, all these problems, they immediately got lifted very high up in the organizations because it has this massive impact, business impact when you talk about risks. And yeah, so that's what then requires you to do this systematic process and what we have seen on top of this, because I think governance for me is not. Governance for me is the starting point.
Petar Tsankov [00:08:15]: Like this is like you need to open your eyes and see what you have in the organization. But then the second, the immediate next big challenge is well, what do you do about the high risk ones? And we can later chat about the EU act as well. How do you actually assess the risks or how do you assess compliance? These are now the topics that I see becoming very, very relevant because you have to address them right now. Suddenly you know, you know, you know what you have in, you know, you need to make sure they, they are compliant, safe and so on. And that's where we do see Kind of now kind of two that kind of dynamics. One is we see like individual teams in organizations like adopting specific solutions like AI applications. Could be identity management, could be, you know, predicting prices, whatever it is. And these people, they do realize that, well, this model is critical.
Petar Tsankov [00:09:07]: I have to make sure it works. So let me make sure I don't get fired. So it has to be checked, thoroughly checked. And they actually are seeking out explicitly like going out and seeking for experts to validate them. But I think governance would flip everything upside down because suddenly everybody will know. Well, actually These are the 50 important AI applications in the organizations. You have to go and check them. That's becoming top down now driven on how you, how you address risk at the organization, which is also typically how you deal with risk.
Petar Tsankov [00:09:37]: Right. You don't rely on like a diligent employee or a manager to make sure that they do this on their own behalf. That's kind of opportunistic.
Demetrios [00:09:48]: It does feel like since it was so easy to get AI out there, if you played it fast and loose and you put something into production and you didn't think through all of the risks and the governance, then it came back to bite you. And I like that you mentioned that got escalated up very quickly. And so now you're seeing teams that are specifically built for this purpose. And I would imagine this is more of. Yeah, when you get to a certain size of a company and you get to a certain type of AI or use case, then you 100% need that. Have you seen that be the case?
Petar Tsankov [00:10:36]: Yeah. So virtually any organization at this point needs this. So I wouldn't put like a size threshold, but I mean there's some minimum size of threshold, you know, maturity of the company where you would have that volume of solutions where you would need this oversight. But we do see this being definitely centralized because it requires specific expertise like understanding of risk management. And we often also see that this becomes an extended function to risk teams that are now also not purely dealing with kind of the classic risk models, but also being tasked with and to onboard AI engineers that capable people that really understand also the technology so that they can think about this and ensure that they set a process to validate them. So that's kind of the two ways we see it. But it's definitely, definitely becoming a thing like at any organization.
Demetrios [00:11:38]: Yeah. So I want to talk about a tool that you just open sourced recently. And props to you all for the name.
Petar Tsankov [00:11:47]: I think it's.
Demetrios [00:11:49]: Yeah, so it's comply. Right. But it's compl AI Yeah, exactly.
Petar Tsankov [00:11:55]: Comply.
Demetrios [00:11:56]: YI yeah. What is it?
Petar Tsankov [00:11:58]: Yeah, so comply is what it is. So the reason we started this is to address the big challenge with the high level regulations. So right now there's a lot of fear around. Specifically this is for the EUA act, is the first compliance centered evaluation framework for the EUIA act for language models. So what this means you can take any language model and you can run the evaluation framework and it will give you scores, like concrete scores between 0 and 1 to show you how compliant the model is against the specific principles defined in the EU AI Act. So this is what it is. And what we had to do to make this possible is so I don't know if you read the EU AI act, it's very, very high level. It does have a lot of technical terms as well inside the text, but it's very high level.
Petar Tsankov [00:12:52]: And this was really scaring a lot of people because you suddenly have a regulation that you have to comply with. If you don't comply with, there's like serious fines, like 35 million euro or 7% of revenue, but you don't know even how to show compliance. You don't know, you have models, you have no idea how well they are against the EUA Act. So that was, you know, to address this, you had to basically bridge this gap. You have to take the regulation, the high level regulatory requirements, then map those into, translate those into technical requirements. So something that's taken, you know, that's actually actionable, that you can measure and that's effectively what we did. So mapping the requirements, the high level requirements, the technical requirements, and then each technical requirement on its own is mapped into concrete evaluation, you know, algorithms and benchmarks that you can run and then measure, measure the numbers. So that was what the effort was.
Petar Tsankov [00:13:50]: And I think the reason this got a lot of attention is that, you know, this was really the first time. Also, in addition to the project, once we implemented this, we evaluated many of the available, the public models and there's lots of leadership boards online that talk about performance capabilities, how well these models are performing, but this is the first time that we understood how they perform against the UAI Act. And that was kind of now interesting to see, like, well, how do they actually perform now?
