Evolving AI Governance for an LLM World
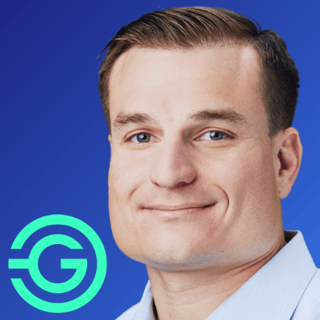
Diego Oppenheimer is a serial entrepreneur, product developer and investor with an extensive background in all things data. Currently, he is a Partner at Factory a venture fund specialized in AI investments as well as a co-founder at Guardrails AI. Previously he was an executive vice president at DataRobot, Founder and CEO at Algorithmia (acquired by DataRobot) and shipped some of Microsoft’s most used data analysis products including Excel, PowerBI and SQL Server.
Diego is active in AI/ML communities as a founding member and strategic advisor for the AI Infrastructure Alliance and MLops.Community and works with leaders to define AI industry standards and best practices. Diego holds a Bachelor's degree in Information Systems and a Masters degree in Business Intelligence and Data Analytics from Carnegie Mellon University.
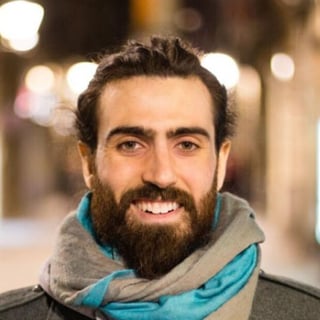
At the moment Demetrios is immersing himself in Machine Learning by interviewing experts from around the world in the weekly MLOps.community meetups. Demetrios is constantly learning and engaging in new activities to get uncomfortable and learn from his mistakes. He tries to bring creativity into every aspect of his life, whether that be analyzing the best paths forward, overcoming obstacles, or building lego houses with his daughter.
As new LLM-driven applications reach production we need to revisit some of our traditional AI Governance frameworks. Diego will provide a brief introduction on what is changing in a critical step to seeing more of these applications go live.
Um, our super cool t-shirt. Okay. Actually, maybe I'll give the floor to someone else. You talking about me? Yeah. So I just had to come over here because I've been getting a lot of crap from our next speaker. I had to come over here and let 'em know how Everybody that is on this track, you should go over and watch the track number one.
Cause this one, you're not gonna get anything useful from Diego right now. Oh, what's up man? I just wanted to rub that in your face for a minute. I left the guys hanging, I left the fireside chat hanging so that I could introduce you right now. And I can't hear you. You see now? Oh, there we go. There we go.
Okay. You even got your shirt on. Oh, look at that shirt. Look at that shirt. So if anybody wants to buy that shirt, you can find it right here. Diego keeps asking me for this specific one. I hallucinate more than chat G P T, but I already sent you one man. We're a community that is. Funded by Great. Uh, actually, your old company was one of our first sponsors.
Let's just say that right now and give a huge shout out to Arrhythmia your old company. And also, I am going to come clean a lot of the, no, where is it? The report that was right here, the report that we just wrote, and we just came out with. I kind of copied the structure from the last arrhythmia report that you guys put out.
I'm gonna come clean and say that Asan, you know, like it's, uh, whatever gets the information out. It's, it's all good. Oh, that's not, that's not what you were telling me on WhatsApp. You're being all nice on the live stream. Oh, I love it. All right, man. Well, I'm gonna let you talk cause I got a, I got a fireside chat to get to that everyone should come over and watch.
Uh, but I'm sure you got incredible information. Diego's, for everyone that wants to know this Diego's uh, talk at the last. Conference. It was one of the most viewed talks, so I have quite high expectations of this talk right now, and I may be zoning out of my own fireside chat because I'm trying to follow along with what you're saying.
Diego, I'll get off the stage now and I'll see you in a bit.
Great. Hey everyone. Uh, happy to be back here at the conference. Uh, so, uh, it's a quick talk. You know, everybody's favorite subject, which is actually kind of like governance and compliance. Uh, so the general idea is that, um, for, for those of you who don't know me, I'm Ggo Oppenheimer. Currently, the uh, uh, A partner at a, uh, AI focused fund.
I used to run a company called Arrhythmia. I've been, uh, I worked at DataRobot for a while. Um, I was in the space of model risk management and governance of ML models for a long time. Uh, and I thought it would be really interesting to kind of understand a little bit how this needs to evolve in an L l M world.
Uh, obviously it's a very short talk, but, uh, you know, I'm happy to chat more, uh, later about this. So, the general idea here is that, you know, why, how do we have AI governance? Like, why does this exist? Why do we care about about it? And it really is because, um, If you're, especially if you're in a, kind of like a, in an industry that has some sort of regulation or compliance concerns, you know, we really need to have an understand how this technology is used, uh, what kind of accountability and, uh, responsibility we need to take for AI actions.
