Explainability in the MLOps Cycle
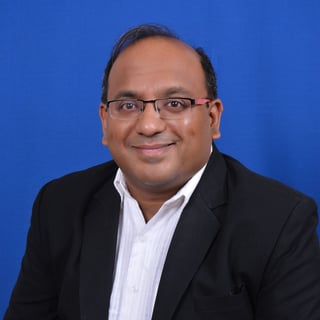
Dattaraj Jagdish Rao is the author of the book “Keras to Kubernetes: The Journey of a Machine Learning Model to Production”. Dattaraj leads the AI Research Lab at Persistent and is responsible for driving thought leadership in AI/ML across the company. He leads a team that explores state-of-the-art algorithms in Knowledge Graphs, NLU, Responsible AI, MLOps and demonstrates applicability in Healthcare, Banking, and Industrial domains. Earlier, he worked at General Electric (GE) for 19 years building Industrial IoT solutions for Predictive Maintenance, Digital Twins, and Machine Vision.
Dattaraj held several Technology Leadership roles at Global Research, GE Power, and Transportation (now part of Wabtec). He led the Innovation team out of Bangalore that incubated video track inspection from an idea into a commercial Product. Dattaraj has 11 patents in Machine Learning and Computer Vision areas.
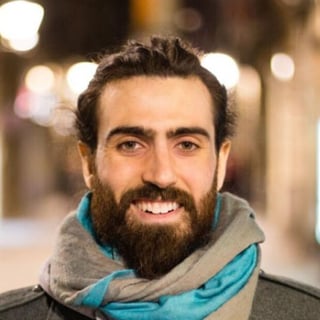
At the moment Demetrios is immersing himself in Machine Learning by interviewing experts from around the world in the weekly MLOps.community meetups. Demetrios is constantly learning and engaging in new activities to get uncomfortable and learn from his mistakes. He tries to bring creativity into every aspect of his life, whether that be analyzing the best paths forward, overcoming obstacles, or building lego houses with his daughter.
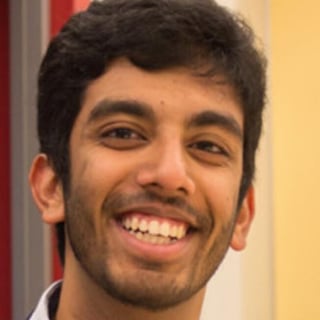
Vishnu Rachakonda is the operations lead for the MLOps Community and co-hosts the MLOps Coffee Sessions podcast. He is a machine learning engineer at Tesseract Health, a 4Catalyzer company focused on retinal imaging. In this role, he builds machine learning models for clinical workflow augmentation and diagnostics in on-device and cloud use cases. Since studying bioengineering at Penn, Vishnu has been actively working in the fields of computational biomedicine and MLOps. In his spare time, Vishnu enjoys suspending all logic to watch Indian action movies, playing chess, and writing.
When it comes to Dattaraj's interest, you'll hear about his top 3 areas in Machine Learning. What he sees as up and coming, what he's investing his company's time into and where he invests his own time.
Learn more about rule-based systems, deploying rule-based systems , and how to incorporate systems into more systems. there is no difference between ML systems and deploying models. It's just that this machine learning model is much smarter than traditional rule based models.
Hey. Hi everyone. My name is Dattaraj Rao. I'm a Chief data scientist at Persistent. I head the AI research team here working on some cutting edge technologies around MLOps, knowledge platforms, responsible ai, and uh, next best action. Huh? How? I like my coffee. I, I'm based in Goa, India and I allow my traditional Indian, uh, south Indian style filtered coffee super strong.
And I will take that over any cappuccino. So that's how I like my. What is up listeners? This is another addition of the Lops Community podcast. I am Demetrios Brinkman, and today I am joined by none other than Mr. Vishnu Racon. Nice, nice. Very good job pronouncing that Demetrius. You know, over the course of our friendship, over the course of the two years, Demetrius and I have been doing this cause I think now we've just hit the two year mark.
That Demetrius's gotten a lot better at that pronunciation. Uh, it started off . Then it went to Racon and now it's Racha. But man, it's not the easiest last name, but I can empathize because I do not have the easiest name either. So I feel it for you anyway, today we're not here to talk about last names. We are here to talk about machine learning operations, and we had none.
Then that garage on the podcast, I'm super stoked about this because he's one of those guys that I feel like was a. I'm just gonna pat myself on the back. It was an incredible find on my part, although there's probably a ton of people that know about him already because he has written a book and he was, he's written one of the OG m l ops books and we talk about it in the podcast.
The book is incredible because the principles that he presents are everlasting.
It's not one of those books that you read and then a year later, as technology continues it to advance, it no longer is valuable. It is. That you can keep on your shelf and refer back to it because of those principles, and you see the way that he thinks it's like that. I mean, I don't know about you. Yeah.