Demetrios [00:14:24]: Does this work for fine tuned models also? Can I throw this on top of whatever fine tune model I've created?
Petar Tsankov [00:14:33]: Absolutely, yeah. So this is, you can integrate. So right now it is for language models. So if you want to do some other modality that would not work, but it doesn't Matter. So you can take. Yes. As long as you can plug in the model and you have kind of an inference API to run it, then you can do it. And yeah, so that's, that's why also how, how we were managed to evaluate the models from OpenAI, Anthropic, Mistro, Alibaba and few.
Petar Tsankov [00:15:00]: Few of the other ones.
Demetrios [00:15:02]: Yeah. Well, it's fascinating that some of the models, they just have said, hey, we're not going to play in the EU because of the EU app. Right. I think Llama is one that I am, that comes to mind right away. And it's that the llama 405B model or llama 3.2 basically was not released in the EU. Right. And so do you feel like this is a way to combat that and maybe skirt around it?
Petar Tsankov [00:15:34]: Yeah, so I think, I think that that's ultimately what we want to address. I think it's not surprising that vendors that are training the models are worried about deploying here because if you have these strict rules, but they're not actionable, meaning you don't know if you comply or not, it's just opaque, so you want to stay out. So in a way the reason of this work is that to show that, well, kind of let's just not adopt this traditional narrative regulation is bad. This is like some things have to be regulated. If it's powerful technology, you have to regulate, you have to put some baseline safety and so on. But let's make it actionable. Right? Let's make sure that any organization can actually evaluate their models, can understand against which principles the models are performing well, against which ones, not so much and where effort should be placed. If you have this kind of actionable benchmark evaluation frameworks that can guide you what to do, I don't see that's not really scary.
Petar Tsankov [00:16:45]: This is just giving a directions on how to improve your models. And if you look at what are actually the kind of the core principles and requirements that you need to satisfy for compliance? Well, they are very meaningful. These are not things that generally AI vendors would not want to comply with. You know, we're talking about things like toxicity or, you know, leaking problems. These are useful things. You know, vendors want to have those, but you also need to have the proper benchmarks to evaluate it.
Demetrios [00:17:15]: Well, one thing that I was thinking about as you're mentioning how vague the EU AI act is, and really the whole part of this work is making it actionable. How did you take the vague words of the EU AI act and, and then create something that is actionable.
Petar Tsankov [00:17:35]: Yeah, so that was, let me summarize one year of effort into a few sentences. Now there were a lot of people involved also, just to be clear and to acknowledge all the contributors. So we also worked with ETH Zurich. So they were very bright. Some of the brightest PhD students working on AI safety were involved in this effort.
Demetrios [00:17:55]: Nice.
Petar Tsankov [00:17:56]: Also Insights, which is one of the SOPHIA based research institutes created in partnership with ETH and epfl. So, you know, they also actively participated. So it was a very broad effort with also we had people with legal degrees or legal backgrounds that did also have an AI background. So you do need this kind of mix of expertise to make this possible. So it was really going through the acts, finding all the technical terms, kind of to understand what is the intent behind these principles and then mapping them to the common technical requirements that where we do, there's actually real tangible work in research that can be applied. So this will be things like robustness, fairness for cybersecurity, that would be prompt leakage attacks and so on. So this was really what the first phase of the effort was. And it was kind of a, again, a big collaboration.
Petar Tsankov [00:18:57]: And right now what's actually interesting is the EO AI office. So we are also very closely, you know, this is not something. We have been in contact with the EU AI office and the European Commission about this effort. So they have been very positive. You know, we're really welcoming this work because they realize they also have to do it because otherwise they themselves are getting a lot of criticism that you're putting something out that is not actionable.
Demetrios [00:19:22]: So you've been steeped in this for the past, I don't know how long. So. And when you say terms like the EU AI branch does xyz, I have no idea what that means. Like the EU AI branch is that. How many people are we talking here? What is their profile? I know there's some lawyers, there's some technical people in there, and this is, I also know that this legislation goes through many phases and then it gets passed and then this happens and maybe it gets appealed. So can you just break down the whole law system and branch of government that we're dealing with in the EU here and what that looks like?
