MLOps vs LLMOps
speakers
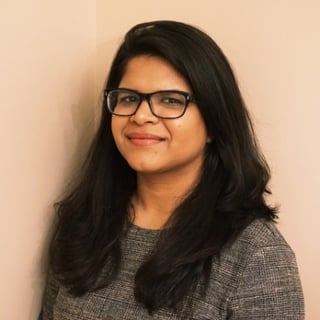
A passionate and impact-driven leader whose expertise spans leading teams, architecting ML and data-intensive applications, and driving enterprise data strategy. Richa has worked for a Tier A Start-up developing feature platforms and in financial companies, leading ML Engineering teams to drive data-driven business decisions. Richa enjoys reading technical blogs focussed on system design and plays an active role in the MLOps Community.
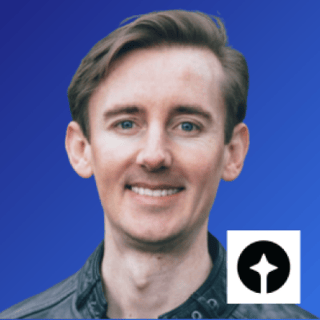
Willem Pienaar, CTO of Cleric, is a builder with a focus on LLM agents, MLOps, and open-source tooling. He is the creator of Feast, an open-source feature store, and contributed to the creation of both the feature store and MLOps categories.
Before starting Cleric, Willem led the open-source engineering team at Tecton and established the ML platform team at Gojek, where he built high-scale ML systems for the Southeast Asian Decacorn.
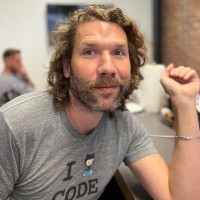
Chris Van Pelt is a co-founder of Weights & Biases, a developer MLOps platform. In 2009, Chris founded Figure Eight/CrowdFlower. Over the past 12 years, Chris has dedicated his career optimizing ML workflows and teaching ML practitioners, making machine learning more accessible to all. Chris has worked as a studio artist, computer scientist, and web engineer. He studied both art and computer science at Hope College.
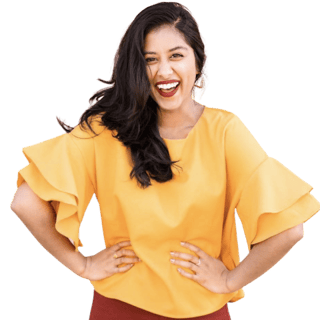
Aparna Dhinakaran is the Co-Founder and Chief Product Officer at Arize AI, a pioneer and early leader in machine learning (ML) observability. A frequent speaker at top conferences and thought leader in the space, Dhinakaran was recently named to the Forbes 30 Under 30. Before Arize, Dhinakaran was an ML engineer and leader at Uber, Apple, and TubeMogul (acquired by Adobe). During her time at Uber, she built several core ML Infrastructure platforms, including Michelangelo. She has a bachelor’s from UC Berkeley's Electrical Engineering and Computer Science program, where she published research with Berkeley's AI Research group. She is on a leave of absence from the Computer Vision Ph.D. program at Cornell University.
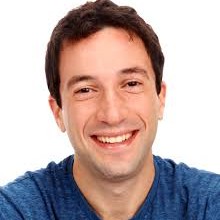
Alex Ratner is the Co-founder and CEO of Snorkel AI and an Assistant Professor of Computer Science at the University of Washington.
Prior to Snorkel AI and UW, he completed his Ph.D. in CS advised by Christopher Ré at Stanford, where he started and led the Snorkel open-source project, and where his research focused on applying data management and statistical learning techniques to emerging machine learning workflows such as creating and managing training data and applying this to real-world problems in medicine, knowledge base construction, and more. Previously, he earned his A.B. in Physics from Harvard University.
