Machine Learning from the Viewpoint of Investors
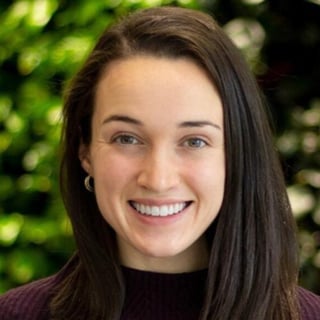
Leigh Marie Braswell is an investor at Founders Fund. Before joining Founders Fund, she was an early engineer & the first product manager at Scale AI, where she originally built & later led product development for the LiDAR/3D annotation products, used by many autonomous vehicles, robots, and AR/VR companies as a core step in their machine learning lifecycles. She also has done software development at Blend, machine learning at Google, and quantitative trading at Jane Street.
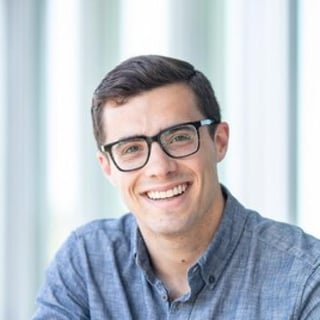
Davis is currently a principal on the investment team at Innovation Endeavors, an early-stage venture firm focused on highly technical companies. He primarily focuses on software infrastructure, especially data tooling and security. Prior to Innovation Endeavors, Davis was a product manager at Google, where he worked on the Pixel phone and the developer platform for the Google Assistant. Davis studied computer science and electrical engineering in college.
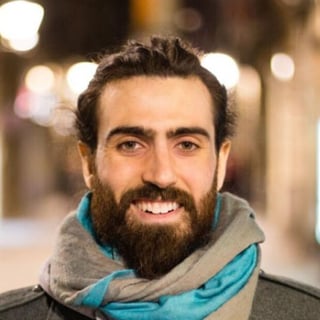
At the moment Demetrios is immersing himself in Machine Learning by interviewing experts from around the world in the weekly MLOps.community meetups. Demetrios is constantly learning and engaging in new activities to get uncomfortable and learn from his mistakes. He tries to bring creativity into every aspect of his life, whether that be analyzing the best paths forward, overcoming obstacles, or building lego houses with his daughter.
Machine learning is a rapidly evolving space that can be hard to keep track of. Every year, thousands of research papers are published in the space, and hundreds of new companies are built both in applied machine learning as well as in machine learning tooling.
In this podcast, we interview two investors who focus heavily on machine learning to get their take on the state of the machine learning industry today: Leigh-Marie Braswell at Founders Fund and Davis Treybig at Innovation Endeavors. We discuss their perspectives on opportunities within MLOps and applied machine learning, common pitfalls and challenges seen in machine learning startups, and new projects they find exciting and interesting in the space.
Investing in MLOps // Leigh Marie Braswell and Davis Treybig // Coffee Sessions #81
0:00 Demetrios All right, y'all – we got a special MLOps Community Coffee Session podcast for you today. I'm talking with Davis, Treybig of Innovation Endeavors and Leigh Marie Braswell of Founders Fund. Both of these two incredible guests are specifically focused on data products. Davis was a PM at Google before he started working at Innovation Endeavors and Leigh Marie was actually a PM at Scale AI before she went over to Founders Fund. So, this was a cool conversation. I'm pretty excited about what and where we went. We talked about what kind of things they are looking for in the market, the bigger macro picture of MLOps, where they think consolidation is ripe for happening in the next year or so, different parts of the ML workflow, and what tools could potentially be combined, or what tools might go and eat up other tools. And then we talked even about open source, how they look at deals when there is an open source product and the community around it, the structure, and also the momentum that it has – a hot take from that was, for me, kind of eye opening that you don't necessarily always have to build an enterprise offering on top of an open source tool. So if you're an open source team that's just got an incredible amount of traction with your open source product, Davis mentioned that you can create an adjacent enterprise tool that is very, very complementary to the open source tool that you have this huge following around. I appreciated this conversation so much because it is a way for me to get out of the nitty gritty of MLOps and also see their macro perspectives and look at what they are investing in, look at their vision for the future, and also how they go about doing deals. So that's it for me and this intro. In case anyone would like to reach out to these two because you've got a hot new MLOps product that you want to showcase to them, you can find their information in the description below. For now, I will leave it at that. Enjoy the conversation. [intro music] 2:25 Demetrios Welcome, Davis and Leigh Marie. This is awesome to have you both here. I think this is the first time that I've been on a podcast, where I've actually met the two people that I'm interviewing in person. And luckily enough… 2:40 Leigh Marie Oh, wow! 2:41 Demetrios [chuckles] Yeah. This rare occasion for what we have been living in the last, what, three years? So we got to meet up in separate meetings, but I got to meet both of you. And after meeting both of you, I thought it would be super cool to have you both on a podcast like this, just talking about what you're seeing in the market, because both of you are investing in companies that are going to be changing the landscape that we look at in the MLOps space. I know that you're probably up to your nose in seeing MLOps pitches and all that good stuff. So I think it's good to start out with just like, “Where are we now, in MLOps? What are we looking at? What have you been seeing? What are some things that you want to call out as trends or different things?” And maybe Leigh Marie, can you start us off?