Petar Tsankov [00:20:08]: Yeah, I probably cannot, I don't, I don't fully understand it myself either. But we do have a, you know, we do understand which are the core offices within the European Commission. There's public portals where you can contribute, could contribute this work. And the way they work is again, by setting up these Special working groups with external experts that are capable to kind of fill in these gaps that they would not do on their own or that they cannot do on their own. Meaning that you don't want, for example, the EU cannot create a benchmark and endorse like this is the benchmark for compliance. This is not the intended goal for the EU AI Act. The goal is to outline, to have the legal document outline the principles and then through the help of these working groups, translate it, make it actionable. And then other organizations would provide, like us, would be providing benchmarks, evaluation frameworks to demonstrate compliance.
Petar Tsankov [00:21:07]: So this is, so it's really. There are key, I would say there are key people within the office that are, you know, driving these initiatives.
Demetrios [00:21:17]: Okay, yeah. And what type of Personas are you dealing with in these different branches? Because that always fascinates me. Is it like a school teacher that decides they want to do some AI on the side or are these folks very well versed in AI And I would imagine law too.
Petar Tsankov [00:21:36]: Yeah, I would say that. I mean it is the one that we have been in contact with. So very well versed, well versed in, you know, low. So not, not, not, you know, don't imagine like engineers that are writing Pytorch code and trying to train, training models. So, but this is also needed. So I also don't want to be, you know, I think that's, that, that, that does make sense because ultimately it is, that's the goal. You have to have the end to end framework that makes sense from a legal point of view. And there are also things, you know, many things we also acknowledge are non technical.
Petar Tsankov [00:22:08]: Right. So there are many things that go beyond this. So kind of what we can contribute and help is exactly on the technical aspects, how they interpret them and how do you benchmark them? Because that's really kind of the key. And that's where we've been having our core focus.
Demetrios [00:22:26]: And so now when you look at these different models, the large language model providers have anything stick out to you as far as, oh, I wouldn't have expected that model to be failing on this compliance issue or this toxicity, whatever it may be.
Petar Tsankov [00:22:43]: Yeah, there were a few interesting findings, I would say. So overall the results were not kind of terrible. So the models did perform fairly okay. On many of the principles. So that was kind of first positive thing that we're not in a situation where models are really collapsing and dying across the, the benchmarks. But what was very visible is first that definitely a lot of the models are heavily optimized purely for capabilities and performance because they perform very, very high scores on capability benchmarks. But on some of the compliance benchmarks, you do have a big gap. So you're talking that, let's say if it's on the compliance benchmarks, performance drops to 0.5 or 0.6, which is really quite low.
Petar Tsankov [00:23:32]: Another very interesting thing was that there were some kind of differences. If you look at close and open models, that actually made sense. Like if you look at. So the act has one of the principles is that like cybersecurity capabilities, meaning that you don't want to leak prompts or other. To put other guards and defenses built into the models. And if you look at the close vendors, like OpenAI, for example, so anthropic. So they have really spent efforts to make sure that their models are secure, which makes sense because you just have an API, you don't know what's inside. So you have to kind of protect what's behind what's behind this API.
Petar Tsankov [00:24:11]: But then if you look at the open models, like Mistral models, they didn't perform so well, which kind of makes sense. Like, why would you invest so much time to protect the model? Because it's open, it's out. If I have white box access to this model, I can make it do whatever I want anyway. So that was kind of surprising but also logical and another, I think logical thing that we saw for some of the benchmarks that have very direct PR kind of implications. The models were very optimized, specifically toxicity, because all the model vendors, everybody tried to get them to do some. Get aggressive and talk. Yeah, nonsense and things like this. This was just basically what the media was capturing at the beginning when the models were coming out.
Petar Tsankov [00:24:58]: And they have definitely. Everybody has spent a lot of effort to optimize because the benchmarks perform, you know, the models perform very well across these benchmarks. And I want to add, I think that's actually a very kind of positive sign overall because this means that whatever we are kind of the vendors, the AI vendors care about and are aware that this is bad, we have to fixates. We have before we release, they actually have been able to make solid progress. So that's very positive. And that kind of means that it's just a matter of kind of providing this global visibility. Well across all the principles for the ACT and all the regulatory requirements, if they have the visibility on how things perform, they would be able to also make progress. So that's overall very positive to see as a trend.
Demetrios [00:25:48]: Yeah, it's almost like you can't improve what you don't measure. So if you're able to measure it, then the improvement will happen. But I also wonder, when you're talking about these benchmarks, what are some examples that you're running the models through?
