LLM Use Cases in Production Panel
speakers
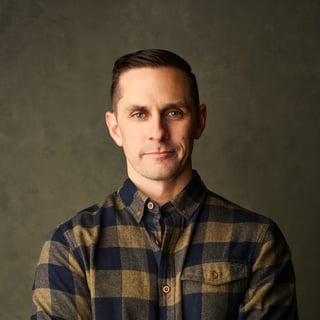
Greg has mentored thousands of developers and founders, empowering them to build AI-centric applications. By crafting tutorial-based content, Greg aims to guide everyone from seasoned builders to ambitious indie hackers. Some of his popular works: 'Introduction to LangChain Part 1, Part 2' (+145K views), and 'How To Question A Book' featuring Pinecone (+115K Views). Greg partners with companies during their product launches, feature enhancements, and funding rounds. His objective is to cultivate not just awareness, but also a practical understanding of how to optimally utilize a company's tools. He previously led Growth @ Salesforce for Sales & Service Clouds in addition to being early on at Digits, a FinTech Series-C company.
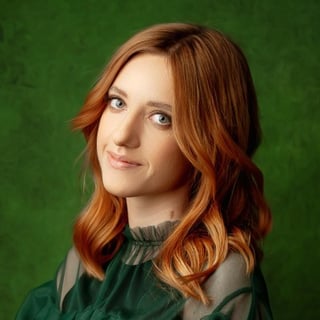
Senior AI Engineer@Chaptr working on LLMs. PhD, author of datasets, scientific papers, and publications with over 1800 citations, holding numerous scholarships and awards. Daily, she conducts her research on her grant "Detecting and overcoming bias in data with explainable artificial intelligence" Preludium, awarded by Polish National Centre. She is a co-organizer of PolEval2021 and PolEval 2022 tasks with punctuation prediction and restoration.
She organizes and actively contributes to the scientific community in her free time: she managed and led the team during the HearAI project focused on modeling Sign Language. A former organizer and a team leader at the open-source project. As an ML Expert, she supports the project "Susana" designed to detect and read product expiry dates to help the Blind "see".
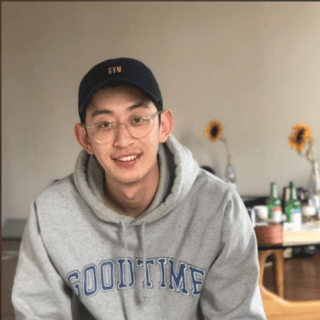
Jason is a machine learning engineer and technical advisor.
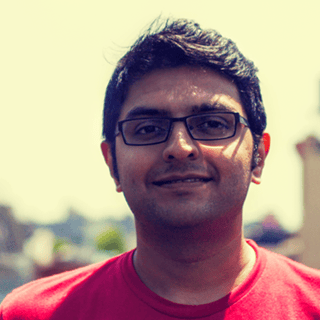
Arjun Kannan builds products, businesses, and teams. Currently building ResiDesk, bringing AI copilots to help real estate forecast renewals, reduce turnover, and hit their budget. Arjun built and led product and engineering functions at Climb Credit (serving 100k students, doubling loan growth for 3 years straight) and at BlackRock (creating $400mm in annual revenue), and helped build multiple startups and small companies before that.
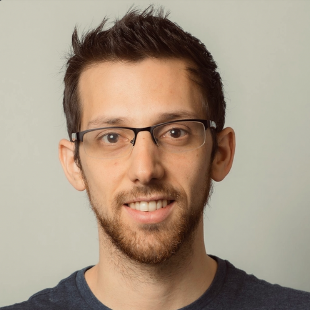
I'm a tech entrepreneur and I spent the last decade founding companies that drive societal change.
I am now building Deep Matter, a startup still in stealth mode...
I was most recently building Telepath, the world's most developer-friendly machine learning platform. Throughout my previous projects, I had learned that building machine learning powered applications is hard - especially hard when you don't have a background in data science. I believe that this is choking innovation, especially in industries that can't support large data teams.
