Innovative Gen AI Applications: Beyond Text // MLOps Mini Summit #5
speakers
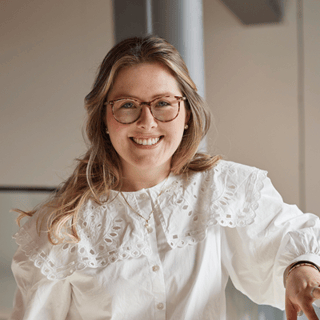
Diana is a Principal Data Scientist at QuantumBlack. Primarily working leveraging AI and GenAI with research and development teams from pharmaceutical and chemical clients. She is passionate about molecule discovery and working with multidisciplinary teams to enable AI-driven research. Diana holds an MSc in Computational Statistics and Machine Learning and has a background in Mathematics and Systems and Computing Engineering.
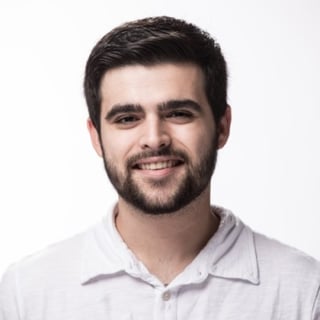
Nick Schenone joined McKinsey’s digital practice in 2023 as a Senior Machine Learning Engineer via the acquisition of the Iguazio MLOps platform. He has experience in North America across banking, manufacturing, and retail sectors where he focused on topics including Data Science, MLOps, Generative AI, and DevOps.
SUMMARY
Generative AI in Molecule Discovery Molecules are all around us, in our medicines, clothes, and even our food. Finding new molecules is crucial for better treatments, eco-friendly products, and saving the planet. Different industries have been using Machine Learning and AI to discover molecules, but now there's gen AI, which can enable further breakthroughs. During this talk, we explore some use cases where gen AI can make a big difference in finding new molecules.
Optimizing Gen AI in Call Center Applications There are many great off-the-shelf gen AI models and tools available, however, using them in production often requires additional engineering effort. In this talk, we explore the challenges faced when building a gen AI use case for a call center application such as maximizing GPU utilization, speeding up the overall pipeline using parallelization and domain knowledge, and moving from POC to production.
TRANSCRIPT
Demetrios [00:00:00]: Hold up.
Demetrios [00:00:00]: Wait a minute.
Demetrios [00:00:01]: We gotta talk real fast because I am so excited about the MlOps community conference that is happening on June 25 in San Francisco. It is our first in person conference ever. Honestly, I'm shaking in my boots because it's something that I've wanted to do for ages. We've been doing the online version of this. Hopefully I've gained enough of your trust for you to be able to say that I know when this guy has a conference, it's going to be quality. Funny enough, we are doing it. The whole theme is about AI quality. I teamed up with my buddy Moe at Kalenna, who knows a thing or two about AI quality.
Demetrios [00:00:39]: And we are going to have some of the most impressive speakers that you could think of. I'm not going to list them all here because it would probably take the next two to five minutes, but just know we've got the CTO of Cruz coming to give a little keynote. We've got the CEO of you.com coming. We've got chip, we've got Linus. We've got the whole crew that you would expect. And I am going to be doing all kinds of extracurricular activities that will be fun and maybe a little bit cringe. You may hear or see me playing the guitar. Just come.
Demetrios [00:01:19]: It's going to be an awesome time. Would love to have you there. And that is again, June 25 in San Francisco. See you all there.
Ben Epstein [00:01:33]: Thank you, everybody, for joining. We've been on a bit of a hiatus, but we're coming back strong. We have a really good session today with Nick and Diana. Nick being a senior machine learning engineer from Aguazio, which is now quantum black, and Diana also coming as a senior principal data scientist from QuantumBlack. We're going to learn a lot about what they're presenting, what they're building this week, and they have some really great and long presentations today. So I think we're just going to let them both give a quick introduction and then we'll jump right into presentations. So welcome to both of you. Diana, why don't you kick us off with a quick introduction?
Diana C. Montañes Mondragon [00:02:09]: Sure. Thank you, Ben. Very excited to be here. So, as you said, I'm a principal data scientist in quantum black, based out of the London office. I have a background in mathematics and computer science, and I did then a master's in machine learning. And I've been working in consulting a data science for the past more than six years, covering tons of different industries, from mining copper mining in Chile to pharma companies, and going through banking, airlines and other industries. So very excited to be here and share a bit on what we're doing with generative models for molecule discovery.
Ben Epstein [00:02:51]: Awesome. And Nick.
Nick Schenone [00:02:52]: Hey, guys. My name is Nick. I'm a senior machine learning engineer at Quantum Black. Guasio is now part of quantum Black, and I have a background in data science and DevOps been working with the firm for about a year now, working across a few different areas, including retail, manufacturing, banking, and a few others, whether it's traditional machine learning projects or also generative AI projects. So very excited to be here today and show you some cool stuff.
Ben Epstein [00:03:22]: Awesome. And just a reminder to everybody watching live. If you have questions, just drop them in the chat. I'm following along over there, and I'll interrupt Nick and Diana during their presentations with your great questions. I think we'll kick it off now. So, Nick, I'll take you off, and Diana, we will get started whenever you're ready.
