GenAI in Production - Challenges and Trends
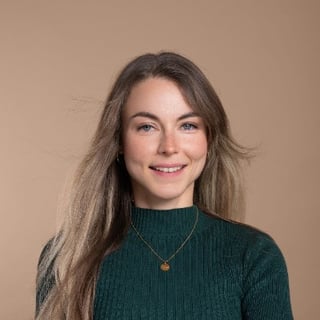
Verena leverages GenAI in natural language to elevate business competitiveness and navigate its transformative impact. Her varied experience in multiple roles and sectors underpins her ability to extract business value from AI, blending deep technical expertise with strong business acumen. Post-graduation, she consulted in Data Science at Deloitte and then advanced her skills in NLP, Deep Learning, and GenAI as a Research Scientist at Alexa team, Amazon. Passionate about gender diversity in tech, she mentors women to thrive in this field.
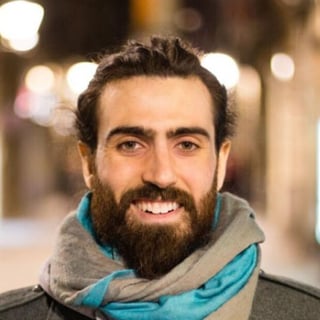
At the moment Demetrios is immersing himself in Machine Learning by interviewing experts from around the world in the weekly MLOps.community meetups. Demetrios is constantly learning and engaging in new activities to get uncomfortable and learn from his mistakes. He tries to bring creativity into every aspect of his life, whether that be analyzing the best paths forward, overcoming obstacles, or building lego houses with his daughter.
The goal of this talk is to provide insights into challenges for Generative AI in production as well as trends aiming to solve some of these challenges. The challenges and trends Verena sees are:
Model size and moving towards a mixture of expert architectures context window - breakthroughs for context lengths from unimodality to multimodality, next step large action models? regulation in the form of the EU AI Act
Verena uses the differences between Gemini 1.0 and Gemini 1.5 to exemplify some of these trends.
Join us at our first in-person conference on June 25 all about AI Quality: https://www.aiqualityconference.com/
Demetrios [00:00:05]: Hold up.
Demetrios [00:00:05]: Wait a minute. We gotta talk real fast because I am so excited about the MlOps community conference that is happening on June 25 in San Francisco. It is our first in-person conference ever. Honestly, I'm shaking in my boots because it's something that I've wanted to do for ages. We've been doing the online version of this, and hopefully I've gained enough of.
Demetrios [00:00:26]: Your trust for you to be able.
Demetrios [00:00:27]: To say that I'm. I know when this guy has a conference, it's going to be quality. Funny enough, we are doing it. The whole theme is about AI quality. I teamed up with my buddy Mo at Colina, who knows a thing or two about AI quality, and we are going to have some of the most impressive speakers that you could think of. I'm not going to list them all here because it would probably take the next two to five minutes, but just know we've got the CTO of cruise coming to give a little keynote. We've got the CEO of you.com coming. We've got chip, we've got Linus.
Demetrios [00:01:07]: We've got the whole crew that you would expect. And I am going to be doing all kinds of extracurricular activities that will be fun and maybe a little bit cringe. You may hear or see me playing the guitar. Just come. It's going to be an awesome time. Would love to have you there. And that is again June 25 in San Francisco. See you all there.
Verena Weber [00:01:32]: Hi everyone. My name is Verena and I work as a self employed, generative AI consultant or also chief AI officer on demand. So basically I help companies going on the AI journey, transforming into an AI centered or AI integrated business. So this starts with architecture, the AI strategy, going over to governance, and also helping them with integration and adoption. And yeah, I usually have my coffee black, so no sugar, no milk, just black.
Demetrios [00:02:15]: ML Ops community we are back from another podcast. I'm your host, Dmitrios. And today, what a conversation with Marina. If there's one thing and one thing only that you can take away from this conversation, it is the cliche of starting with a problem. Don't start with some technology that you shoehorn into figuring out if it will solve your problem. Farina really harped on this and harped on how, when she has been the most successful in her career, it is because she has been problem focused and problem oriented and then gone out to find what solutions there are to solve those problems, as opposed to scouring Twitter or papers and all the newest stuff that comes out in this AI world. And then looking for a problem to link it up with. I know it sounds like it's something that we talk about quite a bit and it is easy to say it, but it is hard to live by it.