For example, I previously co-founded Call Time AI, where we used Artificial Intelligence to assemble and study the largest database of political contributions. The company powered progressive campaigns from school board to the Presidency. As of October, 2020, we helped Democrats raise tens of millions of dollars. In April of 2021, we sold Call Time to Political Data Inc.. Our success, in large part, is due to our ability to productionize machine learning.
I believe that knowledge is unbounded, and that everything that is not forbidden by laws of nature is achievable, given the right knowledge. This holds immense promise for the future of intelligence and therefore for the future of well-being. I believe that the process of mining knowledge should be done honestly and responsibly, and that wielding it should be done with care. I co-founded Telepath to give more tools to more people to access more knowledge.
I'm fascinated by the relationship between technology, science and history. I graduated from UC Berkeley with degrees in Astrophysics and Classics and have published several papers on those topics. I was previously a researcher at the Getty Villa where I wrote about Ancient Greek math and at the Weizmann Institute, where I researched supernovae.
I currently live in New York City. I enjoy advising startups, thinking about how they can make for an excellent vehicle for addressing the Israeli-Palestinian conflict, and hearing from random folks who stumble on my LinkedIn profile. Reach out, friend!
SUMMARY
From startups achieving significant value with minor capabilities to AI revolutionizing sales calls and raising sales by 30%, we explore a series of interesting real-world use cases. Understanding the objectives and complexities of various industries, exploring the challenges of launching products, and highlighting the vital integration of the human touch with technology, this episode is a treasure trove of insights.
TRANSCRIPT
Adam Becker [00:00:00]: This panel is going to be absolutely fascinating. It's going to be a discussion about use cases for llms in production. Now, it's absolutely stellar lineup for this conversation. Gregory, I will let you probably do the introduction. Is everybody here? We got Greg here. Hello, Greg.
Greg Kamradt [00:00:17]: Hello.
Adam Becker [00:00:19]: Okay, Arjun, can I call Arjun? Is Arjun here? Let's see it. We got Arjun here. And Jason. Good to see you, Jason, it's been a while. How are you? Okay, guys, I'm stoked for this. You have 30 minutes for this panel. Greg, if I understand it right, you're going to be moderating it. And Agnesca, I don't think see your video yet, but I will be coming back in about 25, 26 minutes to bother you.
Adam Becker [00:00:53]: And until then.
Greg Kamradt [00:00:56]: Beautiful. I think that's the exit to kick us off. Well, either way. Hello, everybody. My name is Greg and I'm going to be leading a panel today on LLM, use cases in production. Now, this is a use case that is near and dear to my heart because I am obsessed with knowing how is AI actually going to turn into customer value? How is it going to make all of our lives a little bit better? And we have an awesome panel today with three folks. I will let the introductions be by each person, but either way, let's just jump into it because we just have 30 minutes today. So, Jason, can you start us off with a quick little introduction?
Jason Liu [00:01:28]: Hey, guys, I'm Jason. I'm right now the creator of a library called instructor that helps use language models, do structured outputs and validation. And then I also work as an independent consultant for a couple companies, such know, trunk tools, narrow and rewind AI and I mostly focus on things around, like transcription, summarization, and also a lot of rag.
Greg Kamradt [00:01:49]: That's super cool. And Jason is being a little bit modest because he shares a lot of really awesome content on Twitter as well. So make sure to go follow him there. And then, Arjun, do you want to do a quick intro for us?
Jason Liu [00:02:00]: Yeah.
Arjun Kannan [00:02:01]: Arjun, co founder of a company called Residesk. We do customer service for rental buildings, so if you ever talk to your landlord, we are the person on the other side, but we do that at scale. And, yeah, as you can probably imagine, we're a customer service business, so llms form a very large part of a very large portion of everything we do. So excited to get into it.
