Machine Learning Operations — What is it and Why Do We Need It?
speakers
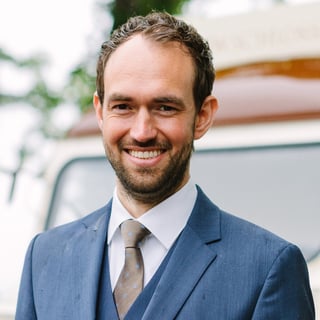
NIKLAS KÜHL studied Industrial Engineering & Management at the Karlsruhe Institute of Technology (KIT) (Bachelor and Master). During his studies, he gained practical experience in IT by working at Porsche in both national and international roles. Niklas has been working on machine learning (ML) and artificial intelligence (AI) in different domains since 2014. In 2017, he gained his PhD (summa cum laude) in Information Systems with a focus on applied machine learning from KIT. In 2020, he joined IBM.
As of today, Niklas engages in two complementary roles: He is head of the Applied AI in Services Lab at the Karlsruhe Institute of Technology (KIT), and, furthermore, he works as a Managing Consultant for Data Science at IBM. In his academic and practical projects, he is working on conceptualizing, designing, and implementing AI in Systems with a focus on robust and fair AI as well as the effective collaboration between users and intelligent agents. Currently, he and his team are actively working on different ML & AI solutions within industrial services, sales forecasting, production lines or even creativity. Niklas is internationally collaborating with multiple institutions like the University of Texas and the MIT-IBM Watson AI Lab.
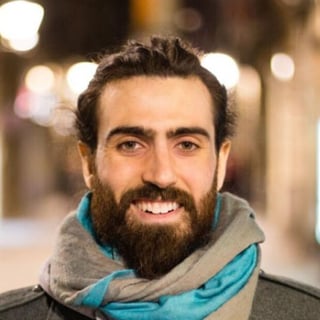
At the moment Demetrios is immersing himself in Machine Learning by interviewing experts from around the world in the weekly MLOps.community meetups. Demetrios is constantly learning and engaging in new activities to get uncomfortable and learn from his mistakes. He tries to bring creativity into every aspect of his life, whether that be analyzing the best paths forward, overcoming obstacles, or building lego houses with his daughter.
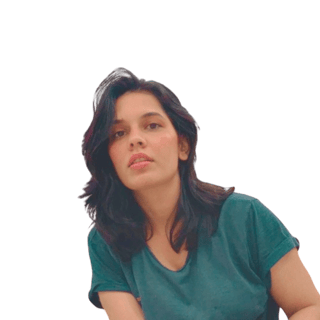
Abi is a machine learning engineer and an independent consultant with over 7 years of experience in the industry using ML research and adapting it to solve real-world engineering challenges for businesses for a wide range of companies ranging from e-commerce, insurance, education and media & entertainment where she is responsible for machine learning infrastructure design and model development, integration and deployment at scale for data analysis, computer vision, audio-speech synthesis as well as natural language processing. She is also currently writing and working in autonomous agents and evaluation frameworks for large language models as a researcher at Bolkay.
Prior to consulting, Abi was a visiting research scholar at UCLA working at the Cognitive Sciences Lab with Dr. Judea Pearl on developing intelligent agents and has authored research papers in AutoML and Reinforcement Learning (later accepted for poster presentation at AAAI 2020) and invited reviewer, area-chair and co-chair on multiple conferences including AABI 2023, PyData NYC ‘22, ACL ‘21, NeurIPS ‘18, PyData LA ‘18.
SUMMARY
The final goal of all industrial machine learning (ML) projects is to develop ML products and rapidly bring them into production.
However, it is highly challenging to automate and operationalize ML products and thus many ML endeavors fail to deliver on their expectations. The paradigm of Machine Learning Operations (MLOps) addresses this issue.
TRANSCRIPT
So, hi, I'm, uh, Nicholas. I actually have two jobs. On the one hand, I'm working as a managing consultant, uh, in the area of data science for IBM consulting. And on the other hand, I'm leading a small research lab called the Applied AI and Services Lab with eight researchers, where we do a lot of research on the interface of academia and industry and where we try to bridge that gap and use novel approaches from theory to apply them to practice.
I rarely drink coffee only once a week, and then it depends what the occasion is. So if it's a socially event, I'll have a cappuccino. Uh, if it's only to get some work done, it's just black coffee. Welcome everyone to the ML Ops Community podcast. This is your host Demetrios, and I am here today with Abby.
We just spoke with Nicholas, who wrote some incredible papers that you probably have seen. They went and they had their moment on social media. If you are all involved in the ML ops world, you probably saw these. The paper is called Machine learning Operations Overview or ML Ops Overview Definition and Architecture.
Abby, what were your take? I love this conversation, but one of my favorite things from this conversation was his focus on the product side of things, where he said, lops is not just about the technical side of things, which is the package of the tooling and the frameworks, but you have to think about the entirety, which goes from the first point when we are really thinking if there's a scope for this project and if there's going to be written on investment for this project.
So good. Yeah, and his definition of ML ops, I've been looking for a clean definition. I love what he said, so keep your ears out for that when you're listening to this, because I could not put it in a better way. I think he articulated what it actually means to be doing ML ops by the. Before we jump into this full conversation, I wanna mention that we have three different newsletters you can subscribe to on our website if you are not already.
Also, it would mean the word to us if you give us a rating and leave a review for the podcast. Yeah. Some of the reviews that we've gotten thus far have just been, ugh, incredible. I will read one of them to you right now, Abby. It is. Worst podcast that I still come back to. So thank you. Whoever, John 75 0 4 is, I appreciate that rating.
Keep 'em coming. We read 'em. All Means the world to us.
