Foundational Models are the Future but... with Alex Ratner CEO of Snorkel AI
speakers
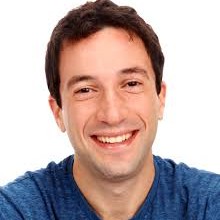
Alex Ratner is the Co-founder and CEO of Snorkel AI and an Assistant Professor of Computer Science at the University of Washington.
Prior to Snorkel AI and UW, he completed his Ph.D. in CS advised by Christopher Ré at Stanford, where he started and led the Snorkel open-source project, and where his research focused on applying data management and statistical learning techniques to emerging machine learning workflows such as creating and managing training data and applying this to real-world problems in medicine, knowledge base construction, and more. Previously, he earned his A.B. in Physics from Harvard University.
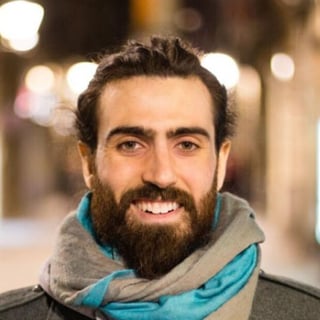
At the moment Demetrios is immersing himself in Machine Learning by interviewing experts from around the world in the weekly MLOps.community meetups. Demetrios is constantly learning and engaging in new activities to get uncomfortable and learn from his mistakes. He tries to bring creativity into every aspect of his life, whether that be analyzing the best paths forward, overcoming obstacles, or building lego houses with his daughter.
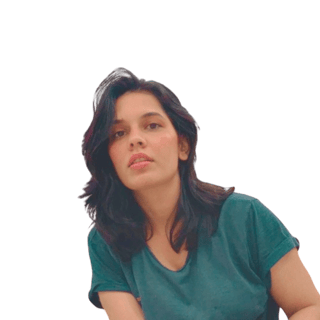
Abi is a machine learning engineer and an independent consultant with over 7 years of experience in the industry using ML research and adapting it to solve real-world engineering challenges for businesses for a wide range of companies ranging from e-commerce, insurance, education and media & entertainment where she is responsible for machine learning infrastructure design and model development, integration and deployment at scale for data analysis, computer vision, audio-speech synthesis as well as natural language processing. She is also currently writing and working in autonomous agents and evaluation frameworks for large language models as a researcher at Bolkay.
Prior to consulting, Abi was a visiting research scholar at UCLA working at the Cognitive Sciences Lab with Dr. Judea Pearl on developing intelligent agents and has authored research papers in AutoML and Reinforcement Learning (later accepted for poster presentation at AAAI 2020) and invited reviewer, area-chair and co-chair on multiple conferences including AABI 2023, PyData NYC ‘22, ACL ‘21, NeurIPS ‘18, PyData LA ‘18.
SUMMARY
Foundation models are rightfully being compared to other game-changing industrial advances like steam engines or electric motors. They’re core to the transition of AI from a bespoke, less predictable science to an industrialized, democratized practice. Before they can achieve this impact, however, we need to bridge the cost, quality, and control gaps.
Snorkel Flow Foundation Model Suite is the fastest way for AI/ML teams to put foundation models to use. For some projects, this means fine-tuning a foundation model for production dramatically faster by creating programmatically labeling training data. For others, the optimal solution will be using Snorkel Flow’s distill, combine, and correct approach to extract the most relevant knowledge from foundation models and encode that value into the right-sized models for your use case.
AI/ML teams can determine which Foundation Model Suite capabilities to use (and in what combination) to optimize for cost, quality, and control using Snorkel Flow’s integrated workflow for programmatic labeling, model training, and rapid-guided iteration.
TRANSCRIPT
Hey, I'm Alex. I'm, uh, one of the co-founders and CEO at Snorkel ai, uh, led the Snorkel project at Stanford prior to spinning out. I'm also on Faculty University of Washington. And, um, I think the, the prompt question was what kind of coffee do I drink? So, My, uh, my embarrassingly stereotypical, uh, uh, uh, Silicon Valley answer is I actually, I actually use a, uh, a kind of flash frozen coffee called commenter.
Um, it, it's, it's awesome if you, if you wanna check it out. And basically they ship these little capsules that you, uh, run under hot water and just plop into, uh, a cup of a cup of hot water. And, and that's, that's what I drink because it's. Um, I wish I was sophisticated enough to have, you know, to master the art of, of making it myself.
But, uh, that, that's, that's easy and fast and it's, it's pretty delicious if you wanna check it out. So that's my, uh, it's my coffee answer. Welcome back everybody. This is another edition of the ML Ops Community podcast. I am your host to Bere Os, and I. Am here with none other than the most incredible co-host.
Abby, what's going on? Abby? How you doing? Ah, I'm wonderful. I just, um, finished my workout, came back, took a . Uh, nothing better than recording an intro about, A podcast that we did a week ago. We've had some time to let this one sink in. And our special guest today was none other than the c e o of snorkel.
Alex, he also has written some pretty incredible papers. You knew him from his papers first before snorkel, right? I mean, he's been. Yeah, I've got a friend who joins Narco in 2017. 2018 18, so I had a chance to read some of his papers and was sort of seeing their open source work from the outside. I wasn't really involved in all of that stuff, whereas the sort of like keeping track.
I love the fact that he is starting to think about foundational models and how. ML ops and these gigantic models are going to start playing together. It's becoming one of the biggest buzzy words of the day or of the year.
I think the foundational models everyone is excited about because of their potential. But there was a lot of stuff that we dug into. We got into the papers, we got into just his view on the world. What were some of your takeaways? so I think the most imp important parts for me first was, um, our discussions around, On sample modeling, uh, which was how to combine different models because he was obviously using the supervision, but he was also starting to combine it with other deep learning techniques to be able to see if they can expand the horizons and stuff.
the other is more so his mention of. How to actually use foundational models because I feel like I've been somebody who's been slightly skeptical of elements. I mean, um, all the G P T three, G p T four, all that stuff is great. Um, but I've always felt like it was, it was.