Greg Kamradt [00:02:23]: Wonderful. And I've actually had the chance to talk with Arjun offline about some of his use cases, and he has some awesome ones I think he's going to share with us today. And then finally Agnishka, can you do an intro for us?
Agnieszka Mikołajczyk-Bareła [00:02:33]: Hi. Hello. I'm glad you can finally see me. My name is Agneska. I did my phd in machine learning regarding the bias in machine learning models and data. And also I worked a couple of years in voicelap AI company when I worked with voicepots and also large language models, training some of them, fine tuning some of them. And now I'm working on the company called chapter when we are working on LLM assistant.
Greg Kamradt [00:03:03]: That's awesome. Thank you very much for joining us today. So, the first question I want to ask is going to be around the very general topic of LLM use cases. Now, when I hear these, I usually don't like to talk to AI companies because they'll just tell you a bunch of product marketing. They won't tell you the real stories about people actually getting value from these things. So I'd love to pose a question to all three of you. Arjun, I'm going to start with you, just to put you on the spot. And I would love to hear about an actual LLM use case that you're using right now in production.
Greg Kamradt [00:03:31]: And you can see the exact value that it's giving.
Arjun Kannan [00:03:36]: Absolutely. There's a hundred that I could go into. But the one that I really like that we're working on right now is the idea of unstructured property documents and actually making it so that we can answer resident questions. So the practical sort of impact of this is that our business is built on being a frontline customer service for residents. Which means if you live in a property and you have a question about the gym, about where your emergency hotline is, how to book an amenity, blah, blah, blah, usually that means you go to somebody at your front desk or you email somebody and you just never hear the answer back. We are in the middle of that. And so that means we're in the business of answering as many questions as we possibly can to help our customers, the owners and operators, save time and money and actually answer questions for our residents. Problem with that is that most of the knowledge about a property is kind of offline and really bespoke.
Arjun Kannan [00:04:31]: It's scribbled down on random notepads. It's in some PDF that was once a poster on somebody's wall, or worse, it's kind of not written down yet until you ask somebody about it. And so we were kind of struggling with this for a very long time. And by a very long time, I mean pre GPT, which is we would have all of these manuals but anytime a resident asks us a question that we didn't know the answer to, it was a very manual process of going back to the property, getting the answer coming back, and then never, ever matching it actually on the next use case. Fast forward to today. So that meant we were answering maybe half of our residents question in any given month, which isn't really saving anybody a lot of time, even though it's decent. Today we're answering about 95% to 99% of resident questions. And the reason it's happening is because we can take the very same random posters, random replies, random questions, throw them all into a vector database.
Arjun Kannan [00:05:29]: We've cut down training time for all of our customers to basically nothing, where earlier they had to solve answer like questionnaires, blah, blah, blah. And now that means most of our customers now use us instead of needing to set up a resident call center or whatever else. And there's a lot of workflows in the middle of all of this too. But my favorite use case right now is just this idea that you can take all this really weird, really unstructured, bespoke knowledge that was just in people's heads and actually make a useful repository of it in a way that just wasn't possible before.
Greg Kamradt [00:06:02]: That's super cool.
Arjun Kannan [00:06:03]: I love that $20 a month.
Greg Kamradt [00:06:04]: And if you were to put an impact metric on that, say it saves us 20 hours a month or whatever it may be, how would you quantify it?
Arjun Kannan [00:06:13]: I would say it's cut down our expected headcount on the number of associates we need by like half. We used to have one person supporting about 7000, 8000 apartments at a time before this. Now they support maybe closer to 20,000.
Greg Kamradt [00:06:37]: Wow, that's awesome. I want to move on to some other panelists here. So, Jason, I know that you're working with a lot of companies and you have a unique perspective because you're seeing a ton of different problems and solutions out there. So what's one of the ones that you like that you want to bring up today?
