FedML Nexus AI: Your Generative AI Platform at Scale
speakers

Salman is a professor, the inaugural director of the USC-Amazon Center for Secure and Trusted Machine Learning (Trusted AI), and the director of the Information Theory and Machine Learning (vITAL) research lab at the Electrical and Computer Engineering Department and Computer Science Department of the University of Southern California. Salman is also the co-founder and CEO of FedML. He received his Ph.D. in Electrical Engineering and Computer Sciences from UC Berkeley in 2008. Salman does research in the areas of information theory, decentralized and federated machine learning, secure and privacy-preserving learning, and computing.
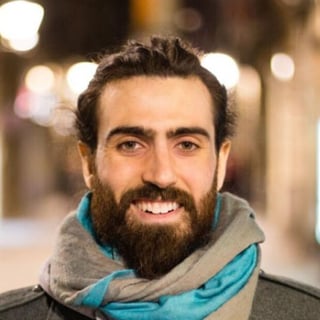
At the moment Demetrios is immersing himself in Machine Learning by interviewing experts from around the world in the weekly MLOps.community meetups. Demetrios is constantly learning and engaging in new activities to get uncomfortable and learn from his mistakes. He tries to bring creativity into every aspect of his life, whether that be analyzing the best paths forward, overcoming obstacles, or building lego houses with his daughter.
SUMMARY
FedML is your generative AI platform at scale to enable developers and enterprises to build and commercialize their own generative AI applications easily, scalably, and economically. Its flagship product, FedML Nexus AI, provides unique features in enterprise AI platforms, model deployment, model serving, AI agent APIs, launching training/Inference jobs on serverless/decentralized GPU cloud, experimental tracking for distributed training, federated learning, security, and privacy.
TRANSCRIPT
Join us at our first in-person conference on June 25 all about AI Quality: https://www.aiqualityconference.com/
Demetrios [00:00:00]: Hold up. Wait a minute. We gotta talk real fast because I am so excited about the MlOps community conference that is happening on June 25 in San Francisco. It is our first in person conference ever. Honestly, I'm shaking in my boots because it's something that I've wanted to do for ages. We've been doing the online version of this, and hopefully I've gained enough of your trust for you to be able to say that I know when this guy has a conference, it's going to be quality. Funny enough, we are doing it. The whole theme is about AI quality.
Demetrios [00:00:34]: I teamed up with my buddy Mo at Kolena, who knows a thing or two about AI quality, and we are going to have some of the most impressive speakers that you could think of. I'm not going to list them all here because it would probably take the next two to five minutes, but just know we've got the CTO of Cruz coming to give a little keynote. We've got the CEO of you.com coming. We've got Chip, we've got Linus. We've got the whole crew that you would expect. And I am going to be doing all kinds of extracurricular activities that will be fun and maybe a little bit cringe. You may hear or see me playing the guitar. Just come.
Demetrios [00:01:19]: It's going to be an awesome time. Would love to have you there. And that is again June 25 in San Francisco. See you all there.
Salman Avestimehr [00:01:28]: I'm Salman Avestimehr. I'm Co-founder and CEO of FEDML. I'm also professor of EC and CS at University of Southern California and director of USC Amazon center on Trustworthy AI. I love coffee. I take it pour over American style.
Demetrios [00:01:48]: Welcome back, MLOps community. We are here with another podcast. I am your host, Demetrios. And today we are talking to Salman all about the FedML platform. I really appreciated how he broke down the maturity levels that he's been seeing out in the wild as he goes. And he's been creating Fedml. They have a specific gen AI platform these days, and he, he's been talking to customers and asking them what their specific pain points are. They go out there in the wild, they see what the pain points are.
Demetrios [00:02:21]: And he also sees, and was able to convey to me in this conversation how customers can get stuck in different places. And what almost like this maturity level is when you encounter hurdles, whether that's on the deployment hurdles, it's the evaluation hurdles, the fine tuning hurdles. And he also showed a bit of a circular motion of, yeah, you deploy. Then you collect data and you evaluate that data and you go back and you retrain and then you deploy and it's like, huh, all this whole AI thing sounds vaguely familiar. I feel like I've seen that before. I just can't put my thing finger on where. Oh yeah, now I remember. This is what we've been doing in ML for ages.
Demetrios [00:03:12]: So Salman was great. He broke down their journey and why and what they've been doing with the FedML platform and who it's for, who he's seen using it, and what the inspiration or what the motives behind people wanting to use a platform like FedMl have been. Hope you enjoy this. As always, leave us a review. Give us some stars, give us some love on social and share it with just one friend if you think it will be valuable for them. Look forward to seeing you on the other side. Sullivan, right now you're based in San.
Salman Avestimehr [00:03:56]: Francisco, I'm guessing in Palo Alto. Yeah.
