How Sama is Improving ML Models to Make AVs Safer
speakers
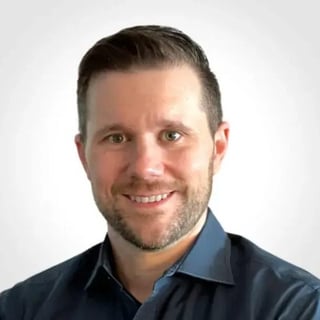
Duncan Curtis is the SVP of Product at Sama, a leader in de-risking ML models, delivering best-in-class data annotation solutions with our enterprise-strength, experience & expertise, and ethical AI approach. To this leadership role, he brings 4 years of Autonomous Vehicle experience as the Head of Product at Zoox (now part of Amazon) and VP of Product at Aptiv, and 4 years of AI experience as a product manager at Google where he delighted the +1B daily active users of the Play Store and Play Games.
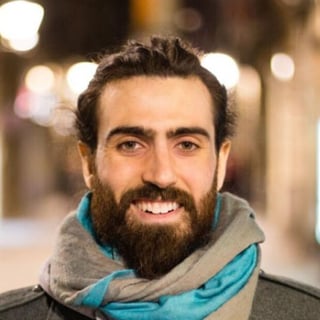
At the moment Demetrios is immersing himself in Machine Learning by interviewing experts from around the world in the weekly MLOps.community meetups. Demetrios is constantly learning and engaging in new activities to get uncomfortable and learn from his mistakes. He tries to bring creativity into every aspect of his life, whether that be analyzing the best paths forward, overcoming obstacles, or building lego houses with his daughter.
SUMMARY
Between Uber’s partnership with NVIDIA and speculation around the U.S.'s President Donald Trump enacting policies that allow fully autonomous vehicles, it’s more important than ever to ensure the accuracy of machine learning models. Yet, the public’s confidence in AVs is shaky due to scary accidents caused by gaps in the tech that Sama is looking to fill.
As one of the industry’s top leaders, Duncan Curtis, SVP of Product and Technology at Sama, would be delighted to share how we can improve the accuracy, speed, and cost-efficiency of ML algorithms for AVs. Sama’s machine learning technologies minimize the risk of model failure and lower the total cost of ownership for car manufacturers including Ford, BMW, and GM, as well as four of the five top OEMs and their Tier 1 suppliers. This is especially timely as Tesla is under investigation for crashes due to its Smart Summon feature and Waymo recently had a passenger trapped in one of its driverless taxis.
TRANSCRIPT
Duncan Curtis [00:00:00]: Duncan Curtis, the SVP of Product and Technology here at Sama. And the way I like my coffee is a triple espresso with three shots of caramel. Boom.
Demetrios [00:00:09]: We are back for another MLOps community podcast. I'm your host, Demetrios. And we're talking data again. What do you know, it's 2025 and we're still talking about data. We just can't not talk about it when it comes to AI and ML. And Duncan is knee deep in the data world. He talks to us a lot about how to think through your data, your data collection, what that even looks like. Things that can be gotchas when it comes to your data and your data strategy and robustness.
Demetrios [00:00:50]: Shit, I'm saying a lot of buzzwords. Let's just get into this conversation. What are you working on these days, man? Because you've got so much cool stuff that you've been doing. Give me a breakdown of your day in, day out.
Duncan Curtis [00:01:09]: Yeah, so we're really focused on empowering AI across some of the largest enterprises in the world. Whether it's in autonomous driving, whether it's in generative AI. The latest model releases we've been working through on those. And so what I am constantly thinking about is we've got our own workforce, a full time workforce and platform. And so I'm just living and breathing the how can I both anticipate what's coming next, but then how can we also really get the benefit of the human in the loop? And so the reason I say that is that, you know, so I've seen the evolution of data annotation for AI really, you know, the whole core of AI being what you put in is what it learns. It's kind of like a kid where if you only teach them like sci fi books, they only know about sci fi topics. And if you never talk about economics or something, they're never going to know about it. And it started like a long time ago where you've got things like, hey, we want to know if there's a dog or a cat in the picture.
Duncan Curtis [00:02:14]: And so you'd go through, you know, are 10,000 images and that's a dog, that's a cat, that's actually a buffalo. That's not, not what you want, what you're, you're interested in. And the thing that's interesting is especially as the annotation can get more and more complex. So if I think of some of the stuff we're doing now in autonomous vehicles, where you've got a lidar capturing Millions and millions of points every second or every frame. You've got multiple cameras. You've got radars and ultrasonics, and you're getting someone to draw and capture the information that's within that and really codify it for an AI to understand. The thing is, the human intelligence in the loop is. And the human in the loop.