Demetrios [00:03:18]: Verena made sure to make it super clear how important it really is. And then a few other pieces that I will give to you without spoiling the whole episode. She talked about how one of the problems that they were having when she was working on the Amazon Alexa program or working at Amazon on Alexa, I guess is another way of putting it. They were having problems when they were retraining the model and they wanted to make sure that if they retrained the model or if they fine tuned the model, that all of the classic utterances, basically Alexa, play your favorite song of the week, you don't want to update the model, and then all of a sudden that doesn't work for half of the user base because that would create a horrible user experience and probably a lot of support tickets. So she recognized this and they went out and they went looking for a solution to this problem. And just so happens that another team at Amazon was working on something very similar. She breaks down how they did that and how they managed to make sure that when updating models, it doesn't just totally skew all the stuff that it's learned in the past. I had a blast talking to her.
Demetrios [00:04:37]: We got into all sorts of cool subjects, even a little bit of a ski trip that she went on, which you will hear about right now in the beginning, and how she has been tackling the entrepreneurship from the slopes. I loved it. And I will say before we jump into the full conversation, thank you to everyone. I am so humbled by the support that we have been getting for the in person conference that we're doing on June 25 in San Francisco. It is incredible. People are talking about flying into San Francisco from around the world. That just like, it melts my heart. I don't know what else to say.
Demetrios [00:05:16]: It is so cool to see the support if you want to get a ticket already, we just released the early bird tickets. It's at AI quality conference.com. We got to give a shout out to the folks at Colena who are helping us and we are partnering with to make this the best conference that you probably will ever go to. I want to do some cool things and I am accepting requests. So if you have ideas on how we can make this a whole lot of fun, I've already thought about setting up different jam rooms potentially. Joe Reese has been contracted to be a dj. I want to have some fun bikes that we can ride around. Right now, I'm literally going into a conversation with a community member, Wes, who owns a coffee farm in Columbia.
Demetrios [00:06:08]: So maybe we'll ship up some coffee beans special for the occasion. There's so much stuff that I'm excited to do. Feel free to reach out to me if you have any suggestions. But June 25, I'll see you all in San Francisco. And thank you so much for the support we've had thus far. Let's get into this conversation with Verada. All right, so you were just skiing in Italy. Where were you skiing?
Verena Weber [00:06:38]: It's a place called Savinha, which is basically on the border to Switzerland. And you could see the Matterhorn, but from the italian side. So that was pretty cool.
Demetrios [00:06:47]: Sounds so incredible. And what were you doing there? Give me the whole breakdown, because I love this idea, and I really want to do something like this.
Verena Weber [00:06:56]: Yeah, so this whole thing was. It was called ski person of influence, which is basically an entrepreneurial ski trip. So you go on a trip with, we were, like, 45 people, so 45 entrepreneurs. And you go skiing, you have a little bit of content sessions in the evening, but, yeah, most of all, you get a lot of chances to speak to other people, what their challenges are, how they're solving them. And, yeah, this whole thing was organized by Daniel Priestley. So he is a very successful entrepreneur who has built a couple of businesses already and now does. Does actually coaching for other entrepreneurs. So maybe you've heard about key person of influence, which is like a quite successful book that he's written.
Verena Weber [00:07:46]: And, yeah, he just organizes this trip because he likes it. It was a super awesome experience, I have to say. Yeah.
Demetrios [00:07:55]: And what were the people that were there working on?
Verena Weber [00:08:00]: It was a pretty mixed group. And so there was, like, one guy who had built a business that built a software for bookkeepers. There were two women who were building a community of bookkeepers, and they're also doing training. So basically teaching them how to make a six figure business out of their bookkeeping services. There were people in the cybersecurity field, people building apps to track your location, which then helps you to submit your tax declaration because you need to show that you're not spending too much time outside the country. It was really cool. Like, he opened this whole event, like, yeah, let's not only ski down black runs, but let's also have black run conversations. So really not just, you know, talking about the superficial things, but really going deep.
Verena Weber [00:09:00]: And what are your current challenges? What are the problems that you're facing. Yeah. And this is really what happened then. During the chalets, we did have a lot of background conversations, as we called it, which was really cool.
Demetrios [00:09:14]: Yeah, yeah. I saw someone doing this with data engineers. They got a bunch of data engineers together, and they said, okay, we're going to have a bit of like a conference, but not really a conference, like a retreat, I think is a better word for it. And we're going to get 40 or 50 data engineers together, handpicked. We're going to go to Switzerland, and we're going to all stay in the same hotel. We'll go skiing during the day, and in the afternoon, evening, we'll be hanging out, but we'll also be sharing stuff about what we're doing and what we've learned over the years. And we have a few speakers, et cetera, et cetera. Friend of the pod, Joe Reese, was there, and that's how I heard about it.
Demetrios [00:09:56]: And I was like, oh, my God, how do I get an invite to that? That sounds like a blast. And then I started thinking about it, and I was thinking we should do something like that. And potentially, since ski season's over and I don't have patience to wait until next ski season, I was thinking about, oh, we can go to Portugal and do it. But surfing instead of skiing.