Jason Liu [00:06:51]: Yeah, I guess the biggest point that I've been sort of seeing as a consultant has been the fact that there's a separation between what is a capability versus the value. Right. If we were to build like a summarization prompt, for example, the value is kind of very unknown. But if we apply the same summarization tactics to something like a sales call, then if we were to be able to help you convert a sale with like 5% more likelihood, that value becomes dramatically higher. Right. If we just capture the right pain points, or we make sure we're capturing the right rapport building opportunities, then you just become a lot more impressive to the client that you're working with. And so within things like narrow and rewind, a lot of the time, it's really been around augmenting the human and making them seem much more impressive as a result of these capabilities.
Arjun Kannan [00:07:39]: Right.
Jason Liu [00:07:39]: Whether it's closing, consulting interviews, like doing research and building out reports for folks, people getting their time back is only one part of that value equation.
Greg Kamradt [00:07:49]: Yeah, I love that, and I love how you positioned it as there's a discrepancy between capability and value, because often on social media, people will correlate, oh, there's a huge capability here, so that must mean there's a huge value, but that's not always the case. So I would love to hear an example of where there's actually a small capability, but you've seen a huge value. So, like the summarization topic you brought up.
Jason Liu [00:08:11]: Yeah, so one of the examples is actually from a startup that I was working with earlier this year where we were just doing extraction out of a transcript. Right, but if you were to sell extraction out of a transcript, that could be a free DBD app. But the audience that they captured was executive. Not executive assistants, executive coaches, or. These are people that will charge $800 to $1,000 an hour to sort of help a board of directors manage the CEO. And they would take about 2 hours to just extract quotes to prepare this presentation. And so the API call for GBD five ends up being $0.50, but the actual upside for the downstream user becomes thousands of dollars because of exactly what they're trying to do. And now, because we have very good metrics on, okay, what is or is not a good quote, we can fine tune against that.
Jason Liu [00:08:57]: You end up charging maybe like $100, $200 for a single API call just because the value is there. Right. If you were to sell summarization as a service, it's very cheap, but if you niche down and capture a very specific target audience, maybe salespeople or people who go door to door and sell solar panels, the more you niche down, the bigger value you can capture, because we know exactly what they're trying to solve.
Greg Kamradt [00:09:22]: Yeah, I absolutely love that. That's really awesome. So then, Agnishka, I want to move over to you and hear about a use case that you're excited about within your line of work right now.
Agnieszka Mikołajczyk-Bareła [00:09:33]: Yeah. So when we usually talk about AI in business or how it affects the business, we usually talk either about lowering the cost or getting higher sales, right? So we already talked about that. Also about higher efficiency. But one of the very nice projects I had in the past was detecting arguments within the sale calls. So we had transcripts, like thousands of hours of transcripts, which we used to detect arguments and objections towards those sales calls. So what actually happened is we could discover what arguments worked better for each objection. So for example, if customer didn't want to buy something, we could say for example that, but we are the leader in the market, but maybe it doesn't work. Maybe the better way would be saying yeah, but actually our price is the best on the market, maybe this will work better.
Agnieszka Mikołajczyk-Bareła [00:10:41]: And we were able to find the best arguments for each objection and our customer used that argument and increased the sales by 30%. So we thought it was really amazing.
Greg Kamradt [00:10:56]: Wow, that's very cool. 30% is a ton on the sales side. Do you know, would they incorporate this into their training or how would they incorporate this data into their workflow?
Agnieszka Mikołajczyk-Bareła [00:11:05]: Yeah, so actually they used it in the training, they talked to their workers, show them how they can talk with clients and they increase the sales. It was easy as that. So just investigating the data. So maybe they had some better colors that used those better arguments, but there was no knowledge sharing along the team. So this helped a lot.
Greg Kamradt [00:11:32]: That's awesome. That's very cool. And so I want to move to another question here. And this is almost around the anti value meaning paths that folks may have gone on that haven't been as fruitful as they wanted. So Arjun, I want to start with you because I know at residesk you're running a lot of experiments, a lot of mini workflows. Which workflows have you started, maybe implemented, but then have cut because the value wasn't there for the overhead it took to maintain.