Duncan Curtis [00:02:56]: But I like the eye to also stand for the intelligence. It's not about how do you draw. Well, even though that is a skill, the actual intelligence that you're capturing is, oh, well, I recognize this about the scene. Oh, I realize that's a car. That car, even though it went behind a truck, is the same car. And I know that because I can see the little, you know, unicorn sticker on the bumper. Or I can. You know, there's all these areas where I'm trying to look at how do we marry up the tooling and technology side that goes with the people side for capturing what's important for AI and trying to minimize the other work that can easily get lumped into it.
Duncan Curtis [00:03:36]: So, you know, even things like, hey, do we allow the AI to. Or how do we get the AI to say, take the first pass on annotating and guessing? You know, in most cases, it's right. But, you know, not. Not. Certainly not 100%. How do you, you know, oh, that car with the unicorn bumper sticker. It doesn't think about the unicorn bumper sticker and it goes, hey, that's car one, and now it's car two, since it drove behind a truck and I didn't see it for a little while, but it's a lot faster if that car is already drawn. And you can be like, oh, hey, here's where you messed up it.
Duncan Curtis [00:04:06]: That's actually still car number one. That's the same car. And so that's a way that we can enable that intelligence to be captured at a fraction of the time. Yeah.
Demetrios [00:04:18]: Now, the idea of where to plug in the human is always fascinating to me. And I'm sure you think at lengths about that because it's not like you can just haphazardly throw in a human step in the pipeline, since it can get very expensive. And that probably is arguably the most expensive part of the pipeline. Right.
Duncan Curtis [00:04:42]: It is in for the vast majority of industries, it's the most expensive thing. Some specialized industries have more expense in the data collection, like if there's very expensive machinery or other things involved. But you're right, like, just in general, it's very much the most expensive part. Now, when you do talk about, say let's say like large language models that the new most expensive part is the cloud training. Just because the data size they're using is huge. But they're all also not having humans look at it. And that's why we still see problems with like ChatGPT and Gemini where you, you're using the Internet with no, with no human filter, which is.
Demetrios [00:05:19]: Yeah.
Duncan Curtis [00:05:21]: Exactly. Oh yeah, yeah, definitely. Yeah. We've seen some definite public stories around what, what sort of happened on that side. But yeah, so when we think about where the human goes in, there's like a few places and you're right. How do I do it in the minimal way to get the maximum benefit to the AI model where we can capture the most intelligence. And so there's even steps before say like labeling in terms of how do we pick the right data? Because if I go like driving and for a really long time, let's say like hours and hours or you think about your commute to work. If you, back in the days, pre pandemic, when everyone was driving to and from work every day of that drive, probably only 10 seconds was actually relevant.
Duncan Curtis [00:06:08]: Most of it is you're in your lane, there's a car in front of you, car beside you, nothing's happening. But when someone cuts you off, maybe something unexpected happens. That's actually that, like, that's the, that's the really interesting part. And so how do you find those pieces in, in those, in that data so that instead of labeling an hour to an hour to get five seconds of use, you're, you're actually only grabbing that five seconds, maybe, let's call it 10, maybe a little bit around it and then you can do that again and again to get that maximum data usage, data value for the AI. And so you can use, not just in that labeling part, but it's also in what we call curation where you take a larger data set and you want to look at it, you want to look at things that are like how do I find things that are interesting? How do I find a good distribution of classes? Because, and it's not just classes, but events. So things like we've seen, you know, in the, in not just self driving, but across, across AI tech for years there's been a lot of bias in the data. You know, back in the day of the old, back from my video game career when we were building, we were taking Fruit Ninja from the phone and putting it on, on one of the consoles they had, they had a sensor where you'd Go in front of it and do your little fruit slicing with your hands. It couldn't pick up some of the people in the office who were of color.
Duncan Curtis [00:07:32]: Oh, wow. Why? Because it turned out that the team who had built it had been a bunch of, you know, white dudes and they'd use themselves as data collection to train it on. And so it performed poorly both on children and on people of color. And so it was like that. That I'm just trying to say it can be exacerbated in many different use cases. Obviously a lot of people like to talk about the current ones with autonomous driving, but this is not a new problem. So we can help in curation where you're looking at, you know, do you have. And let's talk about self driving cars or autonomous vehicles.
Duncan Curtis [00:08:02]: Do you have enough buses and cars and what about bikes and what about skateboarders? Do you. But then you can also dig in as long as you're adding the metadata or have a way to generate it. Do we have enough representation across different attributes? Height, skin tone, a lot of different things that can make sure that you're, you're, you're going to see that. And also goes to not just those, but things like weather conditions, like bright sunny days, sun glare, snow, nighttime, rain. There's a lot of different attributes that can really make sure that you want to get good distribution to have as best of a chance that you've caught the edge case before you see it in production.