Diana C. Montañes Mondragon [00:03:38]: So, as I mentioned, I've been working across different industries, but in the past few years, I have been working most of the time with the research and development teams from pharma, biotech, and chemical companies. And we have been leveraging AI to solve most of their important challenges. And molecule discovery is one of them, and it's probably the one I'm the most excited about. So today, I want to share with you some thoughts around it. So, let's start with the basics. What is a molecule? So, some of you might remember from your years during high school, or if you have a background in chemistry, molecules are they kind of smallest identifiable group of atoms that make up your substance, that retains such, that it retains the substance properties. So the kind of main example is water. I guess we all know water, and its molecule is h.
Diana C. Montañes Mondragon [00:04:40]: Two o. So two hydrogen atoms coming together with an oxygen atoms. With an oxygen atom. But this is just like a basic molecule. Nowadays, molecules are everywhere around us, like, from the medicines we take to, like, get better, to the clothes that we wear, the food that we eat. And most of the, you know, plastics that we use have, are made of molecules. So this is very, very relevant. And this is why discovering the problem of one or two discovery can have a huge impact.
Diana C. Montañes Mondragon [00:05:18]: Because any industry that you look around it, most likely the raw materials have a molecule underneath it or underline it that make the key components of that industry. Why molecule discovery is relevant? Well, as I mentioned, it's going to be crucial to find better treatments. We can hopefully find eco friendly products, and we're going to be able to advance the scientific knowledge to, like, other domains. Today, I want to focus in two topics, high level. So the first one, I'm going to talk about chemical language models, and then I'm going to cover a bit large language models. And for each one of these, I want to share some thoughts on how they are being applied for molecule discovery and how we can leverage them. So let's start with chemical language models. So before I deep dive into the content, I want to, like, rewind for, I don't know, a year, almost and a half since chat GPT came out, and remember a bit how the large language models are trained.
Diana C. Montañes Mondragon [00:06:27]: So we know that these large language models are trained on huge amount of text data. But basically the way it works is that out of this text, some words are kind of masked out, and the model is trained to learn which one is the next word or which one is the word that is missing based on the context. So in a similar way, these architectures for like transformers, gans, auto encoders have been modified and adapted to be able to be used in chemical language models. So how does this work? So here I have a caffeine molecule that, you know, it's part of the coffee that depending on where you are, hopefully you're having one. And this caffeine molecule can be represented in different ways. So this, for example, is the string representation, and it's called asmyo representation. And it basically is a composition of all of the atoms that make up the molecule and how they are kind of connected to each other. And if you look at this, you can already start thinking, okay, you know, we can use each one of these atoms and the sequence as text, how we can leverage these already existing architectures, but this is not capturing the whole kind of intricacy that underlines the chemistry.
Diana C. Montañes Mondragon [00:07:54]: So we have other representations. So another natural one is a graph representation that if you look at the diagram, you can already imagine, okay, if we take the atoms as the nodes and the bonds as the edges, we can already recreate something. And this is exactly what this adjacency matrix is representing, like which atoms are linked to each other. And this is a much more rich representation of molecules that give us more information. So nowadays, if you look around, if you google, there's many different models and modifications that have been done to then be trained on molecules to be able to understand them better. And why is this relevant? Well, I'm going to talk about two use cases where this can be applicable. So the first one is probably like the most obvious one, which is molecule generation. So we want to generate new molecules using a generative model, hopefully using some properties as our goal.
Diana C. Montañes Mondragon [00:09:02]: So we want to generate molecules that have specific properties using a generative model. This, of course, is not a new problem. I mean, this is what chemists do all the time. And if we take a look at, like, history, most of the molecules that we have nowadays, they have been proposed by chemists based on experience and experimentation. Of course, we have some that are, like, natural, like they are found in nature. We have others that were kind of discovered by experimentation and experience. But funnily enough, we also have a lot of molecules that were discovered by chance. And then the way the field has evolved is that chemists learned and understood what the role of the different bits and pieces of the molecule are.
Diana C. Montañes Mondragon [00:09:52]: So now they modify the molecule in, like, in different ways to assign it or remove properties from it. What we're seeing right now with the explosion of generative models is, is that we have huge generative models that are trained on existing molecular databases. And this is already very exciting. We can produce a lot of molecules using one of these models. However, there are some challenges, in my experience, playing a bit with these models as part of one of the projects. The main problem that we were facing is that we were producing a lot of non feasible molecules. So then we had to sit down with a chemist and start, like, filtering them out based on basic rules that hopefully we're going to be incorporating more in these models. And the second challenge is that we are not really fully innovating.
Diana C. Montañes Mondragon [00:10:58]: We're not really thinking out of the box. So hopefully, we're going to be able to see more innovation as we move forward. But again, this is just the beginning. I'm really excited and looking forward to have models that support a generation of model of novel, feasible molecules with the desired properties. And hopefully, this can help us progress in the different fields. However, like, the generation is just like one piece of the puzzle. Another piece of the puzzle is, for example, let's think about the case of pharma companies. Whenever a pharma or biotech company has a program to design a drug for a specific target or disease, they usually have, like, either databases.