Verena Weber [00:10:18]: Yeah, yeah. Actually, everyone was really, really energized after the wind, and we started talking, oh, we should do it in summer again. We don't want to wait until next year when Daniel does the ski trip again, but let's have something in summer. Yeah. And there were actually a couple of people who do a similar thing for their audience, for their clients, I bet. Yeah. It's a really nice idea.
Demetrios [00:10:40]: It feels like it could be a really cool way to bond with people and socialize and then learn a ton that you wouldn't necessarily learn from a talk at a conference. You get to go deeper.
Verena Weber [00:10:54]: Exactly. It's not only about what you learn conceptually and intellectually, but it's also this kind of energy that you take with you, this inspiration, this sense of possibilities that changes how you feel about yourself, how you see the world. And I think that is probably also something you don't take away from conference.
Demetrios [00:11:17]: Yeah. Yeah, for sure. So you've had quite an awesome background. In general, I want to get into what you're doing now with coaching and with your AI consulting stuff. But first, we should probably start with what you were doing over the past, like, 510 years.
Verena Weber [00:11:37]: Well, I mean, I started originally with statistics. So really classical master in statistics. So back then, actually, there wasn't a lot of data science machine learning programs happening yet. But luckily, I did have some courses in data science and machine learning during my master, and then kind of enjoyed that much more because it was more trial and error, less based on assumptions, and then decided to just stay with that field. And back at the time, so I was doing my master's degree in Berlin already and really liked the city, didn't want to move anywhere else. So I was trying to find a data science role in Berlin. But at the time, you wouldn't believe that now. But there was hardly any company hiring data scientists, not even Salando.
Verena Weber [00:12:27]: Salando was just hiring in Dublin, so I didn't want to move to Dublin. I can understand why, exactly.
Demetrios [00:12:34]: No. Hate to all the Dubliners out there, but Berlin is an awesome city, and I wouldn't want to leave if I was living there either.
Verena Weber [00:12:40]: Yeah. And also the weather in Dublin is harsher than it is in Berlin. And Berlin can be harsh already. Yeah, that is really where my limit is. Yeah. But then, luckily, I found a data scientist role at Deloitte. So basically, consulting company that at that time had a specialized unit for data engineering and data science, which was cool because you could try a lot of different things. So I tried everything from time series anomaly detection, NLP, computer vision, everything.
Verena Weber [00:13:18]: Yeah. But then, I mean, at the time, also, data science was really kind of more things that people would try if they were very innovative, but it was not at the center of attention as it is now. So, basically, it was a lot of proof of concept stuff that we did, but not that much moving into production or going further than that. So then I was looking for a job where I could go over this proof of concept stage, spend a little bit of time at Edgar Digital, then went to mobility, which was part of eBay at the time, because they then had way more data. Because I realized that the more classical and older companies didn't have that much data yet where you could really work with. They were, what, a paradigm or a.
Demetrios [00:14:07]: Paradox, I should say.
Verena Weber [00:14:09]: They were kind of trying, you know, because it's always easier if you build something from the ground up, right? And then you can build this data driven thing right into the company. That's always easier compared to when you have to make this transformation.
Demetrios [00:14:24]: You know, what's funny about that, which I have been thinking a lot about lately, is that you have the companies who started, so you have those typical companies that you're talking about in 2018. They weren't considered advanced, and they were still trying to go through that digital transformation journey, and it was very slow and they weren't really getting on the bandwagon. But then you have the tech forward companies that were doing that, and they had to make a lot of design decisions in 2018 and 2019 on how they were going to build their stack, which today feels like, oh, they have to support legacy tools and they have to either get off of that legacy or they, if they're contributing to open source, they have to be, like, the main contributor to that open source project because they made that decision in 2018 when that's all there was. But now in 2024, there's much easier ways of doing things. And so if you were one of those companies that in the 2010s was very slow to move and now you're finally getting on board with it, you can potentially have a much easier avenue to getting things under production. And the easiest example of this is just like, look at what LLMs did, and they just exploded and got everyone into the AI sphere. Super easy. But I'm even thinking about all the infrastructure that you need and how that decision, like, I always come back to this conversation that I had with a guy that works at Pinterest and he was saying, yeah, in 2016, we started our ML journey, and so we had to create infrastructure around machine learning in 2016, which we are continuously updating and we are continuously trying to see what's the ROI.
Demetrios [00:16:23]: If we shift off of this tool, do we need to continue supporting it and all of that stuff? But I digress. That was a little bit of a tangent, so I didn't want to take you off course there.