Demetrios [00:04:00]: All right. And you're living the dream running FedMl. I know that you have this whole generative AI platform that we want to get into, but I think we should just start with like who have you been talking to? What are some challenges that you've been seeing out there that gave you the inspiration for what you are doing?
Salman Avestimehr [00:04:23]: That's right. Especially if you look at it like the generative oil. Right. There has been rapidly growing and every day there are new challenges. Right. The way we view it is there is a segment of the market that they want to build their own models, right. So there is like a challenge of ownership. Ownership I think is very broad in the sense that maybe many developers want to build applications off of maybe other models, like let's say OpenAI, GPT four, et cetera.
Salman Avestimehr [00:04:53]: But there is a large group of developers who want to have ownership. Enterprises also want to have ownership, for example, your data, your IP. So the first challenge we are seeing is how can we help people who want to build deploy their own models, their own applications? In this complex landscape, there is a very complex Jnioi software stack that you need to know on how to build, how to deploy your models, how to create applications. And one part of the challenges that we are seeing is, again, ownership and how to be your own open AI.
Demetrios [00:05:35]: Yeah.
Salman Avestimehr [00:05:36]: The second we are hearing is scalability. So scalability would mean as maybe your application becomes popular or you want to maybe serve many users, then you need to have capability to scale your application. Maybe at the scale that chat GPT is scaling. There is a lot of infrastructure behind chat GPT. I mean, a developer only sees the API, but there is a lot of capability. Azure cloud behind it, Azure AI behind it, et cetera. So I would say ownership and scalability are the two key worries that we are seeing again across individual developers, smaller startups, enterprises.
Demetrios [00:06:19]: Yeah, with that ownership, I think one thing that I've been hearing over and over is control and how people want control, not only of the models, but just of what is going on in their system. And you get to a point where I think that it's kind of in the journey of, all right, we'll start with something really fast and see if it has value with OpenAI and then ideally we're going to transition off of it. If you can use OpenAI at the beginning, because there's a lot of companies that can't just go and use that OpenAI API. I'm sure you talked to quite a few of them and they have to figure out, all right, how can we iterate quickly? How can we make sure that we're not losing out? Because we can't hit that API and we can't just figure out like product iterations over and over and empower anyone on the product team, or on any team for that matter, that knows how to work with APIs to create something with AI.
Salman Avestimehr [00:07:26]: Exactly. And that control even simply, let's say you have your model or maybe the API you are calling having observability monitoring. How are people using that? What are the interactions? When does the model maybe give the wrong answers? What is the feedback, how to bring that for your next iteration, etcetera? Definitely ownership is very broad. Ownership, control. Privacy is also connected to that. Like as you want to have ownership, right, you want to have privacy. Again, another concern that, you know, many companies deal with. I mean, as you are using these services, how much are you leaking and how much are you exposing? All of them, I would say ownership is very broad.
Demetrios [00:08:11]: How are you dealing with that problem of data leakage and privacy?
Salman Avestimehr [00:08:16]: There are different ways. So one part of it is like on premise deployment. So for example, let's say you are an enterprise, you have a lot of proprietary data that you want to use for building your models. And later maybe you want to offer the service to your own, you know, users, your own employees, etcetera. Federman we have a great solution that offers fully on premise. It's like you are building, you are deploying everything between your own VPN. So that's great because nothing is leaving your trusted environment. So that's where you can think about it what enterprises are looking for full control, full ownership, but they need to have the capability in house being a platform.
Salman Avestimehr [00:09:09]: We can offer that. So that's one way. The other one is, you know, let's say you are a developer, right? You want to build a solution, right? That's where you can have your own dedicated endpoints and deploy the models on your own dedicated servers. For example, if you are using a API that you are getting right, these calls will go to other servers, maybe other models. Something that many startups will come to us is because they get dedicated deployment of their models or their agents. That way it's like belongs to them, the ownership, so you can trust them. Then the other layer of it actually goes higher, is kind of as you build that essentially, I mean, your models will expose the training data, so there is no way around that. It's like the train model has been used.
Salman Avestimehr [00:09:59]: If the model you interact with, it can expose the training data. I would call that a safety layer that we are building. So it's kind of understanding before sanitizing the response or maybe filtering the questions. So that becomes like a layer that after building and deploying the model, you want to make sure you analyze and figure out what questions are appropriate to respond. What questions, for example, maybe are asking sensitive data about your company and you should filter them. But I think the safety layer becoming very important layer. So essentially, you know, there are different layers at the time of building the model, when you are deploying the model, and after production, how to bring in privacy.