Dr. Rara, just to give you guys a little bit of context here, he's actually the chief data scientist at Persistence Systems, which is a, uh, IT services and software engineering services provider based out of India. And prior to working at Persistent, he spent close to 20 years working in a number of different technology leadership roles at ge, including in their research division, their power division, their transportation division.
And I thought that what he brought was such a great. Engineering mindset to this podcast, this conversation to the machine learning problem space. And you can see that in how he structures his answers, how he structured the book, uh, a very structured thinker, um, which I always appreciate. It helps with the communication and it helps with actually like, you know, figuring out what the insight is that people are offering.
And I give, I give you credit to ris. This was a great find and a great podcast. Well, thank you very much. Dude. One thing that I'm a little bit bummed we didn't even get to get into is he has 11 patents around computer vision and we didn't even get to talk to that at all. I mean, we were so enthralled with all the other cool LOP stuff that he's been getting into my favorite part of the podcast, which you guys will hear more about are the three top areas that he's super interested in when it comes to machine learning. What he sees is up and coming, what he's investing his time and his company's time into. I think that was great to talk about and, and think a bit, uh, bigger about.
What about you, Demetrius? What was your favorite part? I like the end when he talks about the patterns and, oh no, actually, you know what I mean? Along those lines, it was kind of the same thread, but when he talked about how he was going from rule based systems and you would deploy rule based systems into a system, system systems.
And more systems. And then when he made the jump into machine learning systems or machine learning models that you would deploy into systems and how those are effectively the same thing. It's just now this machine learning model is much smarter. But you can look at everything that goes around deploying a rule-based system or a machine learning model into a system and just like, stop me if I say systems too much, but.
Idea around that and how he just really broke down the patterns that are still the same and that you can hold onto and you should be thinking about whether you're doing rule-based or machine learning. I thought that was awesome and that was probably my favorite. Yeah, great conversation. Let's get into it.
Let's do it. Before we do, I want to mention that this podcast is brought to you by none other than super wise. They are a machine learning monitoring tool and I have had the opportunity to meet the whole Supervis team. They sponsor the podcast. They are. What you need. If you are looking for machine learning monitoring on any level at any scale, you can check out some of the episodes that we have done with the founders.
We'll leave that in the show notes. If you want to dive deeper and you just so happen to be looking for some machine learning monitoring tools right now. Check out. Super wise.ai to get all of your monitoring needs met. Now let's go into it.
Dos Raj, it's great to have you on this podcast, man. I'm very excited to talk with you. Yeah, likewise. Thanks Demetrios. Thanks, fish. So you've got a pretty incredible background. You've been in the game, as we could say, for like. Over 25 years, I think. And you studied mechanical engineering at school and then you went on to pursue this software engineering at General Electric, working on developing monitoring systems for rotating machinery.
Can you give us, like just that thread, what drives you, what brought you through the last 25? Yeah, it's, it's 24 actually, but yeah, , it's, it's, it's a, we can round up, man. It's all good. But, um, yeah, so, uh, I, I started off, like I said, I'm, I'm a mechanical engineer, uh, from, uh, Goa, India here. That's where I live now.
Also, So I moved back to my hometown, uh, where I studied and, uh, uh, what I mean, engineering always fascinated me. I guess I always wanted to be an engineer and, uh, to be honest, I didn't, uh, in the academics, I didn't get through computer science, so I was always missing out on, on that. So that was something that I kind of self-learn even while doing my mechanical engineering, I was picking up, uh, learning new stuff in computer vision.
In, in computer science. Sorry. And then, uh, I think what, what really worked is that discontinuous things to learn. So over the 24 years, 25 years, I think, uh, what has really stuck with me, what is driving me is the, the fact that I can learn new things. I get scared that one day I wake up and I won't be able to learn new things.
So, I mean, that's something that scares me. I, every morning I just, whether it's a podcast or reading something on pocket or a Kindle book, I just kind of pick up things. And that's what I like to do. Uh, if you follow me on LinkedIn, I'm, I'm like sharing things almost every day, three or four posts. Uh, just something somebody has done.
Some interesting model that has come in the market on an nlp. Something around lops. A lot of great content recently on lops, uh, including your fantastic podcast. So I think, uh, we do, yeah, some plugs there, , but, uh, yeah, I think that that's, that's what really drives me. So, uh, so after my education in mechanical engineering, I really wanted to do something fun in computers.
I really was not into a classical formula based system. So I got into numerical methods. I built like a finite element website. So it started with Fortran then, uh, cause photon was, uh, uh, was the only language to learn at that time. This was like in, uh, 98, uh, 97. And then, uh, Uh, then I, Java was just coming up some microsystem at ris Java, I think 2.0 at that time.
So I just picked up Java because the interface was nice. So my, my thing on Java was not about cross platform, server side, nothing. I just wanted to know, wanted my code to look pretty on the screen because Java had some fancy charts, which for turned in. And then, uh, that's what I did. I built like a, a. At that time, there was something called Java ate, which was the big next big thing.