Verena Weber [00:16:35]: No, absolutely. And I mean, that actually brings me to my next step. So I spent, before I became a self employed consultant, I spent almost four years as a research scientist at Amazon Alexa and for a natural language processing device, or, I mean, virtual assistant, Alexa is actually pretty old right there. Like eight years or something by now, I think. So that is super old if you think about what happened alone in the last two years in that space. And also there, right when they started, the whole field was in a different state. So one of their main challenges now is actually working through all that legacy and being able to adopt all these new technologies that also keep changing at such a rapid pace.
Demetrios [00:17:26]: That's so true. Yeah. And were you all using, I imagine it was all using Sagemaker for this, or is it homegrown?
Verena Weber [00:17:38]: Yeah, it's a lot of homegrown tools that we were using. I mean, of course it does rely on AWS at the end, but I didn't use like this normal sagemaker interface that other people are familiar with. So Amazon actually likes building their own tools. And within Alexa, yeah, I think the only tool that everyone would know that we used was slack, but also only after a while. So when I joined, Amazon still had their own tool, which was called Chine, but really sucked. So I eventually removed to slack.
Demetrios [00:18:12]: So tell me more about Amazon and working there and what were some of the key challenges you were working on?
Verena Weber [00:18:18]: Yeah, so I was a research scientist in the team based in Berlin. So we were responsible for natural language understanding models for German, French, and then later also for English and Great Britain. So our work was basically divided between two parts. So one part was the really maintenance, operational stuff where you had to retrain models, deploy new releases, updates of the model, and sometimes also stuff like making sure the training data is all right. So really more things that were on the maintenance side. But then on the other hand, we really had also research projects where we were trying to find ways how we could improve the model or improve the processes around how we work with the model and basically automating processes around model deployment or improving the model itself. And that was pretty cool because how that was different from previous data science rules that I had was, it has much more, it had much more of an academic component. So we were really reading the newspapers and then also going to academic conferences.
Verena Weber [00:19:39]: And part of our goals was also to publish our own papers, which I really enjoyed.
Demetrios [00:19:44]: Nice.
Verena Weber [00:19:45]: And of course, yeah, I got to work with really cutting edge technologies or all the deep learning stuff that usually in other companies you of course, don't work with because it doesn't make sense for them. But since in Alexa, machine learning is the core product, right. Yeah. You really invest a lot of time and effort into improving that product. So that was cool.
Demetrios [00:20:10]: What were some of the papers that you read? And then you felt like, oh, let's try this and see if it will make a difference. Do you remember?
Verena Weber [00:20:18]: I mean, usually it worked the other way around. So usually we would start looking at the problems. So what are the problems that we really need to solve that are holding either our spec or are holding or really negatively impacting the customer experience? So, I mean, one of the approaches at Amazon is you work backwards from the customer, right. And you always work backwards from the problem. So usually we define the problem and then you go looking for the papers. It hardly was the case that we looked at the paper and then thought, okay, this is also a problem that we could solve. But actually, there was one example. Indeed, it was a paper that was published by AWS.
Verena Weber [00:21:01]: So one of our colleagues, basically, and they looked. So the paper was called positive congruent training, and it looked into how you can minimize the negative flips between updates. And a negative flip is basically. So if the previous model interpreted a training instance correctly, but then the new model interprets it incorrectly, you have a negative flip, something you don't want. And that is particularly the case if you have a model that's running in production because you want to keep the user experience constant. And this is especially important for Alexa, if there's important requests or utterances, as we call it, and then you retrain the model, and all of a sudden, that doesn't work. It's pretty annoying for the user and also for us, because then we get a high severity ticket. So we had a very complicated process of making sure that after every retraining, the model still works on these very frequent utterances.
Verena Weber [00:22:05]: So basically, we applied this technique of adding another term in the loss function to prevent these negative flips from happening during the model training and not after.
Demetrios [00:22:21]: It feels like that is still so relevant today, because how many times have we heard people talk about how some random OpenAI update happens, and now my prompts don't work as well?
Verena Weber [00:22:34]: Yeah, no.
Demetrios [00:22:36]: And that potentially could be one of the things that might help in that case.
Verena Weber [00:22:41]: Yeah. And it's also not an easy one to solve. Right. I mean, even with this technique, you can prevent some negative flips, but it doesn't mean you prevent them all.
Demetrios [00:22:52]: As you were talking about that, I wonder if you had some kind of understanding of the data of, like, the distribution of what people asked Alexa. And there's got to be a ton of common questions that people are asking. Right. And then it's almost, I imagine there's like a bell curve. And did you, did you look into that and make sure, okay, the majority of questions, we can never let those go awry. And all the other stuff, let's try to minimize that. But if it's that bell curve, I want to make sure that all these really important questions that people are asking continuously, we never get that. Like, we have to really test to make sure that isn't going haywire.