Demetrios [00:08:42]: Yeah. Finding all of the anomalies is always the fun part, I would imagine. But then this robust data set is really where the work comes in. And trying to think through all of the different pieces too.
Duncan Curtis [00:08:58]: There is. Yeah. And, and it's a mix as well, because you, it's not just raw collected data, real world data. You've also got synthetic data as part of the mix. I know. You know, for example, you know, there's a reason why Waymo has been around for, you know, 13, 14 years and is while they have deployed and have now, I think they just said they passed 50 million rider driverless miles or rider only miles, which is an amazing milestone there. They haven't stopped and they're not deployed everywhere. Because the amount of edge cases and simulation you want to do is huge.
Duncan Curtis [00:09:36]: Because. Yep. I mean.
Demetrios [00:09:38]: Yeah, and I remember seeing some. You always catch those videos that pop up on and do their rounds on the Internet. And one was where there was a horse in carriage and it wasn't clear because the training data was never with a horse and carriage. So again, that's like. You'll get these anomalies pop up and the model has no idea what to classify it as.
Duncan Curtis [00:10:06]: I. That's a great example. Yeah, you would say, you know, and you can look back at your training data. Is like, what representation of those classes do we have? Like, is that actually something like, have we seen four horses? Maybe we've seen a thousand horses, but how many carriages or horses and carriages together have we seen? One that I like is a relatively simple example, but it can show that in another way. Another aspect is, go back a few years and we've got. Even if you've got good distribution of classes. Let's take skateboarders, for example. So, okay, we've got the concept of skateboarders.
Duncan Curtis [00:10:44]: It's a human. They've got a little flat thing under their feet. They can travel up to. Let's call it 15 miles per hour maybe in most cases.
Demetrios [00:10:53]: I would have taken you as a kilometers per hour guy.
Duncan Curtis [00:10:57]: I am. But you're converted. Make sure that I speak both now I'm bilingual, metric and imperial. So. But if we're doing. If you're doing up to maybe 15 miles per hour. And then this weird thing started happening. There was these things that looked a lot like skateboards, but they could do 40 or 50.
Duncan Curtis [00:11:16]: And so if you're training behaviors on a certain class type and you're like, great, it's a human standing upright on a flat board and maybe the handle thing, but you're not going to really notice that too much. And it's to say going on a sidewalk or on a cross street, and you're like, okay, well, it can probably only do about 15, so I don't need to slow down right now. And suddenly you get one tearing along at 40 or 50. I mean, that's a recipe for disaster. And so being able to identify those and then quickly reinforcing the model to say, hey, this is a new class type. This is actually scooters. Whole different thing. And it's a really different.
Duncan Curtis [00:11:49]: And models can learn the difference, but it needs to know to learn the difference.
Demetrios [00:11:55]: Exactly. You got to retrain. Feels like you just gotta go collect a bunch of data. Driving around India.
Duncan Curtis [00:12:02]: Oh, man. There's a reason that no one has even tried to deploy there. Man, that is like you're looking for, like, difficulty challenges. I'm like, oh, like road rules. What are road rules?
Demetrios [00:12:13]: No, don't exist. But it somehow works. I remember I got. I. When I was in India for a while, I bought myself a scooter and in the beginning, it was the most adrenaline inducing thing that I could ever do, go out on my own. And for some reason I decided to go out on my own with two other friends on the back of the scooter because it's India and when in Rome. And I remember that by the end of my stint there, there was, I was passing camels and elephants and whatever else. The rickshaws, the don't even talk about the cows that would just randomly pop up.
Duncan Curtis [00:12:53]: Yep.
Demetrios [00:12:54]: And it was no thing to me. Like my own internal model had then assimilated everything and I realized, okay, cool camel. Yeah, just another day.
Duncan Curtis [00:13:05]: Yeah. But now you know how camels move. Now you know that camels, like, first time you drive by a camel or an elephant that can crush you, you just, you're like, you're like, ah, what's going to happen? Whereas now that you've seen a thousand elephants and you're like, oh, it's not going to turn around and crush me. It's just, it's going to ignore me because I'm one of 500 in this minute. That's going to pass it. It's going to ignore it. But I really like the fact that you, your mental model had to change. And that's a really good analogy for how these driving models need to adapt and change.
Duncan Curtis [00:13:33]: And by the way, there's even differences regionally between driving styles.
Demetrios [00:13:37]: Oh yeah.
Duncan Curtis [00:13:37]: Where you get like, not just. There's also laws that are different between state to state in the U.S. for example, to Europe to, to Asia. There are lots of different, lots of different law changes that you need to respect built into the model. But also people just drive differently. You know, you think about, you know, drivers in New York and how aggressive they could be. Or you get, you know, like, hey, make sure that you're driving fast. If you're in California, I know that if you're over in the far left, you better be doing at least 10 or 15 over the limit because otherwise you're like blocking traffic.