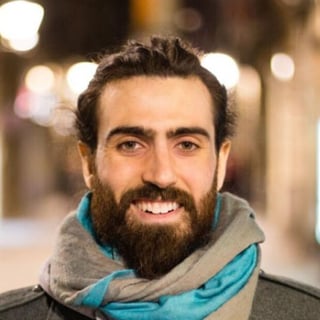
At the moment Demetrios is immersing himself in Machine Learning by interviewing experts from around the world in the weekly MLOps.community meetups. Demetrios is constantly learning and engaging in new activities to get uncomfortable and learn from his mistakes. He tries to bring creativity into every aspect of his life, whether that be analyzing the best paths forward, overcoming obstacles, or building lego houses with his daughter.
SUMMARY
What do MLOps and LLMOps have in common? What has changed? Are these just new buzzwords or is there validity in calling this ops something new?
TRANSCRIPT
But without further ado, I think it's time for a little panel Risha. What's happening? Well, I'm hoping my phone doesn't ring and say seek shelter because we are in the middle of a thunder and tornado storm in the greater Philadelphia area. So fingers crossed. Oh no. Well, if it does, um, then you get, you stay safe.
I'll jump on and, and play your part for you. So then we'll, um, we'll also bring on b b bbo. Where, who else do we have here? Mr. Willem? What's up dude? And we got Chris. Oh, this is a great panel. Where's Aparna? Yes. And last but not least, we've got Alex. But Alex calling Alex to the stage. Where is he? There he is.
All right. So there's a ton of us. I'm gonna hop off, reach out, I'm gonna hand over the talking stick to you and we'll get it started. All right, thanks Ringo. Um, welcome panel. Um, let's just take a quick second to go around the room, introduce ourselves, and then we'll dive right into the questions. I'll kick it off.
My name is Richa, such Dave. I work for JPMorgan Chase and I'm in the Chase Rewards Department and lead a team of data engineering and operations. And we work on the business side to help them make data-driven decisions. I'll pass it over to you, Aparna. Awesome. Hey everyone. Good to get to see you all. Uh, excited to be on this panel.
My name's Aparna, founder of Arise Eye Arises ML Observability, and I guess LM Observability, which would be the topic of, of today. So excited to, to, you know, have, have a great combo with all of you. Wonderful. Thanks, Aparna. Over to you, Chris. Hey everyone. I'm Chris Van Pell, co-founder of, uh, weights and Biases.
I'm repping the brand today. Uh, our mission is to build, uh, developer tools for, uh, machine learning or l l m, prompt engineering, uh, engineers. Welcome, Chris, Alex. Hey everyone. Um, I'm Alex, I'm one of the co-founders and CEO at Snorkel. I'm also, um, affiliate assistant professor at University of Washington.
Uh, and before that I was working on the snorkel project at Stanford, all things, what we call data centric ai, so, uh, labeling, but also curating, sampling, filtering, cleaning, all the data that goes into. Either training models from scratch or fine tuning, pre-training, instruction, tuning, um, large language models or foundation models as we like to call 'em.
So excited for this, uh, this chat. And Richard, thanks for hosting, of course. And this panel would not be complete without Woo, Willem, you're next. Hey folks. Yeah, my name's Will. I've been in the data end AI space for about, uh, you know, six, eight years now already. Um, leading ML platform teams, ML infra teams, and building in the open source space.
Uh, recently most focused on feature stores, but, uh, really syncing my teeth into the l LM space in the last couple of months. Well, thank you all for me. Yeah, this is definitely a great panel and I'm excited for all of the learnings and will Nice to see you again. So I'll ki kick it off with you. Can you walk us through what do you think in your experience with the, the high level differences between LLM ops and ML ops?
And it's quite a tongue, tongue twister, LLM ops, I, I always make sure I have the right number of Ls. Yeah, I feel like this question we can probably expand and talk about for 30 minutes to an hour, so I'll try and keep it as brief as possible. But, um, I think a big part of it for me is abstraction and generalization.
Um, to me l mops is, um, language oriented, right? And so there's a big question around how you frame your use case as a builder. Um, to what an LM can do because it's so generalized. Um, but I think we've seen a democratizing effect of, um, these LLMs bringing in new folks that couldn't previously. Um, uh, Traditionally stacks and solutions.