Petar Tsankov [00:26:07]: You mean what specific benchmarks we have run? Yeah, so these are of course specific, depending on whatever the requirement is. But let's say if you're looking at toxicity as a simple example, that would be there's well known also available benchmarks instead of prompts that kind of try to trigger the models to output toxic outputs. And then it's a matter of just running the prompts, getting the outputs and then using a toxicity classifier. There's also plenty of those from Hugging Face and other vendors to assess how toxic is the outputs. And then that makes it then measurable for this specific requirements. But that's again just one example and many of the other technical requirements that we had to assess were pretty challenging, I would say, meaning that the benchmarks themselves are very limited. So if you think about things like copyright, for example, so the model should not be using copyrighted contents for training and know, you know the examples from. There's many lawsuits now from New York Times and other organizations that are pursuing this.
Petar Tsankov [00:27:18]: But it's very hard to test this because you just don't have a global overview of all the copyrighted content in the world so that you can go and assess the models. So ultimately what you end up doing is. And that's what the current benchmarks do. They would take a set of copyrighted books and then you can do, you know, specific kind of your limited on what you check. So what this means is that because the benchmark itself is not complete, it's. Yeah, you just don't have a complete knowledge. So you would have to either rely on other people checking if their own copyrighted content has been part of it as a way to police this, or. Yeah, so it's not clear basically how to handle this.
Petar Tsankov [00:27:55]: And there's multiple such benchmarks that's just kind of hard to make them fully complete. But at least this is a good first starting point that you can get like for kind of for the basic, you know, for the obvious things you could already evaluate and see how the models perform.
Demetrios [00:28:12]: Well, it feels like that could fall flat too specifically with the copyright because you as an end user of a model do not have access to the training data.
Petar Tsankov [00:28:28]: A lot of yeah, but you can test. So if you have the model, you could still there are methods to test if the model has, you know, if it produces like very close to almost like verbatim specific contents and there's ways to kind of trigger, trigger the models to generate this, then you can with very high confidence. And then if you go to chords, then, you know, I don't know how they would set up this. Maybe there would be some way to force the vendors to provide access to this data. So, and that's maybe to take as a side remark, is still globally an open point? Like, how would, how would you, how much would actually AI vendors expose for compliance and general, like risk assessments? Because a lot of, as you said, you know, if you just expose the training data, that becomes very easy. I can just kind of grab the training, you know, search and try to find if, if they're using my specific operated content. But they would have to expose and they would not want, of course, because that's very often the core IP kind of thing that you want to protect. But one big trend that we do see right now kind of beyond the AI vendor is also generally how, where the world is going.
Petar Tsankov [00:29:43]: So Gartner is explicitly actually teaching or kind of mandating procurement departments in the contract. So if you're, let's say you're buying an AI solution in the terms, to have explicit terms that would allow for white box assessments of the models, meaning that let's say if I'm buying a solution to identify, like, to reach, let's say, identity cards or some other, you know, critical, critical task, then I would need, you know, you may want to put this in the contract so that you could go and validate properly. And I think that is a way to go because you cannot. Yeah, you just need white box assessment for some of the. To. To conduct like really thorough validation of these models.
Demetrios [00:30:28]: Yeah. Unless you are a vendor that is using one of these large models in the background. And then how are you going to. It's like, well, OpenAI or Anthropic doesn't give me whiteboard at box access. So how am I going to give that to you?
Petar Tsankov [00:30:45]: Yep, that will be tricky how to resolve this. So this is, I think still this will take, you know, maybe when we do another podcast in 12 months, we'll see, we'll be more clear, we'll see where things go. But this is one of the kind of tension points now that we see because definitely organizations demand. So the demand, I mean, they don't need the rights just to inspect the training data. They just need proper access to validate that the models would perform if they're used for a business critical use case in the organization. And kind of one way to do is you go to the, you know, you almost have to force the vendors to do it and they are more willing to do it with third parties that have limited access. But ultimately that will have to be result in a kind of more invasive way for the vendors, which is possible by the way. So you could, so you don't necessarily have to expose everything to whoever is buying the solution.
Petar Tsankov [00:31:47]: So you could. These are, I mean it's not like you would go and manually start reading the parameters and reading the training data. So in any way you're automating the benchmarks, the tests that you're running. So there is a way and we're looking into this kind of. How do you deploy a specific kind of solution that would run all the validation locally at the vendor and then only expose the relevant results for compliance or risk or whatever it is only that gets exposed to the client. So that's also another way. But you would again have to be, you will still need to get the vendor to actually deploy your piece of kind of checking software to export these results.
Demetrios [00:32:28]: Yeah, I wonder if we're going to see some type of SOC2 or SOC AI type of certification that will come out and that becomes the norm.
Petar Tsankov [00:32:40]: The norm.
Demetrios [00:32:40]: Like we have SOC 2.