Nicholas, we should start with a little bit of how you came to get into ml. I know it's been a windy road for you. I want to hear, uh, the quick summary before we actually jump into the incredible paper that you all wrote. Thank you. So ml, not ml, op. I mean ML and then ML Labs give us the Yeah, you can make it as flourished as you, you give us how you got started in the field, the entire field.
Yeah. Yeah. So, absolutely no. So actually it was, I think back in 2014, I just finished my, my master's studies in industrial management and engineering. Um, and I was looking for an interesting PhD topic. And in 2014, machine learning was a very, very promising field. But still a lot of interesting applications have not been done yet.
So there were a lot of opportunities to work on that. Um, and I was quite open to exploring something new. Of course, it's your PhD, right? So you have three and a half years to work on that, so you'd rather do something that you, that you love. Um, so I thought I'll definitely want to use machine learning as a methodology.
And on the other end then I looked for an interesting applic. And I found one. So I started working with Twitter data a lot, mining Twitter data, uh, building models. I love that. Predicting customer needs in Twitter data. And this was basically my, my PhD and three and a half years went over crazily fast.
And after that, um, I was at this important stage where some will continue doing a postdoc, other will go into, others will go into, in. But at that time, I already started my, my own lab, um, at my institute. So I continued and I found many more interesting applications of, of machine learning. And from that on, I started building my own team.
The team got larger. At a later point, I joined IBM as a data scientist. Um, so. From there on a lot of new avenues started, but it actually all started at one conference where, where I was confronted with the idea of using machine learning as a methodology for, um, for designing innovative products. And that really made sense to me at that time.
Incredible. And so you got to see just the amazing examples of how humanity can be incredible people by scraping a bunch of Twitter data. I'm sure , so did you, I did review a lot of Twitter data, as you can imagine. And uh, as you put it correctly, Demetrius. Quite a few interesting tweets among that . Yeah.
The only thing better would probably be if you scraped a bunch of Reddit data than Yes. The next product. So actually my product started with, uh, scraping Reddit, Facebook, and Twitter, but Facebook closed down its API while I was developing and the Reddit API didn't work as well. Um, so in the end, I was just left with, with Twitter, so I got my fair share of Reddit posts and Facebook posts as.
And did you create any products? You, you mentioned that you, you created some products in the lab. Were they to help companies see if there was buying intent on Twitter? Was it something like that? That's exactly the, the correct direction. So what I did, I, I built an, an artifact, a product which I call need mining.
So what it did is it actually mined Twitter data for customer needs. Um, and I did that for different domains. I think one of the most interesting back at that time were electric vehicles. Um, so I wanted to support in better understanding why are people hesitant to using EVs? Um, is it the range? Is it the price?
The convenience, the charging infrastructure, stuff like that. So I wanted to support actually both society, but also industry a little bit with that to gain more understanding on why are people not using EVs? Why are we potentially not addressing their, their needs? So the product is called Need Mining Uhhuh,
And were there any conclusions on why people didn't want EVs? Yes. I mean, now it feels. They're much more accepted. Right. Especially society is embracing them. But back in those days, and what, when was this? 2016. 20 20 14 to 2017. Okay. And so what was the conclusion there? Um, I mean, most of the stuff is, is fixed by 2022, I'd say.
Um, but back then it was missing charging infrastructure. Mm-hmm. , um, a range that works for most of the people in their daily lives, but not if they wanna drive on holiday, especially not if they're long, if they're commuters, uh, which have to, to go. Four distances together with the missing charging infrastructure.
That was quite an issue. But I mean, this was, these were on a meta level, the main needs, but if you looked on the, the highest frequency, it was always charging stations not working either. Technically not working. Or they were technically working, but your charging card or your charging app was not compatible, and this was a big issue in 2015, 2000, 2015, 2016.
Um, because there's a lot of there. Were you yet to carry with you 40 different charging cards, um, of different charging providers? And then check which one would work with the charging station. Uh, this works a lot better. Um, as of today, uh, I can tell from, from my own. Incredible. Yeah. I mean, there's still some of those complaints, right?
Especially if you're doing a, a long road trip. But let's get into that tech a little bit. You were using NLP to figure out what people were saying and, and extracting insights from that. Can you explain, break down like how you did that? Absolutely. So there are two parts of that. The first part is identifying whether a tweet contains a need, regardless of what that precise need is.
First of all, it's all about identifying need tweets, so tweets containing needs, and then there's the second part and that part. It's more interesting to cluster the needs once we know that there are needs contained. And of course, this is all based on natural language processing, actually very basic, very simple stuff back at that time, right?
Things like tokenization, uh, removing stop words, things like that. A little bit of semantic pre-processing, uh, using hypers, things like that. Uh, but really not too advanced to be honest, and already worked pretty well in identifying the needs, uh, identifying the tweets that contained the needs. And then after that I tried all different things like, uh, unsupervised learning for, clustering the needs into different buckets, uh, having pre-trained models that did that.
And some things work better, some work not so good. Um, I think if I would do it again now with, with the foundation models that you have at hand right now with all the pre-trained models, with things like, like bird, um, I think the results would be much, much better. But to be honest, at that time it was more of a proof of concept.
Can we do this at all? Can we drive innovation with this automatable scalable approach? Previously people would go over to the customers manually, do interviews with them, do focus groups, surveys, and it was all very manual. And I was like, wait. But people willingly express their needs on platforms like Twitter, like Reddi, like Facebook.
Why don't we just use that data that is available, uh, where we have much, much larger set of data. So that brings me to the next question, which is it seems like a lot of work you were doing was around cons, consumer, customer behavior, production. Was that a niche that you picked out for yourself or were there some techniques that sort of interested you to go into these particular kind of applications?