3:41 Leigh Marie Absolutely. Yeah. So I guess to kind of clarify, when I'm talking about MLOps, it's the act of putting machine learning in production. Typically, the term tends to apply when it's more complex, so when it's deep learning or something like that. And kind of the reality of the situation is – not that many companies are using or urgently need deep learning. There are quite a few, there are now well known use cases of deep learning where that is what you need, you can't substitute with something more simple. But given how early it is on the adoption curve, this MLOps sort of infra startup market is extremely crowded, which is kind of an interesting dynamic. And I think that's because if you are an ML engineer and you're trying to put a model into production, it is very complicated and there are currently no sort of “consensus choices” for multiple things that you need to do. So tons of startups have popped up to try to fill that need. But yeah. Right now, it’s very crowded. I would say there are multiple categories where there's not a de facto solution like, “What's the de facto infra active learning tool?” or “Tool for collaborating with non technical stakeholders,” there's just no consensus and a lot of startups. 5:07 Davis Yeah, it's definitely a crowded space. I think there’s tons of point solutions. I think the core categories that are going to end up existing in this space still need to be figured out. You see, you know – I think to build an MLOps stack today is 6, 7, 8 tools. I talked to many data scientists who were just overwhelmed, right? It's so hard to understand what to do and how to stitch these things together. So I think you're going to see some consolidation. I think there are also some segments where terms are very ill defined, and people don't really even know what they're looking for. Like, I think feature stores are a good example of that. There are others as well. So it's interesting that we've seen such an explosion in this space, kind of this wave one, wave two of startups. But probably, in spite of that, there's a lot more to be figured out. 5:49 Demetrios Speaking about consolidation – where do you think is ripe for consolidation? Where do you think there are currently a few tools that are happening right now and could potentially get eaten by a bigger Databricks or DataRobot, and you see that happening? Or combining – a new tool comes out, and it combines a few different pieces. Davis, maybe you can go on this one and then we'll let Leigh Marie. 6:19 Davis Yeah, there are a couple of things that immediately come to mind. One is that today, if you look at I guess, model orchestration and all the components associated with that – tracking and experiments, tracking models, etc. – I see a lot of companies are stitching together, they're doing a lot of the orchestration with Airflow, they're using something like MLflow, or Weights & Biases, a couple of these other tools for basically tracking all the metadata associated with managing these pipelines. That, to me, feels like it's kind of all the same thing, right? Which is like, I'm running these trainings, running these hyper parameter tunings, orchestrating all these models and I think you kind of want all of that packaged together. So, a newer startup or newer product that's kind of going in more in that direction is something like Metaflow, where you're starting to get a lot more of that bundled together. So I think that's one area where you'll probably see consolidation. I've wondered for a while if you'll see more of it on the data prep side. Today, labeling is very distinct from tools like Aquarium Learning that are similar, which are more about understanding skew in my data, understanding where I need to augment the data, etc. I feel like data prep in general, especially, it's also tied very closely to feature engineering. I'm wondering if that should be more consolidated or tightly linked. Though, there are reasons that some of it should be separate. And the last is on more of the monitoring, kind of post-production post-deployment side. One notable thing I find weird there is the separation of some explainability tools versus monitoring tools. And more broadly, I'm seeing more monitoring tools, more towards thinking about monitoring the end to end performance – across training, testing, validation performance, and understanding skew or drift across those and what that means for iterating on the model. So those are three immediate categories that come to my mind. 8:03 Leigh Marie Yeah, I definitely agree with those categories, I think, just to add maybe a little bit of color to two of them – the most recent one that you mentioned was explainability, monitoring, which totally makes sense when you want to monitor… Another part of this is also the data. So, right now there's this split between data quality monitoring and then ML Monitoring, and there's probably a lot of overlap, like when your model goes off the rails, it might be because of bad data. And right now you have tools that are kind of ML-specific or non-ML-specific, like Monte Carlo versus, I don't know, Arize. I wonder if there's some potential for consolidation there. Then also, on the monitoring side, you have tools where their sort of tagline is, “Detect when your model’s misbehaving.” Well, what's the next immediate thing that you want to do? You want to fix it, right? So I think there is a lot of work that could happen when it comes to “Okay, the monitoring tool has flagged something. What do you need to do?” Like, “How do you need to retrain?” Or, like “Can the model still be going in production? What's the failsafe?” I think that's a category that's ripe for consolidation. A startup like Gantry I think has written a little bit about this. Then, of course, I'd be remiss not to mention on the data prep quality side, I spent four years as an engineer and product manager at Scale and I think Scale is already starting to see this area as ripe for consolidation. Scale started out labeling training data for autonomous vehicle companies, they quickly realized that the same infrastructure that you can use to label training data for AV companies, you can use to label training data for all types of use cases like NLP, or different types of 3D data because that core quality infrastructure is the same. So they kind of consolidated that view and now, you see them expanding to, I think recently they announced a big push into synthetic data. So data that mirrors the quality of real world data to augment your training set in a cheaper way than just collecting a bunch more real world data to find edge cases. And then also, they have the Scale Nucleus product, which is somewhat of an Aquarium competitor, where you can see all your data, see if your data set is balanced, and figure out what data you need to label this into Scale. 