MLOps + BI?
speakers
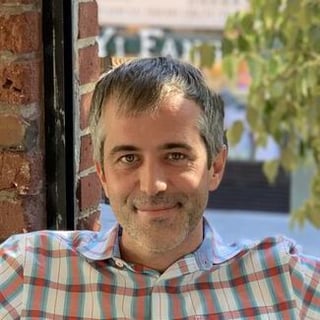
Maxime Beauchemin is the founder and CEO of Preset. Original creator of Apache Superset. Max has worked at the leading edge of data and analytics his entire career, helping shape the discipline in influential roles at data-dependent companies like Yahoo!, Lyft, Airbnb, Facebook, and Ubisoft.
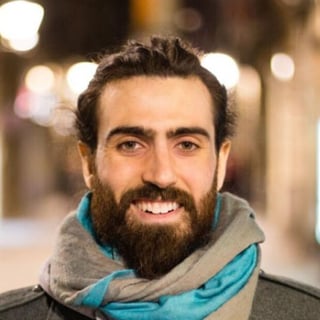
At the moment Demetrios is immersing himself in Machine Learning by interviewing experts from around the world in the weekly MLOps.community meetups. Demetrios is constantly learning and engaging in new activities to get uncomfortable and learn from his mistakes. He tries to bring creativity into every aspect of his life, whether that be analyzing the best paths forward, overcoming obstacles, or building lego houses with his daughter.
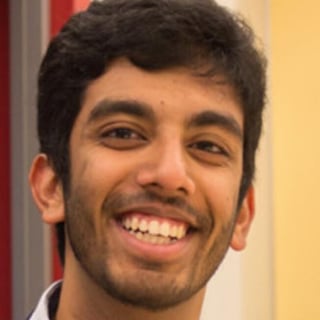
Vishnu Rachakonda is the operations lead for the MLOps Community and co-hosts the MLOps Coffee Sessions podcast. He is a machine learning engineer at Tesseract Health, a 4Catalyzer company focused on retinal imaging. In this role, he builds machine learning models for clinical workflow augmentation and diagnostics in on-device and cloud use cases. Since studying bioengineering at Penn, Vishnu has been actively working in the fields of computational biomedicine and MLOps. In his spare time, Vishnu enjoys suspending all logic to watch Indian action movies, playing chess, and writing.
TRANSCRIPT
Quotes
"One thing that makes sense is the data as gravity to do it right. It's a heavy thing and data wants to be together in one place. Ultimately data wants to be joined, unioned, and brought together, to bring it all in one place and call it a data warehouse, data shack, or whatever you want to call it."
"It makes sense to take all your data and bring it to one place where you can make sense of it. Does it make sense to use the compute resources inside the data warehouse to do ML? Bring the compute where the data is, bring the data where the compute is."
"It does make sense if it is on a cluster on Snowflake or a big query cluster to bring the compute right there. It's easier to move the compute than moving the data."
"No one really knows the lost art of data modeling very well."
"In dimensional modeling, they decouple what is the fact that you have fact tables and dimension tables. What we see in ML is people want to have these more feature stores so you take an entity like a user and put all the attributes including the facts as attributes of the entity."
"Often, a very common practice is having these time windows. How many times did someone do this over a certain period of time? Which is usually bound to now."
"I think beyond the idea of a feature store and beyond the idea of making prediction and feeding ML model, there's a bunch of reason why it's really cool to have entity-centric metrics. The feature store is greater in its use than it is to just train models."
"If you have a million attributes about a user pre-computed when comes time to retrieve, there's a lot of value in knowing these things. There's a lot of value in having the data organized in that way. That's what I call entity-centric modeling."
I think the idea that metadata is growing is definitely super clear to me to write this idea too that we need a metadata warehouse."
"I think the more your data platform gets complex, ages, and has history, the more important that is to make sense to stuff."
"What if it was a new market that no one really knows about, maybe an emerging market? I think that becomes it's less of an internal and a data warehouse type question and maybe more of a research problem."
"I think in data as in many many things and fast-moving businesses, you have to do both the short term and the long term solution in parallel at the same time." The reality is, do you always have the resources to do both often or not."
"Clearly, it is different people with different concerns and different backgrounds operating on different timeframes. I think on the ML side, notebooks are just really great. What is a notebook, really? It's a program that you execute in not always the sequential order. So the repl stuff really works. I think it's important for ML practitioners, and the open-ended power of just like an interpreter, as opposed to something like SQL, which is not sufficient, that's for sure."
"If you do take the frontier stuff on the ML side of the house, where that is, let's say, notebooks. Notebooks are also useful for data engineers, too. For more specific things in data engineering, you might want to use notebooks too. The answer is in the air."
"Airflow will just allow you to express sets of jobs that depend on each other and provide the guarantees about the order of those jobs and when they’re gonna run. When you think about it, it's not even a data pipeline-specific thing, where it's very generic."
"Airflow certainly enabled people to do all sorts of things and in whatever ways."
"There are services that emerge that do certain things very, very well. And then Airflow is pushed back to become much more of an orchestrator and less of a compute engine, like a generic batch compute engine."
"I want to disrupt business intelligence and I want to change the way that mortals work with data every day. At the time, and now still, it is much more interesting to me than the data pipelines and orchestration. So after maybe two years of Airflow, I was like, “Okay, I built this thing. I’ll let it fly and let it grow.”