Diana C. Montañes Mondragon [00:11:51]: And as part of their iPad, they have thousands of molecules. And once they receive a new target, they need to understand, okay, is any of the molecules that we have useful for this specific target? So they want to understand the properties that the molecule have or well, that the set of molecules have, and to understand if they can use them for a specific purpose. And this is where the second use case comes in, we want to create, to be able to create models or property prediction functions that, based on a molecular representation, are going to able to give us the properties or predict the properties of our molecules. And why is this relevant? Well, because we don't have enough resources to test every single molecule every single time. We want to do this exploration much more efficient and better. Again, this is not a new problem since, you know, statistical models started to be used and machine learning models got in their eyes, researchers and chemists started leveraging these models to try to predict properties out of the molecules. And for me, when I started looking into this domain, I was surprised of all, all of the work that went into, like, feature engineering. And there's a lot of kind of different features that are created to codify as much information as possible from each one of the molecules.
Diana C. Montañes Mondragon [00:13:26]: However, this initial approach had some challenges, or there were some challenges that are faced because of the field. The first one is that if we want to train models in a supervised manner, we need a lot of labeled data. But these datasets are usually small because we have very few labeled samples. Again, because testing in the lab is not as easy. And the second challenge is that it's hard for these models to generalize. So usually, once you've trained a model with a specific data set, it's hard to use another molecule from another data set, bring it and have a very, a good prediction of the properties for that molecule. So again, what we're seeing now is that these foundational models that have been trained in very large data sets are being super useful. And like, why is this relevant? How does it, how did this happen? Well, these models are trained in a self supervised model, so they are like, they have a self supervised pre training, which means that you don't need to have a target in order to be able to build a model that captures the essence of the language, in this case the chemical language and the molecule language.
Diana C. Montañes Mondragon [00:14:48]: And then these models can be fine tuned for a specific predictive task downstream. So you have a model that hopefully on, theoretically is going to be more generalizable because you're going to be learning from a huge, huge space of molecules, and then you can further refine it to predict a specific bias. And this is what we're seeing nowadays. However, again, this is just the beginning. You know, as we know, with more data, we are going to have better performance and better understanding. So as we have larger specialized data sets and we improve the model architectures so that they reflect better, like the chemical, the chemistry behind the molecules, we're going to be able to hopefully capture better the molecular properties and make better predictions. So this is super exciting, and hopefully it's going to bring a lot of innovation in the field. And why do I say innovation? Well, I found these numbers when I was preparing for this talk, and there's like multiple papers, there's a paper in which they try to estimate how big is the space of small molecules, and it's on the order of ten to the 60.
Diana C. Montañes Mondragon [00:16:04]: However, the largest molecular database that we have available has around ten to the eight compounds. So we still have a lot of order of magnitudes to explore. I tried to calculate a percentage, but I don't know how informative that percentage is. And I also tried hard to find a way to understand what ten to the 60 looks like. And I think I failed a bit because of all of the dimensions I could think of, none of them was this big. So, for example, if you think of the number of drops of water that we have on earth, it is only ten to the 18 or ten to the 23. So it's not even close to ten to the 60. And also the number of stars in the universe is around ten to the 22 and ten to the 24.
Diana C. Montañes Mondragon [00:17:01]: So again, not yet there. So even if we reach kind of the number of drops of water or the number of stars in the universe, we're going to have a lot of space to explore. So this is super exciting, you know, like, we have like a very, very wide space still to explore. However, if we think about the ten to the eight compounds that we already know, this is already kind of pretty big. However, the way, like, science works and these discoveries work is that potentially, or what I've seen happening is that many of these compounds were produced or synthetized or discovered for a very specific use case, and most likely they are being used for that, or they have been replaced for something else. But this is very kind of industry or use case specific. What we would like to do, and to further expand the molecule discovery is to be able to repurpose these molecules or these materials, to be able to use them in other domains where they are relevant. And this is where the second topic comes into place, the large language models.
Diana C. Montañes Mondragon [00:18:11]: So, again, being able to use the available literature and extract all of the different chemical insights is key for molecule discovery. This is more like molecule repurposing, but in a way, it's also discovering interesting molecules. This is not a new problem. We have seen, like, over time, that their NLP methods have been used to extract, like to identify the entities in the text and extract the relevant knowledge. And then databases have been created based on these and even kind of knowledge graphs to be able to be then be queried and extract the relevant information. However, with llms, there's a kind of changing paradigm, and now we can use general purpose llms to help us for this specific task. And this is the famous retrieval generation use case that hopefully, I think most of you might have heard of as it's being quite used around in the industry. So the basic understanding of it is like we ingest and process these big data sources and store them in a vector database.
Diana C. Montañes Mondragon [00:19:26]: So then whenever a user asks a question, this question is kind of embedded and compared to the vector database. So the documents that are most relevant for this question are retrieved. And then you can select how many documents you retrieved, depending on how big your prompt is. And then you can combine your question. You can create a prompt that combines the question and as context you can pass like the different articles that you identified. And all of these can be kind of processed by an LLM that uses the information in the prompt to generate an answer to give to the user. And this has been already very, very useful. Again, as moving forward, as we see like multimodal models getting better and being fine tuned with domain specific requirements, we can expect like more precise information and more relevant.