Demetrios [00:10:44]: Yeah, it does feel like if you wanted to do this yourself, what would these different pieces entail? Right, the building of the model, what have you generally seen people using and doing in that regard? And then when you need to deploy it, what are some other things, common design patterns for that? And then once it's out there in the wild, everything that is also a potential for people to do if they want to do it on their own, because it, it's almost like most people have it too easy when they can just hit the OpenAI API and you get a little bit spoiled if you can do that, or if you can just like talk with chat GPT but then if you want to productionize something in house, like you're saying, you're probably going to have to hire an engineer. And whatever that engineer salary is these days, I hear AI engineers or ML engineers aren't cheap. And so whatever that salary is, plus whatever the cost of doing the business on top of it is going to be. So have you seen people that are trying to do this in house, and then they come and they say, all right, we are pivoting to an easier solution.
Salman Avestimehr [00:12:02]: Exactly. Actually, we have seen people who want to build it in house. The problem of that is exactly the layers that you mentioned. Maybe you mentioned the engineers, but I would even add more to it. Typically, to build it, you start with maybe ML scientists. ML scientist knows the model, knows the algorithms, etcetera. Then you need to have ML engineers, then you have to have the infra team, because to build it in house, you need to have a very strong infra team. Typically many companies.
Salman Avestimehr [00:12:30]: Maybe you are good at the ML science and engineering. Even if you can get the engineering, infra is another layer. You never want to, you know, have that. And I think that's the role of, let's say, FedML as a platform company. The goal is you don't need that. Right. That's exactly the exciting part. We help them along the journey to deploy their own applications, maybe create their own models.
Salman Avestimehr [00:12:58]: Right. That way you don't need to hire the infra team. Why would you need it? I mean, the FedML platform is going to take care of all those challenges at the infra layer. What does the ML engineer would do? We try to maybe simplify what the ML scientists want to deploy. So makes it simpler. So the angle that we have is don't give up on your dream of ownership and control to go with a simple approach, building off of the models that you don't control, the models that can change, still do that, but we help you along the way. So that's how we respond to that.
Demetrios [00:13:36]: Now, talk to me about the platform itself, because as you probably are very familiar with, there's a ton of llm tooling options out there. Once you've deployed that model, it's not just that you're using the model and then you're done. If you have some kind of a rag solution, you've got a lot of glue that goes in between that. Are you also adding glue or are you saying we've got your model endpoint? We also can give you a bit of guardrails, as you were talking about, and then you can bring your vector database or you can bring your whatever orchestration framework that you like.
Salman Avestimehr [00:14:16]: Yeah, I would say actually for us is of course we offer the glues, but it's much more beyond that. It's like the depth of what you can do. So essentially, as you mentioned, there are maybe many toolings that you can quickly get an API from a model, combine it with a rack system, create an agent. But I would say they don't give you a depth that you need for a commercial enterprise solution like a larger scale. Let's say you want to have a very large, largely deployed, usable application that is used at million active users. So what are those? For example, let's say in the journey you start with a model. The first step of it is that you need to deploy and serve the model. That one is much more beyond than just getting the API that can serve the model.
Salman Avestimehr [00:15:05]: API is just the upfront that you would see in the back of it. If you want to have your own way of serving the model, you need to maybe create dedicated endpoint, let it auto scale freely as the demand goes up, maybe create more endpoints and more replicate across more gpu's and as the demand goes down less. That's the way that you can manage the cost, that's the way that you can control the quality. As you are serving the model, you need to have a lot of observability, for example, how people are using that, what is the SLA they are getting? What is the query per second even more? What are the prediction locks, for example, what has been the questions? What has been the answers so you can use them later in fine tuning? So what I would say is that for example, even in the deployment part, there is a lot that we can offer people to build a scalable endpoint that is production ready. Like we let people productionize, not just having a simple, like a demo. Yeah, so that's like on the deployment, then we try to, you know, let people go along the journey. Like typically you deploy the model, you have a scalable endpoint. The next phase is that as you collect more data and people are interacting, you always want to make your model better.
Demetrios [00:16:26]: Yeah.
Salman Avestimehr [00:16:27]: So that's where we help people fine tune the models, right? We help people. For example, we offer zero code fine tuning, right? That way is like we have already. Let's say you are a maybe software engineer, you are a data scientist, maybe you cannot write the code for fine tuning. That way you can just change some hyper parameters and start fine tuning the model from the data that you collect in the deployment. Then you want to do more advanced, for example, maybe a distributed training of it. We offer mlops for the training, but the other angle of it, as you mentioned, is that maybe you want to start doing maybe, maybe creating an agent with it, so on and so forth. We also support that. But essentially I would say it's kind of for each of those as you want to go deeper.
Salman Avestimehr [00:17:12]: And again, the vision is that you want to build an application at a scale of chat, GPT and what OpenAI is doing for your own application. We help you do it without having a huge infra team or no infra team at all.
Demetrios [00:17:26]: So let's say that I do have one or two overworked SRes and they're saying, all right, cool, I want to use Fedml, but we've got our GKE instances or we've got AWs, Eks or EKC instances and we want to know how that plugs in to FedMl. Is it just something that you can put on top of it? How does it interact with the infrastructure that I already have?