"My passion really went to Superset and about three years ago, I ended up starting a commercial open source company around Superset called Preset, and that's really just following my passion and interest in the stuff that I wanted to work on. Also the risk/reward I think is more interesting or it's higher risk, higher reward on the data versus data consumption layer."
"There are so many vendors in business intelligence that it's harder to get an open-source to come in and disrupt, because there are very, very established vendors where orchestration is kind of this little bit more of a new segment."
"I think through innovation we can disrupt and I think startups have been disruptive. But I would say there are two things that we do that are very disruptive. One is open source."
"Open-source is just this tidal wave of disruption. It comes in and it's free as in freedom and free as in better too, so that means, you can just pick it up, use it, and get value out of it – all disruptive. That's a version of freedom. You can also extend it, you can build integration points, and you can extend things, you can make it your own. That's extremely powerful."
"I think people are pretty fed up with vendor lock-in too, and proprietary languages and tools, and just being at the mercy of companies now."
"You can just prove value, use it, ramp up to five users and make sure that it works for you before you even talk to a salesperson. And you might not even have to talk to a salesperson ever, which is fantastic."
“How does data inform even the intuitive part of what we should be working on – or what we should not be working on – based on data?”
"Really often, we don't use data to power much of the processes and most of the roles. So you might work in customer success at a company and not be data-driven – just pick up your tickets and get on the phone with people and help them."
"You could have a really intricate set of dashboards around not only what the customer success team does every day, but also the new things that they might want to try or that they might want to do."
"Essentially, data powers every role and every process and there's a question of, “How do we make sure that all of the data that is needed by most of the people every day in an organization is available to create the validation and the opportunities – validate the intuition or invalidate some intuition?”
"I think a big part of data science is making models. And a big part of making models is training models. And a big part of training the model is about prepping the data to train your models and then you want to be rigorous around that, which you should be, especially given the impact that we've seen ML can have in the world."
"Provenance and reproducibility for something like that seem really, really important. So that means a lot of the best practices to make sure that data engineering is done right do apply to data prep for training models and concerns around reproducibility provenance, “What was this machine learning model trained with, and can we reproduce that?” That becomes very important."
"The rigor in the data engineering process does apply to everything related to preparing datasets to train models."
"Moving fast and breaking things and really living by it was really impressive and power empowering to see."
Transcript
0:00 Demetrios
[intro music] Vishnu, you see all the kids' toys behind me?
0:04 Vishnu
Oh, yeah. Where are you at? 0:05
Demetrios I am officially in Toronto for the MLOps World Event. It’s going to be in person. You are threatening to come.
0:14
Vishnu
I'm threatening. It's a firm threat and you gotta send me the times. [chuckles]
0:18
Demetrios
Yeah. We're gonna try and record some live stuff at the event so it should be cool. But today we're talking with Maxime Beauchemin – you know how I am with the names, I cannot get them right for the life of me. But this guy, if you have not heard of him, you probably have used a tool that he has created, whether that was Airflow or Preset. The dude is all over the place. And… sorry, did I say Preset? Is it Preset?
0:48
Vishnu
Preset is the managed company that he runs, but Superset is the original tool, which is now run by the Apache foundation.
0:55
Demetrios
Yeah, there we go. That's what it was. I knew there was something. So, I loved some of his hot takes around why he would go and start a company around… Preset. Well, no, what is it? Oh, God… I can't remember the name of it now. I just started thinking about Preset.
1:13
Vishnu
So, he started Superset which is open source, and then started Preset. And the hot takes we're about data business intelligence.
1:20 Demetrios
There we go. That's what it was. So I have a total mind blank right now and thank you for walking me through that. The other part, which we just dove right into, and I really enjoyed that we got right into it – it was this entity-centric type of thing. What did you take away from that? Because I know that you were loving it. [chuckles]
1:43
Vishnu
Yeah, for sure. My big takeaway – and I'll go through Max's bio in a second, everybody should get to know who this man is and what he's done for all of us – but what we talked about was entity-centric data modeling. Why that's important is organizations are increasingly generating tons of data that we want stored. We're putting that into the data warehouse and using it to drive analytics and machine learning outcomes, but how we make that repeatable, reliable, scalable, and maintainable is through data modeling, which not enough companies do well. That was Max's point. And he gave us a sort of understanding of how to think about doing data modeling in a modern context that isn't just, “Hey, go read Kimball's books about dimensional modeling.” That stuff was written before we had like the MLOps and machine learning stuff we have today and he gave us an intro into how to think about it in 2022, with features stores and all the other sort of systems that are around it. So, it was cool.
2:45
Demetrios
Love it. Yeah, let's get into it, man. That was really good.
2:51 Vishnu
Let me take you through the bio real quick.
2:52
Demetrios
Yeah, for sure.
2:53 Vishnu
Max – you should follow Max Beauchemin because he is currently the founder and CEO of Preset, but more relevant to this podcast, he's actually the original creator of both Apache Superset (a business intelligence open source tool) and Apache Airflow, which is a data orchestration tool that probably all of you have interacted with. He has had an exciting career shaped at places like Facebook in 2012, Airbnb in 2014, and Lyft. So many cool blog posts – check him out.