Diana C. Montañes Mondragon [00:20:27]: Like most of the chemistry papers have a lot of diagrams, a lot of images. So being able to also extract the information that is represented in these visual forms is going to be key for this use case. Now I want to share with you a demo where we are leveraging the rag use case. This is the eureka knowledge agent, which is a UI interface that underneath we have a rag use case. We have ingested millions of different papers and chemical knowledge. Let me just share. So we have in just a publication, scientific preprints grants us patents and clinical research studies. And for each one of them, this is like the timeline that we have for each one.
Diana C. Montañes Mondragon [00:21:25]: And we can select like, okay, all of them or whichever we want. I'm gonna select another of the demo uses. So we select, okay, what is the database that we want to explore and probably the years we submit, the configuration. And depending on how kind of in depth we want to go, we can select the way we're going to query the LNM. We can make one LNM call with just like the top results, or we can like do a comprehensive summary that you can do up to ten LLM calls. So here, for example, we have a query in which we say we want to retrieve all of the chemical components that can serve as refrigerants. We submit the document query search and this is going to retrieve or is going to identify which are the top chunks or documents that are relevant for this specific query query. And then you have also the option to write a prompt.
Diana C. Montañes Mondragon [00:22:30]: So here, like, you know, there has been a lot of work done in prompt engineering and how you want to present these prompts. So in this case we're saying that you're a professional chemist and based off all the chemistry text that you're going to see, please identify the substances that could serve as refrigerant and just give me the chemical substance and we submit this prompt. We also specified that we want to have a JSON that has the refrigerant name as a column and we can look at the results here. So here we have, our first column is kind of the refrigerant name, but as part of the application we also have other columns that give us, okay, a hallucination score which is basically checking how much do I trust this value, all of the document ids that were retrieved for this answer. And we can also check, you know, the document chunks. So you can see like already this is super helpful to be able to navigate all of the documents that have been like published. If you think about it, there's like when I was preparing for this talk, I think there are like 500,000 chemistry or chemistry related papers published per year. So if you were reading like one every minute, it would take up to like twelve months to go through them.
Diana C. Montañes Mondragon [00:24:00]: And this is like a most efficient way to go through all of the knowledge. This is kind of high level. What I wanted to share from these, I'm going to quickly go back to the presentation just to share the last screen. So to wrap up a bit what we have seen. So I've spoken about molecules and how like the relevance of molecules and why it is important to do like molecule discovery. We have discussed how chemical language models can help us both in the prediction and in the generation tasks, but also how large language models can help us extract chemistry insights to further, you know, improve our knowledge in our, in this domain. So I hope you enjoyed the talk and I'm happy to answer any questions.
Ben Epstein [00:24:58]: Thank you so much for giving that talk. That is incredibly interesting. I feel like we are talking so much about customer support and very generic and general text extraction tasks. To see it applied to molecular data and biology is really, really awesome. I think we will save some questions for the end and we'll jump in and let Nick give his talk as well. Just to make sure that everybody has enough time. We're going to take a little bit of shift from molecular and biology to call center analysis and call center insights. We're going to see the gambit of things that you can take advantage of.
Ben Epstein [00:25:35]: So thank you, Diana. Again, Nick, whenever you're ready, your screen should be shared and you should be able to kick it off.
Nick Schenone [00:25:42]: So, hey, everyone, Nick here going to be talking to you today about optimizing Genai in a call center application. So I'm going to be talking today about a use case that we built out with a banking client. So I'm going to be covering kind of an overview of what it is, some of the challenges that they ran into, and an overall architecture of the use case. I'd like to show it to you in action, both the end state of the UI as well as the intermediary artifacts in the pipeline. And then I want to take a deep dive on a few of the different components to talk about how the team used domain knowledge and iterative improvements to improve the overall analysis that the LLM produced, as well as the overall efficiency and resource utilization of the compute pool that they had. So, without further ado, let's go ahead and get right into it. The goal of this application was to take a bunch of historical call audio files between call center agents and between customers, and use that to improve customer experience. The idea was to do things like summarize what were the calls about, or what was the sentiment, or was the issue resolved.
Nick Schenone [00:26:56]: You could do things like, for example, build profiles of customers that the next time that they call, we're not asking the same five or ten questions over and over, but this was really designed to be step one of many where you could use this historical information in the calls, not just for the call center purposes, but also for other downstream applications in the future. For example, live agent support or generating recommendations, or like I said, customer profiles. This is a really interesting way that you could leverage data that you already have, but was stored in an unstructured format that made it difficult to have any use before. Generative AI. Some of the interesting things about this particular engagement, there were some challenges. There's a few things up on the screen. I'm really only going to highlight one. The one I want to highlight is in blue.
Nick Schenone [00:27:46]: Like I mentioned, this was a banking client. So for regulatory purposes, their production environment was on Prem. So they actually had a hybrid environment. The development happened in the cloud, in Azure, and the production environment was on Prem. So this actually meant a few different things. One is that the tooling had to work in both places and you had to be able to like switch between one to the other without major refactoring and switching text. Two, it meant that they were not able to leverage some of the more powerful API based models. Everything had to be hosted on prem using open source models.
Nick Schenone [00:28:19]: So they were not able to leverage some of the state of the art stuff, and they had to be a little bit more selective about the models that they used. And then number three, it meant that they had a limited pool of compute resources. Sometimes when I'm in the cloud and I run into scaling issues, my solution is throw hardware at it, give me a bigger gpu. They did not have that luxury. They had a limited number of GPU's that they had, and they had to use them to the fullest extent that they could with the limited resources that they had. So with some of that background in mind, I want to talk about the overall architecture of this application. This is the overall pipeline. Like I said, it was running.