Um, but I think the, a key difference for me is the offline flow, right? That's an area that has become somewhat optional right now, and I think for application builders that has enabled. New use cases to be unlocked and for folks that you know, weren't in the space to suddenly build, right? You can just start with a model in production.
Frame your use case, um, around an L l M and, and get that in front of users and, and get. Traffic onto that model and then figure out the next steps and the offline flow where, whether there's debt collection, whether it's training, um, that that whole step has become a secondary step as, as opposed to the first step.
Um, and I think that's, that's a key thing and I'll kind of leave it there and that the rest of the panel can expand on that. Yeah. Uh, thanks Willam. Anybody wants to add on to what Willem just said? Yeah, I mean, I think Bo's point was, was a really good one. You know, when we think about data scientists and ML engineers and what the workflow has been like for, call it statistical ai, traditional ai, there's, there's a lot of names I'm, I'm hearing floating for, you know, non lll m type of models.
Um, but, but what happens is a big chunk of that work is in the training of, of the model and. I think that, you know, when we think about how do you go improve the outcomes, you think about, well I'm gonna go and train it to, to get X percent increase in performance. That's become a fundamental shift in this new LL mops world.
Is that it? There's so much you can do with prompt engineering, and actually I think this is what kind of what Willem's point was, which is in the, you know, the offline world. It might not look like training. It might not look like fine tuning. It might just be iterating on what is the prompt, what is the context that I might wanna incorporate into the prompt?
And it's a very fundamentally different, different workflow and different way of thinking about improvement than, than in the past, which is, um, Which is where, you know, for, for me, the, the one big thing I've been thinking about is, well, how does this change the persona? Does it, you know, are, are there going to be just data scientists who are building these models, just ML engineers?
Does it potentially open up the persona a bit to, you know, I've actually already seen job postings for L L M engineers, and so does it open up kind of that persona for a wider audience because. There's less of an emphasis on, on training and more of an emphasis on, call it prompt, and prompt, prompt, iteration.
Um, the one thing I do think will, will stay true in both ML lops and LLM ops though, um, is it's very easy in the LLM ops world to, to, I think, try things really quickly, get a Twitter demo up, post it on Twitter, but just as an ML ops. Very, very hard to get something to work robustly or work consistently.
And so, um, that happy to, I, I think, dive into that and, and talk about that more. Yeah, for sure. So yeah, it's interesting you're saying that probably the way the teams are going to be structured, the personas, the life cycle itself might change. But for like companies that are getting up to speed with the LM world, I know like MOPS is still something that is not very easily understood with the introduction of mops.
What does it mean for companies to strategize? Can they take what they've already built and carry forward? Is it a completely separate initiative? So curious, Alex, if you have any thoughts around around that. Yeah, I'll, I'll, uh, respond to some of the great points that while and partner raised. I think there's a, um, Uh, I guess my short answer to your direct question is I think there's a lot of the ml lops infrastructure that is going to remain the same.
Um, and this has a lot to do with the appropriate models to actually serve in production for some subset of use cases, we, we often call 'em predictive use cases, uh, classification, extraction, uh, a lot of the traditional things that, um, actually a lot of enterprise value still rests on today versus some of these larger, more generalist agents that kind of have open-ended.
You know, q and a chat, dialogue, et cetera. Um, I guess I said I, I already talked long enough that I'm not, I'm, I'm, uh, it, I'll just, uh, cut to the punchline. In my opinion, there's a lot of those, um, those tasks, uh, more predictive tasks that still probably are gonna make up a lot of the, the value that enterprises get out of ai.
They can be distilled down, um, you know, orders of magnitude to traditional models, basically, think of it as like going from a generalist to a specialist. Um, one way that we've been thinking about these, these foundation models or large language models is, um, as kind of first mile tools. Uh, par your point about, you know, it's very easy to make a demo, but the last mile still remains very, very hard, right?