Arjun Kannan [00:11:59]: I think when we started, we approached it, the mistake was, I think over optimizing and over engineering so broadly, the mistake was solving this like an engineering problem instead of a product problem. And what I mean by that is when the LLMs came out, we spent way too much time optimizing for the best possible prompt, the best possible latency, which meant that I think our lead time to ship something with an LLM was just like not bad, but it was still on the order of weeks instead of on the order of hours or days. And the biggest shift for us was just changing that to be like, no, just ship what you can and then evaluate it with an LLM continuously in production. And that changed quite a bit of, quite a bit of our entire workflow and ended up being valuable. So, concrete example is we want an LLM that scores every conversation that we have. Much like what Agnesko is talking about with a resident.
Jason Liu [00:12:59]: Right.
Arjun Kannan [00:13:00]: And we spun our wheels for way too long trying to figure out what are the exact segments that we want to score along, et cetera, et cetera. And that took us like months and was a waste of time. And instead what we should have been doing from the beginning. What we do now is just like, score it, tell me anything that's worth noting and then collect 1000 examples. Use that to develop your buckets, use that to score your next thousand examples, and then go from there. It's the same lesson, I think, that you learn with every new tech paradigm, which is think about it from the product angle first, even though the engineering angle is so much more interesting or it seems so much more interesting at the beginning. So we over optimized at the beginning and didn't really monitor continuously, and that was a massive pattern that we needed to change.
Greg Kamradt [00:13:46]: Yeah, that's very cool. It just reminds me of the old adage around, just get the MVP out, start there, and then iterate on performance after and optimize later. Yeah, cool. Jason, I'm very curious to hear your answer to this question, too, because I know you engaged a lot of projects with a lot of different companies. What has started? But it wasn't worth the overhead to keep on maintaining or it didn't work out.
Jason Liu [00:14:09]: I guess a lot of it has almost been at an organizational level. I think just in the same sense that Arjun talked about how instead of engineering, we should focus on product. One of the big things people started trying to do is sort of plan too much ahead of time. Right. I think in engineering it's very easy to think of, okay, what are the exact deterministic results we're looking for? What are the 20 tickets that we can break this problem down into? And then what are the edge cases we expect to bump into? Right. The issue here is just that it's product and not engineering. A lot of the machine learning optimization pipeline is very much science and not engineering. Right.
Jason Liu [00:14:47]: And so I find that a lot of these junior engineers, or engineers transitioning into doing more AI related work probably just spend too much time guessing the outcomes rather than optimizing a test pipeline. We could spend the week writing up this documentation, proposing what could be fixed or what needs to be improved. But instead of doing that, if we focus on metrics, hypotheses and experiments, we end up being able to iterate a lot quickly, a lot more quickly without sort of the overhead and the complexity of trying to guess everything.
Greg Kamradt [00:15:18]: Yeah, absolutely. You brought it up just a little bit. But I like the advice for junior engineers trying to get into more AI related work, so less on the technical side, but what advice do you give to them more on the meta level about their learning path and which direction they should be pointing?
Jason Liu [00:15:32]: Yeah. Understand how we should be focused on evaluations, be educated on what kind of metrics and evaluation tools we have at our disposal, and really focus on building experiments that are very fast to run. Right. When a test takes 1 minute to run versus a test that takes an hour to run, this really changes the way that we do the work that we want to do. And in particular, it gives us the ability to build an intuition as to how this problem is going about. And then on top of that, just making sure we look at the data, if we have evaluations, we can now look at things that are performing well and performing poorly and build this internal intuition that will, that will be something that you take with you for the rest of your career.