Nick Schenone [00:28:58]: In this case, the development was on Azure, but the production environment was on prem. So the actual application itself was unchanged between the two, using an open source tool called ML run, which is used for a number of different things that we'll talk about a little bit later. The application was a batch application, so it was taking a batch of historical audio files, running through some analysis, and then at the end of the day populating a database. And this database was populating applications and dashboards and all kinds of fun stuff. So the pipeline itself was a few different steps. The first was called diarization. I was actually unfamiliar with this term before learning about this case study. Diarization is essentially attributing certain segments of audio, or if you think about a transcript, certain lines in a transcript to a speaker.
Nick Schenone [00:29:43]: So speaker one says this, speaker two says this, speaker one says right, so on and so forth. So it's attributing speaker labels to particular parts of the text. The second part was transcription and translation. So the transcription was taking the raw audio and turning that into text that the LLM could then take and analyze. And the translation piece was because the audio calls themselves were in a non english language, but a lot of the open source llms just performed better on english text. So they found that doing that translation piece actually helped the performance quite a bit. From there it went through a PII recognition step where they were looking for things like names, emails, phone numbers were sensitive information that they wanted to scrub out and anonymize, and they did this so that they could send it to the LLM. And they also did this so that they could then take this data and send it to the cloud for further development.
Nick Schenone [00:30:36]: Then finally we get to the LLM piece where they're doing analysis and summarization and all the fun prompt engineering stuff that we've come to love about generative AI. You'll notice that a majority of this pipeline is not LLM stuff. A majority of it is cleansing and preprocessing data, which I would say is definitely the case as well with traditional MO. So that has not really changed here, just slightly different flavor of what we're doing. So that's all the slide where I have for you. What I'd like to do next is hop into the application itself and show you around. And then we'll deep dive on a few of the pipeline components. So we should be seeing here a radio kind of prototype front end in the actual production environment.
Nick Schenone [00:31:17]: Further for the client looks maybe a little bit nicer and a little bit different, but for our purposes, this shows us everything that we need to know. So we have a table here, and we'll get into the table in a bit. Each one of these rows is a call. So we can see there's a call id, the particular person that they were talking to, the particular call center agent, and some other metadata. You can click here to play the audio file. I don't know if that comes through, but right now there is speech being spoken at me in Spanish. And then we have a transcript over here on the right hand side that is in English and has these agent and client labels. This is the diarization in action.
Nick Schenone [00:31:57]: So being very explicit about who is speaking when. Then we have here all of the features that were generated by the LLM. So there's an audio file, transcription file, anonymized file. If I keep going, we get to the more interesting stuff, like the topic of the call. What was the call itself about? A summary. So how did the call go in? High level. And then if I keep going here, there is a few other things that include, for example, was the concern addressed that this person called about what was the overall tone of the client? This is the person calling into the call center. What was the tone of the call center agent? Was the upsell attempted when you call into the call center? Like, oh, would you like to upgrade your Internet plan or upgrade your tv package or that kind of stuff? Was the upsell actually attempted? Was it successful? And then there's some numerical metrics here.
Nick Schenone [00:32:50]: Kind of interesting how these were generated, but these are some more subjective measures. Professionalism or kindness or active listening, things like that. They're being generated about the call. All these things here are essentially the end state of the application. This is what we are aiming to build out. Again, we started just with a raw audio file and are left here with highly structured data that we can use in a database for an application, as well as the translated, transcribed and diarized transcript here that we can use for further analysis. Having this in mind, I'd like to then take a look at the pipeline and run through some of the intermediary artifacts and show you what that looks like. And then we'll do a deep dive on a few of those pipeline components.
Nick Schenone [00:33:34]: I'm going to hop into the pipeline UI and this is what it looks like. Apologies if the text is a little bit small. I'm zoomed in as much as I can. You'll notice that in the pipeline I showed there were only four steps, and there's a lot more than four here. So what's going on? Most of this is housekeeping. So insert calls. This is a database operation, update calls, database, database, database, post processing and database. So most of this is housekeeping.
Nick Schenone [00:34:01]: The main four steps that are doing real work are the four that we talked about. So, diarization, transcription, piI recognition and analysis. So these are the four steps. So I'm going to start in with diarization. Just show you what this looks like here. So again, diarization, what this is doing. We have some parameters going in here. So for example speaker labels, and this is agent and client.
Nick Schenone [00:34:23]: We saw these in the transcript and we go to artifacts, we can see essentially what it's doing and what it's giving us is per audio file, give us a segment of the agent. When are they speaking? So agent is speaking from 0.2 to 2.9 seconds, and client is speaking between 7.2 and 9.2 seconds, so on and so forth. So it's doing this for every segment in the audio, for those speaker labels that we passed in. This is then being passed into the transcription step, which is doing the actual transcription. So the parameters, we can see that we're using a node group of t four GPU's. So this is a GPU based step. Each one of these steps is running in its own container on Kubernetes and can have its own runtime resources. The first step did not use GPU's, and this one does.