Where is there other applications as well? Like adverse area and all of that stuff was actually coming up around 2017. Cans were sort of a big thing as well. Excellent question. Um, so this has a little bit to do with, with the history of the institute where I did by PhD because they are first and foremost interested in innovation.
Um, so how can we drive innovation oftentimes in an interdisciplinary manner? And it was not really important what kind of tool set you use to drive that. And I was among the first ones who actually used machine learning in, in that group. Um, so this was kind of the angle that I had and. Meant, okay, if I wanna design better product, if you have a, have a design thinking mindset, then obviously you need to understand your customers.
And then obviously this is the data that you will look for is firsthand data generated by, by users and potential users. So this was the angle that I had back then. Nowadays I'm more open, to be honest. So we do work a lot with data from, from factories with iot data. Um, to be honest, it's, it's, it's not a single specific industry or field right now, but this is where I started initially.
I see. Um, so that brings me to one more question on this, which is given you, say you were the first one from your lab who was working machine learning, and particularly in these kind of applications, how was, was it easy or was it hard to figure out the management side of these things? Because that's one piece which is still broken, which is the communication between machine learning existence and the management.
Uh, Is that these companies, because there are sometimes quite some expectations of what machine learning can achieve first and what cannot. Excellent. Um, so luckily I was not the first, but among the first one. So there were two colleagues who did this as well. So we were all struggling at the, at the same time.
Um, and to be very honest with you, I think there were no expectations at all back at that time. Um, because. Of course, machine learning research was already a big community, but it was mostly driven around, um, developing new architectures, developing new, new algorithms. It has, it had less focus on the application side, although this did already happen in the industry.
Research was more focused on, on the algorithms. So in the end, talking to potential customers, talking, um, to companies who could use that, that product, they had actually no expectations at all. They were just like, okay, if it, if it helps at even a tiny bit, um, it does help us, right? Because as of now, there's an entirely manual process.
Now, looking at my consulting work today at, at ibm, the world has changed. Now the expectations are quite high in when it comes to what can, what can machine learning do? And, and this is something that's really important and and great for mentioning that, Abby, is that you communicate what are realistic, um, expectations when it comes to ML products, right?
Mm. And. This is not easy, typically. Well, especially with the current hype around machine learning, and I'm sure people probably see stuff that's like, wow, I created this image out of text, and then they extrapolate out a little bit more and they're like, you know what? We'll just throw some AI at it and that will solve our all our problem.
Absolutely because the tools become so convenient, more and more, um, like, and I mean, that's also a great part, right? That that's awesome. I mean, this is a technology that, that a lot of people should, should be using and experimenting with. I mean, this is great. It hopefully helps with the adoption of ML products, but in the end, it's exactly what you just said, right?
It, it raises some expectations that are sometimes hard to meet. And as I work a lot. German SMEs, small medium enterprises. Um, it's, you, you either meet two, you meet two types of people, right? The, the one are so hyped about that and think that, uh, ML products can solve all the problems, uh, regardless of the data that they have, and the other ones are highly skeptical.
Um, so it's always interesting, uh, to, to work with these people and to get realistic expectations. So what we were also wondering, Let me just, um, grab this before we jump into the paper. I wanted to, um, ask about like, One thing that comes up a lot in the lops community is budgeting, and when you are doing production machine learning at scale, there's a lot of times that people will try and figure out ways to bring down the cost because it seems that when you're putting machine learning into production, whether it is the cost of the engineers that you need, or it's just the AWS spend, it's expensive.
Have you looked at that and figured out like how there are ways or tricks or special secret sauce that you may have? That's actually one of the main challenges that we are facing right now, because, I mean, and we will get into that, right? But if you really wanna excel at ML ops, then this requires different roles, right?
And an interaction of these different roles to be really successful, to really operationalize ML products. Now, on the other end, this means. A lot of costs because you might need five people, six people, 10 people, 50 people. Um, and then on top of that, of course, are the computational resources that are required.
And this is really an issue right now because before we start a project, we need to convince the customer that it's worthwhile exploring that and. Depending a lot on what experiences they already made with ML products. Some are more positive, some are more negative. They wanna know what the return of of investment is.
Um, and this is really interesting. And to be honest, this would be something maybe for a different podcast, but it would be really interesting to drive more research in that area. Hmm. How can I be really good at estimating? The value of ML products at a very early stage, how can I make this tangible to the customer?
What we typically do is, um, and this is part of the ML ops paradigm saying, um, whatever I build, even, it's, even if it's a tiny proof of concept, um, you build it in a way that it's gonna be a productive, scalable from day, day zero on mm-hmm. . And in my experience, what's really, really helpful, Show something shiny at the very, very early stage.
Um, so the customer really can see where this is going, uh, becomes hooked even if your model is not working properly yet. But showing something early is really, really helpful. And I mean that tools. As of now, which help us in doing these, these rapid development cycles, and in my experience, this really helps, uh, then to secure the budget that is needed to, to really build a successful ML ops team.
And then in the end, a successful ML product. Oh, I love that. Okay. This is something really interesting that even the high interest, uh, high interest debt paper, I think that's one of the most popular papers in the field, given it was quite early and ahead of its time with all the debt that accumulates from, um, deploying these machine learning models, production and the changes that happen when it comes to the data sources, data dependencies, and all these things.
So that brings me to your paper right now. Um, Researched quite a lot of articles, talked to quite a lot of people. What was the entire process for you like and what inspired you to work on this paper Specif. Yeah. Um, so first of all it's, it's a team effort, right? So there are two po, two more people that should be mentioned here.
