Overcoming Bias in Computer Vision and Voice Recognition
speakers
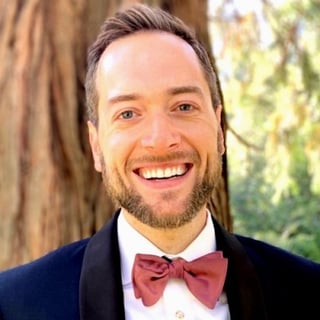
Versatile technologist with experience in software systems, technical marketing, public speaking, and relationship building. Skilled coder, collaborator, designer, and educator.

Rajpreet Thethy has 20 years of experience working in Tech. She currently serves as a Staff TPM of Data at AssemblyAI, a series C startup building industry-leading Speech ASR models. Prior to AssemblyAI, she was at Google for ~20 years working across various functions including Operations, Finance, and Machine Learning / AI. She holds a Bachelors in Computer Science and MBA from Santa Clara University in California. When she’s not working with data, Rajpreet is an avid painter and loves being in front of a canvas.
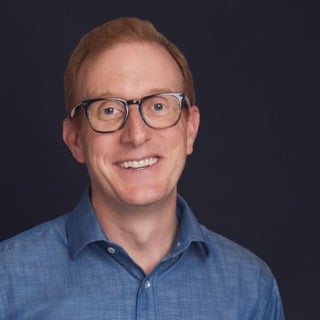
Doug Aley has spent his 25 year career helping to found, lead, and scale technology ventures. He is currently Paravision's CEO and an operating partner at Atomic VC. Prior to Paravision, Mr. Aley held positions in product management at Amazon ($AMZN), was VP of Marketing, Product, and Business Development at the voice recognition company, Jott Networks (acquired by $NUAN), helped Zulily scale from $100M to $700M in sales as VP of Product and Corporate Development in the run up to the company’s IPO ($QVCA.O), held the same roles at Room77 (acquired by $GOOG), and started and led Minted’s (private company) digital growth team. At 19, he co-founded and was CEO of his first company, Level Access (sold majority stake to JMI Equity in 2017). He is an investor and advisor in numerous technology startups and sat on the Board of Trustees at The Marin Montessori School for the past 6 years (stepped down in 2023). Mr. Aley holds a BA from Stanford University and an MBA from Harvard Business School, and lives in Marin County, CA with his wife, Susan, and their two boys.
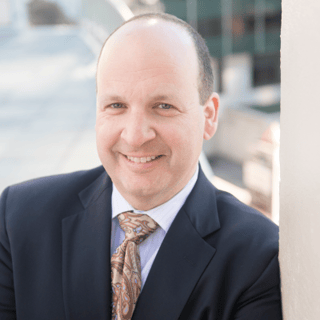
Peter Kant is the Founder and CEO of Enabled Intelligence, Inc, an artificial intelligence technology company providing accurate data labeling and AI technology development for U.S. defense and intelligence agencies while providing meaningful high-tech employment to veterans and neurodiverse professionals.
Peter has two decades of experience at the nexus of business, government, technology and policy including leadership positions in state and federal government, large companies, start-ups, and non-profits. He also currently serves on multiple corporate boards and advisory boards of venture funds including: Culpepper Garden, Distributed Spectrum, Ditto, Picogrid, and Spectrohm.
Peter has led multiple high technology companies over his career. Previous roles include: CEO of Accion Systems, a space satellite thruster hardware company, developing and manufacturing engines for satellites; CEO of Synapse Technology, an In-Q-Tel backed AI security company, Executive Vice President, Federal Partnerships at SRI International a non-profit research and development institute headquartered in Silicon Valley; and as Executive Vice President at OSI Systems, Inc, a publicly-traded security and medical technology firm.
Peter started his career in government service working in political positions at the state and federal levels. He worked in the U.S. Congress; served as Policy Director for the Texas House of Representatives; and served as an appointee in President Clinton’s administration.