We, we all, everyone who's, you know, and AI knows that problem and, and knows that at least for a. Subset especially of highly complex and valuable problems. That's not changing. That's why self-driving has been here next year for the last decade. Um, that's why the last mile is always difficult. Um, but you know, I think these, these big generalist models will give us a very powerful base to start the explorations and to, uh, we often refer to in our platform as warm, start the problem.
Um, and then you need to. Trained specialists that branch off of them, and those specialists can be much smaller and often will just look like a traditional ML model artifact. Um, we had a paper with some, uh, uh, one of my great students at UDub and, and, uh, some Google AI collaborators on a, a distillation, new distillation approach basically of kind of using a big model to teach a smaller model.
But this is an old technique and really, you know, the intuition of, of how you can get this crazy result of a tiny model that's doing far better than the big model. Is this just generalist to specialist? You don't always need a generalist jack of all trades. That's hundreds of billions of parameters when you have a specific narrow problem you need to do really well at.
So I think a lot of, um, a lot of enterprise value, a lot of the problems that actually get shipped to production. Really are going to need specialists. And I think for specialists we're gonna see a lot of building off of big, often open source models that are more than good enough to give you that, that warm start and then specialization and those specialists will most likely run on existing ML ops infrastructure.
So the serving infrastructure will look almost identical, I think when you truly do need a generalist. And I think that we're still figuring out what subset of problems are real there when you, but, but you know, when you do need some kind of, you know, generalist co-pilot or chat bot, um, then it will look like a new type of serving mechanism because then you can't shrink it down as much.
That's serving. And then training, I, I've already talked too much like at myself off, but training, I would just say I think there's a, there's a chunk of the world that will look like, you know, a part what you were articulating quite nicely, where it's more about the prompt engineering. There's a chunk of the world where actually because you're working on specialized data or, or high value and or high value use cases, you are gonna need to continue training a piece of the model, an adapter, fine tuning, et cetera.
Um, actually a lot of that we're gonna see converging in context learning and fine tuning. Um, there's some cool new papers out about how those can be, you know, conversion. So it will, in our view, come back to just the kind of engineering of the data and context you put into the model, whether it's through training.
I e fine tuning or through context windows, it'll really be more about that. Um, so that's, I think on the, in summary, I think on the training side it's gonna be a lot about these data operations, whether it's a prompt or a tuned prompt, or a prompt that includes a set of examples, which is now back to a labeled dataset or, or a mixture of all these things, plus some external context.
It's gonna be all that data engineering. Um, and then more so than the actual kind of hyper parameter algorithm model architecture selection. Um, and then on the serving side, it's gonna be kind of a split between these highly specialized models that'll look like traditional lops, um, and traditional models actually would be my bet.
And the kind of true general generalist where you, you do need a gigantic model and the ops will look a little bit different. So that's my, that's my hot take. Um, but I'll pause there. Talk too long. Yeah. Thanks for the great insights, Alex. Um, Chris, I have a question for you. So in your own world, when you're talking to different companies, data science teams, et cetera, practitioners that are in the weeds, how are they thinking about using open source tools, let's say for a financial firm?
Um, how are they making sure that the data is safe and it's easy or, or is it safe for them to leverage the open source tools that are available out there? Or is there a lot of talk of trying to build some of these LM models in-house as well? Yeah, well, I mean, it'd say it's still early days. Um, we're all obviously very excited about LLMs, but I think.
Getting these into big enterprises doing real work is, is, you know, still yet to, to really happen in a meaningful way. Um, I talk to security teams and, and data teams often and you know, it is a big concern. Um, To, you know, just send this data out to, to say something like Open AI's, um, api, they've mitigated this with, uh, you know, allowing you to have kind of a, an isolated, um, Azure native API in the, in the case of OpenAI, but, I think there's still folks that, that want to own the entire stack or literally, you know, can't send out the data to any third party at all.
And that's, uh, that's when either, you know, building something from scratch or, or partnering with, uh, folks like, uh, mosaic, uh, to help, you know, build out something, um, for themselves. And there's a whole, you know, there's a bunch of startups now just focusing on like, Hey, we'll build you in l l m, um, It remains to be seen if that, you know, that's a big burden to, to manage and deploy and deal with one of these large, um, LLMs.