You know, how do we actually put, provide safeguards for privacy, protecting personal data, uh, in particular in this kind of like world around like what are the kind of. Guardrails that we need to build to ensure robust, dis phony and risk assessments of these AI systems. And this is not about, um, you know, kind of fear-mongering in any way.
This is literally about like, how do we become tactical and practical about applying these kind of principles and these, uh, you know, in a, in a way, Um, that, you know, is, is useful and that we can use this technology, which is some of the greatest technology that we'll probably see in our lifetime. Uh, you know, to, uh, things that really matter, you know, like medicine and the government and financial services.
You know, like things that really kind of like move forward humanity in one way. Uh, we need to come up with these systems so that we can use them in a, in a proper way. And a lot of the governance principles are really have a historical content of, you know, why, why, first of all, why do they exist? Super rapid, uh, advancement in AI technology.
I think anybody on this, uh, you know, on this conference can probably attest to that. Uh, but also it's actually building on. Traditional governance frameworks, things that already exist in it, which are really about like, how do we do security? How do we actually understand that we're strategically investing in the right things?
How do we look at, uh, you know, the ROI of our investments versus the risk that we take? Um, and this is really about kind of like prioritizing compliance, fairness, transparency and accountability of AI systems. So, One of the things is like, if we go look at, I think calling old ML is not the right thing, but like previous two foundational models, right?
Kind of how AI governance workflows, uh, really were, uh, really about right. And they have a couple categories that really matter, especially if you were in financial services or in government or in, uh, you know, kind of like life sciences. You, you know, we're usually required or should have had a, um, the, um, You know, complete catalog of models, uh, which would had, uh, you know, model risk documentation, description of the sources, uh, training predictions, how you got there, what methodologies you use.
You know, you were usually looking for, hopefully having a flexible, uh, model risk management framework, which kind of give you a gradient of like, this is a high risk model. Hey, we're using this for, you know, credit decisions versus things that we're like, didn't really matter. It's a model that we're using maybe for.
You know, kind of marketing decisions where like, you know, they, they need at least, least scrutiny. So understanding like what level of scrutiny you needed to put on a model perspective, uh, really mattered, you know, but you looked at kind of like where the data came from, how it was developed, what algorithms were used, uh, you know, to develop it, how you trained it.
Um, you looked at. You know, how you would have an efficient process, you know, really around ML ops to integrating into legacy systems and data architectures of those models. Um, what you look at how, kind of like it kind of generated tooling to be able to operate, manage, monitor some of these models and, and their health and production.
And you know, you would look at the kind of like tools to model that model accuracy and data consistency. Uh, you look at why the model changed, if it changed, and you would record those things and then you would try to have some level of STA standard audit and report logs around like, What's happening with these models, who's using them, how they're being accessed, et cetera.
And, and so that you could provide that, especially if you were in, uh, financial services and you might have auditors, or you might have a, uh, you know, some level of, you know, have being able to help to give this out. So the interesting thing, if you look at these, uh, you know, kind of like, uh, you know, it's very model centric, right?
It was really about how did we get to this model, how we built this model, how did we put the, like, the elements that we put into that model. And some of this actually like actually doesn't really translate. Um, into the new L l M world, and I'll explain why. So some of the ch you know, challenges that the traditional AI governments are gonna have, right?
So there's just like a lack of documentation of how we actually trained in these models and where these data sources came from. And it doesn't necessarily mean that you need that, but like I'm just saying like it's very hard to actually have provenance of saying, Hey, I know exactly where all these data sets came from, where we actually got that data, how it was actually trained, and how we got to that.
Um, We see this kind of like uniform model based approach of risk management. Like is this a high degree of model, uh, risk model? Is this a low degree model? Well, in this case, we're sometimes using the same foundational models or these large language models and. We are maybe using them in different instances and in different, they're not fit for purpose, they're more generalized.
So that kind of framework no longer applies to the world that, that we're looking at. Um, we're looking at how to integrate these into, uh, existing, uh, you know, into existing frameworks, into existing legacy systems. And this is actually a fairly complex thing to do, especially today with a specialized hardware that's needed and how you integrate these capabilities into those legacy systems.
Um, the tools. For operating LLMs are changing as we can see, you know, in like half of the talks in this conference. And so how are we actually adapting those for these new kind of frameworks? Uh, uh, for a guy governance, uh, really matters. So, And finally we're like looking at, you know, how do we actually monitor accuracy and data consistency?
Like, does that really matter? What does it mean to actually have drift or degrading input qualities in a L L M world, um, when there's actually changes that we're fine tuning with these, uh, you know, uh, you know, components. Like what is it? Mean for that? Like how, like how are we tracking those? How are we including that in our usage?