Peter earned his undergraduate degree in politics and economics from Brandeis University and his Masters of Public Policy at Duke University. He is a native of Houston, Texas and has lived in Arlington, Virginia for over 25 years.
SUMMARY
This panel will explore the critical issue of bias in AI models, focusing on voice recognition and computer vision technologies, as well as the data used to power models in these domains. Discussions will cover how bias is detected, measured, and mitigated to ensure fairness and inclusivity in AI systems. Panelists will be invited to share best practices, case studies, and strategies for creating unbiased AI applications.
TRANSCRIPT
Skip Everling [00:00:09]: Good afternoon everyone. This is going to be the overcoming bias in computer vision and voice recognition session. There was a bit of a mix up with the stages, so hopefully this is the talk that you want to be at. It definitely is the talk you want to be at, whether or not you intended to be here. My name is Skip Everling. I'm the head of developer relations at the Helena. I'm joined today with three knowledge experts in the domain that we're about to talk about. Very excited about it.
Skip Everling [00:00:35]: The key idea here is of course, bias. Bias, you already know I probably don't have to tell you. Huge impact on everything from user experience to ethical considerations to regulatory and legal framework issues, which we have state Senator Scott Weiner talking a little bit later, who's thinking about this very deeply right now. All kinds of issues that bias directly relates to and particularly how data relates to bias and creates bias. So I'm going to be asking each of our panelists about that today. I'm going to introduce them one by one before we get started. First up is Rajpreet Tethy. She is a TPM at AssemblyAI and she has deep experience in data collection, transcription and annotation operations around ASR models, automated speech recognition models.
Skip Everling [00:01:27]: Prior to AssemblyAI, she spent almost 20 years at Google. She worked on other voice recognition projects there. We're thrilled to have her with us on the panel today to share her expertise on the kinds of bias that appear in the voice recognition domain, with a particular focus on proper data management and how that's key to bias mitigation. Our next guest is Doug Ailey. He's the CEO of Paravision, also an operating partner at Atomic VC. Pairvision is one of the most trusted providers of computer vision technology, thanks to their industry leading accuracy and commitment to minimizing bias. Pairavision consistently ranks among the top global performers in NIST's gold standard face recognition vendor tests. I'm especially eager to hear from Doug today about the methods that pairvision is using for addressing bias in facial recognition technology, given how impactful it can be in the lives of everyday people.
Skip Everling [00:02:19]: And last but not least, we have Peter Kant. He's the founder and CEO of Enabled Intelligence, an AI technology company providing highly accurate data labeling for highly critical scenarios including us defense and intelligence agencies where the quality of data annotation directly relates to life and death applications. Peter has led multiple technology companies in his career and brings deep experience in providing secure and accurate data labeling services. He's leveraging that experience to minimize bias through a carefully considered diverse labeling workforce, including a deliberate focus on incorporating neurodivergent labelers, which I'm very excited to ask him about. So please join me in welcoming our panelists before we get started with questions. So, panelists, the theme here for today, we're going to go in three segments. First off, I'm going to ask you questions about how you define and identify bias in the domains that you work with. I'm gonna give you an opportunity to talk about any real world examples, things that come to mind as far as how bias impacts the outcomes in the real world.
Skip Everling [00:03:25]: And then the most important bit, which I'll leave plenty of time for at the end, how you're mitigating bias. What strategies have you learned? What methods are you doing? What techniques are you finding most effective in addressing bias? And then if we have time at the end, we'll talk a little bit about where you see addressing bias going in the future. Rajpreet, I'm going to start with you. So you're working with the audio domain in particular. So I'm very curious, what types of biases are you encountering in automatic speech recognition?
Rajpreet Thethy [00:03:55]: So, the most common types of bias in voice recognition and ASR systems typically revolves around things like age, gender and accent types. So if you think about an ASR's fundamental function is to be able to recognize speech and convert it to text, bias in ASR means that the model is not able to do this well or not able to do it well for certain groups or certain people. So, for instance, if the model is gender biased, it might not be as well at recognizing male voices or female voices or vice versa. Similarly, if it's age biased, it might not be as good as recognizing kids voices versus adult voices. Essentially, bias can be harmful on many different levels, right? From a fairness perspective, bias can be harmful because it has the potential to perpetuate and reinforce stereotypes, these age groups or genders in society. And from a business perspective, it's ultimately going to lead to less usage and loss of trust in your brand and your product.