So I think, we'll, we'll still see where this, where this ends up, but it's, it's a bit of an unanswered question. The other day, it was, uh, I think, uh, early this week there was a, a startup on Hacker News who's talking about this exact problem. Like we're trying to give better data observability and, and lineage and governance around.
You know, what is is going into these things to potentially redact. So I think we'll, we'll see kind of where, where this lands. But, uh, it's definitely a big question on, on the enterprises minds. Well, thank you. And, and the, the follow up that I have is like, how are they thinking about the cost? As I understand there's a huge cost associated, so how are the companies rationalizing it around the ROI that they're gonna generate from it, or it's still very early to determine that.
Yeah, I mean it's, it's expensive for sure. Um, so I mean, I think a lot of these bigger orgs, they have a budget and they can, you know, very kind of rationally think about would this make sense to take, you know, this massive chunk outta the budget. Uh, but it's also, still, still early and there's a bunch of startups trying to make it a lot cheaper too, so, um, yeah, we'll see.
Yeah. Yeah. I think there's a lot of great progress. Oh, sorry. Go. No, no, go ahead. I, I think there's a lot of great progress to Chris's point on, on, uh, startups and, and a lot of that coming from academia on, on just making these, these operations at all stages cheaper. And then you just have, um, I think as the, the, um, the space matures a bit, although these are all very classical ideas.
You have these different stages you can start at. So it's not either start from scratch, Or taken off the shelf, you know, API based, uh, uh, foundation model. You can, you know, you can take an open source base and you can, you know, build off of that. You can, there's various, uh, there's a, a whole spectrum of kind of customization, uh, you know, kind of entry points.
So I think a lot of organizations, these large enterprises that Chris, you, you know, Chris talked about that, you know, don't necessarily wanna send all their private data to, um, you know, to an open ai and don't necessarily have to because the open source. Models, they're, they're not quite as good, uh, right now as, uh, you know, a kind of general open chat and taking, you know, I don't know, MIT entrances exams and LSATs and all the things that apparently, you know, baby LMS do these days in their free time.
Um, but they are more than good enough to kind of base off of and build a highly accurate specialist. So you have all these options of kind of, where do I start and how do I specialize it? And I think in terms of cost rationalization, One of the appealing things is both being able to use this as a base for kind of jump starting multiple downstream ML projects.
If you have a hundred teams that are gonna use your, you know, foundation model as a base to speed up by 20%, that's a pretty, you know, good ROI calculus. And also the cool thing is that, you know, a lot of how these mo, these foundation models are trained. It's not just pure, okay, go look and do self supervision, train yourself.
You can also use downstream specific tasks through what's called multitask supervision to kind of make it smarter. So basically you can both get a boost for those downstream a hundred tasks, but you can also create this powerful kind of data or supervision flywheel that makes the base better if you own that.
So I think a lot of enterprises are getting quite interested in what if I own that and I both speed up everything else I do downstream, but also kind of have this way of kind of collecting and scaling all the work we do. Um, that's gonna be very interesting and. It doesn't necessarily mean open source versus closed, it just means they need to be able to own their copy of the model.
And there's some interesting models from the cloud players and others emerging around that, along with open source. Yeah, and that's a great point. Will, um, sorry, Alex, the, the one thing that, uh, we definitely should mention on that front too, we're talking about. Foundational model, you know, the, the more private foundational models, then the, the os, the OSS foundational models.
I, you know, in both of those cases, I've been seeing a huge rise in using vector databases to be able to connect that you, you know, it's another, you know, I think about it as two ways. You, you know, there's, there's a privacy component where you might have to use, um, And an OSS model or build your own. Um, but then there's also a connecting your data component.
When you're fine tuning or you're training up your own l l m, it's one approach to be able to give, you know, your, your l l m context of your use case and your data. Another way of doing that, if you wanna just, if you can use just an off the shelf l l m, is to be able to connect to, um, a vector store and pull in context.