10:32 Davis Leigh Marie, one thing you mentioned that I’d be curious to hear your broader take on is – you mentioned the dichotomy between more classic analytical data infrastructure tools, in which there’s all these quality tools, automation tools, etc. and that stack is so different from the ML tooling stack today. I'd be curious more broadly, to hear your take on – besides quality, which is one area that makes sense to me – do you see other areas where it would make more sense for some consolidation versus the stacks being so bifurcated? 11:01 Leigh Marie Yeah, it's interesting. I mean, I think when it comes to data pipelines, you'll potentially see some consolidation as more companies realize, “I don't only just need the bread and butter ETL workflow orchestrator, I also want now to deploy multiple machine learning models in production.” So I do think that there's a bit more of at least brainstorming around the idea of, “Yeah, how do we make a workflow orchestrator for ML and data?” I think that's one area. I think there's also kind of an area of analytics now, where I guess the best way to describe it would be – for an ML model to get made, you want to have a lot of input from not only the ML engineers, but also probably the data scientists who have built models before, the analysts who have to deal with the results of these models or interpret the results of these models, or non-technical users that have to that might have either deep learning or regular models as an integral part of their workflow. I think you're starting to see a rise of lots of analytical tools that realize the need to accommodate stakeholders of various technical complexity, like Hex Data or Streamlit – Companies that accommodate easy sharing of data apps, whether they're ML or not. 12:36 Demetrios Yeah. And I've heard that called, which I liked (I stole this from somebody that I can't remember now) “the last mile of machine learning,” or even MLOps, where you're taking the model and then you want to get that value out of it. And it's that last bit from where you have it in production making predictions to actually seeing, “What is it? Is it helping us? What kind of value are we actually getting from this machine learning?” So I completely understand that. Now, as you both look at deals, and Leigh Marie, when you're analyzing something to invest in, what kind of pain does it need to solve? And how do you recognize if that's a real pain, or it's just a hammer looking for a nail? 13:27 Leigh Marie Yeah, I think this is a great question and I kind of want to zoom out a bit. I think it's really easy, especially for somebody with Davis’ or my background or your background, to just kind of be like, “Oh, there are all these holes in the MLOps landscape! I want to find a company in each one of these buckets.” I even wrote a blog post where if you kind of skimmed it, that would be the conclusion. But I mean – and this kind of echoes Founders Funds’ really only thesis that we have – we are looking for extremely strong teams that are building a truly defensible product. And I think that means that whatever they're building, their strategy needs to have a moat in some way, whether that's an incredible tech insight or some sort of accumulating data advantage. That's ultimately what I'm going to be, in the back of my mind, trying to figure out when I hear any MLOps pitch. I think if someone's pitching and it's a pain point that I experienced as an ML engineer, automatically, the credibility of that pitch goes up. But still, there needs to be a real business plan there and a real strategy. That's what I sometimes, especially now we see this common paradigm – which, there's many examples of it working – of like just very technical teams maybe spinning out of a company or building on top of an open source language. And I think you still need to pay attention to 101 of starting a business. 15:06 Demetrios Excellent. Davis, how do you see it? When you're trying to invest in companies and in the pains that they solve? 15:14 Davis Yeah, I think there are all the basics that apply to any startup, so I won't go into those. But I think some of the unique facets of this market that I guess I think more about. One is “How do you stand out? What's the wedge?” Especially in a market that's as crowded or noisy as this, I think there's a lot of companies that are solving a real problem – it's a real pain point and is kind of relevant to certain people – but it's not building a substantial enough nuance or difference in the way things were being done, or some market shift, that it's not gonna be able to get really huge. And I think, honestly, for a lot of companies, you want to think about how over time you become a platform. So, as Leigh Marie mentioned, Scale is kind of becoming a platform at this point, where they start with a really specific labeling use case, but they were the first to kind of take this API-driven approach to labeling, which I think was unique – some users truly appreciated that. And that gave them this early foothold to expand, expand, expand. I typically want to see something like this, especially in this space. Nowadays, maybe that's “Okay, there are some interesting challenges around edge models. And if I can start by solving one or two of those, maybe eventually it’ll become the framework for deploying end-to-end and the kind of edge or IoT devices.” Or something like that, right? I think other versions of this could be targeting a new user type. A lot of MLOps startups target more of this, like Silicon Valley data science plus ML engineer startup. I actually think there's a ton of opportunity in targeting companies