Duncan Curtis [00:14:11]: And so, you know all these like regional differences as well.
Demetrios [00:14:15]: Exactly. Those come with the culture. One thing that I wanted to touch on was something that you glossed over earlier and how you think a lot about what's coming next. And I'm sure you've got some stories of this, like what I would coin forward combat compatibility failures. And so one story that I heard recently from a friend, I'll kind of kick it off and then see what you have in your bag of tricks. There is a friend told Me, he spent so much time hacking together all these ways to make the context window bigger when ChatGPT first came out, only to in 6 months have all of that work go right out the window as larger context windows became the norm.
Duncan Curtis [00:15:06]: Yeah, that's, yeah, that's a great example. I'm just trying to think of some that like definitely had several of those experiences where you're like, hey, let me prepare for what I think the next technological or the current technological challenges are. And then, oh my God, now I don't need to. I think a good example for that would have been say Sam is fit for nlp, so text based work. So we do a few languages but we're primarily in East Africa with English as our primary language. Yes, we've got some local languages as well, but most companies in the NLP or text space, natural language processing space, we're really looking for, you know, 120 languages, something I really distributed. And our model where we've been focused on, we believe that opportunity is equally distributed. Sorry, talent is equally distributed, but opportunity is not.
Duncan Curtis [00:16:00]: It means that we've had a very strong presence in Nairobi, in Kenya as well as in, in Gole and Kampala nearby. But it means that we've had a much more primarily English based workforce. And so scratching my head around like, hey, building plans for where do we want to, if we want to continue to expand into that market? Do we? Because we believe it's a good fit for people who are coming out of poverty. It's trainable, they've got good, good English skills or good education level skills, but aren't finding jobs. How do we fit that to the, to the AI economy and the work available there and is that an area we want to expand into? And so plans upon plans of like where in the world we would need to open up new centers in order to tap into new groups similar to what we're doing in East Africa to really make sure that we're keeping true to our mission and not just, not just doing randomly but really doing it purposefully. And then Genai came around and we were like, oh no. Oh, it's all text based except that 85, 90% of the core models in the world, English. So in terms of a fit for our existing workforce and all of our training pipelines, our sourcing pipelines, was an amazing fit.
Duncan Curtis [00:17:21]: And we were like, okay, so all of that planning, totally a waste of our time. Okay, Totally, totally not needed at this stage. So yep, that's a fun example.
Demetrios [00:17:31]: How do you look at really specialized jobs or data that needs to be annotated by very specialized or experts. And how do you think through the whole thing of hey, this is a very high skill that is needed to be able to understand this. It's not just like me that can annotate that data. I have to go through years and years of training to be able to annotate it. And so what are some things that you've been looking at doing in that realm?
Duncan Curtis [00:18:12]: Yeah, so I think that's, it's a really interesting space and I think we've seen that big trend, you know, sort of within LLMs as they got through, you know, as the, as the, let's call it, you know, education level of the LLMs has continued to progress is that we're now seeing a lot of the requests being within, let's call it hyper specialized domains where. So one of my anecdotes that I love to tell the story of is one of my most amazing product managers. I've got Patrick, he's actually a data scientist by training originally and so he's worked as a data scientist, has a advanced mathematics degree and was looking through some of these tasks and he goes, dude, I can't do these. Like I'm not at the right level for doing these. And so you, you're talking about like really starting to get into esoteric requirements. And so we've seen some of our competitors in the space and some of the other players actually build professional domain high domain expertise networks that do that. It's not something that we've chosen to do as sama only because we're with our mission, we, we're more focused on how can we take, how can we grab more generalized human intelligence and some domain expertise, but that level of esoteric knowledge, while I think it's really valuable for that knowledge to be captured into LLMs for those particular use cases, I'm seeing that rapidly accelerate and continue to go. And as I've seen in the past, where you look at something like we've now got meta's, Sam, for example, that can segment anything because of all the work that went into that.
Duncan Curtis [00:19:53]: I think that this is to, from my perspective, this is a trend that the data will be collected and then that work is going to go away. And so what we're looking at is what's the next area where we're going to still need human intelligence captured, but it's not going to be of that domain specificity level because I don't believe that the scale is going to be While it's large at the moment, for if you look at each individual domain, it's quite small, even though it's a well paid, you know, well, paying gigs, for example. And so for us, you know, this goes back to your question of you. As the technology changes, what's changing for you? I'm looking more at say, like agentic AI experiences where we're now trying to help provide feedback loops to models that are creating, creating a plan and then trying to execute steps of a plan by like, hey, okay, so if I want to, you know, book you a vacation, you've given me some dates and a location. Okay, well my plan is, okay, let's figure out where that is, let's figure out what you like. Maybe it's, we want to book a Aruba in the summer and okay, well, I know you like hiking, so let's see if there's good hiking trails. I'm gonna, so I'm gonna go out to these different websites and bring that information back and create a calendar, generate some hotels nearby that, oh, maybe you're vegetarian. So I want to make sure I, I go to websites and can specify that.