From, you know, and I've been seeing a lot of different use cases for this getting actually deployed into production. Things like chat bots or I wanna be able to answer questions on these certain documents. And what teams are doing is, is really supplementing the user queries with context from their own knowledge base.
And it's, it's a way less complex approach to. Fi, you know, building up your own l l m or fine tuning your l l m, as you add more documents or knowledge to your knowledge base, you can, you can kind of augment it without having to retrain the whole ll m. Um, but it's a great way to connect the LMS to your own data.
And, you know, the way I think about it is if you can use, you know, an out of the box kind of, kind of public foundational lum, like OpenAI, well, You can directly call it, get, get back a response. If you need to connect it to your data, throw in a vector database. If it's still not giving you good responses, you wanna fix a more general problem, um, you know, you might need to fine tune it.
And then if, if you really can't do any of those options, then I'd kind of add, you know, we've been seeing people then resort to using more of an O OSS type of l lm, but typically that's kind of the. Process, I've been seeing people go through to select the right approach for what, what makes sense for their use case.
I, yeah. Phrasing of like a Go for it. Sorry. Will you go, uh, I think we should, we have about seven minutes left. Uh, hear any hot takes if folks have about how ops is going to change, but wanted to, uh, just tie back, uh, Alex's point and Chris's point on the enterprises. I think if you're mature and you have high value use cases, Um, you probably have an evaluation stack and already existing systems in place.
And for a lot of these companies, they have a little bit of leeway to try open AI or or other mature models and do a like, for, like comparison offline. And I, I'm hearing that from a lot of folks I speak to. And if so, if they've data sets already that are produced for or by ml, uh, systems or even human labeling, they can already see.
How well these, uh, LMS are performing. And then in some cases they're, you know, cutting down nine months of human labeling to, you know, minutes or hours of work. Um, so there's definitely demand. And I think lot, a lot of companies, they see this as a differentiator. So there's pressure to get adoption and now this is figuring out like, how do we actually do this?
And they're exploring open source and other ways to do this reliably. Um, But yeah, par I'd love to also like, get into a topic of perhaps like how does the stack change, right? Like vector stores versus feature stores. Um, or, you know, any other, uh, kind of stack changes maybe on the observability side. Um, have you seen any, any kind of like use case differences there?
Yeah, I, so that Great, great question. So I think. Vector stores have definitely, I'm not the first person to say this, but vector stores have definitely, you know, core part of what I'm seeing in the L L M op stack. Um, I think the embedding analysis and being able to understand, uh, like we've been doing it on the deep learning side for CV and nlp, but definitely, um, something that's been cropping up more on the observability side for LLMs and then, Um, one other n nuanced point that I, I think I'd mentioned is that, um, for l l m observability, if you are using a vector store, being able to trace back.
Was the right context added? If the right context wasn't added, how do you trace it back to, you know, are the most similar documents that are selected, necessarily the most relevant documents or all the types of questions that we're getting asked? And we're seeing actually our, our users troubleshoot, um, in, in production.
So, Um, I think the, the observability stack for LLMs will definitely need to have some, some components of looking at the Vector store and, and being able to troubleshoot the context that that was retrieved.
Um, you know, one thing that I think is different with Lll mops is exactly what you, you said a part of this like, need to really debug or diagnose or, or get into these ever more and more complex chain of, of agents. Like all of the, the cool stuff trending is it's like pretty complex and you can have many different calls out to different submodels or different services and, um, if any one of those things goes wrong, You're gonna get a bad result.
And then, uh, Willem, you mentioned this like evaluation stack, like companies that have a mature evaluation function. I mean, this is, this is really important. Like it's, we're getting a lot of software developers now using these things and it works and it's a cool demo, but if you can't measure how good it is, there's, there's, you know, that's not gonna be good in the, in the long run.
And it, it made degrade and, um, So I think these are the, the big differences here and, and you know, there's gonna be a lot of tools that, that will hopefully help solve these things, but it's, it's early days. I think one way to look at this was, go for it. You go. There's one quick point. I think the LMS versus Moops comparison puts them kind of at the same level.