Skip Everling [00:05:06]: What I'm curious about in particular is how are you seeing the biases impact the performance and fairness of these models?
Rajpreet Thethy [00:05:15]: So there's been a lot of, there's been a lot of studies around, like voice recognition systems not being able to recognize for certain groups, right? So, like age voices, as I mentioned, or for gender types. So there's been a lot of studies around, for example, voice agents working well or being able to better recognize voice male voices rather than female voices.
Skip Everling [00:05:42]: Thank you. Doug, You're working with vision technology in particular. So paravision has accumulated a lot of experience developing these models. You're working with a lot of different data. What kinds of bias are you seeing in vision AI models?
Doug Aley [00:05:56]: The exact same bias that Rajpreet is minus the voice part. And I'm laughing to myself because I ran a voice recognition company back in 2008. We dealt with the same stuff. So thank you for continuing to do that work. Yeah, so what we see are sort of the intersection, and I'm sure you see some of this too. Rajpreet of environment as on top of demographic profile. So we see age, gender, skin tone, effectively multiplied, those topples multiplied times, environmental factors like light saturation, sort of quality of the camera, of the optical sensor, and in distance, to name a few. But there's a sort of much broader matrix than that.
Doug Aley [00:06:42]: And for us, we provide APIs and SDKs as kind of developer tools for companies that want to build high quality, high accuracy face recognition into their products. And so, but it's not, we don't just focus on one use case, we focus on eight different kind of core sectors that range from screen in front of your face like this, to which we would call cooperative versus semi cooperative. I'm passing by an optical sensor versus non cooperative think in an enterprise security environment. And what's perhaps non intuitive is that by doing all of those, you actually reinforce the quality in each individual use case. So I think that's where we've had some breakthroughs, that it's non intuitive. You think that if all you did was focus on cooperative use cases, you'd be very good at that. It turns out being quite the opposite, and we're very fortunate. You mentioned NIST.
Doug Aley [00:07:39]: The National Institute of Standards and Technologies has had a vendor benchmark for the last 15 or so years that we get to take advantage of. So every year we and a host of another 250 companies submit our face recognition models for objective third party benchmarking. And I think face recognition is sort of singular within the AI community, and that it's objective third party benchmarking versus self benchmarking. And so I hope NIST starts doing more work like that. In terms of the impact to everyday consumers. I think the bias is pretty clear, and it's very, very similar bias if you don't have access to certain systems, because the face recognition system can't understand who you are. And there have been several high profile cases of sort of less quality models screwing up, screwing this up. I think London Metropolitan Police, the Detroit Police Department, for what it's worth, we do not sell to law enforcement, state and local law enforcement, because the training and sort of legal frameworks aren't in place yet to make that really great.
Doug Aley [00:08:52]: So I think that's the other piece. And bias is you have to realize in some applications you're going to have a very human element that you want to make sure is interpreting the results of the face recognition models correctly.
Skip Everling [00:09:04]: Got it. Thanks, Doug. Yeah, just a quick follow up. Very quickly, when you're discovering new types of bias, what are you discovering? Obviously there are some obvious demographic bias types. What about, is there things beyond that?
Doug Aley [00:09:16]: Yeah, I mean, it's usually, again, for us, it's somewhere in that matrix where we don't have enough data. And usually we have very, very tight feedback loops with our customers. We don't host anything, but we do have very tight feedback loops with our customers. So if they see something that feels like we're not getting it, we then go and do a data collection. And we're very careful with our data collection, too. Everything is biomedical. The people that are having their data collected, there's full biometric consent for that data collection. And so we do a data collection.