Greg Kamradt [00:16:14]: Nice. I love it. A test that takes a minute to run versus an hour, or a test that takes a week to set up and then you can finally run it is also a pain in the butt. So I want to switch gears to you, Agnishka, about understanding how you prioritize your energy. So there's a lot of things we can all work on, but because the tools maybe aren't as mature as some other tech stacks, well, it might take a while to go implement those, and we need to prioritize our energy very well. How do you think about the projects that you're taking on in your career or your work? And I guess, yeah. Could you step us through how you pick your projects?
Agnieszka Mikołajczyk-Bareła [00:16:48]: Yeah, this is very interesting. Very interesting problem. I myself often have problem with managing my time, but what I usually do is listening to podcasts. So learning from others, listening to podcasts, reading blogs, reading papers, and just being up to date of what's happening. So because I'm learning and I'm learning from others failures, others successes, I can avoid my failures myself. So I think this is very important to just be up to date with everything. I know it's very difficult and it's sometimes almost impossible to catch up on everything, but I myself go into archive website every three days and check every paper. I don't read all of them.
Agnieszka Mikołajczyk-Bareła [00:17:42]: I read the title. I can see if it's worth checking and I read it and I think this is very important to survive in this field.
Greg Kamradt [00:17:53]: Yeah, absolutely. And so when you check archive and you have this idea around, hey, we're going to eventually need to be building products that provide value to customers. What sort of things catch your eye as you scan through those?
Agnieszka Mikołajczyk-Bareła [00:18:05]: Okay. Because I have a PhD background, I can quickly search for those papers read and I know what's happening there. So first I read an abstract and I can see if it's a nice paper, I can see if it's written nice. And this will probably mean that it has a good value. So I go through the methodology, usually through the results, and when I see that the results are good enough for me to read, I read the whole paper. If it will bring value to our product we are developing or if we have an idea based on that paper for a new product, then we can move with it. And also it's pretty nice to use LLM assistance to summarize those papers. So you can always use either GPT or Gemini or other LLM to help you summarize the paper and find the key points.
Greg Kamradt [00:18:57]: You love it? I love it. Arjun, I want to move over to you. So at residesk, you're running the company again. You only have a finite amount of energy to pick your projects. What's your evaluation criteria? How do you think about what you take on?
Arjun Kannan [00:19:13]: So I'm going to give you the boring answer, which is it goes back to where the company is and what our customers want. We happen to be before our customers started telling us what they needed, it was much more of a discovery tool. So it was closer to what Agnesko was saying, which is discovering all the capabilities that we have with this technology and surfacing new features in the hope that one of them turns into a hook that captures user value. But nowadays it's much more of we have a pretty strongly validated pipeline in terms of customer problems we need to work on for the next couple of months. Almost too validated, I would say. So now it's really about we're constantly having to make trade offs between where do we choose to expand our margins, aka automate our humans versus expand our product and increase prices, aka make our human team much more awesome. Right now, my general framework is much more weighted towards focusing on use cases that make our human team much more awesome. So that's why I go back to improving our understanding of documentation, improving our understanding of our customers, like investor playbooks.
Arjun Kannan [00:20:26]: So that anytime a great conversation goes through our system that matches something that makes them look good to their investors, like we surface that, things like that. So that's sort of our general outlook. Llms haven't fundamentally changed the way that we prioritize things with the business. What they have changed is the cost of experimenting within those priorities. So business priorities are always like, grow your top line, grow your bottom line. LLMs have just made it far easier to run ten things that help you do that instead of do two things at a time with a small team.
Greg Kamradt [00:21:04]: Totally. And I know there's popular frameworks out there to do prioritization, like the impact and effort. Do you follow that line of thought, or how do you prioritize what you work on? Internally?
Arjun Kannan [00:21:15]: We do a very rough impact and effort prioritization, or at least we used to. The denominator or the effort just ended up becoming so small that everything started to feel like it was a good priority. And so now instead, we've basically run a lot of tests around what a customer might pay for something that we do, and that has replaced the effort piece for us, because a lot of projects in the medium term for us are all roughly equivalent in terms of how much time they're going to take, but they're vastly different in terms of how much a customer would pay for them.