I mean, some of you may not even remember, but these, uh, apple was like the, the web browser was the app running s and the web browser was the thing at that time in the two thousands. And that's what I did. Uh, I, I built, uh, a website with cha, uh, based FM software for numerical analysis and, uh, calculating stress on, uh, models.
So, and that was very, in fact, that's the thing that got me the job in ge. So GE was trying to do the sim, something similar. They were building design software and uh, I got my first job in Bangalore. I moved to Bangalore from Goa and worked there for four years building design software. And then I, uh, within GE I got a, another opportu.
I wanted to move out, uh, explore the world a bit. So I moved to the us. I moved to Norfolk, Virginia, and uh, spent like nine years there. And, uh, there we were built, I was working with G Power there. And we built, uh, some, uh, remote monitoring software for gas turbines. That's the rotating, uh, equipment. So this, this was actually an acquisition by g G Power.
They had a very nice novel software, uh, for capturing any anomalies that happened on machines. You could define rules saying that if some thresholds are violated, uh, flag some. and they used to use concepts like even no ML yet. It was purely rule based. It was, uh, fuzzy logic, uh, lot of cool stuff, but very deterministic.
And then slowly we started at G Power getting into, uh, neural networks. Neural were very big at that time when playing vanilla neural networks, uh, your MLS . So that we started getting into that. Uh, and then. Back in, uh, 2013, I wanted to move back to my hometown, um, or actually move back to India. So I got another job through G at GE Transportation, and I moved back to Bangalore.
And then, uh, at, uh, Bangalore, uh, we worked some very interesting work in computer vision. So that was my first official foreign to machine learning where we built really interesting computer vision models, open CV based stuff, a lot of very new computer vision stuff at that time. I mean, Uh, object detection, et cetera.
And which, which became very popular. I actually got like around 11 patents in that domain, uh, where we actually had a product which could, uh, which was where the camera mounted on a, on a locomotive, and from that camera viewed monitor the railway track and find anomalies. So that became very popular and uh, that was one of.
One of, I will say, highlighters for my career. And then, uh, after that, uh, like four years back, I moved, I wanted to move back to my hometown and got this amazing job at Persistent as a chief data scientist. So this was something that, uh, I really wanted to move back to Hometown. It was a very, High position, and I'm actually now at persistent running the AI lab for, for them.
And, uh, this is like, uh, I have some really smart, uh, data scientists working for me. We have some really niche areas that we work on, like federated learning, responsible ai, a lot of work in lops knowledge graphs, uh, next gen NLP systems. So we get to do a lot of cool work. So yeah, that's where, that's where I am today.
And enjoying. But still learning. So I get to wake up in the morning, learn new stuff, so definitely something I enjoy. Awesome, awesome. Yeah, no, thank you for taking us through that. That was, uh, illuminating to to hear, you know, where you started and, and you know, all the experiences you gathered along the way and where you are now.
And where I'd love to dive in is there, you know, is to work at Persistent. What's you're interested in right now? You know, I was taking a look at your LinkedIn, uh, you clearly just wrote a book in Kara, you know Kubernetes. I, I see the, the, the thirst. To learn and the drive to the curiosity in you. And you know, I'm kind of curious from your perch at.
The, as a chief data scientist at Persistent, what are the top three areas of machine learning that you're most interested in? You know, I've seen you share things about large language models. I've seen you share things about responsible ai, ML ops, you know, other sorts of modeling techniques, bio bioinformatics models, all kinds of stuff.
Like what are your top three areas that you're most interested in and that you're champion championing work on at persistent. Yeah, that's a very good question, . So I would say the top three areas definitely will start with the knowledge platforms and knowledge graphs. So, uh, definitely we do a lot of work on, uh, large language models, but, uh, one of the, and persistent, uh, we are like a technology services company.
Like we are a billion dollar company now. We make, uh, uh, uh, and. A lot of our work is for banking and financial sector and healthcare. So those are the two. Most of our customers, two major customers. We do some work for industrial also, and ISVs, but bfs, I and bank and healthcare are two prime and, and a lot of these areas, uh, Just large language models don't, uh, fit the card.
We do need to capture the context, so capturing context, extracting entities, capturing the relations. So knowledge platform becomes very important. You need to be able to explain why you are providing certain recommendation or why you are giving a search result. So explain explainability of this large language models using a knowledge graph.
I think that is one area and I, I wouldn't say we. Uh, figured, but we have some very interesting offerings that, uh, uh, tackle this. So that'll be one. Second area is, uh, definitely around responsible. Uh, lops is something we are doing, but we tend to get more into interpretability and explainability of ML models.
Again, our banking and healthcare customers, uh, we are in a very regulatory environment. We, we have to prove why a particular loan was approved or no, or why a particular, uh, diagnosis was provided to a patient. So interpretability, expandability becomes very important. And then, uh, one of the things that really a lot of customers, uh, I see not enough customers spend time is to understand, uh, ecosystem in production, how the data drifts, how the concept drifts.