Verena Weber [00:23:40]: Yeah, absolutely. I mean, there is really, like, a set of utterances or requests that are super frequent that we really also had to make sure work perfectly all the time. And then there's this very long tail where you have a lot of things that are very infrequent but still, you have a lot of these continuing on.
Demetrios [00:24:06]: The theme of just like, what you were doing in your day to day while you were working on Alexa. Are there any other challenges that you went through as you were working on that? And you were like, ooh, this is a really novel way of solving this problem that we encountered.
Verena Weber [00:24:23]: I mean, there were a couple of things, right. But I think, I don't know if it's necessarily totally novel, but it was also very specific setup. But I think one project that I really enjoyed, and that was really cool, was kind of making the model more robust to small changes in the input utterance. So what we saw is that even small changes, like the user saying please also could actually make a change in prediction. Of course, not something you want. Right. So what we did, and there's also a paper about it, so that, that's why I can talk about it, we trained a t five model on these utterances with a small variation and basically had that generate more of such variations and then use that in our training data to make the model more robust against these small variations. And that was pretty cool.
Verena Weber [00:25:25]: Yeah.
Demetrios [00:25:27]: Which again, now is so obvious for anyone who has spent five minutes prompting and trying to understand how to prompt better or what words are going to be taken differently when prompting. So if you prompted, then you know that by saying please or by throwing in, changing up the word order, it's going to drastically, sometimes drastically, sometimes not so drastically change the output. And it feels like you were discovering that a little bit before the rest of the world. And so the way that you solve that was by creating synthetic data with this t five model and then using it as more robust data for the training. Is that what I understand?
Verena Weber [00:26:15]: Yes, exactly. Yeah. And also part of it for testing. Yeah. To see if we actually make it more robust.
Demetrios [00:26:22]: It seems like you were doing that for training data, but have you also thought about doing it for training data, for fine tuning training data?
Verena Weber [00:26:30]: Oh, yeah. I mean, if I speak about training data, it is fine tuning. It's not pre training. Yeah, sorry, I forgot to mention.
Demetrios [00:26:39]: Okay.
Verena Weber [00:26:40]: Yeah. We're. I mean, because usually what, what we were working on were this, was this fine tuning step, because you don't do pre training all the time. Right. You do that once, and then every update is just a fine tuning step that you do.
Demetrios [00:26:53]: So you were constantly just fine tuning the last version of the model?
Verena Weber [00:26:58]: Yeah, yeah.
Demetrios [00:27:00]: Interesting. And don't you get into a little bit of hot water with, like, one of my favorite words in this space, like catastrophic forgetting.
Verena Weber [00:27:11]: Yeah, I think for Alexa, it's fine, because you have so much training data, and it's not like you're changing, you know? I mean, everything that a model still needs to know is still in the training data, in the fine tuning set, and it's there to a large extent. So we didn't have that problem that much longer.
Demetrios [00:27:33]: Oh, that's awesome. Okay, that's good to know. And so then the other piece, last part about working on Alexa, and then I want to get into some of these different topics that I know you brought up. It was around the training data and ensuring the quality of that. And so you had the synthetic data. Were you manually checking that out to make sure that it was okay? And if it's all audio data, it feels like it's much more time consuming to label it and quality check it than if it's text, right? Or is that just my false intuition?
Verena Weber [00:28:16]: No, you're right, probably. I mean, I'm not a specialist in analyzing audio data, but I would assume so.
Demetrios [00:28:21]: All right, real quick, some words from our sponsor, Zeliz, and then we'll be right back into the program. Are you struggling with building your retrieval augmented generation, aka rag applications? You are not alone. We all know tracking unstructured data conversion and retrieval is no small feat. But there's a solution available now introducing Zliz cloud pipelines from the team behind the open source vector database, Milvis. This robust tool transforms unstructured data into searchable vectors in an easy manner. It's designed for performance. Zliz cloud platforms pipelines Zliz cloud pipelines also offer scalability and reliability. They simplify your workflow, eliminating the need for complex customizations or infrastructure changes.
Demetrios [00:29:16]: Elevate your rag with high quality vector pipelines. Try Zilliz cloud pipelines for [email protected]. You can find the link in the description. Let's get back into this podcast.
Verena Weber [00:29:28]: But yeah, I mean, the way Alexa worked when I was there, I mean, I don't know if they changed the system in the meantime. So I want to be clear about how it worked when I was there. So you basically transcribed the audio into text, and then you sent the text to the natural language understanding model. So all our input that we got was text, so we could basically just analyze the text. And to your other point, about whether we check the quality of the generated data. Yes, we did. And we did actually some kind of exploratory analysis, bot checking and these kind of things. And then based on that, we derived some heuristics and methodologies to clean up the generated training data because you definitely couldn't use everything that came out of the t five model, but we had to put some fidgering mechanisms in place.