Salman Avestimehr [00:17:57]: Actually that's a great point. So another key component of it, as I mentioned, the your part of FedML, we spend a lot of time. The last part, we say your general platform at a scale. The scale would mean FedML is a platform that you can deploy it. Multi cloud. For example, let's say Azure AI is a platform running on Azure cloud or Vertex AI platform for Google Cloud. FedML is a general platform that you can run it on any cloud. And that's how we let people escape.
Salman Avestimehr [00:18:29]: Now, on your question, let's say you are maybe a startup company. You are using many instances, maybe you are getting credit and using AWS, right? What you can do is add your own instances or your own cloth inside your own compute that you can use in Federmat. But the bigger advantage that we offer is FedML already offers multi cloud through partnering with many GPU providers, right? So that way you don't need to shop around and find the GPU's that you would need, right? So for example, let's say you are a, you know, maybe a developer. There are many choices that you have for offering GPU's, right? There are many GPU providers and the prices are changing, the capabilities are changing. Federmail gives you the offer that you don't need to shop around is a multi cloud, multi provider. We always find the best resources for you. So it becomes both offering you platform and the infra. And the difference is that this infra is multi cloud, multi provider.
Salman Avestimehr [00:19:35]: But you can also add your own infra to it. It's very easy. It's like you add your instances quickly and use FedML to deploy, launch, fine tune on your own instances.
Demetrios [00:19:46]: And so talk to me about the evaluation piece because I know that is a hot topic when it comes to how people are doing it, what they're doing, what kind of results they're seeing. Have you noticed any trends on best practices when it comes to evaluating the models and the products that people are putting out there?
Salman Avestimehr [00:20:06]: A part of it is maybe one part of evaluation is just how good your model is for that one. There are various benchmarks, I would say for general purpose llms, there are very well known benchmarks that you can use to assess your model. It becomes more challenging as you are building vertical llms. So for example, let's say you want to have a very good LLM that is focusing on maybe healthcare questions. So there is less of benchmark. There are some benchmarks, but I think is less known for different verticals, how good your model is. Okay, so that's where I would say one part that maybe the first year there has been a lot of effort on benchmarking for general purpose lnms, vertically focused llms. I think there is going to be many more benchmarks coming up to help people evaluate the models, right? And figuring out how well are they doing.
Salman Avestimehr [00:21:02]: Or maybe you want to choose between different models. Which one are you going to choose? For example, for coding, like helping you writing codes, there are already good benchmarks, right? So that's where I would say an example of a vertical LLM, that there are good benchmarks. You can evaluate it and do that. But many others we have lack of benchmark. The other part of it is like other measures. For example, you have a model, how much is hallucinating, how much is the credibility score of it, and that's the other side that we are seeing. For example, there are multiple layers for that. It's like you want to assess hallucination, you want to assess the correctness, you want to assess the privacy leakage in the model.
Salman Avestimehr [00:21:45]: I think these are converging into separate layers that maybe, I think soon you can just click and choose which one of them you want to enable, which one of them you don't want to have. But there is also much deeper question behind that. Deeper question is, for example, you can think about, let's say an LLM comes up with a response. How would I assess the correctness of response, especially when the questions are open ended, right? So that's a very interesting problem, very challenging problem. The reason is, you know, again, assessing correct correctness, typically in machine learning there has been a lot of work when is like a binary hypothesis testing or multi class hypothesis testing, you know what the classes are and you want to figure out is the class being detected correctly. That's why there is a lot of good assessment. And evaluation, say, for computer vision models, where the classes are clear, either the object is a cat or a dog, or, you know, something. Lnms are very different.
Salman Avestimehr [00:22:49]: These are dynamic responses. They are open ended. No clear classes becomes a very interesting research problem by itself, which is like, you know, how would I assess the correctness or evaluate it for that? Actually, we do a lot of research in our group. For example, we have a recent paper on meaning aware response scoring. It's kind of what is the meaning of this sentence and how to put weight on different tokens. So for example, let's say you ask me, do you ask an LLM, right? What is the capital of France? Right? And then it would say the capital of France is a beautiful city called Paris. In this sentence, the key component is Paris. Not all tokens have the same weight.
Salman Avestimehr [00:23:40]: That's the only token that affects whether the response is correct or not. So it's kind of, how would I assess? By paying attention to the meaning, the correctness of the sentence, and figuring out the important tokens, less important tokens, etcetera. So you can imagine, I mean, those questions, you know, on the, when you go to the practical side, people are looking for simpler, quicker things to do. As you go towards the research, it becomes very deep questions that still we don't know, you know, how to address them. But the research community is also moving very fast in the domain, and I feel there is going to be a lot of innovation coming for evaluation by itself to the community, which can help even building better models as we evaluate them better, helps to figure out what is the new training data to be used to make the performance better, because that's the bottleneck that we are facing now.