Nick Schenone [00:35:10]: We can see that under parameters we're using the OpenAI whisper model in order to do this transcription. We'll talk a little bit more about some improvements that were made in order to do that. Then under artifacts here, we can see the mapping of the audio file to the transcription file. Now, we can't actually see the transcript in this particular step, but we are able to see it in the next step, which is Pii recognition. So under PII recognition we can see that we're passing in some parameters around, hey, what kind of entities are we looking for? So in this case, person, email and phone number. And then what are the mappings that we want to do? So this is a dictionary mapping that's kind of saying, hey, any person you see replace that John Doe, any email you see replace johndomail.com, and so on and so forth. So we go under artifacts, we can actually see a highlighted transcript. So I got a little bit zoomed in here.
Nick Schenone [00:36:00]: Apologies for that. Actually, I think I can make this bigger. There we go. So here we can see the diarized, translated and transcribed transcript here. So we can see these agent and these client labels. The text is in English, the original audio was in Spanish, and now we can see these little highlights of the entities that were detected. So these are then going to be replaced with those anonymized values that we saw, and that's what's eventually going to be sent to the LLM. And then finally we have the analysis step.
Nick Schenone [00:36:30]: So there's a bunch of different parameters being passed into here. We'll talk about some of those later. One of which is the model name. In this case they were using mistral seven B openorca GPTQ. So it's a mistral seven B base model, fine tuned on the open orca dataset and the GPT queue. It is quantized, I believe it's quantized in four bit. And then essentially the most important artifact here is this question answering analysis data frame, which is essentially what we saw in our application. So what went in was that transcript that we saw before.
Nick Schenone [00:37:02]: In this case it was actually the anonymized version. And what comes out is a very structured, essentially table where we have those different columns that we saw in our end application, and the different features that the LLM engineered as part of this process. So overall, that's a majority of the pipeline, that's a majority of the application itself, and it's pretty much everything that the team did. Now, I wanted to deep dive on at least two of the different components. Three, if we have time and talk about some of the incremental improvements that they made and some of the challenges that they ran into along the way. Starting first with this diarization step. So as a little bit of background, before the McKinsey quantum block team was involved in this project, the banking client tried to solve this themselves and they came up with a solution that worked. But they weren't happy with it for a few reasons.
Nick Schenone [00:37:53]: One is that the quality of the models analysis was not the best, and the second was that they were not able to efficiently utilize all of their GPU resources. Remember, we're talking about on Prem, where we have a limited number of GPU's and you have to use them to the best that you can. One of the first things that the team did is they added this diarization step. Just to refresh. I'm going to go back to the application. These are these agent and client labels. So again, we're dealing with open source models. In this case, it was a 7 billion parameter model that is much less powerful than some of the API based ones.
Nick Schenone [00:38:27]: So if you didn't have the stylization step, all you're sending to the model is basically a big block of a big block of text, and the model has to infer who is speaking and when. And if you have a more powerful model, you can get away with that. But in this case, they found that adding these explicit labels here really helped the model determine who was talking and when, and really helped it answer some of the questions that it was being asked. So just introducing that step in the first place was a big win. And then the way that they did that was first using an approach called, using a tool called PI anode. So over here in these tabs, I have all the different components of the pipeline. All these are open source, by the way, so you can take a look yourself and take a look at the source code. I'm not going to deep dive into these too much, but I just wanted to show this.
Nick Schenone [00:39:13]: So there's a diary step using PI inote. So there's a little link here for PI anote audio and then the GitHub link. And what this is is a deep learning based tool for diarization and speaker detection, all sorts of really cool stuff. And it worked. They found that it worked, but it was also very heavy. One of the downfalls they found, one of the downsides they found with this particular tool is that at the time, maybe this has changed. It was only able to process one audio file at a time, so they weren't able to get the speed that they were looking for. They tried a few different things to improve the overall efficiency, one of which included distribution across multiple workers.
Nick Schenone [00:39:55]: We'll talk about that in a little bit. What they eventually landed on was actually a completely different approach. What they did was they took domain knowledge of how the audio is stored. I wasn't aware of this, but apparently call centers, they store their data in a certain way. So if you think of a regular stereo audio file, there's a left side and there's a right side. The way that call centers store their data is the one speakers on the left channel and the other speakers on the right channel. So this general purpose PI annot tool is for any kind of audio. But what the team did is they took the format of that data to do the diarization.
Nick Schenone [00:40:30]: Because if we think about what the diarization is, it's just, hey, when does speaker one talk? When do they. When do they stop talking? When does speaker two talk? When do they stop talking? They switched to a different approach using a tool called Silver VAD, which is voice activity detector, voice activity detection model, which does exactly that. It detects voice activity and says, hey, someone has talked, is talking, someone is no longer talking. One of the really big benefits of switching to this particular model is that it no longer needs a gpu. It is a cpu based model, and it's really, really fast and efficient. You can run it on a single cpu core, which meant that they could leverage multiple cpu cores to do multiprocessing. So they did this switching from the GPU to the cpu based model and then leveraging multiprocessing to leverage multiple cpu cores at once. They were able to speed this one pipeline step up by 60 times, 60, 60 times this overall pipeline step.