So that is one area of lops. I see a lot of gap, and that is another. Think that we heavily promoted, persistent to help our customers to understand the dynamics of the data. And then the third area, I would say would be something around privacy. Preserving ai, again, it kind of falls under responsible ai, but that is one area we, uh, VC is going to grow, grow heavily because, uh, like they say, AI is a new electricity and data is the new oil, but, uh, you are seeing cases where people are getting sued for, uh, not uh, uh, not using data without, with consent.
So, uh, especially in the healthcare space, we, we don't have access to data. We have very dummy data in the public repositories on which we are supposed to build models. So we really see something like Fred Learning or, uh, confidential computing can really help solve that problem, keep the data private, and use only the right insights and, uh, only use the data with, uh, right governance models.
So I think to, to, uh, give a long answer to your original, uh, uh, definitely knowledge graphs. Uh, ML Ops responsible and privacy preserving ai. These three areas, I would say are the, our top focus areas in persistent research. And definitely on my top list. That was extremely illuminating to hear. Thank you for sharing all that.
I always like to ask our guests what kinds of, you know, things they're interested in, what kind of things they're animating them, and I wanna dive deeper into one particular. Which is, you talked a lot about how, you know, large language models, while they're interesting, may not necessarily be sufficient for a task because of the context that's required.
I, I'm personally, like, I'm very interested in diving deeper into that point because, you know, we're really seeing LLMs have a huge moment right now. I mean, um, the, the, the, the interest is very high and we're seeing a lot of discussion around that in our community. And so I was wondering if you could give an example of a use case where an ll.
Was not a good application for the natural language sort of task that you might have used. And I understand that, you know, certain things are, are confidential and, and you can't share the total details. But even a toy example, it would be very helpful to understand that more. Yeah, yeah. That's a, that's a, that's a good point.
And uh, but by no means, I'm saying that LLMs are not, Uh, effective or powerful? They, they're definitely totally, totally amazing. Uh, so the thing, uh, that, uh, so typically some of the traditional use cases for lm like question answering or your summarization. So we saw, uh, some, like, one of the examples we had was, uh, this text research.
Okay. One of the customers was looking at, uh, texts and documents on the internet and, uh, like text bulleting is published and then you would want to. Query that text. Text or this is the like Virginia State text or, uh, some gst. So if there is a text update, how would you extract that information? So, uh, we started with LLMs and uh, we could ask some questions.
We could, uh, extract the text, uh, process. I mean, we started with the pre-train model, fine tune on some existing. Uh, corpus that we had scraped from the, from the net. And then we, we built a question answering system, which was pretty good. It was giving the relevant answers, but we could not justify because everything is, as you know, LLM says, the, the whole idea is they convert your, uh, raw text to embeddings.
And then that embeddings is what is used to find, uh, matching semantically, matching, uh, sentence also, or a paragraph. So we could find the right answers, but we could not justify, uh, like how it would be like a text. So in that use case, uh, what really worked for us would be, uh, we wanted to extract specific entities like which county had how much person tax, and, uh, uh, what was the, what was the imposition type.
So these specific, uh, entities, I would say related to the tax domain. So we, we change the problem. To er problem. So we had specific entities that we extract. We had some relationships that we extract, and then the key thing was building a knowledge graph. So a knowledge graph would then start relating these entities and, uh, to each other.
So if, uh, Lancaster County was an entity, 6.5% was a, uh, was a new numerical entity, and then, uh, say good tax was a imposition. So seeing that Lancaster County from this particular date, from 2006, the sales tax would rise to 6%. Uh, that became a knowledge that we will store in the knowledge. So this particular fact now we could keep it as a sentence in, as an LLM and do a matching, but uh, as you can see, a knowledge graph, because we are showing this as relations, it gets very complex.
Now I can do queries like tell me all counties who have, uh, greater than 6% sales tax since 2016. So now you, you get the structure of your data, you get a semantics of the data and that becomes very, I. So some of these queries. So, uh, I mean, sorry, uh, just to answer the question, uh, you could answer to the point questions using llm, but using a knowledge graph, you can do much more.
You can explore the space better and make it more explainable that that's what we saw. This was just one example. . I think that that's, that's, that's a great example. I think it's, it's very, um, it neatly summarizes how it really depends on the task, right? It depends on the task at hand. Uh, you know, what the, and then, you know, based on the problem, you designed the solution.
And I think that's an obvious statement to make. But sometimes, particularly as practitioners of a particular art or a particular technology, we get excited about the potential of the technology without necessarily considering exactly, you know, what kinds of problems it's best suited to solve. Uh, and it seems that, you know, what you're kind of saying is, you know, for certain tasks where exploring, um, a space with particular.