Um, Dominic and, and Sebastian. So the three of us, we are the co-authors of that, of that paper. That's a humble man right there, making sure that we know there are two others credit. You can't take all the credit for it, but they didn't. I think when I reached out to. To see if they wanted to be on the podcast.
They didn't respond. So for now we're gonna say it was all you , but I like that you say yeah, no, no, no, no. There were two other people. Let's not forget that. No, of course we internally coordinated who, who will do the interview, but uh, yeah, no, that's classic. So, um, yes, apart from me being humble, um, To be honest, I I, so I'm, I'm a bit of an, of an oddball in both worlds in the academic space because I also work at a data scientist at a large American company at ibm.
And on the other hand, for my fellow data scientists, I'm a little bit odd because I do research and teaching at, at a large German university. Um, but to me personally, this is my dream job because I get to. What's currently happening in industry. But on the other hand, I also have the tools and the theories that I think are oftentimes really helpful in addressing a lot of these, a lot of these problems.
And ML ops for me was a phenomena that we did use already in projects in terms of it, it's basic principles, but to me it was never really clear. What is it all about? I mean, what are its core principles? What are the processes? What is the architecture? I had a rough understanding of that, but it's not like if you, if you would've asked me, it's not like I could have told you immediately.
Right? So this was something I was not really satisfied with. Um, more, more the academic of me, less the, uh, consultant. And then luckily, uh, so I knew that already. I, I want, I knew I wanted to, to write the paper on, on Emma ops and I knew that I wanted to do interviews to do a literature study and a two review and combine all of these three.
Um, and then I met one of my cos Dominic, um, and he was actually searching for, for a Master's thesis. Um, and he wanted to do something on lops. So I thought, okay, well, Perfect. This is the perfect match. Um, let's, let's do it together. And that's how it all, all started. Um, and then we first, uh, dug deep into literature and surprisingly we didn't find a lot.
I mean, of course there are is a lot of literature on ML ops. But when it comes to giving you a good introduction, defining principles, roles, components, architectures, um, there are books which are 300 pages long, but no one can read that or will read that, especially not novices who just get into the topic.
And I would consider myself a novice even, even now. Right. So I think that was, that was something that I, that I, that I wanted to do. And from the, from the literature that you saw, I think back, but when we started, I think none of the papers was peer reviewed. Right. So they were all on, on, on archive or, yeah.
Um, other, other, other service. Right. Where you, you would find open science and I, I thought this was really interesting. Um, not that they, not to say that they're less worth because of that, but that does show that academia was lacking behind in, in, in researching that pH. Um, which even motivated me more.
Okay, this is now the best reason to start investigating that. And after reading all the literature and a lot of that was helpful, we even thought, well, but there's still a gap. We still, we as now speaking as a practitioner, we experience ML ops, um, from a lot of other different aspects. There are a lot of things that are not mentioned in literature uhhuh.
So we definitely need to talk to people who do that on an everyday basis, either from tech C. Or from customers of these tech companies that use these tools. Um, yeah. And this was a setup for, for the study. Yeah. You had to set the record straight in a way. And I think you all did such a nice job with it, because when it first came out, I literally saw it everywhere.
Whether I was looking on LinkedIn or on Twitter or in our moobs community, slack, it was being shared like wildfire. It was just going off, and so I think it resonated. It really like it hit a vein with a lot of the practitioners out there because you put into words things that we had intuitively been feeling and.
But you were able to articulate it. And so for that, I think y'all did a great job. And I'm wondering now that you did that and you have a definition of what ML Ops is, what, what do you, how do you like to say it when someone comes to you and they say, what is ML ops? Because I, I have my definition, but I'm still like trying to figure it out to be honest.
And so I think the, um, I would love to hear what yours is. Yeah. No. Excellent. First of all, thank you so much. Uh, I, I really appreciate that. And I think part of the, the success, if you wanna call it a success, was that I had the outside perspective in not being too deep into these, these areas, and I think that that really helped.
Now, regarding your question on a definition, as you can imagine, this was one of. M things we discussed the most when writing that paper. Um, so we used a lot of time discussing that. And I mean, in the end you come up with a final version, but there were so many other versions of this, of that definition.
In the end, we tried to have one specific focus in that, and that is, let me try to put it in words. Reading out the definition . But every, every machine learning endeavor should result in a machine learning product, and this should be the ultimate goal. So there always needs to be a product in the end that is then maintained, that is continuously run, continuously developed, improved.
But that should always be the goal and everything. Everything that ML Ops does as a paradigm. It should support that claim. It should support the process of developing. Robust, um, oper, fully operationalized machine learning products. Now, this is not a definition, but this is the goal. And I think if you have that perspective on it, then a lot of other things come into place because then suddenly you're gonna ask yourself, so what do I need in order to accomplish that?
Then suddenly we can talk about the principles required, the roles required, um, how do I instantiate? Principles at the interface of, of, um, of the, of, of the role and of the architecture, and so, For me, that's it. Develop, it's, it's a paradigm for successfully developing ML products. Mm. I love that. It's starting with that end state and realizing we want to get here whenever we're doing any kind of machine learning, the goal is that we actually are extracting value from these machine learning models.
And it's the trope. Now, the common trope is that it stays on somebody's laptop and is in Jupiter notebooks forever. And that's why I think a lot of people, Resonate with the idea of like productionizing machine learning. And so I really like, and I appreciate the fact too, that you are not specifically calling out like, oh, lops is these set of tools or these set of pipelines that you set up, but also there is that organizational level involved in the processes that you need.