that mostly have people who know SQL, or mostly have people who are just more like business analysts. And so, is there a vertical, or a user segment, where I can start with this one acute pain point, but grow over time. I think I think about those facets far more in this market, then a lot of other areas that I look at, given just the noisy, crowded nature of it. 17:02 Demetrios That's huge. 17:03 Leigh Marie I love the sort of, point solution to platform, can you go across personas – I think another thing that I think about a lot in terms of the MLOps space, just kind of the knowledge that, with ML, you have a few people – autonomous vehicle companies, big tech companies, grow stage startups, where ML is mission critical – they're spending a lot on these problems. And then you have this long tail – people getting started with machine learning startups where maybe it's not as important, but it's helpful for their business – that is maybe spending a little. So which group is this company going after? Like I'd say Scale was going after the former, maybe now they've expanded a bit. But AV was the focus for a long time (autonomous vehicles). Weights & Biases, I would say, is going after the latter. You know, Weights & Biases’ tracking experiments – anyone getting started with ML needs something like that, either buying them or building something internally. So thinking about, if a startup doesn't fit in either one of those buckets – if it's a tool for everybody, or something like that – I'm a little bit more skeptical, just given the difference in sophistication, tooling wise, that these two different groups need. 18:21 Demetrios Such a good point. So we talked a little bit about – I feel like there's a question to be had here that I'm trying to formulate as I speak, [chuckles] which generally doesn't work out so well, but I'm going to try it anyway. [chuckles] And it's around the idea that you have the modern data stack, which got really popular a while back, and I've been talking with a lot of people about the modern data stack, or people ask about the modern ML stack and my go-to, default answer for that is there's no such thing, nor will there ever be such thing. And the reason for that, I've been trying to write a blog for ages about it, but I don't want to just call out the problem and then basically shit all over it and not give any answers as to what you can do instead. So the “what you can do instead,” I've gotten a little blocked on, but I promise that I will get a blog post out about this. And the reason that I say that there will never be a modern ML stack is because there are so many different use cases. You have – computer vision for healthcare is much different than computer vision that is for autonomous vehicles. And they're still computer vision. But the SLAs of one are much different than the SLAs of the other. Then you have something like structured data, or you have a fraud detection model and then RecSys, and those kind of go in the same bucket – but maybe not exactly and there's specific things that you need for each. And then you have robotics, which is a whole ‘nother ball game altogether. Can we even call that ML? I don't know. That probably should be called something different, maybe like robotics. [chuckles] So the idea there is, because there's such a broad market and such a broad thing when we talk about ML and use cases for ML – I really appreciate this point that you're bringing up like, “Yes, find a point solution and then expand out and try and eat larger and larger pieces.” I don't necessarily see a company being able to expand out all the way to where it's like, “Oh, we need this one for sure, if we're doing any kind of ML.” Do you all see that happening? Maybe Leigh Marie, can you tell us, like if you had to look ahead in the future five years – will there be one that will be useful for any kind of ML? 20:54 Leigh Marie Yeah, I think it's an interesting question. I guess, in terms of “Let's just kind of look at the ML lifecycle,” I agree with you. I don't think there'll be one tool to rule them all, like “For every part of your ML training and deployment, you're going to go to this tool, no matter what sort of company you work at.” I think potentially for data, there could be one of them. I just think you're already starting to see that. Obviously, you know, I’m a bit biased, but you're already starting to see that with Scale becoming the de facto, “I need training data.” And I think they have, in all the examples you mentioned, they have customers. So theoretically, you could see that becoming a sort of universal training platform. But then in terms of the complexity of deployment for all these customers, it's just so different. Like AV, you literally have multiple stacks that you need: perception, planning, you have multiple types of sensor data. For robotics, you need: fleet management, and all this other stuff, like debugging and these huge tensor logs and all that. And then for healthcare, there's a lot of, let's say, privacy concerns that maybe you wouldn't see in some of the other categories. So I just think the problem is way too complex. Then also just data right now, it's not… even the things that we think are kind of solved, aren't even 100% solved, right? You see, Airbyte coming up and challenging FiveTran. There's still – even though ETL is pretty standardized – there's still multiple companies in the space. I think, ultimately it just reflects – I think it's an exciting time to be an investor in the space, because there's just so much data, people are now trying to make more and more sense of it, make smarter decisions, and a lot of companies that can be huge. Generational companies can be built. 