Nick Schenone [00:41:25]: So way, way, way faster and way, way more efficient. And it freed their gpu up to do other stuff. So that was really cool. I thought that the way that they use the domain knowledge of how the data is stored in order to take a shortcut to process this was really interesting. This would not work for any general purpose audio. They built this PI anode version if you wanted to do that, but specifically for call centers and the way that that audio was stored, you can use that Silvero VAD method. The second thing that I wanted to do a little bit of a deep dive on is this transcription step. So again, this transcription step here is taking the diarized segments and also the raw audio and then converting that into our transcription.
Nick Schenone [00:42:12]: The first thing they did is they just took off the shelf OpenAI whisper and tried it out again, it worked. But what they found is that it was not nearly as performant as they were hoping for. A few reasons for this. One is that OpenAI whisper at the time. Again, maybe this has changed since then. Does not support processing multiple audio files at once. It only processes one audio file at a time. Then two, when you load the model onto the GPU, even if you allocate multiple gpu's to the job, it's only going to take up one of those.
Nick Schenone [00:42:45]: Let's say they allocated two gpu's and they ran this thing. GPU number one was about 20% utilization and GPU was at 0%. So they were not making the full use of the compute that they had. So what they did is they did two main things to improve this. One is they implemented batching. They made it so that each OpenAI whisper model was able to process multiple audio files at once. They did this with a task queue, and I think they made a pull request to the transformers library and some other stuff. But eventually they made it so that one copy of this OpenAI whisper model could be processing multiple audio files at once.
Nick Schenone [00:43:24]: The second thing that they did is they introduced parallelization. They used a distributed compute tool called Horvod. This is running on open MPI, and it's used for distributed deep learning across multiple GPU's, and in this case, just distributed computation. So I'm going to go over to this transcribe function here. So there's a bunch of different parameters, and this isn't really what I'm talking about here, but what they added was this little decorator, this open MPI handler, where there is a worker input, in this case, the path to the data. What this guy does is essentially it takes in all the paths, it will then break it up into different chunks and then allocate those chunks of data to different workers. Each one of those workers is going to have a copy of the OpenAI whisper model, and then each copy of that model is going to be processing multiple audio files at once. So there's two levels of multiprocessing here.
Nick Schenone [00:44:19]: One is the distribution across multiple workers, where we're splitting up the data into chunks and sending it to different copies of the model. And then the second is that each copy of the model is then processing multiple audio files at once. So they found that this sped this step up quite a bit and also allowed them to use roughly 100% of their GPU utilization. They're also doing some stuff about being smart, about how they're reading and writing and all sorts of fun stuff. Stuff. So the last thing I wanted to touch on is finally this q and a step, this analysis step where we're actually doing the pipeline analysis, or excuse me, the feature generation. I didn't want to touch, I didn't really want to talk too much about the infrastructure side here, like we were about these first two steps, but just really to highlight some of the interesting stuff that the team did and built out. So I'm going to show here the pipeline code and show some of the raw questions that the LLM saw.
Nick Schenone [00:45:11]: So the way that they wrote this is essentially you would write a quiz for the LLM to ask of your piece of text. So in this case, we can see some of the questions here, like classify the topic or the summary or was the address concerned, so on and so forth. And then there's a second set of questions here that are polling questions. These are those numerical values. So if I scroll way, way down, we get to some of these generation things and we can see, all right, we're plugging in all these questions and then the output columns should have these names. And these are the columns that we saw in our data set that was outputted then in this question configuration. For the second set of questions, what we're actually doing is doing a poll. So we're actually making three calls to the LLM and selecting the most common response as the one that's going to be the output.
Nick Schenone [00:45:55]: So all those numerical values were actually the result of three different calls to the LLM and choosing the most common. So that was what I wanted to cover, talking about some of the ways that the team used domain knowledge, uh, and improved, uh, efficiency in terms of the gpu utilization to uh, make what they built, uh, move from poc to production essentially unchanged. Uh, so it was a really cool use case and I learned a lot in talking with this team and I hope this was interesting for you guys as well. So I'm going to turn it back over to Ben.
Ben Epstein [00:46:26]: Awesome, thank you so much for that. That was really, really cool as well. I feel like we frequently forget how important the domain knowledge, domain expertise is, quality of the data, all these things. But in that example in particular, having the domain expertise to understand the structure of the audio files within the call centers is really, really cool. I'm curious, Nick, really quick on your end, you used a lot of open source tools. I've used MLB run a bunch in the past. Is everything that you use right there in theory, open source usable? Anybody could pick those up, leverage ML run and get that up and running it is.
Nick Schenone [00:47:06]: Yeah, actually the whole example is in a GitHub repo, and then each of the different components is also available.
Ben Epstein [00:47:13]: Awesome. Awesome. Yeah, we should share that link out. That's really great. And I'm curious, were there any other components of ML run that you used? I know ML run has a lot of different things. It's kind of generic. Did this project require, for example, for storing data over time? Did you leverage any feature store type data or long record logging type systems or mono models? Model monitoring type systems there?
Nick Schenone [00:47:35]: So for this particular engagement, not really. It was mainly used as like the containerization, the pipeline orchestration, and the experiment tracking were the main three that we used. There wasn't a whole lot of tabular data that was used for like, inferencing purposes and like model training. If there was, the feature store would be a good fit for that. At the end of the day, they basically just updated databases. Got it. But upcoming, there is going to be some interesting stuff around monitoring this mode.