Duncan Curtis [00:21:20]: And so it's going through a lot of complex steps to get, get to the result, which is, which is a really amazing capability. But one of the things that gentic AI has been very poor at is when it does a very serial set of steps, it does them, it does them one after the other. And if it goes off the rails, it finds it very hard to either A, know that it went off the rails or, or B, recover from that. Now, I'm not saying that that won't be fixed in the future, but that has a lot more breadth and a lot more of the kind of what we're talking about, edge cases that we were talking about earlier in autonomous driving. Think about the edge cases that we manage as a human using different tools every time, I mean, every time they update, you know, the UI on, on a, on your social, on your, whatever social media you use, it's a new learning experience where you're like, okay, well I used to go here to do this and now I've got to take a look at the screen and try to figure out, oh, how do I do this now? And so you even had to recognize at the beginning that, oh no, the UI has changed. And so we believe that that's an area that is going to be very rich for a long period of time as the world and tools that LLMs use is going to continue to evolve. So the Segment Anything is a good example of where we've gotten done through a domain, we've gotten a capability and it's pretty solid. And so the amount of additional research in that area or additional work, unless it's pretty highly specialized could be solved by segment anything.
Duncan Curtis [00:22:50]: So you can take anything model and just be like, hey, I'm a startup and I'm going to use that instead of trying to label millions of images to start from now, if your startup is doing something really specialized, that's probably not going to work, but you're going to be able to use that segment anything model as a basis and you can, you can fine tune it from there.
Demetrios [00:23:13]: Ah, I see. So it's not saying segment anything version 5 is going to be so good because we're just going to start seeing it get better and better.
Duncan Curtis [00:23:22]: No, I'm saying that it's, it's already replaced a set of like human annotation work and AI capabilities that used to be something that there was a large, a large amount of interest in. So it was like, hey, can you help me build a model that can segment this camera video so that I can figure out what's in it? Let's say for a 6 security use case or for like you wanted to do security cameras and be able to detect when people were like sort of like a ring doorbell, where you're like, oh, I want to know if a person's walking up the path. Well, you can use segment Anything as a, as a base model and you know, maybe something like a YOLO and put them together and you can actually have something out of the box running like pretty quickly. Whereas before those capabilities came out and were made available, you would have to start from scratch and put up some video cameras and try to collect a lot of data and then try to have a whole load of annot done to get to a place that's, yeah, probably maybe a little bit better than SAM is to start with, but for a lot more cost. Whereas. So what I'm trying to say is on those specialized like LLM domains, I think it's going to be the same where we're capturing that information and it's going to get encoded into these LLMs and then that's going to be the basis that you can work from. So that field is not going to be around in the same scale that it is at the moment for an extended period of time.
Demetrios [00:24:44]: Yeah, I, I can see that. And I do like this idea of the technology not being the barrier to getting something up and running. And you really can get super far with what we've got right now. So you can go and create a product and there's probably the ability to use segment anything in your product. Like for this for example, this ring camera.
Duncan Curtis [00:25:14]: Yeah, but there's a, there's an interesting thing. There is that I love that it creates so much more growth potential for startups where it's like I can pull something together. I mean I could like think about my like university and college days. I could be like, oh dude, a hackathon over like a 48 hour hackathon with a couple of mates. I could, we could put together a ring doorbell suite. Like not a problem. Like this would be amazing. But the counter side to that is, is it going to be differentiated? So you're, what you're saying is that these technologies will enable new products and the way you put them into products is going to be the differentiation, but the technology is not going to be the competitive differentiator for you because someone else can see your product and do it in 48 hours as well.
Demetrios [00:26:05]: Yeah, the product and the UI and the user experience, all of that is really what we've been seeing. Like going back to these examples of using agents, I think everyone is starting to wake up to the fact that it's a lot more than just the tech. If it's not the agent, like how do we interact with the agent? What is exactly the best way to make it work?
Duncan Curtis [00:26:32]: So I want to give you a hyper relevant, like I think one of the best examples of where design just absolutely crushed it over the last couple of years. Do you know how long generative AI has been around LLMs? Basically a bunch of years.
Demetrios [00:26:48]: Yeah, exactly. Transformers came out in what, 2017, that paper?