Demetrios [00:24:37]: So now this research paper and being able to weight tokens differently, are you then able to pipe that back into FedML and the platform and fine tune with that meaning awareness?
Salman Avestimehr [00:24:51]: Definitely. Exactly. As I mentioned, it's time to think about it. When you have, for example, prediction logs of your model, what it would mean is that you are monitoring how people are asking questions and how the LLM is responding with such a metric. First of all, you can figure out what are the pairs of questions and answers that the LLM is performing poorly. Your model is not performing well. Now you have two choices. Either you can use maybe human in the loop to come up with a better response, right? And use that as a next phase for preparing the data that can remove the weaknesses of your model or create different responses.
Salman Avestimehr [00:25:34]: And that's exactly the loop that we are talking about. It's like, as you deploy the model, you can observe and monitor how people are interacting. How would I use those interactions to constantly make it better?
Demetrios [00:25:47]: Yeah, it reminds me of the DevOps figure eight. It's just got a little bit of a extra figure eight. And I know back in the day in the mlops community, someone put together like an MlOps figure eight with an extra figure on it. And now it almost feels like that. You deploy, you evaluate, you get the information from the evaluation, which then helps you retrain the model and so on and so forth. Then you deploy the new model, and then you're able to do it. I recently posted something on LinkedIn about how the deployment strategies that people have or the ways that people can deploy their models are sometimes a little bit funny and wonky, and especially maybe not as much these days, but you can get people doing, you catch people doing something little quick and dirty, and it's probably not best practice, but whatever it works are there within like FedMl. Do you have ways to be able to test if a model, if you're rolling out a new model, can you like canary deploy that? Or can you do waze so that it is like a b testing it? And then you're able to see, did we with our latest fine tuning just totally disrupt this model, or is it still good? And do we have confidence, like to deploy in confidence?
Salman Avestimehr [00:27:21]: Definitely. Meaning that one component of it is exactly as you mentioned. As you are creating different endpoints, maybe let's say you have model a, model b. For each of those you create endpoints. The first hacky part is as a developer is like you want to quickly check it. Okay? So what we offer is, for example, when you create your endpoint, there is a playground already built for your endpoint, so there you can quickly interact with it. That's what I would call a quick check as a developer before going and calling the endpoint in the application. Right, right there.
Salman Avestimehr [00:27:56]: Trite, ask the questions, make sure it is working fine, maybe there are some issues, take the endpoint down, etcetera. So that's like the first thing is like, think about it, a playground embedded in your endpoint creation. Okay? So that's like the first thing developer typically wants before going to the application. You know, use that. The second part of it is two endpoints, routing the question to both of them. So for example, as you are routing to both generating the response and having your A B test, we call that the API gateway, meaning that for your same endpoint in the application, you can decide how to route the question and maybe sometimes route the question to both endpoints and generate the response. This creates the data for your A B testing. So that's what I would call is like another functionality, having the gateway that you have for your endpoints.
Salman Avestimehr [00:28:55]: And third, it becomes more of a analyzing. That's where we give you the prediction logs, looking at the responses, and then you can make your decision, or even you can let your users, for example, we have a customer, what they wanted in the application, having this gateway that automatically or maybe randomly changes between the two, and then they have a thumbs up and thumbs down from their users. So that way they are randomly collecting, which is a great data for the, you know, for the A B test with the human feedback, which is tom down, they are happier or they are not happy as they are changing the model.
Demetrios [00:29:36]: Yeah, we had one bot in the MLL slack community and it had the thumbs up and thumbs down, and then it also had the little mushroom symbol if it ever hallucinated so that it could give feedback right away. Like, yeah, it's not only that this wasn't helpful, but I think it was totally made up. And then it was another signal to say to the team like, yeah, here's an instance where the data is a little bit tainted or the output is not what you would expect. And so you were mentioning different customers, like who have you been seeing using this platform? Like who's this for? What have they been using it for? Is it mainly just chat bots or what else are they building?
Salman Avestimehr [00:30:23]: Yeah, so let me explain a little bit. So first of all, as a platform, right, we try to attract many groups. For example, this can be individual developers, it can be startups who are about to build their applications deployed, and larger scale enterprises. As you can imagine, each of them have different demands and the reason they come, right? So for example, if you are a starter, who are building your application, maybe to give you an example of applications, let's say you are building the best JNAI models for 3d modeling, or maybe you are building the best JNAI model that is doing vertical LLM, for example, is going to be a great healthcare summarizer because that's the solution you want to offer. Maybe as a SaaS or LLM solution to others, typically startups, the challenges that they would be facing is scaling their solution, building the infrastructure like similar to again, chat GPT, offering the capability on their own model. What it would mean is they come because they want to have the capability of a platform that lets them deploy their models, scale their models, gives them the safety layer for their models. Maybe these are not the capabilities that originally they have, because the original capability is that they are great at building a proprietary model, but they are not strong in scaling, deploying the solution, making it ready for production, adding the safety measure to it, or monitoring the traffic, auto scaling it. Right again, cost becomes a major barrier.