Excellent as a hi because of the predictions not being that good and I was like, ah. So for high stake situation, we can't actually sort of use that one. This is so true. And we give a very interesting perspective. It was like, let's use them as base models and do feature engineering on top of it for u uh, use case specific scenarios.
And that makes perfect sense. That's so true. And the way that he talked. Some of their clients using these models currently that just shows that it's not all hype. There is value being derived from this. And so I'm excited as to where it goes and what we're gonna see in a year or two years from now. The adoption would be very interesting to see on everything. Yeah, it's gonna be really cool as time moves forward. So we gotta give a huge shout out to Snorkel for sponsoring this episode. They are incredible. I mean, I've loved snorkel even before they sponsored this.
I think they're doing some super cool stuff. And if you are interested in anything that we talk about in this episode, definit. Hit them up. You know what their website is already. But we'll leave a link in the description snorkel tot ai, and I think we might as well just get into it. Abby, any last minute things that you wanna say before we jump into the conversation?
Give us a comment on hug. YouTube, Spotify, apple, where? Where you seeing us? Give us all the good ratings in really good comments. , why not share this with a friend while you're at it? That would be in. basically you have, you've been pursuing week supervised learning, but why week supervised learning over say, some other sort of transfer learning or active learning? Uh, well that's a awesome, awesome way to, to dive in. So, you know, I think at a high level, the, the, the broadest interest that.
Has kept me busy for the last almost eight years, which sounds a little, not at all depressing to me. Although it sounds a little, a little one dimensional, uh, if you think about it in one, one way. But if you think about it another way, it's, it's, uh, um, there's just lots of dedication, explore, um, yeah. And there's lots to explore around, around data and, and what we think of as data-centric ai.
So I think, you know, top level concentric circle. Uh, you know, there, there's, and it's not an either or, but there's this, uh, you know, split that, that we make between model centric development where, you know, you might think of, uh, the, the model being relatively fixed and you're, or should the data being relatively fixed, you're iterating on the model.
That's what I often think of as the kind of. You know, Kaggle era of machine learning or the ImageNet era where you just, you know, it's what a lot of us started with. If you look at, you know, five plus years ago, every single paper was about features or algorithms or bespoke model architectures. That's what people were doing out in industry.
If they were, you know, actually working on a new problem and the data was someone else's job, it was something outside of the kind of ML developers responsibility. Right? And so this, this broader, you know, set of things that, that has been captur. Maya now many others interests, which is super exciting, and, and the snorkel team since back in 2015, is this idea of, of broadly data-centric methods where you're, you know, you're assuming that the, the model class can be auto selected or you can kind of pick a standard class that was kind of the forward looking bet that's not, not too forward looking anymore.
Uh, given, given how things have converged in such an exciting way out in the open. and what you're instead doing is really iterating on the data, labeling it, shaping it, developing it, et cetera. So within that, there are all these methods that you can view from the data-centric perspective and, and I'll, I'll rephrase them my own simp, simplistic way, but you know, you've got active learning, which is essentially saying, let's be smart about where we look on our next iteration of developing or labeling.
You've got transfer learning, which is, let's see if we can kind of reuse something from a prior dataset or, or model, uh, to kind of jumpstart the performance. And then you have things like week supervision, which I would phrase as how can we be radically more efficient and, and, and, and scrappy a around how we actually do the labeling once we're, we're looking at something.
So you. kind of see, obviously my way of phrasing it is, is, is going to lead into, to the way we view it, but we view these as, as, as very complimentary techniques. They're all, they're all tools in the toolkit. And it's worth noting the, the kind of, you know, at least the cautionary note I'd give first, which is that, you know, each one of these, you, you can find instances of them being pitched as magical silver bullets.
And, and those kinds of, you know, free energy machine pitches don't, don't really help real practitioners. That's our, that's our strong perspective. After eight years of working in this space, active learning has been around for, for decades. Amazing progress in the field. I have a, a grad student, I, I get to Coady UDub, who I just published a paper on active learning plus week supervision.
Actually, it was some cool results, but, you know, it's, it's, it's not a silver bullet. Even if a little theoretical intuition, is, is that, deciding where. , you need to look next in the data is, is, is as is often as or more difficult than actually, you know, deciding on the decision boundary that you're trying to build and say, training a classifier in the first place.
In other words, intuitively, if you knew exactly where the model needed more signal, that's as harder or, or or harder of a problem than actually training the model in the first place. You don't know what you don't know, so to speak. And so, um, these are all really helpful techniques, but they're not silver bolts and, and.
Our view is, and this is what we've, we've, but both put out research around, but also build around our, on our commercial platform snorkel flow is that they all work together. So, you know, in, in, in our conception of a data-centric, uh, you know, iterative workflow, you know, each iteration you're saying, how can I, uh, label or, or otherwise improve the, the, the quality of my training data and active learning guides you to.
you know, where to look to go next. Where should I label by hand? Where should I label programmatically or with weak supervision? Where should I debug or, or edit? And there are a whole bunch of ways of doing that, but that's the kind of the, the where do I go next? Then there's the, how do I label, do I go through and, and kind of click one data point at a time in the kind of legacy manual labeling approach, which can be appropriate?
And, and, and inevitably is part of the process in in, in tough problem. . But then the weak supervision question that we've been working on for years is, can I be more efficient? Can I write functions at label data? Can I even use, uh, things like foundation models or, or large language models, which we will, uh, we'll, I'll, I'll, I'll take a wild guess.
We'll get into in, in our discussion today, and that gives a hint about how we, oh, how can we not, yeah, how can we not, that gives a hint about how we think about, about fitting it into this, this data-centric work. We can talk about why, but basically how can we use all these more efficient ways to label the data?
Um, also more maintainable ways, things that look more like labeling your data with code so you can now edit and change and share and reuse and govern, uh, which you can't do with big piles and manual labels. And then transfer learning, we think of as just a way to kind of raise the baseline that you're starting with, right?