Doug Aley [00:09:50]: Let's say there was a. We do really well on everybody, but there is a sort of people, younger people wearing hats. I'm using that as an example. That's not something. That's actually something we do quite well on, but younger people wearing hats of a particular sort of skin tone. Right. That would be an example of something where somebody would say, we had a false negative or a false match there and we'd like you to look into it. We then go look into it, do a data collection, reincorporate it into our training models, something, for what it's worth, that Kalena helps us a whole, and we're one of their, if not their first, one of their first customers, and use it religiously.
Doug Aley [00:10:28]: So if you're here and you're already a customer, you already get it. If you're not, become a customer.
Skip Everling [00:10:36]: Thanks for the plug, Peter. Very eager to hear from you. You're working with all different kinds of data, but particularly like highly sensitive applications. When you're looking for bias, what are you detecting? What is the process you have for identifying bias, particularly for the types of clients you're working for? What do they really care about? What's the most impactful for them?
Peter Kant [00:10:56]: Sure. Good question. Because I know you wanted me to stroke your ego by saying it was a good question. No, I'm just kidding. Yeah. Our clients, as skip talked about, we do a lot of military and intelligence and those sort of applications. And our clients are very, very concerned about making a very grave decision, incorrect decision, based on the production of the models or the data or an incorrect one, or being guided in a different way that isn't as helpful. And where we've seen bias is not.
Peter Kant [00:11:26]: I mean, there are, what I would say, the more traditional definitions of bias relative to race, ethnicity, gender, age and the like. We see bias as bias. We do see that, of course, but more so as bias in terms of how previous things have been done. Right. So when you're using an AI and using old data, you're using legacy data to train that AI, which is the best way to do it, the way something was done. And in the parlance of our customers fighting the last war to lose, the current one is a typical format of bias. And how do we detect that? Case in point, maybe we spent. The US has spent 20 years at war or in conflict in the Middle east, and is now supporting allies in Ukraine and Southeast Asia.
Peter Kant [00:12:11]: To a degree, those biomes are completely different. So there was a whole bunch of computer vision models that were trained with desert backgrounds, mediterranean backgrounds, certain types of country, of origin of military and materiel planes from this country versus that country which, when we were in the snowy steppes of Ukraine, none of those models worked. That's a bias. It's not the same as an age bias. Similar to operating in drug detection and counter narcotics activities in Central America is quite different from looking for chinese ships and north korean missile sites in Southeast Asia or in Korea. And those sort of biases are the typical biases that come up. But then there's the operational ones, right? Used to working with, oh, a flanks of tanks looks like this. This is how a war is fought.
Peter Kant [00:13:06]: This is when people are moving. We're now seeing a lot more of being able to model activities of adversary warfighters through social media inputs than it is through overhead imagery and the like of what's coming through. And so the desire to go a bias of, well, if I don't see it in the satellite, then it's not happening, but there's this other signal that's coming from another source, social media. In this case, that bias happens a lot.
Skip Everling [00:13:31]: Very interesting. Very interesting. I want to ask a follow up here in terms of just kind of slightly segueing into this thinking about, is there. I know that given the sensitive domain, you maybe can't talk about specifics, but what would be an example of the type of scenario where mitigating bias was essential in order to achieve some important outcome or to avoid the loss of life or a big mistake in a wartime situation.
Peter Kant [00:14:00]: Yeah, I'll take one that's not war oriented because the public sector does a whole lot more than just blow stuff up. And I'll look at something that we were looking at in helping the Department of Treasury. There is Department of Treasury is looking at home loans, mortgages, the health of those mortgages over time. Who is getting and maybe help some tools for the Federal Reserve bank to do some regulation, Consumer Finance Protection Board to do some regulation about mortgage activities and things like that. And they wanted to see were banks awarding mortgages fairly and they had originally sucked up basically every loan application, not everyone, but loan application and loan award over the last 70 years and the initial find and trained an AI to go through and say, hey, is bank of America doing a good job? Are they being fair? In initial reports? It wasn't bank of America. So I'm not going to say bank of America was biased. That's not true. But just using them as a Pete's bank.