But another way to look at this is if you look at a feature store, you know, The features could be produced by an L l M, right? It could be, you know, this is a system that is at a higher level and it compiles down, um, your data pipelines or produces the code, produces the features and touches on the points that Alex met of the specialist, right?
Like, you know, you could start with the lm and then ultimately you have a more performant, perhaps more brittle, but reliable, code driven system that is optimized for your use case. And, and so they're not perhaps peers in the same level, but more kind of, Student teacher, uh, Alex, you can go. Yeah, I definitely agree with that.
Um, and, and with what, what Chris was saying in terms of how these systems, I mean, there've always been complex chains of interdependencies between deployed AI powered applications, but the kind of democratization of that and the kind of blooming of complexity, we'll see how many of those actually get shipped to production.
But, you know, it, it's definitely kind of coming more to the forefront right now in really interesting ways that require this kind of. More software like debugging through these long traces. Um, I guess, uh, I can give a, um, let's see, a quick hot take, a medium take and a, and a. Cold take, I don't know, which is just like a lukewarm statement, but the hot take I already said is, I think a lot, a huge chunk of the models people actually wanna build, uh, the problems they actually wanna solve With foundation model stay.
And the enterprise will just boil down to, uh, traditional ML models and traditional ML lops. We wanna classify this. It'll start with a foundation model, but it'll end up with just a smaller, uh, model. And you could look at this and, you know, um, look at, uh, um, You know, some of the mature deployers of foundation models for years, like mainly I'm referring to Google, right?
They don't serve the biggest models in production. They distill down to smaller models, um, uh, uh, you know, in, in ways like the work we do with them. So I think that's the hot take is that a lot of mops will just boil down to ML lops as hot as I can get for a conversation that's nerdy. Uh uh, you know, right now when there's so much agreement in the group too, maybe a medium take is, um, I think evaluation I agree with, you know, well, I'm agreeing, so not that hot.
That, um, evaluation. Super important, super challenging. We've taught a generation of data scientists basically, uh, well not to peek at the test set, right? Don't test that hat hack, don't cheat. And what that has translated into is don't look or think at, don't look at or think about the test set and. That's always been a problem in machine learning, right?
You know, um, you actually have to engineer your test set to be representative of what you want your model to do in production. That's actually still a problem for, you know, simple predictive models, but it gets tenfold more messy, tenfold messier when you now have, um, you don't have a simple accuracy score.
You need to build like a custom benchmark and you need to, you know, be careful about where the data came from and whether it was in the training set of some gigantic, you know, web wide, uh, trained model. And so I think evaluation is, is very much in the hotspot right now. A lot of these demos, they look amazing until you actually do a rigorous evaluation.
Then you're like, it's still really cool, but it's not production ready. But we're not even, we're baby steps there. So that's the medium take. And then the lukewarm take might be just that. This goes back to a nice articulation of part of you were making up kind of the, the, the steps of things you do. A lot of the questions that people are asking about, like, should I do a prompt or can I just r it?
I heard that phrase at, at Microsoft build, uh, you know, retrieval, augmented generation of putting in a context, uh, database. Or do I need to fine tune? A lot of these are just pick the right tool for the right job, right? And it, and it really is just, it's always the problem in data sciences. Start with the simplest approach.
And you know, in some cases, yeah, simple, prompt, you'll get a great solution. And in other cases, well your model is missing some context from a structured or unstructured data store. So plug in a database. In other cases, it's actually the, you know, ability to get the right decision. Boundary is not even with the right information is off, so you need to fine tune it.
In other cases it just, Doesn't have a good enough representations for your specific specialized domains, so now you actually have to pre-train. So, you know, I think it'll, it'll all come back to it's not an either or. It's just a pick the right tool for the right job. That's my lukewarm take. Um, kind of just plus winning what Aparna said.