3:20
Demetrios
And do not forget, we started a pretty awesome newsletter that you will want to also subscribe to. If you have not, it's The Best of Slack – or just join us in Slack and get all the information. Drink from the fire hose. Let's talk with Max. [intro music]
3:42
Vishnu
So Max, you just said something pretty interesting. You talked about this idea of introducing entity-centric data modeling and why that's of interest to you. Let me start by saying, I feel like a lot of machine learning is starting to meet the modern data stack. People are trying to do machine learning in the data warehouse and are trying to apply concepts like that. Before we get to actually talking about data modeling, I wanted to get your thoughts on that paradigm of just throwing a ton of data into the data warehouse and then doing machine learning on top of it right there. Does that make sense? Or are we just setting ourselves up for a future problem?
4:26
Maxime
Yeah, I don't know. I think one thing that makes sense is that data has a kind of gravity to it, right? It's kind of a very heavy thing and data wants to be together in one place and, ultimately, all data wants to be joined in union and kind of be brought together. So I think to bring it all in one place and call it data warehouse or late or data shack (or whatever you want to call it) it makes sense to take all your data and bring it to one place where you can make sense of it. Then does it make sense to use the compute resources inside the data warehouse to do ML?
I say bring the compute where the data is. To bring the data to where the compute is – I don't know, it seems like it does make sense if it is on a cluster on Snowflake or a BigQuery cluster, to bring the compute right there. It's easier to move the compute than moving the data. Then, you know, SQL the right set of semantics to express ML transformation, you start having this stuff where you have like… I remember doing some of that stuff at Facebook, where we added UDTFs and UDFs so you'd have ML-related user-defined functions inside your SQL. I think it's not that crazy to think you would have a program running in line in between SQL resources, right? Like, kind of select Star from UDTF, and that UDTF can be whatever language.
I was talking with Drew from DBT recently, and he was saying that they're really interested in people running Python code, they just don't want to be running Python code in DBT itself. They want for other things to run the Python for them, like “I don't want a Python interpreter running random stuff in my own stack.” But I think we're getting there. Snowflake’s got some stuff, I think, where you can run Python in line and things like that. So it doesn't seem that crazy to me. There's been crazier things done in the past.
6:28
Vishnu
You're definitely right that there's been crazier things done in the past, especially when we think about all the hacks that people have applied to data [laughs] over the years, over the generations. I think where that question came from for me – I've been reading a lot of Chad Sanderson stuff (he's the director of data platform at Convoy) and he writes a lot about how data modeling is more crucial than ever. We had him on a meet up recently – check it out to all our listeners – he talks about how data modeling is more crucial than ever with the proliferation of datasets. And the fact that they're all ending up in one place, where sometimes too little structure is applied. So that's where that came from. But I guess my question to you now is – you mentioned entity-centric data modeling. What does that actually mean and how does that apply to ML?
7:18
Maxime
Yes. It's an idea that I've been pushing forward – not talking a whole bunch about, or not even writing a blog post on it – but I was looking at ML practitioners, and what they do, and how they work, and just like ML training and what it requires. And really often, I think what you need is a really clear entity, and then a shit ton of features and attributes and things. And some of these things are just like an attribute of the data, so as you're training some predictions around a user, you might have a lot of demographics on that user, and that's interesting. But you also have a lot of facts and metrics, and 7 day visits and 28 day photo upload on something like Facebook, and then 364 day bookings at Airbnb – you want to take all of these features and then associate them with an entity.
I think stepping back and talking about data modeling, it's a little bit of a lost art, right? We're democratizing the analytics process and everyone is invited and everyone knows SQL and Airflow and DBT, but no one really knows the lost art of data modeling very, very well. So that's kind of an interesting thought. Then getting into dimensional modeling (for the people listening) there's some books written by Ralph Kimball from probably 25-30 years ago, around methodology on how to organize and structure your data and how to create the right data set so that it's best organized for retrieval and consumption. Dimensional Modeling is one of these things where you have typically have what they call the Star schemas or the Snowflake schemas, and it sounds very fancy, but generally, you can think of these schemas as having facts – something like bookings at Airbnb and then dimensions that are collections of entities, things like hosts and you have who is doing the booking, what is the listing for the booking, etc. So, those are dimensions. And those dimensions are entity-centric in some ways. A dimension, like a user or a listing, is in a lot of ways an entity.
In dimensional modeling, they decouple what is the fact – you have fact tables and dimension tables – what we see in ML is that people want to have these more features stores. So you take an entity, like a user, and then you put all the attributes, including the facts as attributes of the entity. Really often, the very common practice is having these time windows, like, “How many times did someone do this over a certain period of time?” Which is usually down to now. Because how many times you booked on Airbnb last year is a great predictor, perhaps, of how many times you will book next year. So this is very useful, and machine learning models may know how to make sense of these metrics and weigh them. Point being like – Okay, so that's a thing now. Call it a feature store.
But then I think beyond the idea of the feature store, and beyond the idea of making predictions and feeding ML models, there's a bunch of other reasons why it's really cool to have entity-centric metrics. Like the feature store is greater in its use than it is to just train models. You can have like, a million attributes about a user at Facebook precomputed – when it comes time to retrieving say something like a scorecard for a user to be like, “Hey, what's happening with this user?” There's a lot of value in knowing these things. And there's a lot of value in having the data organized in that way. And that's what I call entity-centric modeling, where you have a really clear entity, and then an infinity of not only attributes of that user but features in a sense of things like time window, function, and metrics at that level, too.