22:48 Davis Yeah, I think I agree that it's maybe a little bit of a false dichotomy. Right? Like, what is the “modern data stack”? I feel like maybe the simplest definition is just a set of data tools that are kind of warehouse-native – cloud data warehouse native. Maybe you'd incorporate something like, where you have some kind of core ELT-type tool, like DBT is part of that. But aside from that, I think you actually see a large heterogeneity of tools around that, right? Are you using notebooks? Are you using BI tools? Are you creating a custom embedded analytics product? The way you build this periphery actually changes a lot based on companies – some companies have to have very nice or specific tools that are relevant only to one or two use cases and I think it's actually very similar, probably, in the ML space, where some of this upstream stuff around data feature engineering is fairly common across modalities of machine learning deployment, types of models, etc. But as you go later in the stack, it changes a lot. You know, even simple cases, I think, vary a lot as you go towards the end of the stack. There are some interesting companies in machine learning serving, which I think have interesting architectures that are really useful for very specific serving requirements – certain latency requirements, throughput requirements, larger deep learning models, etc. There are a lot of serene cases where you can just get by with a Flask server or something like that. And so I actually don't know if the spaces are that different, I just think [chuckles] there's so much branding and marketing around the dataset modern data stack that it feels more unified than I think it realistically is. 24:18 Leigh Marie Yeah, my favorite thing to joke about with data ML founders is like, “Yeah, what's your marketing term gonna be? Because you need to have something catchy describe your little part of the data stack.” [laughs] So I always like to come up with marketing terms. [laughs] 24:34 Demetrios In MLOps, you can just say whatever you want, and then append “store” to it, and you'll be good. So everything is a “store” these days – you've got a model store, you got the feature store. We got the whatever – what is it? – the evaluation store, is the common one that people have. But yeah, I do like that. I think that's very true. It's marketing at its best. I've heard Joe Reese say that – what happens when you're doing streaming data and you need Kafka on that modern data stack? Is that like the “Postmodern Data Stack”? What are we dealing with here? And then, the next one is going to be the “Renaissance Data Stack” that we have? So it is funny to think about that now. There was something else that I wanted to call out before we move to the next question. And that was, Leigh Marie, you talked about how you have some really strong use cases for machine learning where people are basically all in on using machine learning. And they know that no matter what, either the business is going to go out of business, or we're going to continue using machine learning, whether that's like the thing, the gigantic tech companies, the AV companies, and then you have those tech startups that are using machine learning as part of their business model, and then you have all the rest. And it feels like there's this huge sea of that long tail that is kind of like exploring, but not necessarily all in and I just wonder like, what are all your thoughts on that long tail? It's kind of what we've been talking about – it's so unorganized and so chaotic in those companies. How do you even look at those and tools that can serve that market? Or do you try and stay out of it? 26:36 Leigh Marie Yeah, that's a great question and I think it's something I'm still trying to figure out. Because it's all about, I think, growth rate like, how fast are these companies going to graduate into spending more and more money on whatever tools that they're using? Maybe they're really small today, but a few of them will be huge in the future – and this is just like a common infra thesis in general, right? Like, why cloud providers are successful, why these observability tools like Datadog are extra successful or whatever – you have these few customers that end up graduating and staying with your platform. So it's like, yeah, when is that going to happen? Honestly, I'm still trying to figure out the sort of timeline on my end. I mean, I think you're starting to see tools that certain types of ML engineers just default to, but then you're just trying to figure out, “Okay, well, how much are they actually willing to spend to continue using these tools?” And one that comes to mind is Hugging Face. So Hugging Face – you ask any NLP practitioner, “Where do you go to find the latest models? Where do you go to maybe even play around in a sandbox? Deploy very, very simply?” They'd say, Hugging Face. But yeah, how much are these NLP researchers willing to pay to do that? And will they continue to use that platform as they finish up their PhD and start a company or go to a company or their startup gets bigger or whatever? So it's something that I think every investor is thinking about. But I mean, as we've seen time and time, again, with open source companies, for example, like a GitHub or something like that – lots of usage – usually, you can figure out a way to monetize or to create some sort of uncopyable advantage that's extremely valuable to other companies. 28:43 Demetrios I saw a funny meme that was talking about exactly what you were saying with Hugging Face. I can't remember the exact picture, but it was something along the lines of “Now the time has come where you have to choose.” And it's like, “Do you pay for Hugging Face?” [laughs] 29:04 Leigh Marie [laughs] Yeah, I mean, people love it. So you know? The response is that meme. [laughs] 29:14 Davis [chuckles] The point around paying for Hugging Face is tied to – I think part of the question was this long tail of the ML market. One of the things I actually find interesting about that is, I think the more you target the long tail, the more you go up abstraction levels. So it's going to be less open source, bottoms up, really sophisticated ML engineers adopting tools. Naturally, I actually think it becomes a little bit easier to have a good business model in those cases, where you have less of these concerns around build vs buy, because the build is actually just actually impossible for customers like that. And so, at least on my end, I've been actually really interested in platforms that target more of this long tail of less sophisticated companies, whereas nonetheless, there's a lot of just obvious simple use cases. It's not complex models, but like – everyone in retail has inventory management problems, revenue forecasting