So whether it's classical transfer learning or it's zero shot or few shot methods, uh, Surprise, surprise will, again, take us back to foundation models. Uh, if we, if we dive in there, you know, that's the kind of, okay, how can I start with, with a baseline performance rather than starting from scratch. That's actually why, uh, you know, myself and, and my co-founder Chris, uh, uh, who's, who's uh, um, was part of starting up the Stanford Center for Research and Foundation models, like, uh, likes that foundation model term.
Cause we think of. You know, foundations, they're kind of sturdy, generic foundations. And then you still have to build your specific, you know, custom building or house on top. But you wanna start with, with those strong foundations. So to summarize, you know, transferring, think of it as kind of your warm start versus your cold start.
Uh, your, your kind of base. Most problems are not magically solved by transfer learning if they're complex and non-standard. , right? Um, most problems are not solved by zero few shot learning when they're actually kind of tough, high value, bespoke problems on custom data sets, custom objectives out in, in, in, in, you know, real industry.
But they're great. It's a great way to start active learning is, is kind of the, the where do I go next to label or improve my data? And weak supervision is how do I label my data in a more efficient and maintainable way? So, a little bit long-winded, but that's how I see them all, all fitting together and, and, and playing, playing nicely.
And there are two ways to go from here. One is we directly jump into the foundational models, but I had few more questions about your papers. So I'll ask you the questions about papers and then we can go into detail on foundational models. So one of the things, I was reading your paper on NEMO and you were talking about labeling heuristics and how to allow users to participate or inject their knowledge into the labeling process.
Can you talk a little bit more about that? And Alice thought you thought that we were gonna like, do pleasantries and say hello and go through, like, what's your background? We just jump right into it, man. , I didn't, I I didn't even get, I didn't even get to do a proper intro. We, we, we had no, the two hardest hitting topics I can imagine.
Uh, you know, sick kids at home from preschool, you know, disease vectors and then straight into, you know, paper interrogation. I love it. I love it. I'm Alex by the way. I think, uh, you know, there, there, there's our, there's our. Let's, let's dive right back in. This is, this is the fun way to do it. So, um, the, the, uh, so the NEMO paper, this is from a student I get to work at the University of Washington, uh, Cheng Yu, who's, who's awesome.
This is actually what I was referring to when I said the kind of, uh, recent work on active learning plus programmatic supervision. So the idea at a high level in, in Nemo is it's kind of building on this, this snorkel programmatic supervision line of work where, um, you, you start by, you label data by trying.
Write a, a function rather than just labeling individual data points. And this function, we've, we've, you know, you should call labeling function, labeling heuristic, you know, sometimes we, we vary at the names, uh, to, to, to, to, you know, uh, try to make the, the academic work more, more generic. But, um, you know, I'll, I'll refer to it as a labeling function here, which is our standard terminology.
And, you know, the idea there is, is, like you said, it's a way of injecting domain e. So I can, so let, let's, let's just focus on that part. This is kind of the snorkel idea, and then we'll talk about where active learning comes in and the NEMO work. The basic idea here, one, one way to motivating is actually how we started the, with, with the snorkel project or one of the, the, the, the motivations.
It was this awesome project through DARPA that we were funded under DARPA Simplex, and they had, they paired us with what, what they call SMEs or subject matter experts. So we were working with some, uh, genomicists, uh, some collaborators at Stanford. Some they, they do some awesome stuff on, on pediatric genomics that actually has interventional impact.
So really, really exciting motivating, uh, projects. Unfortunately they can't solve the common cold in, in, in two to four year olds like we were talking about at the beginning. But they just for some, for some random person that just drops in on this DARPA, working with SMEs and the genomics or genomics , if you were just a little bit outside of the box, it's like, wait a minute, is this some like role play game that I don't know about?
Or rpg how get here, you know, . Yeah, exactly. How do we get here? Uh, you know, it's, it's like, uh, you know, I get, I get the hard-hitting questions like setting up flares, you know, all the, all the, all the random other, uh, pieces. But no, I'll, I'll arc back to our, our m ml ops and, uh, and, and, uh, data-centric ai, uh, bit, which is that a lot of what we spent time doing, this is when the data-centric stuff started to hit us in the face Bulk of the project was not building our fancy machine learning models.
Uh, back then in 20 15, 20 16, that's what we kind of thought we should be doing as ML research. Most of the time was spent labeling and relabeling and debugging training data and, and error modes. The model, and all of it consisted of sitting there with a SME saying, okay, the model labeled this, but you said this.
Why is that wrong? What features are we missing? What, what, what things are we missing? Um, and then, okay, let's label this data. And the really interesting thing we did, you. Multiple sessions a week, is that as they were labeling data, these, these collaborators were talking about why they were labeling the data.
Well, I see this, this negation here, and I see this kind of phrase, so I know it's, it's this kind of thing versus that kind of thing. But then all of that information was getting thrown away and not passed onto the machine learning model. All we were giving the machine learning. Was just the XY tules, the here's a data point, here's the label.
So a lot of what we've been pushing on for many years now, you can view it, you know, almost as just saying, let's, let's not be egregiously wasteful with this labeling process. I mean, this API of all that you passed to, the machine learning model is a data point and a label for supervised learning has been incredible for the.
it basically has let people do their model, model center development and, and not even think about the data and all the domain expertise that goes into it, which for a period in the space, I would argue, has been amazing. It's, it's led to this, this gold Russian boom of, of model progress. But if you think about it from a real world sense, why are we playing like 20 or 20,000 questions with a machine learning model?
Are, are, are, are SMEs. The people who are labeling the data, they, they know all this information. They know that I'm gonna label it here cause I see this, this key phrase, I'm gonna label it this way cuz I see this pattern or I reference it with this external resource or, or whatever it might be. And then we just throw it out and we ask the machine learning model to try to statistically reiner it.