Petar Tsankov [00:32:42]: Yeah, yeah, definitely. We do see also for governance now there's specific ISOs that are coming up, but there's still to be determined which ones would be the main ones. Because there is. The reality is there's more than 100 different standards. ISO alone has more than 25. So damn, it's overwhelming. So you need to have a bit simplified this a bit.
Demetrios [00:33:07]: All right. Well the EU AI act definitely defines different categories and I'm wondering if you've seen any specific gaps in these EU categories like cybersecurity, resilience or fairness or the copyright writing.
Petar Tsankov [00:33:27]: So that's a good point. So there are things that are just. There's not non checkable but almost like not a well defined way how to check and then we can argue whether then it makes even sense to have this. So this would be an example, would be the principles around interpretability, explainability of the models. Well, there's not well defined way to actually technically do this. So how are we then going to really properly benchmark and show evidence for this? So these are the areas where kind of it's inevitable that you need to do like close iteration between the high level and the low level just to make sure that these Things ultimately match well together. And I think that's a lesson that also future similar efforts, regulations and so on, will have to be developed in some closer way. Because right now it was just done in a waterfall way.
Petar Tsankov [00:34:23]: It's like, okay, let's define a high level. Hopefully this can translate to something meaningful in the technical layer. And there are some gaps, meaning that not everything is translatable. So I think in the future that would be done ideally differently. So I think they were just rushing basically to. It's a matter of time. Also. You cannot do everything in such a short period of time.
Petar Tsankov [00:34:47]: So that's one example where things were just basically could not be translated in a meaningful way. And this is now communicated. So we'll see what the reaction would be on the other side then in terms of the other principles. So I mentioned some of them, like cybersecurity, we saw some gaps there across closed open models. We saw that in general, one general trend is that the benchmarks themselves, the compliance benchmarks, are just more limited. Like, if you look at capability performance benchmarks, like, there has been a lot of efforts to define those, and these are very good at differentiating how good different models are. That's not the case for compliance benchmarks. Like, if you look at fairness, actually, most of the models have.
Petar Tsankov [00:35:31]: Most of the models have almost equal scores. So that doesn't necessarily mean that the models are equally fair. It could be that just the benchmarks are not complete enough to differentiate and show how the models differ.
Demetrios [00:35:45]: Oh, fascinating. So basically the benchmarks did not have. There weren't enough samples of benchmarks. Yeah, and it wasn't robust enough. And so it's almost like that when the working group gets together and starts to do some work for this EUAI act, that's one of the things that they can do. They can dig into creating more robustness for these different benchmarks.
Petar Tsankov [00:36:13]: Yeah, exactly. Because in a way, the effort goes on two layers. So one is just to translate them into. What do you need to check? Let's say it's robustness, cybersecurity and so on. And then you map this to specific benchmark or evaluation method. Doesn't necessarily have to be a static benchmark. It could also be something that you dynamically generate by kind of looking at the responses of the model. And then the effort needs to be also at that level, kind of to make them comprehensive enough.
Petar Tsankov [00:36:45]: And I do think that a lot of the focus now would shift towards this because now that there is a global realization that it's not just about Capabilities, but also about safety and compliance. Now there's quite a lot of effort also on that layer, so progress will be made eventually.
Demetrios [00:37:05]: And do you think the safety and compliance is more of an afterthought? Like you still have to, you have to know that it's capable of doing something before you go to the safety and compliance step.
Petar Tsankov [00:37:20]: Right.
Demetrios [00:37:20]: But I think a lot of times, like you said, so much is put on the capability. Can we get there? And then safety and compliance gets shoved in at the end and trying to like, okay, we, we got there, we can see that it works. Let's now cross our T's and dot our I's.
Petar Tsankov [00:37:41]: Yeah, I think that, I mean, especially it doesn't make sense to push this to the, to the end. It's like, I mean, in code security is the same issue, right? You don't just write code and then start. So you do this from the start. Typically you don't do it at the end. It's much more efficient, much more meaningful. So, and that's ultimately the goal here. If the evaluation frameworks, the benchmarks are readily available, so you could kind of quickly see how you perform, then it makes sense to do this early on because it does affect, it does affect how you build the model. So you don't want to kind of have it ready and then spend like another six months trying to re.
Petar Tsankov [00:38:21]: Optimize it in a magical way also for compliance, because you have to do it. I think it would be, ultimately it would be done very early, like quite early on. Meaning that these benchmarks would run early, but they just need to exist and kind of, that's the key point now. Well, now it does exist, so there's no need to, you know, there's no need to ignore this basically. So it's, it makes it much easier for vendors to plug this in.