problems, things like this, and for supply chain, they all have a lot of the same issues. One company I find pretty interesting in this space is Continual, which is a kind of SQL-first declarative ML platform. It just sits on your data warehouse, but anyone – if you just know SQL – you can basically deploy a production model. So you could also think of it as like a next gen Data Robot, that's more production-oriented, and fits more of like the DBT-style model of working. I actually think there's a huge market for tools like that. Similarly, I think there's a pretty big market for – you have all this growth of these mega transformer-based language models, or pre-trained models or systems like this nowadays, where with just a little bit of fine tuning, it can actually mostly solve the use case for a lot of forms of data and images, texts, etc. I think that's going to get to the point where you can offer really nice abstractions to less sophisticated teams and allow them to do really interesting use cases on top of data types like that. So I feel like we're starting to get to the point where maybe there's interesting companies to be built that really focus primarily, actually, on the long tail of the market. But there's challenges too. 31:08 Leigh Marie For sure. I mean, I'm very optimistic about those types of companies as well. I think, then the question just becomes – you have to build a product that's really easy for these people to use, which looks very different from your typical, ML info product. And then you need to have a real sales motion. You're not having people go on GitHub and [chuckles] adopting the open source version, and then going to graduating into the enterprise paid version – you're targeting a very different type of customer, or maybe types of customer. then it almost becomes… and do these customers even really need ML? Or do they just need better analytics? Right? 31:56 Davis Yeah, yeah. 31:57 Leigh Marie There's always that complexity/simplicity trade off and “What's the optimal ROI for these different personas?” There's a lot of unanswered questions in my mind. I'm confident that really great founders can figure it out. They can pivot, they can create the product and sales motion that works. But yeah, I definitely don't have all the answers there. 32:22 Demetrios That’s huge. Huge. Speaking about open source and how you look at open source tools – and, Davis, I remember you said this earlier – there has to be that wedge. There has to be some way to garner attention. When you're looking at open source tools that don't have the business model figured out yet, like a Hugging Face… Well, I mean, they're a little bit further along, but I imagine the ones who have just spun out of the gigantic company (name whatever one you want) but, how do you look at that as an investment? And you're kind of sitting there going like, “Okay, we can get a lot of users. The community is strong here. But will that translate into dollars?” 33:08 Davis Yeah, it's an interesting question. Open source undoubtedly has a ton of benefits in this space, especially if you're targeting more technical user personas – it lets them try things on their own and you can typically create more of a community around open source projects, which matters a lot in a space where it's important to stand out. Also, for products that are more infrastructure-oriented, it solves this lock-in objection, especially if you're still an early stage startup. Even if you go under, they can still keep running it and that makes it a lot easier to get your foot in the door in the early days. So it makes sense as a model for many companies in this space. What I personally look for is – it's okay if they don't plan to make revenue or monetize for a while, but I want to see some kind of strategy or thinking about what that will eventually be. Right? Because the problem that can emerge is, if you just build open source and say, “I'm not going to think about monetization for a while,” you may eventually realize, “Oh, God! I really put a little bit too much in the open source, but I can't pull it back now because my community will revolt and then I'm dead.” But then you're kind of stuck with nowhere to go. So it's really important early on, I think, to be strategic about “What do I want to be free and open? And what do I want to be in an enterprise or paid tier, even if I'm not going to build the enterprise or paid tier for three, four, five years?” What I have a harder time with is founders who are like, “Oh, yeah. We're just building this and we'll figure that out later.” Sometimes it works. Sometimes it's more obvious what monetization can be. But it's not always clear. 34:39 Leigh Marie Yeah, I totally agree with that. Then also just, I don't really like the mindset of “Oh, we'll just completely figure it out later.” [chuckles] Typically having at least some ideas or some sort of a strategy. Obviously, the first strategy is not going to work or you're gonna have to pivot. Every great company has so many crazy pivots. But yeah, just having an if/then, sort of, going down the rabbit hole of all the ways that this could play out, and the game theory of it is pretty important. 35:13 Davis Yeah. One of the areas where this gets interesting is, I do think – in the MLOps space in particular – you see a lot of these cases where it started with a team who was building out a large tech company where probably, when they first built the open source, they weren't thinking about starting a company around it. Or sometimes you see these really long-running open source projects, which eventually get monetized – maybe Dask is an example of that – where there's so much inertia and community behind the project, but it wasn't tactically curated around the idea of startup formation. I think in those cases, it’s interesting, because on one hand, like, “Wow, this is a legendary founding team and there's such a strong community behind the project” But at the same time, they may not have that same clarity. And I think sometimes in those cases, it actually ends up making more sense to build an enterprise company around the open source that's more adjacent, rather than open core. An analogy I would make is in the front end web stack, like Vercel has done a good job of this on top of Next.js, where it's, “Oh, it's not open core, it's just going to a different product, but very complementary.” So that can sometimes make it too, depending on the legacy of the open source project in the space. 