Ben Epstein [00:48:01]: Yeah, you could imagine a lot of that tabular data being passed down to further downstream models to make more predictions. That's a really cool system. Dana, I'm curious for you. You weren't leveraging ML run in your project, but obviously a lot of unique and valuable pieces of technology. Which of those are kind of internal to quantum black, and which of those are things that users or customers can actually leverage in their projects?
Diana C. Montañes Mondragon [00:48:24]: Sure. So I think we have a mix of different ones. So many of the foundational models that we leverage are actually open source, same as with large language models. It's very hard for a specific organization that is not expert in the domain just to go and train these big models. So you can find different open source models, chemical models, but also internally, we have a lot of assets that we have developed. So usually the way we work is that whenever we start working with an organization, we bring the assets that we have developed internally, and then we tailor them to their specific use case, and then this becomes their own kind of analytic software to keep improving and keep building on it.
Nick Schenone [00:49:19]: Got it?
Ben Epstein [00:49:19]: Got it. Okay. That makes sense to me. And I'm curious, in terms of the foundation models, obviously, for Nick's presentation and domain of work there, general language models would be really good at summarizing call center conversations or extracting data, because that's very common. But obviously for genomic, molecular biological data, did you find that you needed to use any domain adapted type language models, like ones that were fine tuned on quote purposes? That had to do with molecular data or did general models work pretty well.
Diana C. Montañes Mondragon [00:49:49]: For you depending on the use case, like for general kind of knowledge extraction at the moment, large language models are doing like an okay job, but I haven't seen a knowledge kind of pre trained only on scientific kind of knowledge data. I'm really excited for these to kind of happen soon and hopefully we're able to extract unidentified end is more accurate. Yeah, so this is for the knowledge extraction. If we think about the molecular representation for these, we do have a specific model. So there's like models trained on small molecules, models trained on, like, large molecules on like, proteins. So like there's a wide variety even on like, polymers. So there's like different type of models depending on the use case that you. That you want to, like, build on their leverage for? Yeah, each specific use case.
Ben Epstein [00:50:44]: Got it. Got it. That makes a lot of sense. Are there any other domains that you guys have been working with and seeing success in? Large language models beyond kind of the standard ones that we've been seeing in industry?
Diana C. Montañes Mondragon [00:50:55]: I mean, I'm very excited about, like, the work that it's being done, but it's again, related with biology and chemistry in, like, proteins, and understanding how, like, proteins bind to each other, which is like, the protein docking is a huge kind of challenge to understand better the biology. But beyond that, I don't know. Nick?
Nick Schenone [00:51:17]: Yeah, I'd say that even across different verticals, thinking about manufacturing or retail or banking or things like that, there's also things that are common across them. So things like content generation, or content synthesis and analysis, or like knowledge retrieval and things like that. So a lot of those things, I would say, are common even across different verticals. And those are a lot of the things that we're seeing. But there's a lot of interesting stuff too, that people are still coming up with, and new use cases every day.
Ben Epstein [00:51:51]: Yeah, that's very true. I'm constantly excited to see all the new technology that's coming out with LMS, how they're being applied to things I honestly never would have expected. And I'm curious because your projects were both so disparate from each other and tackled such different domains using the same technology. Were there any common, like project management or deliverable estimate tools and tracking that you were using? I feel like in a lot of data science, ML projects I've been in, a common problem versus software is being able to figure out when you actually think the model is good enough, how to track progress, how to actually make sure you're improving week over week. How are you guys handling that? Internally?
Diana C. Montañes Mondragon [00:52:30]: I can share a bit of my experience and I think it's a bit of like, it depends on the project, which is not a great answer. But overall, I mean, we usually work as part of squads with general consultants and data scientists, machine learning engineers, data engineers, Uiux designers, and we follow a methodology of project management in, in a kind of consulting way. So depending on the client setup and the client tools, we could be using Jira for tracking tasks or Trello or any of the Microsoft tools for project management. And it's more about having an iterative way of working. So I think that's probably what's common for all the projects is like we, we work like on the sprints, try to be like as agile as possible, but like knowing that agile for an analytics project is going to have like some nuances as we like, you know, depend on the data, what the data is contributing to give us. So that's a bit like my experience.
Nick Schenone [00:53:41]: That's been my experience as well. Awesome. I don't have much to add there.
Ben Epstein [00:53:45]: Yeah, no, it makes sense. It's very cool to see a lot of very cutting edge technology that we see kind of in the lab and hugging face demos, but actually being used for real use cases. So that's really awesome. Cool. We're just about up on time. Thank you guys both so much. Thank you to quantum Black as well for making these and other events for the MLabs community possible. It's really great to see the work shared by the community and if you're interested in these kinds of conversations and other fantastic conversations, make sure to sign up at the AI quality conference.
Ben Epstein [00:54:15]: It's going to to be in California in a couple of months. It's going to be a really, really great event. I'm going to drop the link for anybody to go to. Hopefully you guys are joining. It's going to be one of the biggest, I think best events for AI this year. But yeah, thank you guys both so much and thanks everybody for listening in.
Diana C. Montañes Mondragon [00:54:33]: Thanks for having us and looking forward to the event.
Nick Schenone [00:54:37]: Same here. Thanks everyone.