11:32
Demetrios
So it's a little funny, because I remember visiting someone's office when I was in London, and they were talking about how they have so much metadata now that they need metadata for their metadata. [chuckles] And it kind of feels like you're touching on that a little bit. Like there’s a big amount of stuff that is going on, but this entity-centric view will be a way around that. It's like, you have the main protagonist and then everything else that goes on there could possibly be a remedy for that.
12:13
Maxime
Yes, it’s a really interesting thought. I think the idea that metadata is growing is definitely super clear to me, too. This idea, too, that we need a metadata warehouse the same way we have a data warehouse – we need to take all the metadata from the company, like information about lineage and data provenance and data ops information, like operational metadata, what was computed and run, by which script and who's the owner of that stuff and where it’s coming from, and business metadata, too, of things like labels and definitions. We certainly have more and more of that.
I think the stuff like Amundsen and DataHub, if you're familiar with these open source projects, is trying to treat all that metadata and bring it all in a central place, where you build kind of a graph of all your metadata and your organization or all your data assets and bring it all together so you can see the kind of neighborhood – so you look at a table and you know where it's coming from, what's upstream and downstream of it, what’s the owner, that kind of stuff. That kind of stuff is like, the more your data platform gets complex and ages and has history, the more important that is to make sense of stuff. Otherwise, you're stuck with tribal knowledge and asking your peers like, “Can I trust this table? Is that reliable? Who owns this stuff?” So that's an interesting thought. As everyone talks about data and how there’s exabytes and like, tons of data everywhere and it's growing exponentially – I think it's a similar challenge with metadata.
14:02
Vishnu
Your point about the data platform and its age and the relative knowledge, and then how it kind of becomes like, “Hey, what's going on here? What do you think about this table? What is this feature? How is it actually computed? How are we actually defining whether someone is active or not?” Right. That's where all this tooling for tooling starts to evolve. The question I have for you, which is actually very much like my job, so I'm gonna ask you to do my job here. [chuckles] Let's say you're in a rapidly growing business, and said rapidly growing business has mountains and mountains of data and very, very pressing business questions to answer, “Hey, we have a business model to figure out here and you need to tell me whether or not my TAM is what I think it is today.” So you answer all these analytical questions and it doesn't feel like you have a ton of time to model the data. And it doesn't feel like you have a ton of time to think about, “Well, what are the entities that map rapidly to a map to a rapidly changing business model?” And say that the said data professional was in a team of two people – with a team of two people – how would you go about wrapping your arms around this problem like data organization for a rapidly scaling organization?
15:13
Maxime
Right. Well, first TAM is mostly an external problem, so maybe that's not the best example. [cross-talk] specialized in that’s already done the analysis for the TAM of your market. Well, let's say what if it was a new market that no one really knows about? Maybe an emerging market? I think that becomes – it's less of an internal and a data warehouse type question, and maybe more of a research problem, for the case of TAM. But let's say, if the question is more like your CAC to LTV ratio – customer acquisition cost divided by lifetime value of customers – pretty intricate, complex, intra-looking question inside your organization. You have to say, what is the lifetime value of a customer? You need to figure out, you know – look at your history and your customers and do some projections, and then CAC is “How much does it cost me to get a customer?” That's pretty introspective of a question, too. So how do you do this? For me, I think, historically, or a pattern that I've seen in the past is – if you're in a rich-ish organization, you're gonna do whatever you need to do to get an answer to that question this week and that will typically will be a data analyst, (or I guess, nowadays, maybe it's more an analyst engineer) who's gonna go and hit some raw tables and write a shift on a SQL and then maybe get a half decent answer to that question. [chuckles]
16:42 Vishnu
Flashbacks to last week. [chuckles]
16:43 Maxime
Yeah, so that might be like a little bit kind of putting a finger in the air and then “The best answer I could get you this week is this” and then hopefully, this person (maybe it's the same person, maybe it's a different person, but someone else who is working closely with this person) and they will be thinking about, “How do we do this so we can get an answer to this question every week for the foreseeable future and we can actually measure the trends?” And that's a little bit more the data engineer persona, who tends to work at a much slower pace, but does things right – builds things that will be there a year or two from now, and maybe five years from now, still the person treats their art or their craft or what they do, as a little bit more infrastructure that will have to be maintained in the future. And then maybe, “Is it the same person or not?” Probably not, right?
One is probably vertically aligned and working on a weekly timeframe and the other one thinks more in terms of maybe quarters, and then uses source control and code review and thinks about data modeling and costs and makes sure that everything that they've built so far will land every morning before 9am or has some SLAs and that sort of thing. So the answer is, in data, as in many, many things and fast moving businesses, you have to do both the short term and the long term solution in parallel at the same time. The reality is, do you always have the resources to do both? Often not, right? So it's likely that you give an answer this week and you put it in your pilot project, and maybe you don't get to it until something's on fire or an executive is like, “Oh, I need my CAC to LTV every Monday morning,” or whatever.
18:35
Vishnu
I'm kind of processing that answer because there's a lot to dig into there. But what sticks with me is this idea that like – yeah, you're right. Somebody's got to be working on fighting fires, and somebody's got to be working on building the foundation, so to speak. Splitting that difference and putting two different roles there and having two different mandates to say like “We're going to be doing both in parallel,” I think is really important for data teams to both build and maintain trust, and also support the business for the long term. Because it's the twin mandate that you kind of have when you're a data professional.