Demetrios [00:30:25]: So this feels like a good segue into one of these themes that we wanted to talk about, which was, like, shifting from unimodal to multimodal. And when you said, like, oh, yeah, we would transcribe the utterances into text and then send the text to the model, I instinctively was like, yeah, of course, that makes total sense. I should have known that already. I feel a little bit like an idiot since I didn't know. But.
Verena Weber [00:30:55]: No, actually not. I mean, there were also. Yeah, I mean, that's. That's just, I think, yeah, that's the fact that Alexa is really old. Right. That's. That's why the system is built that way. But there were projects that.
Verena Weber [00:31:09]: Yeah. Or there were also ideas where we thought, okay, maybe we can just make this audio to intent or domain. Yeah, yeah, but that's. That's just. But it's an old system that takes time to change. And then, of course, it also has its advantages if you break it down in steps. Right.
Demetrios [00:31:29]: Yeah, yeah. The pros and cons and the trade offs for that. And my mind is racing on what trade offs you would look at in those cases. And at the end of the day, it probably comes down to how accurate it is and how fast it is.
Verena Weber [00:31:46]: Yeah. And also how easy to maintain it is. Right. Yeah. Because I think this maintenance part is a little bit easier if you break it down into steps. And also if something goes wrong. Right. It's easier to fix it.
Demetrios [00:32:06]: Yeah. The debug ability. So as you're thinking about the shift from unimodal to motimoto, what are some things that come to your mind?
Verena Weber [00:32:18]: Yeah, I mean, it's just been, I think, groundbreaking. Right. That now you can just input almost everything you can think of into these large language models, and it's just making the application space so much bigger. I mean, just creating a marketing video or putting in some lines of text, you get a video. I mean, that's amazing. Right. It's just crazy to see that as we just spoke about how things used to be divided in step by step things, and you had these models that were specialized on one modality, and we saw this happening with large language models too. Right.
Verena Weber [00:32:59]: Where they were at first only focused on text, but now we're really, with Gemini, you can have video, audio, text, so many different modalities. And it's just amazing because you can basically use it for everything for a much wider variety of tasks.
Demetrios [00:33:20]: Yeah. And also you can get more clear on the prompts, too, or get more specific. I think it's, if you can give a photo and of the text, it's much more accurate in my eyes.
Verena Weber [00:33:36]: Yeah, yeah, maybe. Yeah. And then I think, yeah, one example that I saw was pretty cool, where you basically upload a photo of.
Demetrios [00:33:48]: A.
Verena Weber [00:33:49]: Scene in a book, and then the model gets you the right pages from the book, where you upload basically a photo, or you just put in how you solve a problem, a physics problem, and then the model says, oh, this is where you went wrong, and this is how you need to do it. So I think also the applications in how we can use this technology in education and self learning has really expanded through this multimodality.
Demetrios [00:34:17]: So when you're working with companies and you're seeing these different ideas, I can imagine that you, if you're anything like me, you're like, wow, we could do this and that. And there's this super cool cutting edge way of utilizing the newest model when in reality that may be overengineering it. And what you want to do is just get some kind of MVP out that doesn't need to use all the newest cutting edge.
Verena Weber [00:34:45]: Yeah, absolutely. I mean, I'm a big proponent of not using the cutting edge stuff. If you're just starting out, I would never recommend anyone who is just getting started on their AI journey to immediately start with the news stuff unless they have a use case that absolutely requires it. Right. But I am, and I actually just recently gave a talk at a meetup where I really pointed out that you should not start thinking about the solution and then figure out which problem you can solve with the solution. But you need to start with the problem, define the problem clearly, and then find solutions for that problem. And I always encourage people to start with a very simple solution. And for every problem, you can find a very simple solution.
Verena Weber [00:35:38]: It just maybe doesn't perform as well, but you can find a simple solution, and then from there go to the more complex ones and always benchmark it against a simple one and then compare. What does it cost you to go to that more sophisticated solution, and what are the performance gains that you get? And oftentimes this increase in performance does not justify the cost. And it's also not just about performance. Also, like, do you have the capacity to maintain all that stuff? Right, because it becomes way more complex to update systems once you have a deep learning model. Right. So there's a lot of factors that you actually need to consider when you pick that solution, not just. Yeah. Is it really cool? Is it really fancy? Do we want to try it out?
Demetrios [00:36:29]: Yeah. The classic engineering.
Verena Weber [00:36:32]: Exactly. I know it seems so obvious and so trivial. Right? It seems like, oh, we've been hearing this a lot, but yet I still see people getting carried away with it.