36:16 Demetrios Wow. Yeah, I hadn't thought about that at all. That's a great way of looking at it, because I was stuck in that like, “Oh. Well, if you have this open source product, you just have to figure out a way to do something on top of it,” as opposed to doing something that is very complementary to it, because you know that project inside and out. And you know that there is a huge community around it that's got momentum – it has all of that. Now, changing gears a little bit, again – I want to talk about something that is… this is my theory, and people who listen to the show probably have heard me say it a few times in the last couple of weeks. But it feels like there are a ton of startups happening right now – not just in the MLOps space, although there are a lot in MLOps space – and because of that, because of all this money that is going into the market, it's become really difficult to hire people. And that is almost in a way, because it's easier to go and found your own company than it is to go and build a company for someone else. So I think a lot of incentives are for great engineers to go and build their companies themselves, how they want to make it. How do you all look at that? How do you coach different founders through that if they have to go through it? Are there tips and tricks that you've found for hiring, for creating a magnet that you want to get people to rally behind, where engineers want to go and work with a team and a vision? 37:56 Leigh Marie I really love this question. I love that you asked this question. Because yeah, I think this is on the top of every founder’s mind right now. I have not spoken to a founder where this is not just something that they're thinking about constantly. And I think it also should be on the top of every investor’s mind, when they're deciding whether or not to invest in a company – will this company be able to hire? Because it is such a huge question right now. You're even seeing, I think, a lot more acquisitions of companies that are early stages in order to bootstrap hiring, which is another symptom of this very competitive job market. A lot of macro stuff. But I think one thing that I see a lot of, that's helpful for founders to hire their first team members – honestly, their personal networks and the investor networks of the very early stages of the company. Just, if they come out of a startup where there's a lot of strong tech talent, I think that can really bootstrap hiring, or a school with a lot of strong tech talent. It was funny. At Scale many of the first hires were MIT poker club members [laughs] that's where we all met – the CEO at Scale. But that's maybe to get the first few hires. Then I think, after that, you want to create some sort of unique community, or at least something that I've seen work really well. Whether it's having a podcast, or doing a newsletter, or some sort of competition, or hackathon, or an in-person event, which these days – especially in places like San Francisco – can be rather unique. Or maybe even if you're bigger, throwing a conference, or having a really active Twitter account, or having a programming language that you kind of have a monopoly on, like Jane Street, which is a quant trading firm – essentially has somewhat of a monopoly on OCaml developers, because that's what their stack is. And it's such a unique language. So I think there are all these sorts of strategies that I think founders should be – and great founders are – thinking about constantly like, “How do I curate this brand for the type of people that I want to attract?” And this is, quite frankly, something that I think investors can be particularly helpful for, building strategies around these different sorts of channels. 40:20 Davis Yeah. I can say that in MLOps in particular, relative to other categories, we specifically look for founders who can just be natural talent magnets, like they are some form of luminary, they're famous in the field in some way. Because while I think hiring overall is an issue for every single startup I work with, the pool of talent who know how to build machine learning systems and infrastructures is so small, so highly paid, so easily drawn to the many companies that want to hire them, that I think it's dramatically exacerbated in this market. So I think the first step is, if you're going to be a founder, feel like you have a network you can pull from easily and be a good storyteller so you can recruit people. In terms of tips, I don't know if I have too many more aside from what we're already mentioned. Maybe one other thing would be – I think if you can create some aspirational feeling around your startup that is tied to some concept that really gets some people excited or gets them going, that can be another way to attract talent. I'm just gonna make something up, but… maybe you believe that the future of machine learning is all declarative systems. It's like this “data-first Andrew Ng model” or something like that. If you're the first startup to really brand around that, regardless of what you do, maybe you just find some people who are just like – they believe in that concept, that idea, they want to be part of that movement and so they want to come work for you. I think that type of emotional framing around your startup can be powerful if done right. But, of course, you need to be smart about doing that in a unique, authentic way. If you just throw some random term, it won't work well. 42:01 Demetrios [chuckle] Which we’ve all seen. 42:02 Leigh Marie Yeah, like “Democratizing ML” like two years ago would be an example. More unique “democratizing” anything these days, I just feel like it's what I see on every single company's website. I’m not saying it's a bad mission, but it's just yeah… not something, as you said, that's very unique or probably not going to stir up a very emotional response in who you’re trying to hire. 42:21 Demetrios [chuckles] Yeah, it's not cutting edge anymore. That's so true. 42:25 Leigh Marie Not anymore. [laughs] 