Constraints or with a particular structure is important. You know, a tried and true approach of named entity recognition and sort of mapping the knowledge and structuring the knowledge that way is better than sort of the more, uh, the ostensibly more powerful, but a little bit less clear method of like a large language math model.
And, and this is just one example. Of course, there are plenty of cases where LLMs are, are useful, uh, for, for sorting. Much larger spaces of data where you may not have as much, um, a priority understanding of like what kinds of entities are important and what things, you know, you, what relationships you wanna identify.
It sounds like, so, am I summarizing this correctly? Yeah, absolutely. You summarized it well. Uh, definitely. So is this something that you feel like will change in the future, or is that just an inherent problem with ll. Well, uh, I don't see this as a problem. I see the problem with the hype of LLMs. Like I think, uh, every people are saying LLM is like the next best, best thing after sliced bread.
And they, they're throwing LLMs and solving everything. So in the real world, I think, uh, we just need to like, like we should know correctly said, it depends on the problem. Like some problems, like a pure question answering solution. Even conversational ai, I think LLMs are doing a fantastic job and, uh, they can, they can do really well.
But if you are going to get into the knowledge part, you want to extract knowledge, structure the knowledge and answer. With giving concrete evidence. Uh, and again, knowledge graph is not like a competing technology. You use LLMs, even NR Today the best techniques are based on LLMs. I, transformers, um, have, uh, out have beaten most of the benchmarks for nr.
So you use LLMs to, but then you to extract knowledge, not just blindly use LLM to unsolved any problem. So I think that's the main difference. I, I, I see, uh, the LLM with a context based knowledge graph. As an application area rather than just a pure L llm, uh, this thing. Yeah, I see that. I see that. Cool. So then, um, let's, let's keep cruising because there is something that I know both of us wanted to talk about, and you mentioned it for a second when you were given a bit your background.
It's around the remote monitoring and diagnostics and diagnostic, sorry. And you said something before we hit record. That is cool. And I wanted to bring back into this conversation right now, which was around how the ideas. Didn't really change. Like you find yourself doing the same thing with different technology.
In the beginning, you were using a very rule based system and then you transition to now a machine learning based system, but there's still some fundamentals that didn't change there. And can you give us what exactly it was that didn't change? Yeah. Uh, and that, uh, example was more around, uh, like in, in, when I was doing remote monitoring and diagnostics.
Uh, the idea was, uh, you would, uh, uh, uh, at that time they would be called domain expert or, uh, like engineering experts who would compile certain rules. So rules would be something like if the, uh, if the, if the input temperature exceeds so much value. Uh, at this particular notch you may have an alert.
Alert, so change the operation. So as simple as a simple instruction checking for thresholds. Uh, so, so they would write this rule. So this was a domain knowledge in the mind of a. Of an expert and you would capture that, that, that knowledge as a rule, put it, package it as a rule and send it to a system where it would, this inference would happen and the rule would run, and the rule would trigger on the real machine whenever the temperature cross the threshold and you'll get an alarm back.
So that was basically in a nutshell, I mean, real reality is much more complex, but a simple example that would be how a remote monitoring and diagnostic system would run. You would send rules, package them for a particular device. Uh, like in this case, device could be a turbine, and you would get an alert back whenever certain rules would be violated so that it was information for the end user to do something.
Now with, uh, now with ML and with. Uh, in a, in a way, the way I look at it, you are pretty much doing the same thing. You are, uh, have a development environment where you are, uh, instead of writing rules, you, you have some training data that you're pulling, you're building a model, uh, using some GPUs or, uh, uh, some, maybe even some low code ML tools like auto ml.
You build a model. Once you're happy with the model, you, you test it on your training and test and validation. If when you're happy with your position, recall, now that model becomes like your like equivalent to a rule. You send it to a to a machine. Now this could be in the cloud or it could be an edge device, and now that model will run continuously on live data.
and, uh, it'll, again trigger alerts whenever certain thresholds internally would. Now, this model is much smarter. Now, in the rule case, uh, the, the domain expert need to figure everything out, like what is approximately, what should be the temperature that should change. But now this ML model has been trained on a lot more data.
It has seen probably the way I would say it, uh, it has seen the world more because you are given it so many examples of real world data. So this is a very, uh, it's equivalent to that domain person writing those rules manually. So all you have changed it. Instead of some domain person writing rules, you are given it data and made the, uh, made it learn.
But the, the ops part remains the same. You're sending the rule out, uh, the inference is happening and you are getting alerts whenever the. Rule chain now the only difference. So, so that is the same part. So that is awesome. Yeah, I understand what you're saying. If I can just reiterate that back to make sure that I am hearing you clearly, it's that instead of having to figure out a hundred or 200 or 200,000 rules, And hard code them into the system.
You deploy the model and that is going to figure out the rules, and it's going to be much smarter than all of these hard coded rules because it's going to be able to understand the data and interpret the data in a way that these hard coded rules wouldn't have been able to. Exactly. Yep. That, that's, that's an excellent summarization.