Why not just give it to the model? So that's a little bit of a long-winded arc of saying what is this idea of a labeling function, this programmatic supervision or, or weak supervision? It's that we can label training sets often tend to a hundred times more efficiently measured in time taken to do the labeling by just giving more information, labeling with, with functions, uh, or, or heuristics or pattern.
rather than just by hand and accepting that it's gonna be a little messier than individual data labels, and we're gonna have to clean up that mess. Algorithmically, which is the weak supervision part. So I'll take a, a brief pause there, and then I can get to the, the, the NEMO paper, which, well, I'll, I'll give the, the quick bit there, which then ties into active learning, which is basically saying, okay, that's the, going back to where we started, that's the, that's the, how do I label?
Let's, let's, let's get richer information from the, the, our experts who are labeling the data in the form of a labeling function rather than just, you know, click, click, click. still, we can also be smarter about what data we show those people to, to label next. So regardless of whether you're just clicking and labeling in the legacy way or you're writing a, a labeling function to give richer information, you can still be smarter about where you look next to, to, to build that.
These labeling functions don't just come in and vacuum. They come from looking at the data and saying, , oh, I should, I should label it this way. Or, you know, et cetera. And so the Nemo paper combines the two and says, let's both be smart about how we label and where we label on each turn of the of, of the of, of the, of the crank.
this also ties up with another thing. You talk about geocentric AI in something which has been my personal experience, which is, Often the model you pick, whether it's for classification or regression problem, or even for labeling, it depends very heavily on the DR itself. And in your originals article paper that you published in 2017, you said there are, there are two directions from here.
One is we figure out an end to end system for. They programming for textual data, and the other is we look at different modalities, including images with ET sector. Would it be fair to say weak supervision works very well for textual data and may or may not work? I don't know the research on that one, but it does, it work equally well for other modalities.
So we, we started in text because we're, we're. , uh, we're, we're big nerds around a lot of the really exciting problems and natural language processing and, and knowledge extraction, which is a lot of what we worked in. You know, there, there's such a gold mine. I mean, I could draw on about this too, about, you know, all this information that's kind of, uh, more, you know, more accessible than ever before in one way, you know, you can.
I mean, I won't, I won't, you know, repeat the kind of, you know, I don't know, uh, uh, uh, you know, uh, market stats on, on data availability. We know there's a lot, a lot of data out. There's more accessible than ever before, but it's also. The knowledge in that data is so inaccessible. So a lot of what we do, we started with what we do today is all about, you know, classifying, tagging, extracting, pulling, structured, usable data out of unstructured data, whether it's, you know, a bunch of scientific papers, uh, back in the Stanford days, or whether it's, um, you know, uh, multi hundred page contracts in a bank or, or, you know, or an insurance agency, et cetera.
So that's where we started. But image has been a, a big focus. Actually, my co-founder at, at the snorkel company, PERMA did PhD. Uh, or the, the big applied focus was on image and video, and I think we've actually published more papers, especially on the medical side, a couple nature comms papers on image and video and week supervision there than we even did on text, which is a weird artifact, but, but it is, is true.
And so we're actually really excited, uh, about progress there. And actually that takes us to one thing that we've been teasing, but I know what I wanna talk about, which is foundation models today, which is how foundation models, uh, we see fitting into this. This picture, I'll, I'll just preview that. A lot, a lot of the image use cases are, are accelerated by these foundation models as well.
So one way that we'll get to. , I wanna take a top down. But, uh, this idea of a labeling function, you can kind of think maybe in more simple ways about, oh, I'll in text, I'll look for a pattern, or I'll look for a key phrase or something like that. But in both te, an image that might seem harder. How do I do that over pixels?
There are answers to that. You can, you know, you can. Uh, run feature detectors or object detectors to give you building blocks that you can then write labeling functions over. That's some work we published in 2017. Um, you can use metadata that may be, you know, uh, textual or structured, but you can also now use labeling functions based on foundation models that allow you to kind of write heuristics, write labeling functions over image using, uh, some of these great.
Image-based or multimodal foundation models that give you these kind of powerful ways. You can literally just write a natural language prompt to, to, to write a labeling function over image. Now, given these, these amazing models, and so the foundation models play a big part of that about, of that strategy as well, which is why we've been seeing some exciting image results and our, and our rolling that out also now on the, on the, on the company side.
Um, I love that. So one thing that I think foundational models do not get enough credit for is the use cases around. Enterprise, like actual things that make money, right? Like you hear a lot of people saying, oh, well yeah, foundational models are great. If you want to make a cat riding on a rocket going over the moon, that's cool and it just gives you some picture.
Or it's doing some like runway. ML is doing some insane things when it comes to video. , but when it's like, but let's get down to business and the adults in the room still need to predict fraud, you can't do that with the foundational models right now. I think you have a bit of a different opinion, like you, you know, enterprise use cases when it comes to foundational models.
Can you break some of those down for us? Yeah, I, I, I think it's a, it's, it's a, you know, a great, a great prelude because there's incredible excitement and incredible real. Value and magic in, in these foundation models and the way that they've gotten scaled up. and yet we see such a gap between that and, and real enterprise usage.
And so the fact that that gap exists. is, is very real from what we see, right? We work with a bunch of, you know, the top US banks and government agencies and, and, and insurance, uh, uh, you know, uh, healthcare Telecom across a lot of verticals. And in a lot of these settings where there's, you know, high business or mission or, or, or, or patient value, which, you know, high value also goes with, you know, stricter constraints and, and, and, and greater consequences and governance, uh, stricture, et cetera.
You, you, you don't see foundation models being, being used. And, um, so, so let me take a step back and, and, and give our, our view on this, uh, from, from experience in these, in these use case settings. And I'll characterize kind of two gaps here around the challenges of adaptation and deployment and, and those two things adapting foundation models to these, you know, to the, the challenging custom use cases in that, that have high value in enterprises and.
Solving the deployment challenges of how do I actually serve, you know, a foundation model in production. You know, quick preview answer is that, that, that most enterprises can't and don't think they will be able to for a couple of years for not just cost and latency, but also governance reasons. Um, so how do we solve those adaptation deployment challenges?