Demetrios [00:38:49]: And how do you see people using this? Is it just that they will plug this into their test suites or their evaluation suites and have it as one extra layer of evaluating their whole system?
Petar Tsankov [00:39:03]: So that's a, I guess a more complicated question here because it depends, like what do they want to achieve, right. And earlier mentioned also like safety and compliance, like what do they actually care about? So, because a lot of this, and ideally these things are very well aligned, meaning that, so compliance, basically the regulatory compliance aspect. So this just makes certain some things mandatory. So typically you want this to be a subset of the general broad things of safety, things you care about. So these would be kind of the things that we all globally aligned as we want and you can watch whatever else you care about for the business or for whatever motivations. So then yeah, so this is kind of one key point like what is what, what do they want to achieve? So if you do fall into a category where you must be compliant, then it's kind of easy answer. Then you know, you just have to plug this in and then how the way you would use it is very early on that would become not part of as important as the capability performance metrics because it's a must do thing. Right.
Petar Tsankov [00:40:09]: So that's kind of one use case. And here we do expect that both vendors, but also the companies that would be organization that would be auditing, ultimately these vendors, they would also rely on, they would have to rely on such benchmarks because let's say you want to validate an application for compliance, you just cannot do it unless you, unless, unless you run such a benchmark to get the real technical metrics that's going to demonstrate compliance, technical compliance with respect to the Act. So that would be one use case and kind of the auditors themselves, but developers as well, I think they would also use them for the things again that they care about. So again, and we do see this already being a priority, like toxicity is already a priority because you don't want to deploy a benchmark that provides toxic outputs. And now just this kind of gives you now more comprehensive evaluation benchmarks to pick from to kind of make an even better safer models internally.
Demetrios [00:41:13]: And do you fear overfitting on these benchmarks and not really getting a good understanding because now that there are benchmarks and people do know that regulators are going to be using the benchmarks or auditors potentially are going to be using the benchmarks, there's an easy way to counteract that without actually getting to the root of the problem. Right?
Petar Tsankov [00:41:39]: Yeah, that's the definitely. I mean it's a challenge also for it also determines how you are building these evaluation frameworks. So I have seen several, I'm not going to name like the providers of these benchmarks, but basically they would not allow you to run the benchmark more than once. So what is this? So you do need to make sure that these benchmarks are dynamic enough so that you cannot overfit like this. So it's a kind of responsibility on both sides. Also the benchmarks need to be smart enough to not allow you to overfit to those. Otherwise you have to either hide them and do some other things to ensure that organizations are not overfitting to do to those. Because you could if you have.
Petar Tsankov [00:42:22]: If it's a static benchmarks, I mean. Yeah, that's a very hard thing to solve it.
Demetrios [00:42:29]: It's just. It's been happening for the last two years. Right. And I think a lot of the sentiment that I've been seeing out there is that benchmarks are really kind of bullshit because of that, because models just can get over fit or all of a sudden it's used in the training data and boom, they pass all benchmarks. And then when you give it some similar questions but that aren't on the benchmarks, they fail horribly at it.
Petar Tsankov [00:42:57]: Yeah, yeah. That's why they should not be only static. So they have to really be dynamic, like looking white box, looking at the model, trying to understand like where to go next to evaluate it. And this would of lift the barons and makes it make it much harder to overfit to them. You have to do this. Yeah.
Demetrios [00:43:15]: The other thing that I was thinking about is do you foresee a world where there are different tiers of compliance for this or. Let's say that I really want to be sure that everything checks the boxes. So I'm going to. I'm going to use the full package and throw everything at it. But then there's another use case where I want it to comply, but like I want it to be C level compliance. I don't really necessarily need to make sure that it passes with flying colors. Or do you think that it's just kind of going to be one size fits all? Compliance is compliance.
Petar Tsankov [00:43:57]: Yeah. So that's a tricky one. So I do, I do, I do. See. Definitely see, compliance is a Boolean thing. That said, the benchmarks themselves, the evaluation framework does give a score. Right. So it can tell you your robust 0.85%.
Petar Tsankov [00:44:13]: That's like specific score you get. So you could kind of optimize to some level and kind of the question would be then, well, who would set the thresholds and what thresholds would be accepted as compliance? Once you have a threshold is Boolean, you're either below or above the threshold and then you can talk about being more compliant, meaning that you probably given like way beyond kind of the threshold so that you're very compliant. Right. So that's an interesting question. I don't have an answer on how would. Exactly. How would we exactly set the thresholds? It is in a way very interesting that the EU AI act is a very. It is really a deep tech regulation.