And I'm really like, I, I think that a lot of times myself and in the lops community, we sometimes get wrapped up in the tooling and we make it, we turn lops into a technological problem. But it's not only that. How did you navigate? That idea of, oh, well Lops is just a set of, you know, you set up these pipelines, you use a few of these off the shelf tools or grab yourself some, uh, vertex AI or SageMaker and you're good.
Yeah. Um, , that's actually an excellent point. So of course we could have gone that way. Um, and if you read the paper, you will. You will see a few names of tools being dropped, like humanities, um, red Hat, OpenShift, things like that. Right. Um, but for, for, for me, well, it was really important to have a sociotechnical perspective on that.
And this is because again, the institute I'm coming from has a focus on, on innovation. And it always says, we cannot only look at technology. We have to look at the socio-technical systems, right? We have to look at the combination of technology, people, and organizations, and only if we have this holistic perspectives on new phenomena, we can really understand them.
Because of course technology is not isolated. Technology will always be used by people within organizations and only by having, having that view, you can really, really understand that. So when we start the project, it was really important for us to not have a strong focus on tools, but always think on a meta level what are, so we do have these tools, but what.
The shared principles behind these tools, what do they have in common? Even when we, we did the interviews, right? We, we had, uh, the, the transcript of the interviews. We had to manually code all of this, this data. Even looking at that, at all of these, these answers from, from the experts within the field, we always try to abstract from that and say, okay, but what is the underly?
Meta level here, what can we, what can we say? Because what you observe in a lot of papers is that technologies come and go, even the precise ones. But what we wanted to do is to have an understanding on what is the, the general idea of the paradigm. What can be still. The case, even in five or 10 years when there are new players, uh, emerging, when old players are not there possibly anymore, right?
This was always what we were, we were aiming at. But to be honest, we had to force ourselves a lot. Uh, not to mention too many tools, not to be specific to, for example, Ernis, but just try to abstract from, from that. On the one hand, but on the other hand, also to keep in mind the organizations and the people that, that, that, that were using it, because that's something that I actually observe on a daily basis in, in a job as a, as a data scientist consultant, is that, um, our customers, they might only have a few data scientists, and then this single data scientist has to be the software engineer.
Oh. That has be the story machine learning engineer. Um, and this is really hard. And, and for, for an organiz. First of all, they need to understand that an ML ops paradigm requires multiple roles in order to function well. Um, but this is something that is not necessarily known to the organizations and we, we need, um, A shift of of, of, uh, expectations there.
One thing that you mentioned earlier of what is doable for a single data scientist and that in order to be successful, we do require amongst others different, different roles with distinct, distinct, um, characteristics and goals. Wait, so when you were doing these, uh, interviews, the user interviews, please tell me you were recording them and then putting them through the tool that you created to figure out or did that, that would've been great.
Uh, you didn't think of it, man. You should have included me in this project. I would've given you all kinds of insights, . But it, it brings up this, this idea, which I love and you. Are speaking of, and it's, it's almost like if we take a step back, I find it fascinating because Abby and I just recently interviewed, um, Simon, who.
Talked about how, and he's been doing machine learning since I think like 1994. If I remember correctly. Abby might be able to fact check me on that, but he was talking about, we asked him, one of the questions that I think Abby asked was around what a team composition should look like, what we should be thinking about when we are starting our projects for machine learning.
And he came back very sternly and. The only thing that matters in the beginning is you figure out the ROI of this machine learning endeavor. Yeah. And then you can figure out how many data scientists you need or how many data engineers you need. Machine learning engineers, whatever you need, you will figure out because you can see what the data's like, what all that, uh, is going to amount to after you recognize how much money it's actually going to make.
Yeah. I can relate. A hundred percent of that from a practitioner's perspective, um, because of course you need to make sure what is the return on invest that, that, that you will receive with. An ML product, and we talked about that earlier, right? How do, how, how can I do a good job in that? How can I be precise at calculating a reasonable roi?
But then on the other hand, also to make sure, um, that I can assign the required resources to what is needed in order to reach atri. So it's kind of a chicken, chicken, egg problem. So from a petitioner's per se, effective, Agree saying that, of course, budget is everything, because if we don't have the budget, maybe there's only one person which has to fulfill seven roles that would be required in theory for a successful lops project.
But that's then just the case. Now, the luxury that I have right now is to lean back and say, Well, but from an academic perspective, uh, of course these are the required roles and this is what the architecture look right, but putting it into practice, I, I can fully, fully, fully agree with them. And that's why I think typically, um, the most successful projects that we did in, in, in, in the realm of lops, um, were not the first ones with that same company, right?
So we would typically, With small ones with the company, showing some successes, um, showing that they would actually receive that ROI that we promised earlier. And then a full blown and ops project, like we would describe it in the paper would be the fourth or the fifth project at that stage. And then we would have the distinct roles and then, All of that worked out.
But of course, this is kind of the reality check on these, on these problems, and again, I have the luxury sitting here and saying, well, look at that paper, and that's how it should be done in theory. This is interesting because I was actually looking at the figure that you've given in the paper, which is basically end to end lops architecture.
And one of the things I've realized, at least in my personal experience working in the industry is a lot of people just expect one person to annoy everything. So I came from like a research background and everybody was expecting, Hey, come do the engineering and everything. And that is still an expectation which people have and more and more c.
Now trying to define this new rule, which is basically called full stack data scientist or somebody who, even if they can't do everything, but at least they're aware of the entire process end to end. So I just wanted to ask you a little bit about that one, which is you said, There are different versions when it comes to working with these teams and there are different stages.
So could you break down these stages with me and talk about what kind of rules, uh, are the companies looking at, these SME companies are looking at when it comes to different stages of the project, and how does the team increase in sizes after different levels? Yeah, so definitely, I mean, this depends a lot on the organizational setup of the, of.