So let's talk about the adaptation one first. There's, there's, um, , and, and I can start with an example, but one way of viewing it is that if you have all these kind of generative, creative, exploratory use cases, I wanna generate that, you know, the, the, the cat on a rocket ship going over the moon, or I want to generate some copy texts that I'm then gonna edit, you know, um, foundation models can do amazing things right out of the box in this kind of Hume, the loop, um, kind of procedure.
One way of thinking about it is there, there are looser accuracy constraints. because you're using it as part of a creative process, not as something that has to spit something out with, with, with, uh, you know, a, a certain high bar of accuracy, right? Yeah. Versus you're augmenting humans' abilities as opposed to replacing it.
Yeah. And, and it's, it's, it's super exciting. And, and, and, and, um, I'm, I'm a, I'm personally a big fan of on the amateur side of, of that, those loops. And I've been playing around like everyone else with these. , but that's very different from the kind of, uh, you know, automation or, or what we would more technically call discriminative versus generative use cases, especially in complex settings where you, you have to achieve a high accuracy bar and also you have to do it in a setting that is not the same as the data these models are trained on, on web text, right.
so that I'll get, actually, let me give an anecdote, uh, really quickly. Th th this was, um, yeah, I was playing around actually, uh, early on with, uh, stable diffusion to, uh, get a, a, uh, little snorkel logo. You can can see this, this is a snorkel logo wearing a, a spacesuit for one of our internal hackathons. But I was saying, okay, what can, what can, what can, uh, you know, generative AI these days with foundation models do here?
I had an awesome experience. It was really exciting. I went through about 30 samples until I got something that looked pretty cool. I couldn't get the octopus wearing the snorkel underwater. Maybe that was too far outside of the, the support distribution, but, but, um, I got a really cool image. So from a creative generative workflow, that was a big success.
But if you think about it from a kind of production automation or, or discriminative modeling per. one success after 30 tries is like a 3.3% kind of hit or accuracy rate, right? That's abysmal. So how do you get from one to the other, and how do you kind of bridge that, that gap, which is both about, you know, tuning the accuracy and then doing it on very bespoke use cases.
Uh, we should always be very careful, I think, about drawing analogies between AI and humans. Given how, how, you know, How, how dumb AI still is and how we're still struggling to solve very simple challenges in, in, in real, you know, production use cases, but one analogy is kind of generalist to specialist. If you have someone who's learned to read on, on, on Reddit and the internet, you, you wouldn't expect a human to be able to just suddenly reach multi a hundred page contracts or analyze very technical fraud or, you know, network telecom data.
you know, right off the bat, they would need to have some so true adaptation. Some, some, some tuning, right? So this gap from generalist to specialist and from kind of loose accuracy constraints in the generative world to high accuracy bar in the discriminative world, that's this kind of adaptation gap.
And the standard way of, of crossing it is by fine tuning, this is an old transfer learning concept, right? You just fine tune the model, uh, on, on labeled training data and. You know, once again, you're back to this data-centric ai, uh, problem. You can do prompting and zero shot or few shot techniques, but most studies show that, and our, our internal results also corroborate that if you want to get on these very tough problems to high accuracy, usually you're just going back to fine tuning on label training data.
Probably less than if you were starting from scratch, but still for these bespoke problems quite a bit. So our view is surprise, surprise that , you know, adaptation, this adaptation gap once again comes back to data-centric development. You're not like, when you wanna do this adaptation gap, you're not going, another way of thinking about is you're not going into the foundation model and, you know, tweaking some of the multi-billion parameters.
I mean, there mm-hmm. , there's some cool work on patching and, and, and, and stuff that's going on. Y you're not, you're, you're kind of leaving that you're, you're fine tuning, you're, you're adapting. And that's all about labeling, editing, developing data once again. So you've got this big adaptation gap that a lot of peop uh, that's at least how we frame it, you know, crossing this gulf from generative to, to, to discriminative or prediction, uh, automation.
And also crossing from kind of generic web techs where all these models are trained to, to custom settings. Yeah. Uh, or specialist setting. . And then the other one that I think a lot of people underestimate is this deployment gap, which is that, you know mm-hmm. , I, I, I talked to one of our, our customers at a, a large, you know, top, top three US bank.
And they, they said that they were, uh, talking, they, they were thinking about submitting sign to their model risk management committee about getting g PT three and production. And they, they likened it to an ARC project, uh, kind of a, a donkey hott, like tilting at windmills, uh, activity . So this was their, you know, their, their pessimism about when that would happen given that they're, you know, they're, even challenges getting modern, deep learning models kind of through to production.
And honestly, I, you know, there are cost barriers. These things are very expensive to, to serve. There are latency barriers. They, they, you know, take time. We're gonna chip away at that. One of the biggest ones is, is when you also throw in governance, because we are just beginning to understand these, these large foundation models, it's actually doing really exciting things on the academic side.
It's almost like a return to natural sciences world where you're kind of poking and prodding empirically rather than just reasoning formally. But yeah, I think enterprises are, are, are justified in, in saying, Hey, we have to understand a bit more before we just kind of, you know, serve G P T three to customers, even if we can solve the cost challenges.
So you've got these adaptation gaps. How do we do the data center development to adapt these models for these very specialist high accuracy bar setting? and you've got these deployment challenges around how the heck do we actually put this into production the next year or two or three in, in, in these large, um, enterprise settings.
And so, um, this is something where, where we have, uh, an opinion as you could guess, and we're actually, uh, about or going to be announcing what we call, uh, our, our, our support and platform for data centric foundation model development. And the, the basic idea here is first of all, for the adaptation, all that fine tuning.
That again, is where we've been working for years about how do you make data labeling and training data development as as rapid as possible through programmatic techniques and active learning and transfer learning and all these things, and the deployment gap we solve by actually using these foundation models to help us label data, which we can then use to train smaller models that fit into our deployment paradigm.
So one, one cool. Set up. We're gonna pu. It'll be up soon, a case study on some public data that we can really share the full results. Um, it's like a hundredweight classification problem on contract, so still even simpler than some of the ones you see in production, but a little bit more representative.