42:27 Davis Yeah, it's an interesting topic. I think this is a broader problem, or challenge, for infrastructure startups. I know a lot of people who are very mission-driven and the mission of a startup can really attract them to work there even though the comp is lower and other things – the work is harder, etc. But for a lot of people, it's easy to associate that with a climate startup or a more application-layer startup. It's harder to focus on that if you're at this infrastructure layer. So I think part of what you need to do as a founder is figure out how to tell that mission-driven story, or that aspirational story, in spite of the fact that you are a tool, rather than solving some very specific use case. But I think if you do that well, you can help people realize that actually, these tools still have impact, they have power – they're important in the world. But it's a little bit harder of a sell. So it's more important to think about how you craft and frame that story. 43:15 Demetrios Amazing point. I'm actually reading this book called “Storyworthy” right now, which – I don't know, if you haven't read it – it's an awesome book about how to tell better stories. I figured it's probably good for me, because I talk to people all day. [chuckles] I don't think anybody would ever regret learning how to tell better stories and I think it's great what you said, Davis – have something that can inspire someone and try to make it so it's a little bit outside of the box. Make sure that you have that ability to be a bit avant garde when you're inspiring people. Now, let's close here with this one. What are some things that have surprised you over the last year? Whether or not that is something that is a company that has blown up – Leigh Marie, I think you mentioned Airbyte before and how we're still looking at Airbyte. We thought it was FiveTran that had dominated that market and now Airbyte is all of a sudden a competitor. Or maybe it's a company that you thought was going to do great and they've tanked. What were some surprises in your eyes? Davis, maybe you can go first on this one. 44:33 Davis You know, it's an interesting question. [chuckles] I think the first thing is maybe just the raw volume of startups I continue to see crop up in this space that continues to exceed my expectations and be an interesting thing to think about as an investor. Maybe one thing I'll mention is – I've gotten the impression that a couple of these companies that are focused on more unique deployment modalities in the machine learning space – like robotics, Edge deployments, etc. – seem to have gotten a lot more rapid interest, or traction, than I maybe would have expected a priori. I talked to so many companies who can barely get the most basic model deployed in the cloud. So, from an investor's lens, I've been really focusing more on [chuckles] the basics before you go to more exotic situations where you need to do quantization and figure out how to optimize for the hardware target, and all these things. But it seems like some of these companies are doing very well. So maybe that was a little bit of a surprise to me. 45:32 Leigh Marie Yeah, definitely a surprise to me, I think you said earlier, there's these two buckets that I put ML startups in – either you're serving these very few use cases that are willing to pay a lot or lots of different use cases, or personas, and they're willing to pay some but not nearly the amount as in the first bucket. I have to confess, earlier, I really just thought it was very hard, if not impossible, to build an ML company that went after the second bucket – the long tail. And now, I've seen multiple examples of companies that have been quite successful doing that like, Weights & Biases, Hugging Face – I think these companies have really broken out and are seeing real traction. So that's something that has surprised me over the past few years. Now, there's a ton of startups trying to go after it, so I don't know, [laughs] how much room there is or how fast it's growing to accommodate all these startups. But who knows? Then one thing that surprised me in the negative sense is, I guess – working at Scale, or just kind of being in college, I thought by now, we’d just see so many examples of robotics just out in the world – at restaurants and things like that. It still feels very… There are definitely some and you can call a self driving cruise car in SF Now (if you're on a very limited list). So there's definitely some proof points that things are happening, but it's unfolding a bit slower than I thought it would be. I think, though robots infra companies are very promising, when is that market going to fully be there? So that's something that has surprised me. 47:16 Demetrios I wonder if that's a lot about society, and the societal norms that we have – our acceptance of those things – as much as it is about the companies themselves being able to prove out value. 47:30 Leigh Marie I think it's a combination. I mean, I still do think it is pretty expensive. If you just wanted to, for example, “I'm a warehouse and I want to replace or augment all of my processes with robots.” I do think it is like a large upfront hardware cost and I'm not 100% sure that the ROI is currently there. But yeah… maybe I'm wrong. 47:55 Demetrios Well, this has been great. This has been so cool. If anyone wants to reach out to you all and talk to you about their new MLOps startups, can they? 48:05 Davis Yeah, definitely. 48:06 Leigh Marie Oh, for sure. Oh my God. 48:08 Demetrios That’s it. What's the best way to talk to you? I know you're both on the community Slack, but you're also on LinkedIn, and Twitter, and all that good stuff. We'll leave all the details. 48:18 Davis For me, the best is just [email protected] – happy to chat with anyone exploring this space. 48:23 Demetrios There we go. Cool. Well, it's been a pleasure. I really appreciate both of you coming on here and showing me how you look at things. I'm super excited about this space, obviously. And I love hearing from investors on how they are gauging it, what the temperature looks like from their point of view. So thanks again. This has been awesome. 48:45 Davis Thanks, Demetrios. 48:46 Leigh Marie Thanks for having us! [outro music]