Duncan Curtis [00:26:51]: 2017. So they've been around for ages. The one thing that changed was When Sam and OpenAI stuck a new stylo interface on it and turned it into a chat like experience and boom, the whole thing took off. But the technological change between the sort of ChatGPT model that we were using there and what they had six months before that and what others had at the time, it wasn't the core technology that was the differentiator. And I see a lot of these, you know, model comparisons where you're looking at like benchmarks in, in different, you know, skill sets and things like that. And while I will actually say that, yes, we've, we've kind of found out for ourselves and not just within my technology teams, but across different teams and people I work with, like my marketing Department loves Claude. It's like the absolute best for when you're like, do it wanting to do writing or you're doing LinkedIn posts and you're like, hey, I know what I want to write, but I want it to be better and I want to take less time doing it. The results you get out of Claude versus ChatGPT is actually significantly better.
Duncan Curtis [00:27:57]: But in a lot of domains, the tech, the differences are very minimal and it's more about how does it integrate into your life. They're like, you know, the voice interface that you can do with ChatGPT is just phenomenal. One of my. I love it. It was a while ago when that first came out. One of my PMs Ed talked about how his commute, he used the voice interaction to get like an extra hour of work done. And so he'd get in, get into the office and he'd be like. And whether it was professional work or his own personal stuff, he would, he would be like having active conversations and get through it and then just do a dump.
Duncan Curtis [00:28:34]: Like when he gets to his destination, be like, all right, well, I've already, like, I've already got lined up. I've either got my research ready for new product areas I'm looking at, or I've gotten, I've got my calendar sorted for, for, you know, my kids stuff on the weekend. Whatever. Whatever it wants. Yeah.
Demetrios [00:28:51]: So we had this guy on here a few weeks ago named Kenny, and he was really talking about data as the greatest bottleneck that we have right now in the AI ML world. I'm assuming you feel kind of the same way you're tackling a similar problem. Right? Like, why do you think that data is so much a bottleneck right now? It feels like it's still hard to go and explore and work with data. Right. And it's not a new idea.
Duncan Curtis [00:29:24]: It's not. Data has been hard for a long time. I think the why we're seeing it as a greater, even greater bottleneck than it's been in the past is the volume of data we're needed to consume is, Is getting exponentially larger. So if I think about like the petabytes of data that the LLM models are being trained on, it's. It's phenomenal. And so when you, you're. That's where we're starting to see. And I'd also say the economic impact of, of things like, you know, OpenAI, where you saw, you know, Reddit actually license their data set to, to Google and, and they changed their Terms, because people had just been.
Duncan Curtis [00:30:04]: In the past, people had just been scraping Reddit and. And, yeah, sorry, allegedly scraping Reddit and using it to train their models. But their terms were very clear. And now they're like, oh, but this is actually a hugely valuable asset. And that was right before they IPO'd. And so it was in. It was a way for them to realize that they're a data. Like the data that they had generated or their users had generated was such a phenomenal product that could be unsold.
Duncan Curtis [00:30:34]: And we're only kind of touching, like I know we've talked about, like, the Open Internet has basically been. Has. Is been scraped. I'd say there's a lot of sub areas like Reddit that are getting smarter about their data and licensing it instead. But then you've also got these huge data stores that are in private companies where it may be interactions with their apps, interactions with their services. And that data was never collected in a way that was intended to be used like this ever again. And so it's. You're ending up with this phenomenal data engineering challenge as well, let alone the scale and size of data, but also just like, oh, my God, it was collected how? You did what with it? How am I even going to, like, make this machine readable or have a human look at this or do any of that? So there's multiple, I think, threads that are making this a big challenge.
Demetrios [00:31:29]: Yeah. I remember hearing a horror story back in the day of a consultant that went in and was saying, okay, we need this data. And they were like, yeah, yeah, we got that. It's in this database. And she was saying, but it's not in this database. I don't see anything since, like, 2011. And they said, huh, that's weird. And so someone went and checked it out, and apparently one of the connectors broke.
Duncan Curtis [00:31:54]: And no one knew.
Demetrios [00:31:56]: Yeah, and no one was checking on it. And so they did not have that data since 2011.
Duncan Curtis [00:32:02]: Yeah, well, I mean, you also think about things like the public sector or very large, slow, highly regulated industries, healthcare and others, where I had a friend reach out to me the other day who was talking about a transformation project they were working on. They were taking a system from Cobalt to a more modern system. And they're like, we're trying to find Cobalt engineers to help us understand how to interact with the system, to be able to make changes or to at least migrate away from it. And it's. It. The. The wealth of hilarious, like, foibles that are going to occur as we go forward is, is, it's, it's really difficult as the world modernizes.
Demetrios [00:32:42]: Yeah. Cobalt engineering is back in high demand, literally.