And the cool thing is if you take a baseline of fine tuning G p T three on a bunch of manual labels to get to a a high accuracy bar using our approaches, you can use less than 1% of the ground truth labeled data and actually train a model that is over a thousand times smaller. and hundreds of times cheaper to run to actually go to production.
So think of it as you're using these big bulky generalist foundation models to help accelerate data centric development of smaller specialist deployment models that are both Oh, wow. More accurate with less n but, but also, um, you know, far cheaper. And, and again, this may sound like it's, you know, too good to be true, but it's really just, it's really that generalist to specialist divide, right.
In an enterprise deployment setting, we're not looking to have. Gigantic generalist creative machine that we can query for anything. We're looking to solve one task on very custom data, very custom objectives with high accuracy and repeatability and, and, you know, uh, uh, you know, ability to, to, to, to apply proper governance.
And so what we're trying to build is, is, is we're aiming to be that bridge between all this foundation model goodness, and these actually deployable models in these production settings, if that makes. I love that it's like this offspring of the foundational model or the, the child of it in a way. And you've got your mini foundational model almost, but it, you are echoing something that I was just talking to a buddy Danny yesterday about, and he had worked at Google and he for like.
Eight years, he was working with researchers on trying to figure out ways to get their foundational models, like finding ways to put them into products. Right? That was one of his main things was syncing between the engineers and the researchers to find different ways to get those foundational models into the different Google products.
And one thing that he talked to me about was he was saying how with these certain types of models, You have to be okay with that lower accuracy score, and you also have to be okay with the idea of. , the risk of error or cannot be high stakes, like what you're putting this into play for. It cannot be high stakes because that accuracy score is so low, so you can't ever, you can't do it where it's like, like we were talking about with these fraud model fraud detections where it could really mess things up.
And so he. Made the comment of like, yeah, you know, social media, you could throw it in there because if somebody sees the wrong post, whatever, it's not really ruining anybody's day, uh, just because of one wrong post or whatever. So now it feels like though, What you're talking about is much more, let's figure out how we can take these gigantic models that are covering so much surface area and then take the pieces that we like, extract those pieces that we like.
Use the foundational model to train those pieces that we like, and then we can have that higher bar and we can put it into much more high stake setting. Yeah. E, e exactly. Uh, said said even better and more succinctly Actually, we, we, we may, we may share this contact though. I, I, uh, um, uh, cuz I, I also, we had a, uh, a Dan from Google Research, uh, who worked in this area come by the lab a couple years back to give a talk and, uh, no way.
I, I won't, won't do full names on, on podcast, but, um, later we can talk. Yeah, yeah. We'll either have definitely, you know, heard about this. Now, taking a step back, just even without. , well, at least without my metaphorical snorkel hat on, cuz I'll keep my literal hat on. Uh, right now I, I desperate need a haircut.
But, um, strict orders from the wife, just keep your hat on whenever you can. , especially on video. Yeah. That's gonna go out onto YouTube . Yeah. Um, the, yeah, I, I think in general, thinking of these settings where I often think of it Yeah. Like, it, it doesn't have to be perfect accuracy or you're, you're kind of competing against a null baseline.
I think in general, these are great places to apply any type of machine learning, uh, especially these, these, you know, foundation models where, where they're not tuned and, and, and, you know, very flexible, but, but not at this kind of like, you know, s accuracy SLA bar. So I, I think that's a good strategy to keep in mind in general.
Right? This is why a lot of ML projects I think are really successful and things. , like, let's take our work we've done on the medical field. We did a lot with radiologists at Stanford and VA Healthcare. We did suffer on triaging rather than automation. We said, okay, like, let's, let's triage a queue that's backed backlogged because the baseline is just nothing.
It's just the doctors reading the order that came in. So any improvement is better versus, okay, let's take on full responsibility of automating what a doctor does, which, you know, is, is a, is. , you know, big consequential bet to make, and I still think we have a ways to go there. So I think in general it's, it's a, it's a great kind of divide to think about it.
It's definitely what we see for foundation models too, right? If you, you know, again, think of these are these big generalist, generative, uh, uh, models that are amazing in what they can do, but they're not fine tuned or specialized to get, you know, amazing accuracy on some specific. In fact, even making them kind of better on generalist tasks often requires fine tuning.
Like there's the flam results that came out from Google recently, and you know, what, what did they do? Um, they, they fine tuned on all these, all these tasks. Uh, so actually you see in the broader space, of course I would say this. I'm, I'm, you know, I'm talking my book. I'm, I'm, I'm sticking to the, the, the, the data centric stick.
But even in, in building these generalist models, you see a lot of data centricity in, in the thinking. Everyone's kind of converging on more or less the same architectures. There's obviously amazing engineering work that goes into it, but you look and see, like, you know, you look at some of the models that come out, you know, this is, you look at the stable diffusion, uh, release, you look at some of the, uh, one of my colleagues, U UDub, Ludwig Schmidt published some interesting work, uh, with his team on, on, on clip models.
Um, uh, open Air Whisper is another one that has some good details in the data. All these models flam, they. One of the biggest vectors of innovation is how you curate the data going into training them. So, um, so even, even in getting these foundation models built, we're seeing, you know, surprise, surprise the data.
They're trained on the curation of that right? Stable diffusion. They had a separate semi-supervised model where they labeled data to look for beautiful images and then bootstrap this model to sub-select down to a trading corpus of nice looking image. Right. So, you know, even in, in just building the foundation models themselves, you're already seeing, you know, data-centric iteration, data-centric development being key.
So, you know, we're just saying, okay, now when you want to take that next step of actually putting them into a production setting, a specialist setting, once again, it, it often comes down to the data, both to fine tune them, but also to be this kind of, Into a deployable artifact that you actually, you know, can, can put into production today.
Very interesting. And this is one thing I often think about, especially because as a developer, the foundational models are very interesting. You get pre-trained model, somebody's spending up the money and giving it to you to play with. That's all cool. Uh, but when it comes to, Hey, are these the best models to work with for.