So that, that's a, and, and then just to add, So what remains same is the way that you're sending this inte, I will just call it intelligence. It could be rule, it could be ML model. You're sending this packet of intelligence to an edge device, making it to inference and getting some insights back as an alert.
Now, what remains same is this communication mechanism to, uh, send it and invoke it. Also, what remains the same is a lot of other things. If you, if you think about it, things like. Like, just like an ML model, maybe biased on certain factors. Now, if I, if I, if that guy building those, that rules says, Hey, if that gas turbine is in this, uh, country is in one of these countries, I don't want it to fire beyond this temperature.
So that is a bias that that person is intentionally adding to the system. Now, my model may be adding this bias. Now I just want to check if those bias are not right. So if he's adding some unwanted bias and, and I can't think of a good example, but if there is a bias, which is not supposed to be right, I wouldn't want a human to be adding that as a rule.
Nor would I want my ML model to learn that from the. So, so the fat checks, the explainability all becomes relevant. It is just that it's, it's a different time. It's a, the model is different, but all the infrastructure deployment, all the lops pieces, same thing with drift. I mean, the data is going to change in production to tomorrow, that turine is going to wear over time.
So after, uh, another one year, there'll be a lot of degradation in the turbine. You will want to change. So you would want to check for that drift, in this case, a physical drift. Similarly, you want to get, collect new data and retain the ML model. So, uh, in my experience, I mean though we didn't call it ML at that time.
It the infrastructure and the rule deployment, the intelligence deployment remains same, is just ML ops is because it is ml. So, uh, it's very interesting that there are a lot of similarities to and differences to this. Totally. Yeah. I think, I think the way that you. The way that you summarized how to think about intelligence systems, right?
And how to, you know, uh, ship those kinds of systems and what kinds of, of, of, of features are the same and different as the kinds of intelligence that we're working to embed into systems has changed is, is, is awesome and it's very helpful. It goes very to the, very much to the heart of what. It is that we are trying to do with machine learning, right?
Which is bake some kind of intelligence in a, in a way that's right now very difficult. But we hope someday through our work and the industry's work will be very easy, you know, into the operation of products. Uh, and I want to use that sort of fundamental set of observations that you offered as a way for us to start talking about your.
Uh, you released a book recently with O'Reilly called Karas to Kubernetes. Uh, and uh, it's, I love the title, uh, sticking with the Caves. Uh, and you know, I wanna start first by asking you, what is it that compelled you to write this book, uh, and what's in it? Uh, and can you give our listeners a little bit of the story of how you got to.
And first of all, thanks for asking about the book. I, I somehow miss that in mind. I, I often tend to do that. I, I tend to miss out on it. You're a very prolific person. There's a lot to cover, probably a few years of your life. So thank you. Thanks so much. Proud of this 24. You have a lot to talk about, so yeah, I'd love to hear about it.
Yeah, so, so, uh, to be honest, writing a book was something that was always on my, uh, bucket list. I mean, that's something I, uh, and just like learning on LinkedIn or, uh, I, I really like sharing my things. So even a lot of my colleagues who also vo for it, I allow to share my experience. If there is a new technology I learned, I would love to share that.
Even LinkedIn, I try tend to put a lot of. Uh, these kind of posts to share information. So, so I really wanted to compile and, and not being from a computer science background, uh, from my mechanical engineering background with the engineering skills and then picking up right from, uh, the basic software languages and then getting into machine learning, I felt I, I brought a different perspective to machine learning.
Uh, so I kind of. It's not like a, a typical somebody who has done the degree in computer science and, and gone through it. I, I kind of brought more practicality. So I really wanted to share, uh, the, my unique perspective. And that is one of the main reasons. And then when I thought about it, I mean, it had been in back of my mind, but uh, especially with my work at G Transportation, with the work on the computer vision project, we really spend a lot of time trying to master a system to deploy intelligence to the.
And then, uh, that was something I, I really saw that, and especially at that time, uh, things like Kubernetes was new Q Flow was, I think David had just, uh, released his first YouTube video on Kubernetes. It was that time. And, uh, so, so we didn't really have any tools. Uh, so in, to be honest, I, after I got your invite, I went back to the book and checked.
I, I have not mentioned lops in the book though. The book is called Kara is, uh, journey of ML Model to Production. I don't call it ML ops because lops was not a word at that time. So, uh, So I think that is what I wanted to share, that taking a model, taking this intelligence, putting it in production, what are some of the loops to go through and like know you said?
I think I found at that time the two top technologies for Carus and Kubernetes. Everybody was building ML models in Carus and everybody was trying to deploy models in Kubernetes. So I tried to do a best of both words. The half of the book talks about carers, a lot of computer vision kind of models, and then.
Uh, uh, and building some ML models, uh, even some text analytics models. And then second half is more around deployment. And the last couple of chapters, which are my favorite, are more my thoughts on how this, uh, this, uh, scenario would change how the ML deployment will change. And at that time, none of these LOP standards were published.