Data science teams, AI teams, whatever they're called in the respective um, company. Um, One typical phenomena that we observe is there's a designated data science unit, a center of excellent or a center of of competence. Um, and then they work together with, with the business units. In some cases they even have satellite data scientists sitting in the business units.
And then you have a core center or a center of data science. Um, and in the first stage it's all about showing. Um, you, you don't have time typically to think about the different roles, although you should. You should, but you do. It just doesn't work. Um, so you need to show some ML products which actually generate value.
And this is the first success criteria, um, from, from, from my point of view. Right? So you typically start with a few data scientists, and I'm just gonna call them data scientist. What they do is they are data scientists. They're an ML engineer, they're a backend engineer. They're the data engineer, the DevOps engineer, and the software engineer, but all within one, one person.
And then if you were successful with your first ML products, then at some point in time, um, they get more headcount. And then of course, the question. Who is that new role gonna be? Right? Who's need the most? Another data scientist, another ML engineer, another software engineer. And this is one of the most interesting phases of these, of these projects.
Again, from an organizational perspective to say, how do you prioritize now the different roles? And how do you, um, how do you do the setup for a successful data science? Um, and now again, I didn't do an empiric study on that, so this is only my, my personal experience. But at some point you have reached the level where all the gears are, are, are working, right, where all the roles are collaborating, where standards are established, where workflows are established.
And then of course, this, at this stage, uh, your products become scalable. They become fully, fully, uh, fully usable products. And that's the interesting stage. But to be honest, a it's really hard for me to say, when is this stage where everything's working flawlessly? And what's that, what's that breaking point?
Right? What's the amount of people you have? This is really hard to say, but it's interesting. It's really interesting to look at these different stages. I would. And how do you define success for different kinds of data science projects, which is, um, so the case, given the fact once the project has been deployed in production, is it, is it just, Hey, the model works and it predicts wherever you want to predict.
Let's say it's a housing thing and it has done that job, or let's say after we start deploying it in production in. A lot of things get involved, especially when we stop working with streaming data as such. So it takes a lot of time before we can monitor and do all of that part, which is the later stages of managing the model and dealing with the drift and all of that.
So at what point do you start looking and defining your model as a successful model or the project as successful in saying, okay, now let's move to the next stage. And we've done what with ails? That's, that's highly individual. Um, as you, as you might expect, and there are a lot of discussions on that depending on the business unit you're working with, the customer that you're working with.
For some, it's only performance, right? In a supervised machine learning model, it's can we continuously achieve that performance in, I don't know, identifying anomalies, um, predicting price. Whatever, things like that, and it's only performance driven. Um, I think it's debatable whether that's that's meaningful.
But of course, companies think in KPIs, they think of numbers, and that's, that's the way that they approach it. So that's, that's one way that you can do it. But to me, a lot of ML products also deliver value on other, on other areas, for example. They give you meaningful insights, what, whatever that means, right?
I mean, this is, this is, this has a lot of perspectives to it and it's, it's hard to say. It definitely gives you insights into your own operations. Uh, even though if it might not reach the performance you once aimed for, it did give you some interesting insights and most of all, it is a very good reflection of your business in many cases because, because it does show you what data do you have, how valuable is that data, even if you are ML product in the.
Turns out not to be successful. This overall process that we also go through in the, in the paper and the workflow, um, reveals a lot of insights on your data quality, on your current stage, um, as a, as a company when it comes to data driven products. So, It's really hard to measure success with a single metric, and it really depends to be honest in the end, um, on the, on the personality of the business stakeholder, because some do understand that and they say, um, Hey, if we get insights into a few anomalies in, in, in our data, this is immensely helpful.
What others say? Say, Hey, we. A performance of, I don't know, F1 score of 90%. And if we are below that, that's terrible. And if we are above that, that's awesome. So I think it's, it's, it's really hard to say, but I think that's a discussion we should have. What makes ML products successful? Is it only a single performance metric or is there more to it?
So that would be really interesting to, to explore further. Also, research wise, , that's your next. There you go. Here we go. So speaking of next papers and all that good stuff, I mean, what are you up to now? You've been doing this paper or you, you did this paper now you mentioned that It is, it's being peer reviewed and hopefully in a few weeks, maybe a few months, it's actually going to be.
Published and put out, but then you have other stuff going on, like your day to day, which involves cheating in triathlons. I hear you. You like to, uh, skip the course and then win what is going on there? Give us the breakdown on that story. On the triathlon. Okay. . So Demetrius of course, uh, required a fun fact about me.
Uh, so I entered that I, that I cheated at a, at a triathlon, not knowingly to be honest. Um, so it's actually, it's actually a, a short story. Uh, so I went to, I think it was actually my first triathlon and I was late, right? You have to bring your bike and get into your swimming gear, things like that. Uh, I had to find a parking spot, so I was really, really late for my cue.
What that meant is I missed the entire introduction where they tell you. Uh, okay, now we swim here, then we bike there, then we run there. So I missed that entire part, and I just came at the very second, um, where the people jumped into the water. That's when I entered, and I also jumped into the water. I did my swimming.
Um, I did my biking, and after the biking, I felt. Well, that went really, really well. Uh, I didn't have any gps or stuff like that, so I was just, just doing the bike tour. Uh, I changed into my running gear, started running, and then I, I, I recognized that I'm, I'm, there's no one else there, right? I'm, I'm really the, the top.
I did feel, I did feel, um, I mean of course it was exhausting, but I was like, is this something feels off? So I walked into the stadium, the people were applauding the announce, said, this is incredible. Uh, Nicholas p coming in, breaking a world record, this is his, his first triathlon, and you already. First place people were going crazy.