Peter Kant [00:14:57]: Pete's bank do a good job. I have a very big bank as my daughter is in college. Anyway, the question was, is it doing well? And initial feedback from the bank, oh, these look really good. However, that 70 years of data that was used to train the model, which was perfectly annotated by a great company and labeled by a great company, had inherent bias. Over the last 70 years, there's something called redlining where whole sections of towns in areas by zip code because they were primarily populated by persons with color, people of color, African Americans and the like, were denied mortgages. So the fact, just because they were in those populations, regardless of their ability to pay their credit history, things like that, the model said that denying mortgages in those places was still good. That was okay, because historically that had been what was done. That's not a data problem.
Peter Kant [00:15:48]: That's not a model development problem. That's an understanding what the inherent bias in the source data is problem. And changing that and identifying that and then training that out, if you will, and figuring out how to train that out. That was a big project that I think yielded solid results.
Skip Everling [00:16:02]: Right. Thank you, Rajpreet. I want to come back to you. So, similarly, what comes to mind when you think about an example of bias cropping up? But then also, I would love for us to start talking about what strategies you're using to mitigate that bias once you've identified what did you do in a particular scenario and how have you changed your procedures and policies.
Rajpreet Thethy [00:16:26]: Right, skip, I'll talk a little bit about bias in data and data collection. So since most AI models are going to rely on some form of data, mitigating bias in data is the first foundational step. And so some of the things that we do in terms of mitigating bias and making it more inclusive is, first of all, really focus on the feature or the product that you're building for, know the business requirements and derive, like, really good data requirements out of that, and then really focus on getting a diverse set of data. I think that's like a foundational first step. So making sure that the data comes from different sources with different, like, scenarios, different perspectives. We want to make sure. So, for instance, for training our ASR models, we want to make sure that the data we use to test and train those models comes from a variety of sources, speakers from different age groups, different demographics, different regions. And then third, we want to make sure that that data is balanced.
Rajpreet Thethy [00:17:32]: Right, and represents the real world. So where we find, like, insufficient data, going back to what Doug was saying, is we want to do additional data collection and augment it to ensure that it's representative of the real world. And then finally, like, when working with vendors, right, you'll often need to work with external vendors as data data sources. So you want to make sure that they're also following, like, the same inclusive business practices and practices to mitigate bias. So one of the things that I always look at when working with vendors is whether they have explicit consent from their participants and whether they're transparent. And they offer, like, metadata around how the data was collected and where it was collected.
Skip Everling [00:18:21]: Yeah, I think both of our other panelists will agree with you on that, Doug, I'm actually very curious to hear. So your experience on the same domain here, what are you using to manage your data collection and address bias? What are your policies?
Doug Aley [00:18:33]: Yeah, so, number one, I'd say people, and I don't mean people in terms of we use, I think we have over 30 at this point, videographers and photographers globally that we count on to do data collections for us and collected biometric consent, among other consents. But what I mean is, like, have a team that leads with curiosity and doesn't think that they're right about everything. And if you start there, then kind of everything else kind of follows its course. So hire for continuous improvement growth mindset and you do a lot of work. And I'll give one example of that. A few years ago, we ended up being number one in the world for the first time on NIST face recognition vendor tests. And our head of machine learning, Bhargava Sarala, after probably about 5 seconds of celebration, started going through the litany of things that we could do better. And that type of mindset, you take a little bit more time to celebrate, which we did.
Doug Aley [00:19:37]: But having that mindset of going back to work, especially when you're dealing with issues where somebody could be potentially denied service. So we are. Face recognition supports the id me that is sort of the backbone of the IRS's identity verification. So somebody could literally be denied sort of the ability to pay their taxes or be identified if we don't get things right. So taking it seriously, hiring for curiosity, and I would say one more thing. I'd reiterate literally everything that you said, but I would say one more thing is get in the arena, which is if you've got a use case that you're, that you're developing for, nothing beats being in the operating arena that you're going to actually launch in. And I think that's something that as software developers and I've been in the software development for 25 plus years now, too frequently we sit at our desks and think that we can throw more data at it and that that will solve the problem. You need to be with the customer in the arena and figure out what for us, kind of what lighting, what external factors are playing a part in that, and that kind of curiosity, that desire to get in there and figure out what's going wrong, is the best tool we have, right?