One thing I wanted to ask you, coming off of that is – this conversation has kind of started off on a very, in general, data team focus, like data engineers, analysts, and what's generally happening in the modern data world. And it sometimes feels like the machine learning world is sometimes a subset of that and other times it's a whole world of its own. You know, they’re kind of slightly different forums and there’s slightly different experts, and I'm wondering what your reaction is to that. Do you feel like the way that we should think about machine learning tooling and stuff is just a subset? Or is it a little bit of its own?
19:56
Maxime
Yeah. There's an interesting parallel to be drawn here. I don't know if you saw, I think it's like ‘the future of data’ Andreessen Horowitz came up with the modern data stack diagram. So they have a blog post and there was kind of the version 1.0 of it, which brought ML and data… they did like the whole data platform with ML as part of it in a single diagram. And then for their 2.0 version of it, they kind of decided, “Okay, this is too complicated. It looks like a spaghetti plate. Let's take the ML stuff and make it in its own diagram.” Which is kind of interesting. I'm not sure if the fact that they did that is informative – it'd be interesting to look at the differences between the two side by side. I don't know if we could pull it here and then look at, “What's the Venn diagram of that?” Like, “What is on both diagrams and what is very unique to one or the other?” I think it does relate to the data warehouse question you asked before, right? Are we kind of converging or diverging here? Clearly it is different people with different concerns and different backgrounds operating on different timeframes. I think on the ML side, notebooks are just really great. What is a notebook, really? It's a program that you execute in not always the sequential order. So the repl stuff really works. I think it's important for ML practitioners, and the open ended power of just like an interpreter, as opposed to something like SQL, which is not sufficient, that's for sure. So, I think the answer is that it's like intricately shared resources across the two, and then some areas where it's intricately diverging in some ways. If you do take the frontier stuff on the ML side of the house, where that is, let's say, notebooks. Notebooks are also useful for data engineers, too. Right? Maybe they spend more time in SQL in general and less time in notebooks, but notebooks are still a pretty great paradigm, depending on what you're trying to do. It might not be an ML use case. But for more specific things in data engineering, you might want to use notebooks too. [cross-talk] The answer is in the air.
22:24
Demetrios
Let's pull on that thread a little bit more, because it feels to me like Airflow is very much a data engineering thing, but then, a ton of data scientists and machine learning engineers love it. Some people have given talks on the community meetup where it's like, “Airflow, the unsung hero of the data scientists,” even. So I wonder about – did you have that in mind? When you were looking at creating a tool like that, or when you're going through it, were you solely focused on a data engineer? And then it just so happened that the data scientist also found a lot of value on it? Or were you doing research and looking at what the data scientists use cases were too?
23:18
Maxime
Yeah. There's a fair amount of history there, too. First, the inspiration for Airflow. With Airflow, I started at Airbnb in 2014. Actually I started in between jobs – in between my job at Facebook and I'd taken the job at Airbnb. I talked with the team and it was really clear that I was going to work on something like Airflow because they didn't really have anything like that. Then I was coming out of Facebook, where Facebook had had this Cambrian explosion of reinventing the whole data platform from scratch because the scale of the data was just too big to fit in an Oracle or Teradata database. So then they had invented things like Hive and Presto – or had been in the making while I was there. But I built their own visualization tools on top of that. I built their own kind of data portal – like the metadata repository I was talking about – we had built one of those. We had probably, I would imagine, five to ten different schedulers, and batch processing, your generic computation-type things like Airflow. And then two of those had been emerging internally at Facebook – one called Dataswarm and one called Databee, and they're both a way to run batch jobs like DAGs of batch jobs, which were expressed as SQL and/or Python. At that point, Dataswarm at Facebook had been used for a general computation – it was used by data scientists. It was used by all sorts of people wearing all sorts of different hats. It's just to do data pipelines, which included a bunch of ML data prep use cases, and probably some proper training as well. So when you think about what Airflow is – it’s just a way to express DAGs of batch jobs that are kind of time bound. Airflow will just allow you to express sets of jobs that depend on each other and provide the guarantees about the order of those jobs and when they’re gonna run. When you think about it, it's not even a data pipeline specific thing, where it's very generic. Like, it's a scheduler and an orchestrator. Then what you're gonna do in there is kind of limited only by what you can trigger in Python [chuckles] which is pretty much anything.
So I knew it was going to be used in all sorts of ways. We were talking earlier about all the funky stuff that people do in the history of data, and Airflow certainly enabled people to do all sorts of things and in whatever ways. There's a really good article – I don't know if you saw it – there's an article called “the Unbundling of Airflow” by Features & Labels. It's a cool article, because it shows all this stuff that you've been doing in Airflow is just like generic computation, generally around data. All these things that you've been doing are now getting catered to in a more directed way. So if you're doing [inaudible] maybe you'd better do that in DBT. If you're doing data sync, they bring data from your SaaS services, then introduce Fivetran or Airbyte. It's got an interesting take on it. I guess things specialize. There are services that emerge that do certain things very, very well. And then Airflow is pushed back to become much more of an orchestrator and less of a compute engine, like a generic batch compute engine.
27:13
Vishnu
For sure, yeah.
27:14 Demetrios
I have to ask before Vishnu jumps in – you've probably been asked this a million times, so forgive me for just following the crowd – as you see Astronomer doing what they're doing, that was never a thought in your mind? Or did you analyze trying to do like a managed Airflow and you decided not to? How did that play out?