Actual business problems, I am often questioning is it the right way to just be able to increase the number of parameters and just optimizing and optimizing further? Should there be better models or should we be putting more research into better models that are generally optimized for these kind of solutions?
Given we've done already done a lot of work in meta learning as well as generator models. Yeah, I, I think, I mean, I think we're gonna see progress from. All kinds of directions, right? So I think, and, and you tell me if I'm misunderstanding the, some of the, the points you're making, but we're, I think we're gonna kind of see chipping away on one end of making these models more specialized and, and making them kind of better for sub domains, right?
You know, like a, a legal, a medical, you know, uh, G P T, whatever it might be. And then, you know, on the, on the, on the front lines of, of, of kind of trying to get these, these productionized, you know, uh, what we're trying to do is just, you know, Find good ways to, to, to use them now. And actually going back to what you were asking about on our first que, the first question about, you know, weak supervision and this idea that, that has underscored a lot of our work of, you know, labeling data programmatically and then being able to combine multiple and perfect signals, which is kind of the, the crux of the, of the thinking there.
That's part of how we, we, we got so excited about, found foundation models in this way of using them. Because you're right, as a, a developer, um, What foundation model should I use? Uh, uh, what's appropriate? First of all, there's, there's a, an exploding universe of them, right? And especially in the open source now, which is fantastically exciting.
And then now on top of it, you know, you have all these different ways of prompting them, right? Which is, you know, I mean, of course you have fine tuning, you have a few shot learning now, but now the, the zero shot technique of prompt. Is, you know, , there's, you know, new, weird little, uh, you know, prompt sentences you have to write every single day coming out.
And so how do I actually now develop the prompts to kind of coax the right information out? Well, in our week supervision, formalisms, that's just another type of labeling function. Um, so, and this is actually one of the features we're releasing. There are actually kind of three that we, we call one is, uh, fine tuner.
So just we're, we're adding support and snorkel flow to fine tune some of the newest, uh, breeds of, of, of, um, of, uh, foundation models. So if, if you actually can ship a foundation model to production, you know, now we can let you fine tune that with this programmatic, uh, uh, data approach that, that we support and have, have built.
And then we have these two other features called Warm Start and, and Prompt Builders. So Warm start is basically, The kind of what I was talking about, transfer lending as a kind of, um, you know, a bootstrap baseline using foundation models with zero shot techniques. Just auto label your data as a first pass on one of these specialist, you know, production problems.
They're not gonna get you up to the accuracy level and all but the simplest of, of, of problems. Like, for example, we were using this with a, um, A large bank who's a customer on an anti-money laundering and know your customer problem, where they had to pull hundreds of, of, of, uh, pieces of information out of these big piles of customer documents.
So some of the simple things like, what's the name? Or, you know, what is the date on this contractor? Or things like that. Actually, just using this kind of warm start zero shot approach could, could get pretty accurately, which is, you know, exciting and you kind of expect the bulk 80, 80 plus. It gave a kind of jumpstart, but, but it was nowhere near accuracy level.
So you can kind of basically warm start versus cold, start your problem and get some of the, some of the low hanging fruit labeled. And then you can use programmatic labeling. Write these labeling functions to rapidly label the rest of the data up to quality and this iterative approach that we support in the snorkel flow.
But now you can also write what we call prompt labeling functions, which is basically writing domain specific prompt. For these various foundation models to try to label parts of the data set. And then the best part is that all of the weak supervision formalisms we've built for years, they're all about, okay, how do I combine all of that signal into my final training set, final model, so that you as the developer, don't need to worry about, Hey, you know, should I be using, you know this, you know, G B D three or Bloom?
Should I be using this prompt or that prompt? You just kind of dump them all in and snorkel takes care of modeling and integrating. , which is, you know, that's how it kind of fits, uh, a little, a little in the weeds, but that's how it fits nicely into the, the, the kind of paradigms we've worked on for, for years.
It's all about, you know, uh, uh, how do I opportunistically use all the signal I have at hand for labeling in a more efficient way. and in this rendition foundation models are just another tool in the toolkit, an immensely powerful one. Uh, but it also an imperfect and tricky one that you have to tune in an engineer and is not going to work magic out of the box on your specific problem.
So why don't you just throw it in with all the other kind of signals in this data centric workflow? That's kind of the approach we take and we've been saying seeing it work pretty well, uh, for customers even in, even in early. So what you're saying is basically use it as a baseline model, the way we use Omo models to sort of foresee the baseline and then we build the model on top of it and see, okay, let's do feature engineering on top of it.
Yeah. Start, start with it as a baseline. Again, that's that kind of concept of foundations and then, you know, do this iteration on improving the. Uh, it could be by writing, you know, engineering specific prompts to kind of fine tune the output of the foundation models. It could be by writing other labeling functions and just say, Hey, if you see this pattern labeled this way, using that kind of specialized domain knowledge and then.
Put it all together. That's what snorkel does for you and turns it into a clean training set that you can use to train or fine tune any model that you want for deployment. I see. Very interesting. Now I'm gonna ask you one more question, one more piece of snorkel that really excited me, which is basically you now have your own hosting infrastructures, Oracle Flow.
How does it compare against gcp? Because I know your focus was building integration as a service platform. Yeah, so, so we redeploy and, and, and, and kind of connect with all the, all the, the cloud environments and, and all the, um, all the main ML vendors. And so, uh, the key for us is we, we just wanna support this data centric workflow.
And so we connect to, you know, whether it's a, a Vertex or SageMaker Azure, uh, or, or a home-built machine learning modeling solution. We just want to get you through this data centric. Workflow on whatever platform. Uh, and, and we actually run the gamut there too. We have our, our snorkel flow cloud solution that is, that, that we host.
But we also do a lot of, you know, customer VPC on-prem deployments actually, because a lot of our customers are working on very, you know, critical problems with sensitive data. That's part of why it's so difficult for them to get it labeled, and it's part of why, you know, the GPT three s of the world don't work out of the box because it's this.
private custom on-prem data. Very critical. So because of that, we're very flexible in the deployment environments. And then just because, I mean, the data science base is amazing in terms of the amount of tools, whether open source vendor that are out there. And so, you know, we think interoperability is a, a, a, a first class design principle for, for any platform.