Yeah, you've all been a great panel, but I believe you're run out of time. Um, Demetris. Yep. Yeah, so this is how I know, oh, you can't see me, but this is how I know the panel's full of executives. They're right on time, literally one minute over and they're like, shit, we're burning money here. We gotta go run businesses.
So I love that. I just wanna know last question from me, and there's some incredible questions in the chat, so maybe we can convince some of you to go into the chat after this, but Alex said it. All righty, so I don't need to hear it again. The rest of the panel, do I need to rebrand? Is it ML Ops going away?
Do I need to rebrand in LLM ops? That's what I wanna know. Chris, I'll go with you first. Uh, I'm in agreement with, with Alex's Takes, I think, uh, it is, it's spot on. Um, it is, it is still early days. We're all kind of like watching and, and wondering how this is gonna, how this is gonna play out, but, um, I don't think a rebrand is necessary.
The, the core fundamentals of let's, you know, measure and have, uh, an audible, auditable system of record is, is gonna hold true. And, um, that's good for, for my business. So maybe I'm, I'm seeing the world as I want to see it, but, well, we'll wait and see. I love it. Well, yeah, I mean, maybe a little biased from my side, but I'll go with that one.
What about you, Aparna? I feel like I'm gonna. Maybe be a little dissenter here. Um, I, I think that, um, it's, it's definitely changed how people think about, um, but like, I think the potential of LLMs is massive. I think it's changed how people think about, um, you know, should I build this or should I just call an api?
And, and, and choose that approach. Um, I think the world of today, you know, LLMs probably don't replace all of traditional ML use cases. Come on. It's so expensive. It's latent. It's not gonna work for internet scale applications. But, um, there's some things that I can't imagine people like sentiment analysis or classification.
I can't imagine people, I. Continuing to build models for, for that when an L l M does so good right out of the box. And so I think, uh, you know, it's probably not an overnight, we're all LLM ops. Uh, we're, we're kind of living in this middle ground of statistical ai, still the most commonly deployed ML that's out there, but LLMs are coming for us, and I think all of us are kind of thinking about, well, Yeah, I actually just had a call this morning where they said CEO has three LLM initiatives, and those are the biggest ml, the biggest projects that our ML or DS organization focused on.
And it's, it's a little bit of, I think this, you know, wave that's coming towards us, and we're all, I think we should, you know, the needs of this model type or this new wave might be different. So being, being able to adapt to something, I, I think we're, we're all trying to do. Yeah, I think from my side, um, MOPS rolls off the tongue a little bit better, so I think you're safe on that front.
But I a hundred percent agree with partner. Um, yeah, I think. Uh, I'm excited about the new use cases that it unlocks reasoning and judgment. Certainly, um, these egen flows and the debugging thing was an important point earlier. There's a lot to be learned still. I think it's early days, but, you know, nobody's ever said, you know, there's an existential risk to, you know, us from XG Boost.
Right? There's clearly a step change with LLMs and we're all waiting to see how this impacts us to, um, over the next. Couple of years. Um, but yeah, I think for now wait and see is the best course of action. And last, I gotta get a word in. You were, you were trying to pull a fast on me. You know, I think it should be FM ops.
I know you said that last time. I'm never, that never happened. And keep ignoring it. We can't have another ops man. Gimme a break. There's too many ops. Oh. So yeah. Foundational model ops does make a lot more sense though. I will give you that.
Socha. Last one before I kick everybody off. Is it in or is it out? Am I rebranding or what? No, you're in for long haul. You've got the right name and you gotta stick to it. And we all know you as somebody who founded this community, and we're gonna stay true to it. There we go. All right, so thank you all for this and thankful Chat.
I, I. I do not know if I have ever been on a virtual call with so many hard hitters before. And it is, uh, an honor to be able to say that I rubbed shoulders with you all. And for those that are in San Francisco, I'll be there in two weeks. So hopefully we can meet in person. I will. I'm gonna kick you all off now because we got some trivia games to play, but this has been absolutely awesome.
Thank you all for the fun times and insights and good stuff.
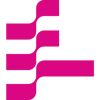