Salman Avestimehr [00:32:05]: If they allocate many gpu's to their mother, they will be bankrupt. They are a startup, they cannot afford like chat GPT spending, what is it like five hundred k a day on serving the mother. So they come, we help them with the way that we let people do across multiple cloud, multiple providers to lower the cost. FeDML cost for example, is four x five X cheaper than what you would get on the compute from let's say major cloud providers. So they can lower the cost. They don't need infra team, they can scale their solution, deploy their solution, getting it ready for production. We see a lot of excitement there. We talked to many startups, they come, they deploy their solutions with us.
Salman Avestimehr [00:32:54]: For example, again, you have a chatbot, it is doing healthcare summarization. That's great. We help you be the backend and the platform that you need for deploying, scaling your solution. Then, as I mentioned, then gradually they start fine tuning that, making the model better. We let them. Okay, you can do all of that with infideml. So that's how they stay. And they do more things inside federation.
Salman Avestimehr [00:33:21]: Then the other side of it is like larger enterprises, as I mentioned, larger enterprises, ownership and everything on premise becomes a critical component. Let's say you are a company a lot of engineers want to use, maybe a coding copilot, right? But you don't want this data is so sensitive that you don't typically they put it in a VPN, zero connection to the outside. So it's not about, you know, it's like fully on premise platform for building the solution, offering it to the employees, etcetera. We help a lot with that. That's the scenario that they need on prem capability to build it and then maybe offer it later to the customers and then individual developers. Typically they come because they need to have access to the state of the art models. For example, Lomo Three was released a few days ago. Currently on FedML model Hub is available as a developer, you can go there, access the API, use Lama three, maybe quickly launch an application with that, create an agent with it, but start doing more things with that.
Salman Avestimehr [00:34:29]: So it's kind of, people come for models, people come for the capabilities and we also offer other features, for example, let's say individual developers, maybe they want to do other capabilities, for example having observability and maybe the experiment tracking for their larger scale distributed training. You are an ML scientist, you need that capability. You can use that in Fedml. Or maybe you're working on Federated learning. FedML is the only mlops platform that you can run federated learning inside the mlops without needing to give you the full platform capability.
Demetrios [00:35:09]: Well, talk to me a little bit more about that, because I know there is some history with federated learning in the platform and you have an open source product that is in the federated learning space. So what's the evolution bid?
Salman Avestimehr [00:35:25]: That's right. So actually the company Federmal, now we are two years old. So currently is much broader services focusing on generative AI platform. But originally we started with offering federated learning, and there is an open source library devoted to that which is actually currently ranked one on offering federated learning for the audience who don't know about it. Federated learning is about decentralized machine learning. It's like how would I train my model on distributed data without collecting the data? So it becomes a privacy preserving method. For example, you want to train an LLM on the data of the users across a smartphone, but you don't want to collect that data and invade the privacy. But still you want to offer an LLM that can tune to their questions.
Salman Avestimehr [00:36:17]: For example, let's say Google currently uses federated learning for the next word prediction that you are using when you are typing on your phone. That's how they learn and train a model that can predict your next word without violating your privacy. So it's a very important scenario. Typically it enhances, I would say, building models by accessing private data in a privacy preserving manner. I would say maybe the reason, and actually we offer generalized services right now with the foundation models, since they are trained on massive amount of data. They are already doing great. Like the bottleneck, I would say in the first year hasn't been more data, has been more of compute and the cloud offerings to let you build the application. That's why in FedML our focus has been there over the past year is like making sure that people can build their generalized solutions with the cloud offering.
Salman Avestimehr [00:37:16]: I would say as the time progresses, maybe a year from now, my prediction would be that data becomes much more of an important bottleneck, because that's the way that you can build much better applications. They say you are building a vertical element, your mode is going to be the data you use to build that and I think it's becoming more important and people maybe, you know, would be more interested to use federated learning as a way to access more data for training and making the general applications better. For now we see a lot of attraction means that there are many companies, maybe because of the privacy they are using federated learning they come to us. So that's one scenario. The other scenario is, as I mentioned, many developers, maybe they are experimenting, they want to have research, figuring out how much this way of training a model can be useful for their scenario. They can come and try that on FedML and deploy that.
Demetrios [00:38:18]: Yeah, it does feel like there's steps to it as far as maturity goes. And so you laid them out nicely there. When it comes to, okay, the first step or the first barrier that you have to overcome is just getting something out working. Then you start to get the evaluation. That's like step two. Then you start to say, all right, I want a more verticalized model. I need more data than just my evaluation data to fine tune this. How can I start to create a little bit more pointed solution? And is that a good representation of what it is? Did I miss any of these different challenges or steps that you've been seeing on this maturity journey?