Demetrios [00:36:45]: Yeah, you're echoing so much of what past guest was talking about. We basically spent 30 minutes talking about that exact same thing. Like, hey, just figure out what your problem is and get some good metrics around that and then try and push those metrics as far as they can go with the most simple solution. Because as. And this was Sam that came on, and Sam said, I've never heard an engineer say that. I wish I would have made the system more complex.
Verena Weber [00:37:16]: Exactly. Yeah. And, yeah, there's so much you can achieve with simple solutions already. And even, you know, I mean, Chechi BT, we all know that now, but there were models before Chechi BT, right. That are already, you know, they can solve a lot of problems that the majority of businesses have right now. Right. And also, I think people sometimes forget what chipt is actually trained to do. Right.
Verena Weber [00:37:44]: It's trained for conversational language understanding. So if your problem is not about conversational language understanding, if it's just a simple classification problem, then start with a classification algorithm and not. I mean, of course chat CPT can give you an answer and it might even be correct, but it's over engineering the problem. And, okay, if you're just doing 100 classifications every five months, maybe it doesn't matter, but once you want to scale and once you have more traffic on that model, it's way too expensive. But, yeah, it doesn't scale and it doesn't make sense for. That's my opinion. I don't think it makes sense to use ChetGPt for classification.
Demetrios [00:38:25]: Doesn't make any sense. That's so true.
Verena Weber [00:38:26]: Even though I heard people doing it, but I still think it doesn't make sense.
Demetrios [00:38:31]: Yeah, it's one of those ones where you see, I remember we had the ball from Angellist. Come on. And he was talking about how they spent six months putting together a classification model, and in two weeks after chat GPT came out, they started using that, and they were benchmarking it against their homegrown model, and chat GPT was just beating it all over the place. And they were like, oh, no. So in that case, it's a little bit like, hmm, where is the. Where's the overengineering happening. It's good that they were honest enough. And what.
Demetrios [00:39:14]: What's the word I'm looking for? Humble enough to recognize. Okay, this model that we worked on for six months, we can throw it out. And now chat GPT is much better. But I think for every one of those stories, you've probably got another ten stories of what you're talking about where chat Chibi may be overkill.
Verena Weber [00:39:32]: Yeah. And then also the question is, what do you compare it to? Right? I mean, if they build like a model based on TF, IDF and maybe some manually engineered features, and then compare it to Chet GPT, maybe not the best comparison. Right. I think what you should do then is take a bird model, get a bird embedding, and then build a classification layer on top and compare that to ChedGPT, where you then already move away from manually engineered features. But you use the technology of deep learning. Right? You get these embeddings, you use a lot like Transformer model or transformer based model, and then use that for your classification, because otherwise you're also a bit comparing similar rules to super sophisticated AI model, which, yeah, maybe is. Of course, they're not comparable in that sense.
Demetrios [00:40:24]: Whereas all those tools that are highlighting how they're using, Bert, you don't see those out there. Everybody's highlighting how they're using chat GPT and AI. It would be funny for someone to zig where everybody else is zagging and safe. We're using the non state of the art.
Verena Weber [00:40:42]: Yeah. And I mean, okay, it also depends a little bit what are the capabilities that you have in your company. Right? Of course, chat GPT is super easy to use. Everyone can build a quick proof of concept with that, and that is great. And if you don't have a lot of traffic on the model, okay, maybe it's a viable solution. But if you really need to build something that needs to scale, then probably you need to look into other options.
Demetrios [00:41:12]: Yeah. Yeah, 100%. So this has been awesome. Varenna, I really appreciate you coming on here and talking to me about all the good stuff that you've been up to. I want to mention to everyone that I have been reading your newsletter diligently, and I appreciate you doing it. We'll leave a link in the description for anyone else that wants to get smarter by getting a little bit of you in their inbox every week. I also appreciate what you are doing when it comes to coaching women. Can we talk about that for a minute?
Verena Weber [00:41:46]: Yes, absolutely. I'd love to. Yeah. So that is a topic that's dear to my heart. And basically it all started when I read that more than 50% of women leave their tech career at the midpoint of their career. And part of that is because they feel like they need to prove themselves more of their gender because of their gender, because they feel like they're not giving the right opportunities to grow. And they basically just give up because they don't see a future in the industry. And of course, women are totally underrepresented in tech roles, and we need more women in tech roles to really make sure that the technology that is being developed is.
Verena Weber [00:42:32]: Yeah. Is more representative of the whole population. So what I do is I coach women to basically take control of the things that they can take control of, because we can change the industry from being male dominated to being balanced immediately. But what we can do is we can adopt certain strategies to work on our confidence, be more visible, be more strategic about our career growth, ask for the things that we want, go after the growth opportunities, deal with the stress and overwhelm that sometimes come with these challenges, and just develop routines that support us in thriving in this environment, even though it comes with certain challenges.