Skip Everling [00:20:53]: Yeah. And I want to ask you about this. I'm going to ask you about this as well, Peter. So when you're thinking about your data annotation, I'm curious. We talked about this a little bit before today, about your experience outsourcing and whether to insource and the considerations you're making there. I'm very curious because this is a big topic of interest for a lot of people who are trying to figure out how to best annotate their data without bias. I'm very curious to get your opinions here.
Doug Aley [00:21:19]: I would say it depends on the sort of field of AI that you're operating in and what you're trying to annotate. But we've not had as much success with data annotation companies and that maybe we're working with the wrong ones. But yeah, I would say we try annually working with a few different data annotation companies. And for us, inevitably, it's much more useful for people to provide the metadata themselves to say, when we're doing a data capture, they say they self identify that data. And even then, you have to have some other people actually looking at the data. Because, for instance, if you're going to use the monk skin tone scale, people will self identify actually as a different skin tone based on the lighting of the picture that they see. Whereas if you change the lighting for them, they will identify as a different color on that monkton skin tone scale. So you have to be very, very careful about how you set up those environments for that.
Doug Aley [00:22:27]: So I would say keep, always keep trying. And the things I would say, especially in the environment that we're in, the things that didn't work last year, may work next year. So keep at it and keep having those theses. I would actually put one more plug in, which is like, synthetic data is one that's come an incredibly long way. Still not there yet for face recognition. I don't know about voice, but when we look at the latest research coming out of idapp or anything like that, the rock curves are still not the right shape and for what we look for and the level of accuracy that we look for. But it's getting better every year, and so we'll keep looking at it every year.
Skip Everling [00:23:06]: Yeah, yeah, certainly that's a topic for a whole other panel. Synthetic data. Peter, how are you constructing your data annotation teams? What considerations are you putting into creating a data labeling workforce and workflow?
Peter Kant [00:23:19]: Sure. We took a very unique approach to this, which is we thought of data labelers as representing the human brain for some degree and all the variety that comes with the human brain. So the idea of looking at how a human brain looks at all this different data, human brains look at all this data, is a way of getting the most diverse and effective data set depending on the use case and the resources. So in looking at that, we looked at neurodiversity. So neurotypical to neurodiversity, the spectrum along what's commonly referred to as the autism spectrum. We found that by hiring a neurodiverse workforce heavily weighted towards folks on the neurodiversity spectrum, some with autism, it is a spectrum. So it varies and labels differ. That let us have a workforce that represented, let's say, common thought process of analyzing data to uncommon and unique and somewhat other helpful processes for doing that, that allowed us to catch.
Peter Kant [00:24:17]: Some are focused on context clues, some aren't fooled by context clues. Right. Some really good at finding tanks at a military base, some much better finding tanks where they shouldn't be, which is what sometimes the military is very interested in in terms of satellite imagery and all that variety of thinking allows for a variety of data, increasing diversity of data, reducing bias, reducing everything from winners bias to personal biases and thought biases, creating a much more fulsome and helpful data set, creating a less biased AI. And that approach has been very helpful to us.
Skip Everling [00:24:53]: Thanks, Peter.
Rajpreet Thethy [00:24:54]: Yeah, I just wanted to add a couple of points around data annotation. So I think like the question you asked about like going with in house versus vendors, some of the factors that, like I looked at is the volume that you're going to have. Right. And whether it's whether like vendors are willing to work with you if you have lower volume. And then the other thing is like how much control you want to have over providing like feedback and performing reviews and audits. Because I think there is this like co employment and compliance issues around giving vendors feedback on their annotation practices.
