Model Monitoring in Practice: Top Trends
speakers
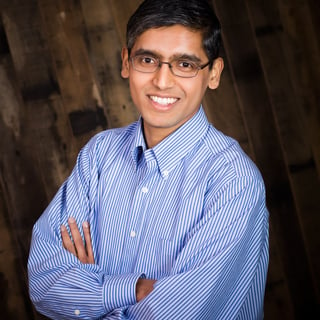
Krishnaram Kenthapadi is the Chief Scientist of Fiddler AI, an enterprise startup building a responsible AI and ML monitoring platform. Previously, he was a Principal Scientist at Amazon AWS AI, where he led the fairness, explainability, privacy, and model understanding initiatives in the Amazon AI platform. Prior to joining Amazon, he led similar efforts at the LinkedIn AI team and served as LinkedIn’s representative on Microsoft’s AI and Ethics in Engineering and Research (AETHER) Advisory Board. Previously, he was a Researcher at Microsoft Research Silicon Valley Lab. Krishnaram received his Ph.D. in Computer Science from Stanford University in 2006. He serves regularly on the program committees of KDD, WWW, WSDM, and related conferences, and co-chaired the 2014 ACM Symposium on Computing for Development. His work has been recognized through awards at NAACL, WWW, SODA, CIKM, ICML AutoML workshop, and Microsoft’s AI/ML conference (MLADS). He has published 50+ papers, with 4500+ citations and filed 150+ patents (70 granted). He has presented tutorials on privacy, fairness, explainable AI, and responsible AI at forums such as KDD ’18 ’19, WSDM ’19, WWW ’19 ’20 '21, FAccT ’20 '21, AAAI ’20 '21, and ICML '21.
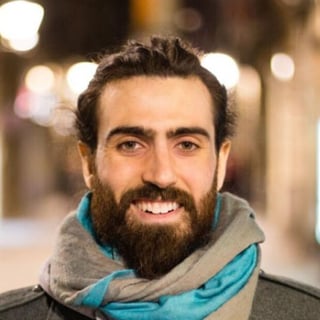
At the moment Demetrios is immersing himself in Machine Learning by interviewing experts from around the world in the weekly MLOps.community meetups. Demetrios is constantly learning and engaging in new activities to get uncomfortable and learn from his mistakes. He tries to bring creativity into every aspect of his life, whether that be analyzing the best paths forward, overcoming obstacles, or building lego houses with his daughter.
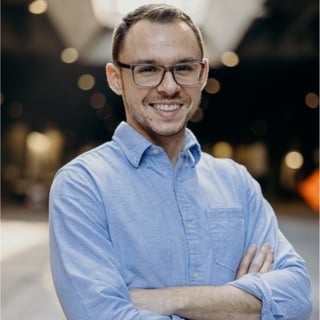
Mihail is a co-CEO of Storia AI, an early-stage startup building an AI-powered creative assistant for video production. He has over a decade of experience researching and engineering AI systems at scale. Previously he built the first deep-learning dialogue systems at the Stanford NLP group. He was also a founding member of Amazon Alexa’s first special projects team where he built the organization’s earliest large language models. Mihail is a serial entrepreneur who previously founded Confetti AI, a machine-learning education company that he led until its acquisition in 2022.
SUMMARY
We first motivate the need for ML model monitoring, as part of a broader AI model governance and responsible AI framework, and provide a roadmap for thinking about model monitoring in practice.
We then present findings and insights on model monitoring in practice based on interviews with various ML practitioners spanning domains such as financial services, healthcare, hiring, online retail, computational advertising, and conversational assistants.
TRANSCRIPT
Model Monitoring in Practice: Top Trends // Krishnaram Kenthapadi // MLOps Coffee Sessions #93
"The scientist is not a person who gives the right answers, he is one who asks the right questions." - Claude Levi-Strauss
The Power of Questions
A wise man knows that answers have no value if you’re asking the wrong questions. And if you’re looking for the right questions when it comes to productionizing ML in a responsible, ethical, and most of all explainable way - look no further!
We have the Chief Scientist of Fiddler AI, Krishnaram Kenthapadi, who has been in the ML game since 2006, sharing his wisdom with us as well as his views on the latest trends. He shines a bright light on some very important topics we should all put more thought into both as individuals and as a community.
From "Why should we care?" to realizing the importance of monitoring ML in production
The fact that ML explainability is becoming a hotter trend by the day is undeniable. Each day there are more and more tools brought to the market trying to solve issues around these topics. This only proves how big of a problem explainability is becoming.
The importance of monitoring your models is recognized by companies due to the social responsibility that comes with it, AI regulations, and for its impact on business and bottom-line metrics. Still, we've yet to reach the stage where but everyone along the process realizes the value of it, in addition to organisation leadership.
The expectation, as Krishnaram shares, is that only a few more years down the road monitoring and explainability will be getting more and more traction as companies start using not just 1 or 2 models but perhaps hundreds of thousands of models in their products.
The AI Hierarchy of Needs
If you haven't yet deployed your first model then - yes, maybe monitoring will be the last thing you would worry about. Krishnaram recognizes that before getting into deep thoughts on monitoring, that we all shouldn’t forget that the ML aspect is only a small part of the entire MLOps ecosystem. It might be the fanciest part and that most DSs and MLEs gravitate around the related topics, but the rest of the ecosystem is as important for a successful project and ultimately a successful business. And as many of the experts we had on the podcast were noting, it’s always good to “start with the end in mind” when thinking about ML products. In other words - monitoring might not yet be your biggest concern, but doing responsible and explainable ML should be a big consideration in mind from the get-go of any project.
"Things start with asking the right questions"
According to Krishnaram’s opinion, often asking the right question is missing when thinking about ML pipelines. Questions like:
- Should we even be using ML?
- Are we collecting the correct representative data?
- Do we need to measure biases?
- Is the model having similar predict behavior across different user groups?
- Is the model starting to degrade in performance when in production?
- Why the model is giving such predictions?
- To what extent do we as humans want an explanation for every prediction?
- To what extent are we comfortable trusting one model if we don't understand how they are working?
- How we as humans are shaping our views of these new changes?
The list goes on. It's not just technical problems that we should be thinking about, but also AI regulations and how people are interacting with and being impacted by AI.
The ethical side of AI
When it comes to ethical issues and labor, people are mainly thinking about manual work. What comes to mind most often is fashionable sneakers made in a sweatshop or coffee beans hand-picked by small African children. But have you thought about how datasets are built in that same ethical aspect?
Some questions that Krishnaram recommends we ask ourselves are:
- Is the data you're using sourced ethically
- Were the people aware of the purposes of their labor?
- Were they paid fairly?
- Could the answers to these questions affect the quality of the datasets and how?
- How would that affect your ML-powered product and end-users?
This is another priceless checklist that needs to be printed out and hung on our walls to remember often.