And at that point I felt, okay, something is wrong. I mean, something, something went wrong here. Um, but you didn't stop and say it then you crossed and finished. No, none of that time I, I ran through in the stadium. Um, and, and the celebrations were later, right? Because. All people had to get in. Um, so I did this together with a friend of mine and he came in, I think 20 minutes later or so, and he was like, wait, you're already here.
And typically when we were training, he was, he was the more athletic one. So I, uh, so what he told me is that in fact, the instructor said, You have to go to the bike route two times, not one time. Um, and then change into the running gear. Uh, so, but I didn't hear that and I didn't have any gps and, well, I thought it was all right.
Um, so I was very ashamed after that because obviously I then, I then cheated and at some point I had to tell them and they figured it out, I think themselves at some point. So I, uh, I didn't even be honest. Did you still pick up the medal and the trophy ? No, to be honest, I went, I went home. I still do have a screenshot of, of, of that placement.
Uh, but I was actually very ashamed after that. Uh, so that was actually my first, uh, triathlon experience. Um, and yeah, apparently first and last, not last to be honest, but, um, I, I did cheat, so I, I feel bad about that. And now it's public. Um, yeah. You've been. From any sanction triathlons? No. They think you're on EPO or something.
You're doing drugs like Lance. But anyway, aside from cheating in triathlons, what else are you up to these days? Yeah, I mean, of course ML ops is not the, the only piece that I'm, that I'm interested in, but. What always interests me is this combination of academia and industry. So what tools, what methodologies, what knowledge do we need to bridge these two worlds of people working on the best algorithms in the field, but on the other end, on the people using it in, in, in, in practice.
And one thing, I mean, there are multiple things that I'm working on. I think. There are, there are three ones that I find most interesting and I'll try to be, try to be quick about them. Um, one is actually, um, inspired by Andrew, uh, and g um, which is called data Centric ai. I dunno if you guys heard about that, but I think it's, again, it's kind of a paradigm.
It's, it's a really. Um, really great idea because what they say is in the past we had a very model centric approach on building ML products. Um, so someone gave us data, we got the data from somewhere, and then we started to build the best possible models on that. And some models were 0.1% search points better than the others.
And we did competitions, but that's not the way that we should think about it. And it's actually something that we also put into the paper and I. It's, it connects well with, with ops because it says you have to look at the holistic picture, right? Um, don't take the data for granted. In some cases, for a factory, it might be easy to simply implement an additional sensor at a stage where it would give us a lot of insights.
So think of that holistically, right? Not only think about what it can do in the model part. But also think about what he can do in the data part. Even if it means, and I know this is strange for computer scientists, but even if it means going into the field and changing a few things around, and I think if you have this, this, this mindset, this changes how successful your products are in the end.
Because sometimes it only does require one additional sensor here or one additional camera here, and it would give you all the things that that you need. So I think this is a very promising field of. A data centric ai, um, not necessarily that you need new methodologies there, but that you just make sure that people working on ML products have this, this viewpoint not only doing hyper parameter optimization, uh, to the last bit, but also thinking about the, the, the overall part.
I don't know. What are your thoughts on that? Is that something that, uh, that you think about? Well, I think that's the beauty of this industry, right? Because we get to work so closely with the business side of things. The machine learning is so tightly coupled to actual business outcomes. And then, like you said, you can actually go into the field and tinker.
Things to see if you can produce better data. So that just opens up so many different avenues on how you can solve problems. And for engineers who enjoy solving, solving problems, it's uh, the world is your oyster. . Absolutely. Absolutely. Yeah. Yeah, that's that's so true. Um, maybe one, one more thing, um, that, that, that I really like, um, and this really applies to the, to the ML products, once they are, once they are in place.
Um, so in the past I have been like, Two, and I'm simplifying here, but there have been two types of people. One saying, okay, all the ML models at some point of time will take over all the jobs in the world. They will, uh, you know, become, become the best predictors in, in every task that we have out there.
And on the other end, people saying, um, ML products or more general AI will never reach that, that, that stage. And I. I think there are arguments for both cases, but what I do observe now in both research and industry is, is, um, a collaboration of of human agents and machine learning agents and. This, this phenomenon of human AI teams is really interesting because there are so many design choices that you can make in how to present predictions to humans.
Um, how do humans interact with these predictions? How do we explain them? Things like that. And I could talk about that for ours, but I think one of the most, Interesting or important aspect that I myself learned in that is that we do not need to build for performance, but we need to build for complementary.
Um, because what you do see is that humans have different strengths. Than the machine learning products. But right now, typically the machine learning products are built in a way to be good at everything. But I don't think that's the way we should approach this, this hybrid intelligence. But we should say, how can we make the best out of these two entities?
And if we start specifically designing ML products for complementary, I think that would. A huge, a huge change, right? Um, because we did some, we did some studies where we, we can show that the, the, the complementary team performance, so the, the performance of both entities together is better if we aim for that complementary, because what's interesting is that in many cases you have an AI product which reach reaches the performance of 90%.
Why would you need the human in, in, in that still, right? Because a human on paper might reach, I dunno, 85%. But so if you simply combine them in, in many cases we still, the, the, the, the, the top that we reach is still this 90% of our, of our AI product. But if we design for complementary now, We can use the strengths of both our entities and maybe come even above what the top AI performance or what the top human performance is.
And I think this is really interesting, really promising. And what I really love about that is this idea of machine and human working together collaboratively on a task instead of saying, oh, the machines will take over or the machines will never take over. Um, and I think that's great and I think there's, there's a lot of stuff to be done to understand better.