And I'm, I'm very happy to say, even if I read that, read the book now, I mean, just yesterday I glanced over the last two chapters. I think, I think most of the things are very relevant, even with the, the state of the art lops things that are happening today. I think a lot of the basics still remain the same.
Uh. Right. And I think, yeah, I think, you know, So the book released in May, 2019. And I have to say that a lot of the, the fundamental concepts that are, you know, in the book, you know, talking about like, well, you know, what is it about machine learning that we should understand important concepts, like why variant straight offs and how to develop models.
You know, how do you think about maturing your infrastructure, going from just a model to a container to then using containerization, you know, software. Uh, it, it, it, it has that incremental component, um, very well laid out. Embedded in the title right char to Kubernetes. What I am curious about is to say, okay, it's uh, it's, it's November, 2022.
O'Reilly comes to you and says, Hey, Dr. Raj, we love the first version of your book. We want version two. We want it to go out in May, 2023. , how, what's, what's the title of that book that has the same message of how to go from problem to ML system and what kinds of new content are you? I. Yeah, and you can just just kind of curious.
Oh, that's a tough, tough. Which, no, that's a great question. But actually I, I have thought about that. In fact, we, I did have a conversation, uh, but, and I didn't want to do the second edition because, uh, unfortunately though the title was catchy, Kubernetes, uh, it was very tool based. I mean, I didn't want to make it tool, so one of the changes I would do is, uh, not make it so tooling based, because at that time when I wrote it, everybody was talking about, I mean, Kubernetes was, I mean, there were like, People were talking about Kubernetes as the technology.
So I would rather, uh, focus on the patterns and that's what actually, even at persistent, when we talk to customers, we try to look at the common patterns that are there. Patterns like drift monitoring, explainability, interpretability. Uh, patterns around, uh, uh, packaging models, uh, validation, um, error analysis.
So I would, uh, I mean, definitely the, the book that I would try to focus around ML patterns, in fact, uh, uh, chip, uh, beat me to it, her, her new book, uh, ML System, patterns of mls, uh, is which, which I love. I think she just released that book and that, that's an amazing book. But, but that is the thing that I had in mind.
Like, uh, if I was going to write a new book, I would focus more on the patterns and just like I explained with that ml, uh, the remote monitoring example, I mean, the patterns have not changed back, uh, from the two thousands. The, you're still packaging intelligence deploying, intelligence monitoring for drift, checking for bias.
So all that remains the same. So I would do something, uh, write something around the generic patterns, and then you can use car's by torch, whatever, uh, technology you want to use. Uh, or, or some local tool like a store pi. Or SageMaker to build some of these things. So even today when, uh, at persistent, when customers come to us for lops, we, we try to focus more around this pattern.
Say that, okay, what is the problem you're trying to solve? What is the best pattern for you? And then, then that can be realized. I mean, if you wanted to be done in SageMaker vortex ai, we can, uh, build it or we can customize that particular tool to do it. But I would say, to answer your question, uh, my next focus would be more around writing about the pattern.
And what are the best, uh, tools, uh, to realize these patterns or, or even if those tools didn't exist, what I would visualize as the tools, uh, to realize some of these. So of course I have to ask, and I love this, that you are abstracted away from the tooling so that it is something that age as well. Age is like a fine wine.
And you can see, like in your book as you were saying, it's Carus and Kubernetes. You didn't really mention ML ops, you didn't really go into it so much and it, and a lot of what is there, the essence of what is there that you're talking about. It lives on beyond Caris, Kubernetes. Even if both of those end up not being useful in 20 years, the ideas that you propose in the book are still useful.
So now when it comes to those patterns that you're talking about, what are some of those, like, can you go into more, you mentioned this, uh, what is it? You mentioned the. The, the type of bias you also mentioned, but, but like I, when I see Pat, when I think about patterns, I, I also think about, there is a great book out there, machine learning design patterns that Lack put out.
And a few other, Sarah and Mike, I think are the other two, um, authors will throw it in the show notes in case anybody wants to know. But they, so they talk about a lot about like the machine learning aspect. There is some ML ops and maybe some deployment stuff in there, but I imagine you have the lens that you can look through and see what are a lot of these ML ops design patterns?
What are things that you see over and over and you say, all right, this is when you're talking to customers, this is the pattern that you're going through. So in this case, these are things that you need to keep in. Yeah. So, uh, some examples would be something around, uh, like to start with a data catalog.
So you would want to, uh, not have your, uh, I mean, I think every, everybody has moved out from the csv, but even if you have a data warehouse like Snowflake, you would want to have some cataloging mechanism. Maybe dvc or, uh, you have some, uh, version queries or, uh, snowflake has this time travel feature that you can go back into, uh, like for a period of time and pull data.