Peter Kant [00:25:31]: Not necessarily just that may be the case before, but. Or in some, in some places. But we work with a, we work with exceedingly small data sets because of some of the military and intelligence use cases or just because we're hungry. We're a small company and we'll take any money we can get. But more so is that feedback we've built into our. And we're an outsourced data to annotation. So I think that's a great way to go. Unless it's with one of our competitors, in which case it's a horrible way to go.
Peter Kant [00:25:55]: But what we built in is automated feedback and direct access to our customers, to the performance metrics in real time. You can see the error rates, what they're errored on, how many things the annotators are going through. Oh, now I want to. This confounder has popped up. I want to change the ontology. The occlusion went like this. I need to keep it. I don't want to keep it all that.
Peter Kant [00:26:15]: We take all that feedback and keep changing because in the end we only get customers if you guys build an AI model that you can sell to someone.
Rajpreet Thethy [00:26:22]: And that's why I'm talking to Peter after the meeting.
Skip Everling [00:26:26]: All right, I'm getting the signal to wrap up. But before we do, I want to give each of you as a panelist just a way to summarize what you see as the key points, key takeaways, key learnings that you've had in your experience working with bias. We'll go along.
Doug Aley [00:26:39]: I'll give you this one.
Rajpreet Thethy [00:26:41]: If I were to summarize, I would say focus on the foundation, which I think is data. Right. All AI is going to rely on data, so really focus on getting diverse, high quality data that represents the real world scenarios. Number two is, I don't think bias is a one and done thing. I think it's a continuous process. So you have to keep monitoring, you have to keep evaluating your models and making improvements, as you might find stuff. And then number three, I think, often gets overlooked. But using customer feedback and bugs as a way to detect potential bias or upcoming bias, and nip that in the butt as soon as possible.
Skip Everling [00:27:25]: Thanks, Rajpreet.
Doug Aley [00:27:29]: All of those things. And I would say start with building your team out to be diverse from the beginning and set a tone where they can be curious. Very, very curious about curious, and have a very good appetite for failure. Because when you find those failures, those are gold. We actually celebrate failure. It's one of our, believe it or not, one of our core values is to celebrate that curiosity and that failure, productive failure. And then the other thing I would say is benchmark. Like crazy.
Doug Aley [00:28:04]: Benchmark, benchmark, benchmark. Even if there aren't publicly available objective benchmarks out there, benchmark internally and get really, really good at turning the feedback that you get from those benchmarks into better models. And then I would say, if you can, and they exist and if they don't in your industry, really fight for them. Work towards getting objective, third party, public benchmarks developed. That actually ends up being a huge competitive boon to you, is if you're a small company or a large company, but it also ends up just making everybody better in the industry. And I know that our industry saw, basically, if you go back ten years ago, face recognition was at like 88% to 90% accuracy. And all of a sudden now it's at like, we're measuring error rates at one e to the negative six now. So that the only reason that is that way is through, like constant, constant benchmarking by any but everybody in the industry.
Skip Everling [00:29:03]: Thanks, Doug.
Peter Kant [00:29:05]: What they said, but I can't really add too much to it. The only thing I would say is add. I will add to it, is bias is not something that one can detect on their own. By definition. Bias is, in this case, the definition here is an inappropriate preference for one way or the other that's not really representative of actual or what the values may be. And that's the definition also of an individual. So having that diversity of a workforce, that diversity of thought coming into, is exceedingly important. And the only other thing I would stress is you cannot code out bias.
Peter Kant [00:29:36]: You have to actually actively work to do it. And think about, you know, as they were talking about, be with the customer, be in the playing field, see where the act, the bias is happening and work that way. You can't just guess at it and hope that the, the signal will come out of the noise.
Skip Everling [00:29:50]: All right, well, thank you, Rajpreet, Doug, Peter, really appreciate you sharing all of your insights and your expertise. And thank you, everyone, for listening. Enjoy the conference.
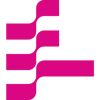