Greg Kamradt [00:21:54]: Yeah, that's a whole nother path, because there's the internal operations, there's building out product, but then there's also how much is the customer going to pay for it. So that's a lot of factors to tackle there. Jason, I want to move to you, and the lens I want to look at this question through specifically is around the user research side. And that's kind of a lame name for how do you identify projects that are going to make the most impact, value wise, to an end user, not necessarily from the technical side?
Jason Liu [00:22:24]: Yeah, I guess here I'm really borrowing from the framework of Alex, from Ozzy, where he talks about the value equation.
Greg Kamradt [00:22:29]: Right.
Jason Liu [00:22:30]: The idea is that the value derived is a function of the dream outcome times, the likelihood of success divided by the time it takes to get there, and the sacrifice that we are willing to give. Right. And so it's an amazing book.
Greg Kamradt [00:22:44]: It is.
Jason Liu [00:22:46]: And ultimately, that has been an incredibly simple framework to apply.
Greg Kamradt [00:22:52]: Right.
Jason Liu [00:22:52]: It's like, okay, are we saving them time by giving them 2 hours, by doing something in ten minutes? Are we giving them the likelihood of success because there's some kind of coaching aspect or some kind of making sure that if a human is 95% accurate, are we getting them to 99% accurate? Or is it purely an effort play where reading legal text is just very, very difficult. And so I think that, for the most part, has been really helpful to apply and at least prioritizing some of these projects. And ultimately the most exciting thing really is around. What is that dream outcome. I think right now we think of simple things like summarization, but really what we want to sell is becoming a better salesperson or becoming like a better partner by having perfect memory. These are kind of things that I'm really excited about.
Greg Kamradt [00:23:38]: That's cool. When you say become a better partner, do you mean a romantic partner here or what are you talking about?
Jason Liu [00:23:45]: Yeah, even in this example, I think about, for example, with rewind, we think a lot about giving you perfect memory. But what is that perfect memory in service of? If you were a salesperson, remembering that thing about you from two weeks ago or the reason you had to reschedule, those are touch points that are very useful. But in the same sense, remembering what kind of flowers someone likes or whether or not you go traveling, you see a postcard, you say, oh, this person thinks about that thing again. The idea of search and memory are now applied to these much more human outcomes. And that is kind of the grand vision.
Greg Kamradt [00:24:22]: Totally. Yeah.
Jason Liu [00:24:23]: If something costs $30 a month but it makes you a better person, it's basically free. But if it costs $30 a month, but it just helps you answer a question every once in a while. That same system, positioned differently, has a different kind of value equation.
Greg Kamradt [00:24:36]: Yeah, I think that's great. And I want to be mindful of time here. So we just have five minutes left. It's amazing how quickly a session like this can go. I'm going to finish up with the fun question, which is talking to your prior self. And so we've all taken on projects. We've all had a couple of lessons learned. So, Agneski, I want to start with you first.
Greg Kamradt [00:24:53]: What would you tell yourself, say, I don't know, call it 18 months ago, about what you've learned over these past years with regards to picking projects that are going to create impact.
Agnieszka Mikołajczyk-Bareła [00:25:07]: Yeah. So definitely, I would say focus more on user value than on exciting research because it's really easy to get lost in exciting AI research in developing the most recent technology. But as Jason said, we are selling the user value. We are selling use cases, not the exact AI script or program.
Greg Kamradt [00:25:34]: That's wonderful, Arjun. I will pass the mic over to you. What have you learned over these past couple years that you would tell your prior self?
Arjun Kannan [00:25:42]: I think the main thing for us has been, don't follow the flash. A lot of our AI intuition comes from seeing great products like rewind or whatever, that are magic. But in the context of what we've built for our company, our customers actually like it when it feels more human and less like AI. And so to us, it was, don't focus too much on the technology. In fact, the best way to have an AI product go get out there in the wild is to make it feel human. Has been the lesson that we've had to learn.