Petar Tsankov [00:44:52]: It is a technical topic where you actually, I mean, just look here, the discussion we're Talking about benchmark thresholds and so on, which is kind of unusual for these type of regulations. So I expect that there would be kind of a commonly understood evaluation frameworks and threshold that basically would keep you safe or keep you compliant. But it's not very clear yet how exactly that would be defined. So I imagine that would be most likely a group of the organizations that would be providing this compliance service. They would have to commonly agree on what doesn't get sued for. Good. Because if you don't get sued for being in compliance, then it's kind of okay. But we're not there yet.
Petar Tsankov [00:45:43]: So this is now the first time the evaluation framework was created. I mean this is really the first technical interpretation. So at least we have some scores and now we can think about, in a way it's very good. We're actually talking about, you know, we can talk about these things like how much is, how much is enough now that it's concrete and measurable.
Demetrios [00:46:02]: Yeah, it's bridging that gap between the law jargon and what we can implement and the actual technical possibilities now. And so how does this reflect in what we're doing and what we're building? And so it's, it, it's nice that you came out with benchmarks to be able to say, look on these levels that the EU act has mapped out specifically we have certain ways that you can test for that.
Petar Tsankov [00:46:33]: Exactly, yes. And this is really just to maybe kind of abstract it a bit more. So this is really a very, I would say, general problem for AI risk. So it's not just the EO AI act, this is just one example, like business risks as well, like you have specific requirements for the model. So these tend to be very, very high level and you need to go through this process on mapping them down to the technical layer. And then like comply is really just one instance on how you solve this. How you take the EU AI act and translate into technical requirements and concrete evaluation methods then underneath to measure these technical requirements. But this is kind of a problem that you see for different regulations, for different standards, for custom business requirements.
Petar Tsankov [00:47:20]: And there things get even more interesting actually and more and harder in a way because then for what we did here with the act and what the EU Commission is doing with these working groups, the code of conduct, you can actually sit down and do this mapping. But imagine then how you would be validating arbitrary models for custom business requirements where you don't have this mapping. So how would you actually go about doing this? You would need to have these expert AI validation teams, somehow or whatever fancy technology would exist in the future that would be able to provide this mapping tool to validate models properly.
Demetrios [00:47:59]: You mentioned this right now is just for LLMs. Do you see it having a world? Because as the Internet likes to say, everything's going multimodal. Do you see it going into the multimodal dimensions next?
Petar Tsankov [00:48:19]: Yeah, for sure. So just. I guess my answer is stay tuned. But yes, for sure. So I mean once the technical interpretation is done then. So the good thing is this doesn't have to be repeated because you can basically then extend the mapping from technical requirements to benchmarks or to end to evaluation methods that support this multimodal or large vision models or whatever the type of model it is. So this is kind of the harder part has been done the thing that actually takes one year and now it's more about extending it with additional technical capabilities to support more modalities. And you have to do this, that's clear.
Petar Tsankov [00:49:00]: But we had to keep it kind of more limited in scope so we could release something tangible already, but for.
Demetrios [00:49:09]: Quote unquote, traditional ML. How does this reflect the EU AI Act? How does that reflect. And specifically maybe these benchmarks or the way that we can take the law jargon and translate it into technical actions. What does that look like, if any. Have you thought about it?
Petar Tsankov [00:49:30]: I mean for like classic, like supervised machine learning, like how do you.
Demetrios [00:49:34]: Yeah, like a fraud detection model or a recommender system or whatever type of thing.
Petar Tsankov [00:49:38]: This also applies there as well. So if you look at kind of more broadly at the act, so that you have the, because you have the different risk categories for Whedon, you have high risk. High risk. Also there's a bunch of requirements, regulatory requirements that do apply. They are also covering the same thing, need to adhere to the same principles, so fairness, robustness and so on. And then for those, the same process applies actually. So you just need to, then you have a model that could be just let's say predicting salaries or being used for recruiting software to hire candidates. So you would need to then show the same, go through the same principles and then implement the same technical requirements, but for, for those models.
Petar Tsankov [00:50:22]: So actually what we did in this work is not just look at the general purpose AI sections that talk about these foundation models, but look at all the regulatory requirements for high risk AI systems and the GPAI models as well. And then kind of we took the union of all the requirements and then mapped those to technical requirements. And then the concrete implementation of these technical requirements was done for language Models. But you could do it for the other supervised machine learning methods as well. Because these methods, they do exist as well.
Demetrios [00:51:00]: Yeah, so for example, like a recommender system, how would that look?