Salman Avestimehr [00:39:06]: No, actually I loved the matrix. It's kind of first is deploying the model, putting it in production, offering your solution in a way that is ready for production. People come to us, we help. Then you evaluate, monitor, figuring out how is it doing so that you can evaluate it and then build the next version. Typically that version you find two need. Maybe you centrally improve that, etcetera. I would say in the future. Exactly is going to be maybe federated across many of your users by using their private data.
Salman Avestimehr [00:39:40]: So that can be maybe a future. But for now we see a lot of the demand is maybe on the first three steps, putting it in production, deploying the model, observing it, figuring out how it has been used, fine tuning that and putting it back in the production.
Demetrios [00:39:57]: Yeah, I do like this vision of it needs to go federated, but maybe it's not right now that it needs to be there. Right now there's bigger blockers than what federated can offer.
Salman Avestimehr [00:40:13]: Correct? Especially for the generali. I would say that's what I mentioned. Maybe next year is a become a great for the general, but for many other models, like as I mentioned, like a small model for a next world prediction or maybe a model for different applications, there is demand for it, right? So that's what we are seeing. But generally correct is less.
Demetrios [00:40:35]: I think that you are open to talking about it, but you've been brewing up some stuff secretly at FedML. What are you working on? That will be your next big announcement or development.
Salman Avestimehr [00:40:49]: That's right. Actually, something special about our company is we have an extremely fast pace. Imagine in these two short years we have been going from now a full gen AI solution, etcetera. We are moving very fast. Something we are very excited about is soon we are releasing our own foundation model. Okay, so, meaning that our vision is platform providing platform to developers, enterprises, companies, together with the foundation model, of course. You know, in Federman model hub, we host a variety of open source models. But the goal of our own foundation model that we have been training from scratch is the vision is aligned with the way that we want to bring ownership to the people.
Salman Avestimehr [00:41:38]: So what do I mean by that? So first of all, our vision is that this foundation model, we are focusing on a small llms as opposed to 70 billion or maybe 100 billion parameter models. Our focus is to having the best small models, let's say the best 2 billion, 3 billion, 70 billion parameter model. And the biggest announcement we are going to have soon is going to be alongside of revealing that model that is going to be ranked in the top in the sense of the performance in the smaller scale nlms. Now, why that? What is the vision for it? So you see, if our vision was that we discussed is the ownership, you have to work with some foundation models that you can afford to maybe dedicated deployment of it in a low cost manner on reasonable GPU's, etcetera. So that's where a smaller scale models is great for ownership, right? So let's say again, you are a startup, you are a company who are building your own solution if you can get a great performance with a small model, right, that is very well aligned with your idea of ownership, because the maintenance of it, the deployment of it, the training of it, improvement of it all makes sense to you as opposed to the biggest model, right? So it's kind of, we are seeing again, for ownership, you need the right size model, typically a smaller, of course the performance should be high, but that's where we want to empower people to build more, own more. The other side of it is, I would say again right now, a lot of foundation model building or the applications that are built on top of that is cloud driven, right? I would say in a year or so there is going to be a lot of demand that you also see a bit brewing this year or on device generalized solutions fully on device. For example, let's say you have a fully on device AI agent on your phone that is doing and helping you with many tasks. So in those scenarios, again, you cannot go with a large model and also aligns very well with our vision of federated learning, which is pushing and bringing ML development to the edge.
Salman Avestimehr [00:43:55]: So we are an integrated edge cloud platform. Our foundation model lets you easily go from cloud to the edge. And for that, you need such a foundation model that is great for the cloud, great for the edge. Okay, so that's our vision, meaning that this foundation model optimized, offered on FedML, leading people to own it, letting people to, you know, making low cost, as well as leading people to bring it on device. And with that, we are also revealing a new concept. You know, federation of models. So federation of models, for example, you have heard about mixture of experts. Mixture of experts is a way to combine models as a way to create, for example, maybe mixed run, you combine smaller 70 billion parameter models to create a very strong model by combining several of them.
Salman Avestimehr [00:44:48]: Model federation is also our angle on how to combine smaller scale models, maybe for a cloud solution that is competing and becoming a very strong collective model that competes with a large model. So these are the two recent innovations that we have been brewing in the past couple of months, and we'll be announcing soon.
Demetrios [00:45:12]: I think everybody would love to go back to, or everybody understands, at least conceptually, the benefits of smaller models. And so if you can prove that for your specific use case, that a much smaller model can do the trick, it's a no brainer to be using that smaller model just because it's so much easier on so many different dimensions. Right. And I do like this idea that you're talking about of pushing llms to the edge. I think I recently just saw a demo about someone doing this with llama three that was quantized or distilled, I can't remember exactly. And I'm pretty sure it was the smallest llama three. Like, how are we going to be interacting with these llms on our phones? Because that's my big question is, if it's just the chatbot, I don't really see the utility in that quite yet. And there's a lot of moments that I would rather use my apps and that way to navigate my phone.