Greg Kamradt [00:26:19]: Cool. I love it. And then, Jason, we'll finish off with you. What have you learned over the past couple of years that you tell your prior self?
Jason Liu [00:26:27]: I mean, for the most part, this is kind of very selfish, but I've just been really enjoying sort of understanding the long tail of issues of what happens in production. Right. I think very many people can get the 80% result, make the tweet, but then when you actually launch a product, you end up just running into things. Okay, now we have a turn problem because we got the virality, but now we can't actually deliver on these promises. And just getting exposure to many different industries and different verticals and seeing what that long tail looks like, whether it's the idiosyncrasies of the questions in a rag application or how difficult it is to even sort of parse specific kinds of data, those are the things I find really enjoyable.
Greg Kamradt [00:27:04]: That's cool. I love Adam. Sorry, you're going to say, I just said, how do I finish it off here? Just with one lesson learned that I've learned myself over here is I've really come to learn the value of a headline before you start building. So if you aren't sure what you're building and you can't succinctly put it into a headline, that's not a great place to start. And also, when you're communicating it to users, if you can't put it in a simple headline that somebody can get right away, well, it's not going to get easier after you've built it. So I think headlines matter for my own clarity and for also communication to other users here. But either way, I want to say thank you to the three panels today. That was a wonderful.
Greg Kamradt [00:27:41]: Again, it's amazing how quickly these things go by. And Adam, we just had a great session here, so we're ready to pass it up. Back off to you.
Adam Becker [00:27:48]: Thank you very much, Greg. Just along that line of thinking about the headline, there's a really good book that I read called working backwards about how Amazon product teams start building their products, the first thing they do is come up with a press release. Is the press release itself even attractive enough to get anybody's attention? If so, then you can go build it. You can't even do this. Forget about it. I want to ask you guys, there's been a couple of questions in the chat, and we didn't really leave time for Q A, so perhaps like rapid fire, like one sentence answer, if you can. Okay, smuriti is asking, could you guide on some references on how to validate quality workflow for LLM outputs?
Agnieszka Mikołajczyk-Bareła [00:28:31]: I think there are lots of open frameworks, for example, Langsmith is one of them. So just use open frameworks.
Jason Liu [00:28:44]: Okay, my answer is revenue.
Arjun Kannan [00:28:48]: Same answer for me. Whatever gets people paying.
Adam Becker [00:28:54]: Nice. Okay, last one is, smriti is asking, where can you source these papers from? Agniska, I think that one was for you.
Agnieszka Mikołajczyk-Bareła [00:29:04]: Yeah, so it's a website called archive Arix. You can search it. And it's a website with a preprint. So papers before the review.
Adam Becker [00:29:19]: Awesome. And then last one, maybe any trick for recurring value from Pablo?
Arjun Kannan [00:29:32]: Interesting one.
Jason Liu [00:29:33]: Do you say recurring value?
Adam Becker [00:29:34]: Oops, connection lost. Can you guys hear me now? Okay, yeah. So I think the question was, any trick for recurring value?
Jason Liu [00:29:54]: You should take this one.
Arjun Kannan [00:29:56]: I'm thinking about it. Ideally, if you have llms in your workflow, they're constantly adding value. So in a sense, that is recurring. But maybe I'll answer a slightly different question here, which is, how do you continuously get more value out of a workflow that you've put in production? And I think the answer is use llms to evaluate your LLM workflow. The meta aspect of using llms is highly underrated. They can do 100 itests for you, do every hour, so you can continuously improve your workflows. And that's how you get better efficiency, better output, and ideally more revenue.
Adam Becker [00:30:35]: Wonderful. Thank you very much, guys. This was a sick panel. I appreciate all of you for showing up and sharing these insights with us.