And, and our, our, um, at least our high level shtick internally and, and, and with customers in terms of our design principles, building our platform, snorkel flow has always. You know, we do want to provide comprehensive support for our workflow and, and in particular, this data-centric workflow. So, you know, um, o over 62% of our customers use us for kind of end-to-end development of applications, start with raw data and get to get to some model.
And they do that in, in snorkel flow. , but we also want to make intraop as easy as possible. So if you wanna label your data and snorkel flow and then go train a model, you know, in your own custom uh, uh, environment, and do some model centric integration there, and then come back to do edits on your data.
We try to make that as easy as possible too. So last question for you from my side, y'all came out of, I. I got a buddy who just went over to Chris Ray's venture capital firm slash incubator slash everything machine. . You all came out of there. How was it? Can you gimme some background on what that was like to you and how it inspired you on what you are doing now?
Well, you'll, you'll, you know, Chris is a, Chris is a, a, a, an extremely unique, mad genius. He's, he's one of the, uh, uh, one of our, or the, actually, I can't, I can't give him too much credit on public, uh, record. It'll, it'll, uh, I can't give him that much. So I'll just say he is one of the smartest people I've ever had the fortune of working with.
He was my advisor back at Stanford. Um, and, uh, he, he's a, he's a, you know, him and, and his, his. . It, it's, it's a, it's a pretty incredible place. So thi this was, uh, back in the quaint all days when it was, it was just, just the lab, Noah, no, no, no, factory. Um, but I mean, yeah, I, I, I could, I could rattle down for a whole nother hour on, on, on things that I learned from, from Chris and from that environment and, you know, continue to learn working with him.
Um, but I, I think maybe just a, a couple things here to tie it back to the themes we've talked. You know, one of the things that I think was pretty unique and, and is still with, with that, with that group that I, I, I both resonated with me, which is why I, I, I, you know, gravitated to working with Chris. Uh, and then, uh, that I, that I also took away was, you know, the importance of.
especially in a field like machine learning where there's so much, you know, there're, you know, people churn up papers, they're new fancy methods every day. There's, there's, there's lots of, you know, there's lots of shiny things floating around. Right. Uh, uh, you know, Chris and the people in his lab are geniuses at, at, at those pieces as well.
The snorkel team, the, the sub-team that spun out. We've, we've made our contributions on the theory and algorithm side. , the importance of just getting your hands dirty with real use cases end to end. Hmm. Um, that anchor point, you know, be being good on the, on the, the, the, the principles, the, the, the, the fundamentals, the, the, you know, the, the, the formalisms.
Um, but also pushing yourself to spend time just, you know, sitting with that genomicist or, or, or clinician or whoever is actually trying to build something and actually taking the weeks, months, years to just see that through. We, we never would've started on data centric AI if we hadn't been doing that.
Cuz we were thinking, okay, we're gonna work on, you know, x fancy machine learning modeling technique. And our users kept saying, okay, no, we're stuck on the data . We just need to label the data. That's what's blocking us. Do you have any solutions there? I mean, it took us long enough, but you, you don't actually, I mean, it sounds trite, but I think it's, it's true in this field, it's, it's very appealing to, because of how we've set things up.
It's wonderful for progress in some ways, but it's very appealing. , download a benchmark data set and try to build the fanciest model to get the score to go up or to build the best cats on rocket ships, forcing yourself to actually work on, uh, real production use cases while also, you know, doing your hard work to study.
You know, uh, uh, Avi, those textbooks that, that, uh, you know, you asked about in the background that, that we, you recognized a couple of them that I'm, I will not confirm or deny if I've read every single page of the ones you'd referenced, but like, you know, learning the fundamentals, but pairing. Just getting your hands dirty with real, real problems, real data.
That was one thing I took away. And then I think Chris and that whole, whole whole group, that whole machine is very good at, of, of, of balancing the two. Because if you just ha, I mean, if you just hack, if you just look at real, real problems, you just hack away without trying to abstract and formalize and, and pull on lessons.
Everything in the academic and, and, and, and animal world we've built up, then you also won't go the right direction. But if you just polish nice abstractions without getting your hands dirty, so it's, it's that unique combination of forcing yourself to do both. We still try to do that with the company. We try to be very hands on with, with, with customers, with new use cases.
Um, not just throw something over the wall. Uh, and that's saying, that's one of many things, but one big thing I took away from that group that I'm, I'm, I'm hoping your friend is, uh, is, is gonna take away and be excited about as well. Incredible, man, this has been so cool, Alex. I appreciate you coming on here and expanding my vision of the power of foundational models.
And what comes next in the marriage of foundational models and ML ops. Hopefully, this has been, uh, absolute pleasure and I want to thank you again for doing this. Well, Demetrius, Avi, thank you so much for the, the awesome conversation. Definitely one of the best I've had because we started right away with, uh, with the hard hitting questions and, and got on the weeds and, and yeah, pulling back on, I think the, the two things I'd, I'd, I'd leave.
I, I think, you know, we talked plenty about it, but at a super high level, really simple things. One, you know, data surprise, surprise, and, and developing data is at the center of everything in ai. And, and it is, you know, even more so the case. Uh, and that'll continue to come to bear when with foundation models coming onto the scene.
And second, you know, there's a bunch of really exciting and really underfilled opportunities, which, you know, we're pursuing, but there's lots of. To kind of bridge these foundation models with real use cases, existing real ML ops infrastructure and, and, and approaches. That's what we're, we're excited to be announcing.
There's lots of stuff to do there though, so I hope other people kind of focus on that because yeah, the generative exploratory use cases are awesome and there's gonna be a boom of creativity there that that's going to be awesome, no doubt. But bridging it to these, these real production use cases is, is a, is a massive white space right now, and so, There's gonna be exciting stuff there and um, it was awesome.
Again, talk about it today. Yes, I love it. Right on dude.