Petar Tsankov [00:51:05]: Yeah, so I mean very simple example would be, let's say one of the principles is around robustness. So then there's very well defined methods for testing robustness for recommendation system. So for example, like small change to input should not dramatically change the output. So this you can start testing and getting a concrete score on how robust is the particular model. So very similarly again just you need to do different type of methods than the ones used for the generative models.
Demetrios [00:51:43]: Yeah, excellent. Well, when it comes to risk, basically AI risk and risk mitigation, what do you see as the differences between the two of those?
Petar Tsankov [00:51:59]: Yeah, so AI risk in general. So this means that how could this impact in the context of an enterprise or organization? So what are the potential possible risks? There's legal risks. We saw this like you deploy a model, it's biased, somebody shoots you for this misinformation. So generally again these are lawsuits that could trigger Reputation risks are also toxicity was a particular principle that really affected, affected the reputation of these companies that are ultimately losing their valuations. Their valuations go down. Compliance risks is also, that is a subset of the general risk. That's one specific risk that you need to be careful with. But I see general risk mitigation as a kind of broader topic that covers all the possible, including the custom business risks that could be impacting the organization.
Petar Tsankov [00:52:56]: Compliance is only a specific subset that's more well defined with more well defined regulations and so on. This is the commonly agreed thing that we need to satisfy. And then in broader risk mitigation we can add whatever else is also could be a potential risk for us. So for example, one of the AI assessments that we did for one of the Swiss banks, this was classic model that predicts prices for cars. Like specific example. Then that was not a particular compliance risk. But of course it's very, very important that this model predicts well, accurately the prices because this could affect directly affect the business and results in serious money, loss of money. So this is kind of custom risk that's for this model within the, within the organization.
Demetrios [00:53:45]: Yeah, it's almost like you need to be able to translate the business requirements into the potential risk that you're willing to take on.
Petar Tsankov [00:53:57]: And that's very hard.
Demetrios [00:53:59]: Yeah, very hard.
Petar Tsankov [00:54:00]: Because you need to have also the like the domain knowledge for the specific use case. Like what does this mean? Right. So it's a challenging thing and that's what we need to deal with ultimately.
Demetrios [00:54:14]: Yeah, it's funny because it's so hard just to translate the business requirements into the technical requirements and then also having that other side of. Okay, what are the risk factors here? So thinking and playing 3D chess in a way.
Petar Tsankov [00:54:33]: Yeah, yeah. Because then you just need to really understand details. Like in this case, you understand, well, okay, this price, these predictions affects the, you know, affects you can lose money basically. So then what does this mean? Well then I have to evaluate the performance and kind of bounce the gap of the, of the error. But then how do you. So then you keep thinking, well, how do I reliably measure the error? Well then I need to have a very good representative data set. So then let me add a specific standard that applies about data quality and kind of checkmark the data. Otherwise I cannot even have reliable model performance evaluation.
Petar Tsankov [00:55:11]: So you have to go through this creative process that would. Given all these things that I have done, I have high confidence that this is going to be okay. So this is something that needs to be more streamlined. I would say right now this just doesn't exist. Like if you say, well this model can result in xyz, then how do you even get started? Yeah, it's very hard topic.
Demetrios [00:55:37]: So you hinted at what you're thinking about when it comes to creating the different benchmarks or the comply for multimodal. What else are you thinking is next for you?
Petar Tsankov [00:55:52]: Yeah, so one big topic is given that now this is available, publicly available, so definitely working directly with the AI, the big AI vendors. So we're starting meetings next week to help them run the framework, explain the results so that they're under clearly unambiguously interpreted and then kind of to calm, not to calm the vendors, but just to show them basically how you can also optimize the model for compliance. So that will be one big effort internally that's kind of non technical. This is just outreach to these vendors to help them out. And the second one is the second immediate step which has been ongoing, is also more closely working with the specific working groups within the EU AI office that are doing this because they are like literally taking this as a first step, first draft of what they need to do and ultimately, you know, to solve this problem you need to, you know, we are aware that we need to refine how the mapping was done, we need to expand the benchmarks. So it's kind of now going into this next level effort that is not just internal within the organization that we collaborated with, but it's kind of at the level of the EU Commission trying to make sure that it's properly mapped out. And this is very short term. So the output of the code of conduct is, I believe, April next year.
Petar Tsankov [00:57:22]: So there's literally a couple of months until more official interpretation have to exist. So they're working on very, very tight timelines. So we're, you know, doing our best also to support in this.
Demetrios [00:57:33]: So if we can use LLAMA next year in the eu, I am going to be thanking you for that. And if we can't, I will blame it all on you.
Petar Tsankov [00:57:44]: I take it as a challenge. Let's go.