And then what does it mean to do auditing and accountability? So these are all challenges that really kind of present themselves when looking at lms. But the one thing is like, does that mean we should not use LLMs? Absolutely not. Right? It means, uh, that we need to start thinking a little bit differently about how do we gonna evolve, uh, you know, into these frameworks and how do we can actually continue using it because, One of the things that's for sure is that the LMS are some of the most powerful machine learning components that we've actually seen, um, in the past, you know, number of decades.
And we absolutely wanna be able to use them. So we just have to find how to evolve these frameworks into a world that we can actually use them and really just understand the risks and where there's a risk and where there's not a risk around that. So, some suggestions, uh, in this very quick talk about what you can look at.
Um, is, you know, how do we think about cataloging where we use the workflow? So it used to be about like what models we have and how we're using them. And I think it's much more important right now to understand the, the, to catalog, the, the workflow, right? If we're gonna be using lms, let's look at the entire workflow, right?
And what versions of what models we're using from the different providers, but also understanding like how we're using them and for what components we're using them. Right. We should include in that documentation what guardrails we're actually building around the calls of each one of those models, and identify any risks that we see for that component, but then also extracting into that full workflow.
You know, we really wanna focus less on the Maha the model was built. That doesn't really get us anywhere and more on the overarching risk of that workflow. Right. Is this something that actually could go wrong? Where can it actually go wrong and try to understand that, right? So the type of error, the cost of the errors and the frequency of the errors is what matters.
But at the workflow level, so then we actually wanna think about risk management, right? And say, okay, well how do we apply that at the full workflow versus just at the model. So let's document the potential failure modes right, of the workflow. And this is the part that you can actually understand. If you actually look, if essentially you look at the box and say, okay, this is the box of risk.
If we can define the outer bounds, we can't really understand where exactly it's gonna fall, cuz you know, these are stochastic methods, but we can understand the, the outer bounds of where they might go off and document that and then accept or reject those boundaries. For each workflow and understand what risk we're taking in each one of those.
We wanna be able to constantly, uh, you know, do constant change management validation. So as LLMs, and especially when we're using external providers, right, you know, are, are providing frequent fine tuning and refinements, how does changing a provider fine tuning on new data are changing versions of, of a model affect that workflow and.
Finally implementing and documenting guardrails that adds structure, type, and quality guarantees to those LM use. So everybody's been talking about hallucinations and you know, what the problem that is, but like actually validating the correctness or not of a workflow and what, correctness is a little bit of an overloaded term, but how do we understand is the l l M providing the proper out, uh, you know, out for that component, the proper structured output.
The tougher P type, the proper quality and guarantees for them. And how can we actually provide more importantly pr uh, you know, corrective action when we actually start thinking about, Hey, this can go wrong. We have defined that boundary, and now this is how we're actually going to go in and, uh, you know, and, and define the corrective action.
This becomes particularly important. So the second, the ability to fall back on documented paths for human experts is a really other one. So even when we go into a world where, you know, we have l l m powered workflows, we should understand the, and have the ability to fall back on a documented path of human experts, uh, when the workflows can't.
Provide certain quality guarantees. So how are we actually gonna understand, hey, we can actually not automate this. We actually need to fall back on something and provide those path is gonna be super important for the this net new governance's framework. And then finally, again, going back to like what is the standard audit report and logs look like?
If I can actually go in and understand every single time I have put implemented guardrails into my organ, into my L L M workflow again. Multiple guardrails, multiple components. We're looking at the workflow level, right? Every single time we've seen an error state or we've had to provide corrective action, we should log that every single time we've got a correct, uh, you know, kind of like component.
We should log that as well. So as we can actually create these audits, reports and logs, you know, we can actually review these workflows. This is how we would actually get not only regulators, you know, kind of content with. You know, the level of, uh, scrutiny that we have for these models, and, but it also actually shows us that the boundaries that we've suggested and how we've suggested those, and the corrective actions, plus the human interventions are noted, and this will actually just increase that assurance around those AI workflows.
Ultimately, the power of LLMs is there, and what we really wanna do is increase AI assurance of those workflows so that we can use them more and more in the components we want. So with that said, I think my time is very much up. Uh, I really appreciate, uh, you taking, uh, some time to listen in today and, uh, if you have any questions, uh, I'll be in the slack or can hit me up on Twitter or on LinkedIn.
Awesome, thank you so much, Diego. I think these frameworks were incredibly helpful and I know I personally am really looking forward to going back through the slides and the recording. Once it's out there and published. So thank you so much.
Alright. Happy dinner. Cool. All right, so.
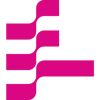