Duncan Curtis [00:32:47]: I'm sure. Like, I want to, I would love to see the, the, the people either coming out of retirement or deciding to pick up that skill, that niche skill because of the high demand. Like, it's stuff that, like, when I did my degree 25 years ago was an old technology. And you're like, oh, you guys aren't using C and you're not even using C, you're using Copo. Wow. I mean, waiting for those assembly systems to come back out and we'll just do some, you know, raw machine commands and like, wow, that's incredible.
Demetrios [00:33:18]: So, so talk to me about Sama and the product itself, because I feel like that's a great. I want to know exactly what it does and where you're playing in.
Duncan Curtis [00:33:29]: Cool. So we're really in the business of getting clients, really highly valuable data sets to train their AI. Now we do things that we don't. We have a platform that we use internally. It's available, but it's not. We're not in the business of take our platform and go, you know, go train a workforce or go use your, your other stuff. What we're really here to do is we consult with companies at the very early stages when they're looking at AI problems and we say, hey, let us help you understand AI. I mean, a lot of companies we work with, while we do work with a lot of the high tech companies, which is great, there's also a big set of people who are going through what I'm calling like the AI digital transformation.
Duncan Curtis [00:34:13]: So it used to be cloud was the last one, and now you're getting to AI, where you've got a lot of industries that are like, hey, I'm getting a lot of pressure from my board. I need to use AI. I genuinely think it could be useful for me. I can kind of grok how it could be used, but I've got no idea how to go from here to there or what order should I do it in. So, you know, we talk with people and say, like, hey, what are your, what are your top pain points? What are your business objectives that you're trying to achieve? What are some of your ideas? Give us, you know, do workshops with them. What's your top 10, top 50, whatever, and then let's talk to you about, like, and what data do you have, by the way? Like, as you said, like, check on that system. Did it stop recording in 2011, like, oh, that's going to be an awkward conversation. And then really understand, like help them understand, like an ROI basis.
Duncan Curtis [00:34:59]: Like how, like what are the problems with your company's experience now? Is it, is your company like all in on AI and everyone's like, hey, let's go that way so you can just start on maybe the biggest, best project and go that way? Or are you, are you someone who might be a director of AI who's, who's maybe a brand, brand new director of AI at a company that's maybe more archaic and set in its ways? And what you really need is I need some quick wins guys like give me something that I can do in a couple of months that's going to get us a win and show some business value and then we can maybe work on to those bigger ones. Or maybe we choose projects that have parts of the company that are all under one division because it's very easy to align everyone to do it. So we work with people there. We also work with them on their data collection strategy. On, hey, how are you going to get the data if you don't have it? How do you take what data you end up with? Find the right data within it. So that curation that I mentioned before and yeah, we do the labeling and annotation and there's some, some clients that, that's all we do for them, you know, as part of our legacy business that's, you know, a large port of, part of what we do, even down to like, hey, do you need us to help like fine tune models or build models like for you and deploy them or we do all of that. So the way I like to think about what we do is we're really here as a partner on your AI journey, depending on what you need. If you're more advanced and you just need, you just need data labeling and you need to, you need someone to, who's seen this across your industry, who can help you build what we call quality rubrics.
Duncan Curtis [00:36:29]: Like how do you think about what's a good annotation and what's a poor annotation? Or do you need to expand further? Do you just, you've already got your data, you know the problem that you're solving, but you've got too much data and you don't have the money to do it. Like, how can we help you with a mix of automation, curation and labeling strategies to help you get the most value and make sure you've got good class distribution and avoiding biases. Like having a bias plan in place so that you've got a methodical way to go about that. Or we go all the way up to hey, look, I've got a business problem and I know I want to use AI. Could you help me along? And maybe we don't do absolutely everything that you need along the way, but being that we've been in the, in the industry for so long, over 15 years we've been doing this, is that we can help you. We've got partners that we can help with if you've got something that doesn't fit within our wheelhouse.
Demetrios [00:37:20]: What are some cool business problems you've been solving recently?
Duncan Curtis [00:37:24]: Ooh, that's, that's a good one. I'm going to give a slightly older one only because I, I find it to be absolutely fascinating. Elephant butts. So one of the projects we did a little while ago was it was in East Africa and we were helping to track elephants to help with anti poaching. And so what it turns out is that the back of an elephant is a fingerprint for elephants. So they're all unique. And so we were solving a problem of how do you like, we want to use AI to track elephants. And it turns out the best way to do that is by having camera footage or whether you're using drones or whether you're using fixed cameras, having a mechanism that can detect elephant butts and then compare them.