Demetrios [00:43:22]: Yeah. The way that we met, we should probably mention that we were on a panel together in one of the ML ops community meetups in Berlin. And it was a panel all about having more underrepresented voices in tech, I think, and women in tech. It was in collaboration with the women in tech or women in data science in Berlin?
Verena Weber [00:43:46]: Girls in tech, I think.
Demetrios [00:43:47]: Girls in tech. That's it. And so I was on that panel, which my wife told me was a very stupid idea right before I left to go to Berlin. She was like, you are about to say something that will get you cancelled. Hopefully they're not recording it. But luckily I got out of it unscathed. And I really appreciated a lot of these ideas that you were sharing, especially around this, what you just said. Like, there's no reason we should have people fleeing from, especially like, females fleeing from tech, because if anything, we need a lot more in tech to counterbalance all of.
Demetrios [00:44:27]: I think right now, there's like, I've been seeing, there's a little bit more female audience of this podcast, which I love seeing, but we could say probably like two or three listeners are female.
Verena Weber [00:44:39]: Okay. Yeah. Well, I definitely appreciated that you were on the panel because it's not something that, you know, we can solve on our own. And we need allies. We need, you know, and also, this newsletter is not directed at women only. It's really about every man that's interested in what are the challenges that women face in these environments, and they're interested to support them. Right. And I think it was really cool that you were part of that panel.
Verena Weber [00:45:08]: Yeah.
Demetrios [00:45:08]: I always feel a little bit awkward when we're organizing the conferences and I'm trying to get more female speakers. We're just speakers from underrepresented groups. It doesn't have to be female. Yeah, but having to, like, specifically say that to people, I never really figured out how to say it. And I always kind of felt very weird being like, uh, no, we can't have you talk because you're not from underrepresented groups. And people, like, the men would get, like, why are you biasing against men? And it's like, no, we just want to have space. And I'm. I think that for me, at least, there's the benchmark that I'm going for in all the events that we're doing, is trying at least to get 50%.
Demetrios [00:45:57]: 50% feels like that's okay. Wow. Yeah, it's great. But it's definitely hard. But it also is, like, it's not outrageous, you know?
Verena Weber [00:46:10]: I mean, given that we only have, like, 20% of women in tech roles, it is quite hard to meet that mark, so. And I think it's also quite unusual to see that.
Demetrios [00:46:21]: Yeah, but people appreciate it. They definitely do. Like, people will write on the feedback forms that we have, and they will say, like, it's cool to see so much diversity.
Verena Weber [00:46:31]: That's nice.
Demetrios [00:46:32]: And so even though you don't necessarily, it's not like, I'm not going to be there in all the messaging and the marketing and be like, we get 50% underrepresented groups to speak. People still notice, and that's cool. It makes me feel good because it's a lot of work to do that.
Verena Weber [00:46:50]: And honestly, I think it's also one way to making females more comfortable speaking at these events, because it's always weird when you feel like you're the only female person in the room. So it's really nice if you go to eventually to an event where this is different. Yeah.
Demetrios [00:47:10]: The truth is, and I firmly believe this, that there are so many incredible people that are doing such cool stuff from these underrepresented groups that it's almost like that's my job, is to go out there and find them. It may be a little bit harder, but that's why we call it work. That's what I gotta do, so.
Verena Weber [00:47:30]: Yeah. And it's cool that you do that, because I tend to find that people from underrepresented groups are just a little bit more quiet. They're shy. They don't necessarily feel safe in these environments. And that is why they don't feel comfortable to be visible. They don't feel comfortable going after these speaking opportunities. But then when they get asked and you even give them this nice environment of 50 50, then it can be a good entry point for them. And then maybe they find out, oh, it's not that bad after all.
Verena Weber [00:48:04]: Maybe, yeah.
Demetrios [00:48:06]: Yeah.
Verena Weber [00:48:06]: I should do this more often.
Demetrios [00:48:07]: Let me just clarify. I try for 50 50. I'm not gonna say I hit it all the time, because now you're gonna hold me to that.
Verena Weber [00:48:14]: I won't.
Demetrios [00:48:15]: I really try really hard, but the. I can't hit it always.
Verena Weber [00:48:20]: I get it.
Demetrios [00:48:21]: I get it. Always. Yeah, but it's not. It's not impossible. And I definitely strive for it because I think it's important. So, Brenda, this has been awesome. I appreciate you coming on here and chatting with me about all this. I had a great conversation.
Verena Weber [00:48:34]: Me too. Thanks for having me. This was really fun.