27:39
Maxime
Oh, yeah. So for context for people who don't necessarily know me and the history – so I started Airflow. I started another project called Superset. So Apache Airflow, Apache Superset – I started both of those at Airbnb in 2014-15. My passion really went towards Superset because it's visual, it's interactive, it's colorful. I'm kind of… I want to disrupt business intelligence and I want to change the way that mortals work with data every day. At the time, and now still, it is much more interesting to me the data pipelines and orchestration. So after maybe two years of Airflow I was like, “Okay, I built this thing. I’ll let it fly and let it grow.” And my passion really went to Superset and about three years ago, I ended up starting a commercial open source company around Superset called Preset, and that's really just following my passion and interest in the stuff that I wanted to work on. Also the risk/reward I think is more interesting or it's higher risk, higher reward on the data versus data consumption layer.[cross-talk]
Yeah, let's talk about it. But first, for people who don't know what Superset is, I urge you to check it out. Superset is an open source, pretty much data consumption, dashboarding, data exploration, data visualization platform. It's very much a competitor to things like Tableau and Looker – so all the things that you can do with Tableau and Looker, you can do on the open source front with Superset. We've been working super hard on it, so it has become really awesome and really competitive. The risk to reward ratio is higher to meet because it's such a very red ocean market. There's so many vendors in business intelligence that it's harder to get open source to come in and disrupt, because there's very, very established vendors where orchestration is kind of this little bit more of a new segment.
I did think about starting to go more in the direction of Airflow and investors came to me and said “Hey, why don't you start an Airflow company?” And my heart and passion was very much on the Superset front. I've been really friendly and following closely to folks at Astronomer. They tried to get me to join early on. I was talking with them super early on and, you know, I'm very friendly with them and a little bit invested as an advisor with them, too. I still have passion to talk about this stuff and being close to that world, but my focus really has been on the data consumption layer, data visualization, dashboarding, and how people make sense of data – that’s what I've been working on.
30:43
Demetrios
So you mentioned it is a red ocean that you're getting into? In a very blunt way, what makes you think that you can disrupt this field?
Maxime
Well, I think there's like two big angles – I think through innovation we can disrupt and I think startups have been disruptive. But I would say there's two things that we do that are very disruptive. One is open source. Open source is just this tidal wave of disruption, right? It comes in and it's free as in freedom and free as in better too, so that means, you can just pick it up, use it, and get value out of it – all disruptive. That's a version of freedom. You can also extend it, you can build integration points, and you can extend things, you can make it your own. That's extremely powerful. I think people are pretty fed up with vendor lock-in too, and proprietary languages and tools, and just being at the mercy of companies now, like Google Cloud on the side of Looker and Salesforce on the side of Tableau. I think people have a new way to discover, validate, and pick and ramp up on software and that new way is closer to what open source is or SaaS.
That's the other angle on how we can disrupt in this market, believe it or not. Just with some of the stuff that comes with SaaS like freemium, preset offers – a free version of Superset of up to five users, for instance. You can just prove value, use it, ramp up to five users and make sure that it works for you before you even talk to a salesperson. And you might not even have to talk to a salesperson ever, which is fantastic. So I think all the things that come from SaaS – things like time to value, and in our case, time to first dashboards, ease of connecting to things. So really focusing on that early journey user experience and then using mechanisms like reengagement emails, things like being able to send people emails saying, “Here's some dashboards you might like in your workspace or that people in your team have created.” So the mechanisms of your typical SaaS company as applied to data visualization BI makes total sense too and will make it more of a reality to disrupt.
33:29
Vishnu
Yeah. This conversation went in exactly the direction I was hoping, despite my complete lack of involvement. So it's outstanding to see that. [laughs] But, I guess what I want to share here is – I work at a company where we struggle a lot with how to do business intelligence right. What that means is, not necessarily that we can't figure out whether to buy Tableau or Power BI, but really, we want business intelligence to work in a fundamentally different way than it has been, which is “Put up a dashboard of KPIs and then check it every day. And over time, people stop paying attention.” Six weeks in people are like, “Is that even around?” We've had that experience with things like QuickSight and the other sort of standard stale offerings, you could say, that are out there.
I think for us, what we find is that there are really two use cases. One is just monitoring to make sure that certain business things that need to happen are happening. And then the other thing, which is more insight driven is, “What are some things we should be doing,” or “What are some things we're aware of or need to explore and get ahead of before it's happening?” And I would really say we want to empower that latter piece a lot more, but really struggle with it.
I was wondering, from your standpoint, from your vantage point – you've been at Facebook, Airbnb, you're now helping commercialize Superset and bring it to the world – what are some of the solutions or the ways that companies should think about that latter part problem of empowering that sort of exploratory work and giving mortals superpowers with data?
35:05
Maxime
Yeah. You were talking about one angle, which is like “How does data inform even the intuitive part of what we should be working on – or what we should not be working on – based on data?” One way to think about it is like, data is kind of a dimension of all the work that every worker does every day. So if you're working on a new product within your company, like a new SKU or a new feature… essentially, for whatever role inside a company, whatever workflow, whatever process – data is a dimension of that. Really often, we don't use data to power much of the processes and most of the roles. So you might work in customer success at a company and not be data-driven – just pick up your tickets and get on the phone with people and help them. But there is a huge data dimension of work, right? And you could have a really intricate set of dashboard around not only what the CS team (the customer success team) does every day, but also the new things that they might want to try or that they might want to do.