Demetrios [00:46:17]: So I don't know if you've seen anything that's, that's interesting in that sphere.
Salman Avestimehr [00:46:21]: Yeah, I would say I think the biggest capability would be having the agent on the phone. So what is this agent so this is an agent that interacts with many API on your phone to do a multi step task for you. So for example, let's say this agent can interact with many APIs. For example, maybe your websites, your various accounts you have. And you would say maybe planning something for you. For example, find a meeting time for me. That works for me and Dimitris and send an email on the response. Multiple steps.
Salman Avestimehr [00:47:00]: The agent. Right. Simplifies you. Okay. So essentially I would say the role of it would be that typically if you want, you can do many things on your phone. They require a lot of steps and interacting with many different apps, this can streamline the process for you, do many tasks and automating it for you, right. So that way you can maybe again, you can make it simple. It's like, you know, you ask the agent, right, this is the topic.
Salman Avestimehr [00:47:30]: I want to have a summary of all the maybe mlops companies that are dealing with, you know, building and deploying llms. For those, you know, list the names, provide a one page, one paragraph summary and create that on a note and email it to me. Very good. So it goes with the website, does the search, gets the summaries, put it on a note, then summarizes that, create the email, send it. That's great. Like it's your own assistant. So, meaning that the idea of, if we believe that AI assistants are gonna be everybody is gonna have their own AI assistant, that they make it better on the phone is the right place because phone is always with you. How do you interact with your AI assistant? So that's what I see as the angle there.
Demetrios [00:48:20]: Okay. Yeah. I hope that day comes about. I wonder how far we are from that. Because of the demos that I've seen with agents these days, it feels like it's still a little bit of a ways off. And I don't know if it's a few months off, a year off, or ten years off, you know, for us to get to an ability where agents can be reliable and I can trust them to have my credit card and buy me a plane ticket in a hotel. And it's not going to like, buy me a plane ticket to Bali when I want to go to Barcelona or something.
Salman Avestimehr [00:48:57]: No, no, you're right. I mean, there is going to be verifications in the middle, meaning that depends on the sensitivity of the task. Some of them you can trust, some of them you can, but I think quicker ones are going to be many content creations on the phone. So that one, you don't want to do it on the cloud. Because of the cost and moving that is very well aligned. So those, I would say immediately coming content creation on the phone is very important. For example, let's say advertisers want to use that to create contents, right? So for example, let's say personalize advertising when the content is on the fly generated for you. Or maybe you want to create various content, like modifying your image, etcetera.
Salman Avestimehr [00:49:38]: Not all of them need to be a cloud service. It can happen on your phone. For example, you take a photo, that photo, you want to interact and say, maybe improve the image, put it next to the other ones, etcetera. Such applications, consumer applications are currently available. You do a lot of that on the cloud. But imagine somebody offers that application completely on the phone. Lower cost even, you know, can be much cheaper. Because if I'm offering that on the cloud, you have to compensate my cloud cost.
Salman Avestimehr [00:50:09]: If it is on the phone, you just need to compensate my innovation. You are using your own compute. So I would say those are quicker, immediate, like some of those generations. Instead of on the cloud, pushing it on the phone, app costs will come lower, cloud costs would be removed. And people maybe feel also safer because your day, your image, for example, is not leaving your phone. Still, you can create exciting images with it, right? Privacy is going to be amplified. That I call immediate. The other ones.
Salman Avestimehr [00:50:44]: You are right. I mean, these agents need to be involved, people need to train them, becoming embedded in your life, etcetera. That becomes more of a future. Maybe next year if we have another podcast together, we will reflect on those.
Demetrios [00:50:58]: Yeah, I hope it's next year. That would be nice because I'm ready to stop working as much and let the agents work for me. I wonder though. Yeah, that's, I think the biggest thing that is on my mind, because there is a huge movement for agents and everybody's saying, you know, agents are coming, everybody that plays with them, they really are trying hard to make them work and be reliable. And it is very obvious to me, this vision of, if we have Asians, what better place to have them than on our right? And why not have that all be federated? Because the phone and federated learning go hand in hand.
Salman Avestimehr [00:51:40]: Exactly.
Demetrios [00:51:41]: It's kind of like when I think about federated learning, I think about my phone.
Salman Avestimehr [00:51:45]: Definitely. I think that's the right way to think.
Demetrios [00:51:48]: Yeah. So, well, I love this. I appreciate you talking to me, Salman, and giving me the whole rundown on what FedMl is doing. The Genai platform that you've created, the loop of how things work within the platform. And so if anybody wants to check it out, I encourage them to reach out to you on LinkedIn. We'll leave a link to your LinkedIn in the description. Or also just go to FedMl website and check that out. It's in the description also.
Salman Avestimehr [00:52:19]: Wonderful. Pleasure talking to you, Demetrios.