Duncan Curtis [00:38:19]: And so just given your comment about India and driving past elephants before, I thought that'd be a good fun one for you. At a larger scale though, I think that some of the interesting problems are meeting. I'm just trying to think about a specific example where we had a client who's has, I'm just trying to think is getting to the stage of validation with their model. So they've now got like good capabilities, but they're suddenly realizing the scope and scale of validation and how pure manual annotation like they've been doing for a while is not going to cut it for the scale they need to get to and trying to find the right balance and not just balance, but the right approach and methodology in order to validate across large volumes of data with a human in the loop at the right points and for the most part with humans in the loop, as opposed to like just doing the basic math where if they continued to do say, let's say single frame full annotation from scratch in a validation use case, it was just going to be exorbitantly expensive and it just didn't make fiscal sense. And so managing through with that partner and talking through the strategies of like, okay, well what if instead of looking at individual frames, we can look at larger sequences and we can look at like medium to long length sequences so that there's a lot of technologies you can use where you could say only annotate frame 1 and frame 20 and or frame 1 and frame 50 and you let AI do the interpolation or extrapolate out further for you. There's a lot of technologies that can help reduce the sort of cost per annotation while keeping that human intelligence high, high in the mix there. So as well as strategies, as I mentioned around like what data do you choose to validate? How do you pick the right things? How do you have good distribution? Like, so that you're not just annotate everything and then hope or at least then observe what you did annotate, but be proactive. Like there are tools that can let you be much more accurate in your prediction of what's in this data.
Duncan Curtis [00:40:33]: So that you're saying this is statistically the better data for us to go after. And yes, we'll do a check afterwards to make sure that we were right or to see where it finally ended up, but much better approach than saying large scale and take a look afterwards.
Demetrios [00:40:48]: So yeah, you know, one thing that I hear a bunch of people talk about and it's a great topic to noodle on is really the ROI of different projects because for many of these use cases I'm sure that you deal with, you have to make a strong case or the your champion inside of the company has to make a strong case for what they're doing and why they're doing it. Even if the board's saying we need AI, we got to be AI first. All of that, you still don't want to just create some kind of a HR chatbot that six months from now or a year down the line, you realize has absolutely no value for the company. Right. So are there ways that you've looked at. Maybe there's rubrics like you were saying, or maybe there's just different scenarios that you've walked someone through that will help illustrate the different ways that you can find business metrics to tie your AI and ML journeys to.
Duncan Curtis [00:42:01]: I think amazing topic. Absolutely love it. This is something I think about all the time, especially engaging with clients. And a lot of it harks back actually to a lot of my training at Google where I that was my first role while I'd been a CEO and Executive producer before that it was my real like introduction to Silicon Valley product management and seeing the like oh cool. And what a lot of it comes down to is MVP's how and proofs of concept. How can you minimally show, get a minimal viable product that shows value in that. Like as you mentioned, what business metrics do we want to move and how can we show we can move it? And I'll give, I'll give an example that we had a, worked with a client who wanted to produce a. I'm going to change the industry just because of the client.
Duncan Curtis [00:42:48]: But they wanted to have an externally facing LLM that could process let's say, let's say insurance claims. So they had an internal team that normally you'd either jump online and use a chatbot to do or you would, or you would, or you'd call up and they wanted to make it self service and do it all, all externally. And they were terrified because of the bar. Sorry, not terrified is not the right word. They've seen a lot of examples where there has been public failures and has led to reputational like damage for companies and that is worth like so much more. That is the, the cost year to your company valuation could be phenomenally large. So one of the ways we, we talked through with them was we said well look, what if we work together and you build this, we can help you with that. Why don't you deploy it with your current internal stakeholders first? So you build it, you deploy it, you can see how much it accelerates their work.
Duncan Curtis [00:43:53]: So you're going to drive some business like your ultimate goal was cost reduction and perhaps improve customer experience. Maybe both of those are your two, your, your two business goals. So while you've got this amazing workforce who's got a lot of experience already, why don't we deploy it with them or a percentage of them and be able to compare how does this group versus the group that's not using this tool perform? Are they faster? Are they able to, to clear more tickets? Are they able to provide better customer experience or is it actually degrading customer experience? Because yeah, you know what, what does your business really care about? And you're doing it internally meaning that they're seeing it on their screen, can copy and paste it and put it into the, they can use it within the tool and, but they have to approve it before it goes to the, the end user. And that may. And that was a great way for them to get very comfortable with it. And it, it also revealed that it, the journey to get to where they needed that model to be externally. It's still not released externally. And they're driving, they've been driving business value for over six months, maybe closer to a year now.
Duncan Curtis [00:44:58]: But it, it showed them, oh, I thought it was going to be so easy to be good to go out there, but it's not, and it can be really scary. But they're already driving business value from a much faster proof of concept. Because, by the way, when, when it first came through, there was a lot of problems with it, which is, which is fine, because the humans were able to catch it and get that feedback loop, but it was able to show value quickly, which.