Essentially, data powers every role and every processes and there's a question of, “How do we make sure that all of the data that is needed by most of the people every day in an organization is available to create the validation and the opportunities – validate the intuition or invalidate some intuition?” Really often, that data is just not there, right? We don't have that data. It's just in Jira – we don't really know what to do. So yeah, I don't know if this is answering that question of “How do we become more data-driven?” I think there's a hunch of how do you show up to a meeting with the analyst-type question like, “Hey, I wonder what our percentage of, or success rate for X is?” And it's like, well, instead of just wondering about it, why doesn’t someone go and get that data and bring it to the next meeting? Or maybe the data is already there so we can validate/invalidate hypotheses instead of it just being ‘my intuition versus your intuition’. That is not super concrete and useful. These organizations that I was at – Lyft, Airbnb, Facebook, Yahoo – I think you'd have a lot of that culture of like, “I wonder about X? Let's get the answer to that question. Let's get that data and bring it to the table.” Just not accepting raw intuition without data.
37:53
Demetrios
Yeah, that makes sense. And it's the cliché – like the data-driven organization. And you hope that most organizations are doing that. So, changing gears a little bit, you spoke about how you play in the data engineering field quite a lot. But you are also building for data scientists and machine learning engineers, because a lot of the stuff that you do inevitably touches on their space, too. Are there things that you've found over time, as a builder in the data engineering space, which you need to watch out for when it comes to building for data scientists?
38:38
Maxime
In terms of tooling or data pipelines, or both?
38:43
Demetrios
Yeah, let's start with data pipelines. But if there's something that's calling at you – for sure, jump on that.
38:51
Maxime
Well, I think a big part of data science is making models. And a big part of making models is training models. And a big part of training the model is about prepping the data to train your models and then you want to be rigorous around that, which you should be, especially given the impact that we've seen ML can have in the world. You look at the Facebook newsfeed ranking algorithm and the impact that has had on polarization and our society and you're like, “Okay, the way that we train this model, or this machine learning – these seem really, really important.” Provenance and reproducibility for something like that seems really, really important. So that means a lot of the best practices to make sure that data engineering is done right does apply to data prep for training models and concerns around reproducibility provenance, “What was this machine learning model trained with and can we reproduce that.” That becomes very important.
I would say – the work that I did around sites, I gave some talks and wrote a blog post on something called functional data engineering, which is applying some of the functional programming paradigm to data engineering, or at least like trying to draw some parallels between the two and seeing where these parallels make sense where they’re virtuous. And then encouraging people to go in that direction to say “Your jobs that are pure functions – your tasks when you do data pipelines – should be your functions. You should use immutable data blocks and create other immutable data blocks and you should have deterministic results. When you run this function with this immutable data block, you should always get this result, so that you can get to some level of guarantees around reproducibility, or at least being able to explain the results that you got.” I think that's important. So the rigor in the data engineering process does apply to everything related to preparing datasets to train models.
41:18
Vishnu
Yeah, I actually love that point – the last point you made. For those of you who haven't read them, go check out Max's blog posts, especially the one about functional programming in the data context. So much of the work that we do as data professionals and as machine learning professionals is just applying the principles of software engineering to data. Like that's what it's about a lot of times. It's figuring out where it works and where it breaks, right? It's a really cool blog post that I would suggest checking out. With that, I want to get into some rapid fire – some lightning round stuff.
41:51 Maxime
Bring it on!
41:52 Vishnu
We’ve got some stuff lined up for you. I'm gonna go first – what was the craziest (crazy might not be the right word, but just gonna use it) the craziest work environment for you to walk into? Facebook in 2012, Airbnb in 2014, or Lyft and 2017?
42:07
Maxime
So “define crazy,” but I would say the most surprising and changing was Facebook in 2012. Huge environment and reinvented everything from the ground up – super dynamic, no rules, and but at the same time tremendous amount of amazing work taking place. That was before a lot of the issues that came up with Facebook or Twitter – that was before there was all this doubt in the air. But, you know, moving fast and breaking things and really living by it was really impressive and power empowering to see. I've never seen anything like it and never imagined that a workplace could work with loose rules like. That was pretty amazed to be part of it and I was really empowered by it, too. [audio cuts out] for sure.
43:01
Vishnu
I gotta say, those three things on your resume and the times that they’re at – it's kind of like playing for Barcelona, then Real Madrid, then Manchester City, like one after the other. [laughs] The next rapidfire question that I have is – which of your blog posts were you most surprised by the reception to?
43:22
Maxime
Goodness. I think “The Rise of the Data Engineer” was super well-received. I'm not necessarily surprised by it, because I think I'd read maybe five years before “The Rise of the Data Scientist,” and I was like “I'm gonna do the same thing for the data engineer!” You know? I think the idea was likely to be fairly big, because I knew that the role was going to be a big role. These terms – we get our data and engineering together, it's just such a powerful merge of concepts that I knew was going to be big. But it's still quoted. Maybe what's surprising is just how long – the life cycle seems pretty long. I would have thought, “Oh, people read it, it gets some comments in the first few months and then it fades away – nobody talks about it again.” But still, people still read this blog post.
44:13
Demetrios
Yeah. Okay, so what is a piece of technology that would surprise people that you're bullish on?