So some way to catalog your data and, and, and publish it. I mean, a lot of people catalog it, but it is, the catalog just remains with the quarter. Uh, so you would want to have some system, even if though it looks mundane, but you would want to have a way to, uh, have your model, uh, data versions published and, uh, your data, data scientists have easy access to them.
So that's one thing I, I think is very valuable. And on the similar lines, when the model is already a model registry, now today model registry is becoming very popular and, uh, of, of course, uh, like today, uh, features towards are also getting. And now there are like vector databases, uh, which do similarities and those are also getting popular, especially with, uh, uh, like Facebook has as fast and Spotify as, and no, especially when you do recommendations, they really help you.
Uh, but I think model logistic is something I would, uh, I don't, I see less. I would, I would really love to see because, uh, model registry becomes something like a model register catalog becomes like your, uh, basic foundation to your ML system. Uh, like many customers I see and we at persist, we, we have customers in different life cycle, right?
From somebody who are very new to ml, two people building hundred hundred models. In production. So we see, uh, a lot of them not paying enough attention to having a very managed, uh, model registry and model governance. So I, I think, because, uh, uh, what what that does is now you have a very organized catalog for your models, and the next step would be to look at patterns like, uh, deploying models of microservices or what is getting popular today is, uh, ML as a.
So another, another pattern we also want to look at. So when we look at ML as a service, many customers are looking at, uh, monetizing those models because, uh, so because typically you look at ML model as, okay, you have a a, a pickle file and some developer will come and pull it, put it in an application and consume it.
But I, but that doesn't, especially, these are very valuable models and the industry that I come from, banking and healthcare, these models are the ones making money for these customers. So they really want to monetize them. I mean, these, these are the main major assets. Uh, uh, they're trained from their data and they can make much more money by cataloging this.
And even making them available as a marketplace. So today, some of the things that some customers actually monetizing them, they have a token based system to actually, uh, uh, invoke these models like Amazon has, like your Amazon marketplace, a marketplace offering where they can charge customers based on usage of this models or even internally, they would want to have a better control of how these models are used so they can manage the life cycle of this.
So again, to a long answer to your question, but. I would say some things like model, model registry data, cataloging these, the multi managing multitenancy. Many times customers have, if you're multiple customers for the same model, how do you do multiple tenancy? These are not some things that a data scientist would think of, but it is something that, uh, an ML engineer or an ML ops person should think of.
And should, we should have, I mean, these are solve problems. Uh, we should have like a standard way to reuse these solutions. And then like the earlier. Once I talked about bias and fairness, I mean, that is something you, you have libraries which can easily calculate debt, but the key thing is you need to consider them.
Many, many customers I've seen are like, they're hesitant to include them because they see bias as a, uh, like a negative thing, or they will get audited. But that is something you need to start incorporating. At least you need to have some audit reports, some model card of your model. It's like we can't be biased if we don't audit it.
that's the, oh man. That is so interesting what you are talking about with the model registries or just like the data scientists that are charging for their models or having that model marketplace, because I know that was something that AIA was doing back in the day and they. Yeah, they ended up getting bought.
Uh, and so I don't know if there are other companies or tools that have come gone to market with that same approach, but it is a fascinating one to think about and to see how, um, How others are, are tackling that problem. I also really like this idea around the multi-tendency and managing multitenancy because it is so difficult when it comes to security.
Right. And like what you need to get past the DevSecOps team, what you need to make sure, yeah. Everything checks out. And if you're a data scientist trying to do that, it's a lot harder. Yeah, exactly. In fact, it's interesting you mentioned about our gothia. I, I spoke to Diego when we were building. Platform.
I mean, back, back before he went to, uh, I think I should, uh, data robot. Sorry. . Yeah. So he actually, we had an interesting discussion over email, but uh, he was sharing some of his experiences and the good thing is he actually made a lot of those, uh, papers public, I mean, the way, uh, behind the scenes how they were doing a gothia.
So I think, yeah, that is one of the very popular, I mean, that's a prime example of a very popular pot. . Yeah. Ah, so cool. They were ahead of their time. Yep. So, That garage. Man, this has been excellent. I cannot thank you enough. Super happy to have you on here and learn from you. You have so much wisdom, you have so much experience, and if anyone wants to get your book, they can go to O'Reilly, I think it is, or Amazon.
Yeah, it's, they'll have it. And they can pick it up. The, uh, it has aged well. There's some books that do not age well because they talk about how to do things in the step by step guide, and then as soon as the new package comes out or the new hot thing on the market comes out, then you recognize that all that you've learned from that book is no longer available or useful to you.
This is not one of those books. Is a book that you will learn a ton from even though it was written three years ago. Over three years ago. Yeah, ago. . Oh man. It's incredible. You did a great job and I am so thankful to be able to speak with you and talk with you about all this cool stuff. Thank you. Thank you.
Thank you so much, so much for that. Thank you, . Thanks. Thanks a lot. It was fun talking to you guys and uh, I love, love your podcast. Thanks. Keep up the.