How this complementary works, what are principles that make it work? So that's something I, I'm really, um, motivated. Interesting. Cause about three years ago I was applying for a PhD in all similar, which was, uh, doing some work around assisted living, but sort of combines machines with humans to sort of help us lead their lives.
And that comes a lot of. The applications were more so in medical healthcare and such, working with depressions or working with autistic patients and all of that. Anyway, uh, so one more question I'll ask you on that one. Uh, what does a day to day and Nicholas life look like? It's really interesting because typically there's not, not the day to day, but I do have two jobs, right?
On the one hand, I'm, I'm, again, managing consultant data science for IBM consulting, so I do consulting work. Um, on the other hand, I'm, I'm leading a, a research lab at the Cal for Service Research Institute, um, where we have aid full-time researchers, so, . Um, I'm doing customer work. I'm doing teaching, I'm doing research, some admin stuff, obviously.
Um, but that's what makes my days interesting because it's, it's that mixture. I think if I was only a researcher, it would be too boring and I would fear losing that grip to reality and industry. Um, on the other end, if I would do only consulting work, uh, I wouldn't really feel, feel satisfied as well. So my typical day is a mixture of doing lectures, um, consulting with PhDs, um, and then having some, some workshops with, with customers.
And typically this. This works really nicely because I can use a lot of knowledge that I generate in the research world. Um, but on the other hand, I also know what the problems in the real world are and what we should approach next. Um, yeah, so I mean, I'm, for me, that's my dream job for someone who's around you, where is one lecture that they can drop in, given by you can shop and given value, if any.
You mean like, like in like a public lecture? A public lecture could be at university. I mean, uh, people sometimes just walk in. If somebody wants to attend one of your talk. Where do they go other than, other than our podcast obviously . That's a good question. Um, so because I do come, come around quite a lot, so if you're in, in South Germany, uh, and you are close to, close to k i t, um, this is typically the spot where, where I hold lectures.
So if you are at in Kru, which is a beautiful town, In southwest Germany. Um, then, then feel free to drop by at, at k i t come to a lecture, or even if you don't come to a lecture, uh, happy, happy to say hi if you come by my office. Nice. So that's probably the, the, the, the physical place where you could be.
But apart from that, you can reach me via LinkedIn, Twitter, and the, the typical channels. There we go. Don't say it too much. I'm close to South Germany, so, uh, I'm right outside of Frankfurt, so I may drop in on you. Beware Now, do com. Last question that I've got for you is what I find fascinating right now, there's so much advancements and so in re in the research field, it feels like things are going very fast and they're coming out with new different papers.
Every week. I know we've talked to some people about this and how back in the day in 2019, you could keep up with all the deep learning papers that came out. Because there was maybe one per week or one per month, and now you definitely cannot just because they're coming out so quickly. And you also see all kinds of wild stuff happening with foundational models.
And then you've got the different piece, which is you're in the industry and you see what people need. How do you look? That gap between what is coming out and the cutting edge of research and then what the companies actually need. And potentially, without sounding too cliche, like how do you feel about the foundational models that are coming out?
Do you feel like those are going to be able to fill some of the gaps that we have when doing machine learning in the industry and not just be for creative types? Excellent question. Um, so let me answer the first part. So this is actually one of my main challenges in my, in. Daily job because the amount of papers, even if you only look at peered ones is too high for a single person, even for an institute to, to read, to comprehend, to reproduce it, it, it simply doesn't work anymore.
So that's something that I'm. Struggling with a lot is to keeping up with the, with the pace. I mean, it's a positive thing that so much is happening, but for an individual it's, it's really hard and can be very, very overwhelming. So this is something I really, honestly struggle with, keeping up with it. And it does happen sometimes that people in a conversation mention some topic that I've never heard about.
Um, and for them it's, it's completely clear what that is because that's their niche, that's their gap. But it's, it's becoming really hard. So, um, I don't, to be honest, I, you are not asking for a solution, but I don't have one. It's, it's really hard. and I, I, I do follow a lot on what's posted on LinkedIn and Twitter, but if that's the best way to go, or PhD's approaching me with, with papers, I mean, that's how I do it.
Uh, but do you wanna get a little meta real fast? I'll tell you, I saw a foundational model. I think it's. GTP three, and it is summarizing papers that come out. And so it's using machine learning for this exact problem, which I, I find a little bit meta, but Sorry, I cut you off. No, you did not. No, I mean, that would be the answer, right?
I mean, here we have the foundational models are, are gonna solve all of our problems. Um, but now that's probably not gonna be the case. So, um, so coming to, to, to, to found foundational models, um, I. I do believe that this is exactly what is needed, but to be honest, more in the industry than in, in, in, in research.
I think in the, in the research field, um, still there are gonna be a lot of specific models. I mean, also in industry there will be still a lot of specific models, but I personally. Really appreciate that we have this trend towards more generalizable models that can then be used on a lot of, lot of different tasks.
As of now, I'm having a real hard time on saying whether this will be successful across all different areas, and I also don't feel. In the position to say whether the current ones are, are, uh, are having, um, the, the, the best effects. But overall, that's something I'm really, really interested in. Actually, that's something we do together with, with IBM research as we speak right now because we're trying to build phone foundational models for, for climate disasters.
Um, so Oh, incredible. And we do believe in, in. But as of now, it's really hard to say if we will succeed, although I do hope for us being successful in that. Well, best of luck to you, man. This has been awesome, Nicholas. Thank you so much for coming on here and telling us all about your life and your paper that you wrote and your cheating habits on your triathlons.
Everything has been awesome. Dude, this is so good. So we'll end it there. Thanks again. Thank you. Thank you guys. Really appreciate it. Thank you, Abby. Thank you. Thank you for having me and inviting me. It was, it was a pleasure. Thanks so much