44:21
Maxime
Wow, that's a tough one. I'm not sure. I feel like I need to really scan through my head all the things that are controversial. You know, maybe I'll bring something that's controversial and bring an angle that I like. I don't know if you've heard about Data Mesh – it's been controversial on Data Twitter and elsewhere. Because I think it's not very prescriptive in terms of solutions and what it means for data modeling or for data products – what it really means. But I think it does a really good job at summarizing the issues that we have around data governance and how people should work with data in modern organizations, additionally to your point. It's like people don't really know, “What are the roles? And what are the approaches? How do we do data right in larger, fast growing companies?” So I think they identify some of the challenges very well. I don't think they offer really clear solutions to it, but they do a good job at summarizing all the issues of our times, you know, data team culture.
45:37
Demetrios
[chuckles] It's hilarious that you say that because when we've had people on here that talk about Data Mesh – I liken it to world peace. It's like, “Yeah, everybody's for world peace. Nobody's saying we don't want world peace. It's just – how do we go about that? How do we get it and actually execute on it? That's another story.” I throw it in that field of my brain. So, all right. [cross-talk]
46:07
Maxime
But more on that – it's like, when you read the paper, you're like, “Oh, my God! This is so good! I relate to everything they say. I can't wait to get to the chapter where they offer the solution and solve it all.” And then you read it and it's like, “Treat data like products.” I'm like, “Okay, that's kind of what I've been doing forever, right?” And then it's like, “Oh, they just renamed this concept to a different name.” So it kind of falls flat a little bit on paper.
46:33
Demetrios
Yeah. It calls out the problems and the pains really well. We were talking about Chad Sanders earlier, and he very much drank that Kool Aid and I think he's one of the ones, in my eyes, that is exploring how to do it and what the remedies could look like at his own job. He's trying to really move the needle. So that's a cool one. All right, last one for you. I'm torn between whether I want to ask you what the last book you read was, or what the most ridiculous piece of marketing around data you've seen has been – so I'll leave it open for you.
47:14
Maxime
Oh, goodness. I think that Data Mesh might be – it’s a little bit like a kind of a consulting/marketing play. Or there's a little bit of naming things kind of land grab there, too. I think that that would go there. I’ve picked up a book that I've just started. Now I gotta go look up the exact title, but it's about the life of Frank Zappa as told by his life partner. I don't know all the details. I have to Google and dig out the exact name. I read like the first chapter – I think it’s “My Life With Zappa” or something like that. I'm just really interested in Zappa as a musical genius – it’s kind of very interesting.
48:01
Demetrios
Did you see the documentary? The recent one that came out? What was it called?
48:03 Maxime
That was good. I think maybe it was just called “Zappa” [cross-talk]
48:09 Demetrios
Yeah, maybe.
48:11 Maxime
But I'm gonna go look at my Amazon purchase list. We can put it in the show notes. Yeah, the documentary was great. You know, there's something amazing about Zappa, is that he's been kind of hoarding his own material. So you've been recording everything – so he's got like somewhere in his basement in one of his houses, he’s got all the tapes and all the movies and all of the everything he ever recorded. So we can expect a lot more of the treasures to emerge out of those archives, which is amazing.
48:50
Demetrios
I'm sure you've seen “Zappa Plays Zappa”?
48:55
Maxime
Yeah the result is [cross-talk]. Yeah, I didn't see it. I know it exists. I’m big on Dweezil. I'm sure it's… yeah, I just don't know him as much.
49:05
Demetrios
It's crazy how much – so Dweezil looks like him and acts like him and everything, It is so crazy how similar it is. Oh, dude, his guitar playing skills are off the chart – like Zappa, like Frank. I went to a few and they get the old legends that used to play with Frank to come on and just like to tour with Dweezil or make some guest appearances. So I saw Return to Forever and then Dweezil played like Zappa plays Zappa. Yeah. It's definitely worth it.
49:47
Maxime
[cross-talk] …that plays with Zappa. But yeah, maybe not for everyone, right? Frank Zappa is kind of an acquired taste. But an American genius. Yeah. Check it out. Maybe it is for you, if you haven't really tried. What's a good gateway album for people to start?
50:03
Demetrios
Joe’s Garage is my favorite. Joe's Garage.
50:05
Maxime
Oh, yeah. It is more like, on the funny side. But so many good albums for the jazz people or a little bit more coming from jazz, there's The Grand Wazoo and Waka/Jawaka that I really like. [cross-talk]
50:21
Vishnu
I'll start with these recommendations.
50:23
Maxime
Let's start another podcast. [laughs]
50:27 Demetrios
Yeah, we can just geek out on that. Well, I mean, I could right now. That's so funny you're saying this, because one of my favorite things in that documentary that they talked about, is how they asked him like, “Why are you doing this? You're spending all this money, you're going overseas, you're contracting orchestras to make your music?” And he just sits there and then they're like, “And you're not going to make any of this money back.” And he tells him straight up, “If it's music that I want to listen to – I've won.” [chuckles] It's just like, that's how I want to live my life. If it's worth doing, because I will enjoy it, then I want to do it and I want to make it happen.
51:06
Maxime
A principle to live by, everybody. If it’s data science that you want to consume or I don't know… [laughs]
51:12
Demetrios
There we go. We can extrapolate that into the MLOps world. Maxime, it's been amazing, man. Thank you so much for coming on here and sharing your wisdom and advice to us all.
51:24
Maxime
Awesome. Well, thank you. It was a pleasure to be on the podcast here.
51:26 Vishnu
Thanks for coming.