Practitioners Guide to MLOps
speakers
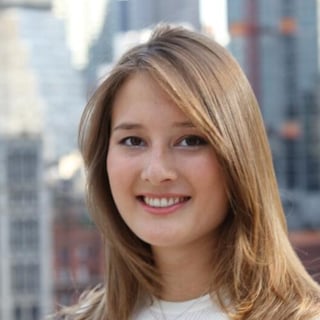
Donna is a Solutions Manager at Google Cloud, responsible for designing, building, and bringing to market smart analytics and AI solutions globally. She is passionate about pushing the boundaries of our thinking with new technologies and creating solutions that have a positive impact. Previously, she was a Technical Account Manager, overseeing the delivery of large-scale ML projects, and part of the AI Practice, developing tools, processes, and solutions for successful ML adoption. She managed and co-authored Google Cloud’s AI Adoption Framework and Practitioners' Guide to MLOps.
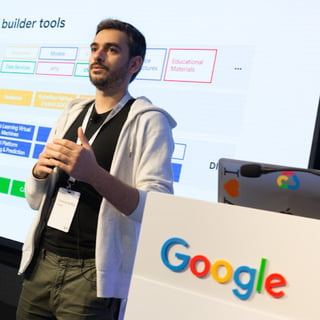
Christos is a machine learning engineer with a focus on the end-to-end ML ecosystem. On a typical day, Christos helps Google customers productionize their ML workloads using Google Cloud products and services with special attention on scalable and maintainable ML environments.
Christos made his ML debut in 2010 while working at DigitalMR, where he led a team of data scientists and developers to build a social media monitoring & analytics tool for the Market Research sector.
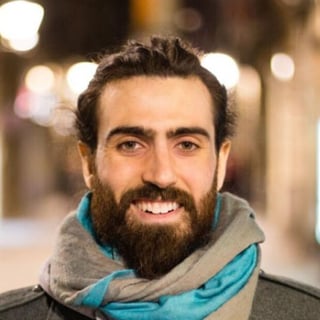
At the moment Demetrios is immersing himself in Machine Learning by interviewing experts from around the world in the weekly MLOps.community meetups. Demetrios is constantly learning and engaging in new activities to get uncomfortable and learn from his mistakes. He tries to bring creativity into every aspect of his life, whether that be analyzing the best paths forward, overcoming obstacles, or building lego houses with his daughter.
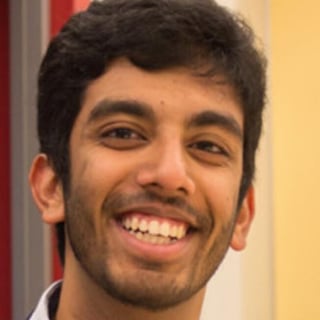
Vishnu Rachakonda is the operations lead for the MLOps Community and co-hosts the MLOps Coffee Sessions podcast. He is a machine learning engineer at Tesseract Health, a 4Catalyzer company focused on retinal imaging. In this role, he builds machine learning models for clinical workflow augmentation and diagnostics in on-device and cloud use cases. Since studying bioengineering at Penn, Vishnu has been actively working in the fields of computational biomedicine and MLOps. In his spare time, Vishnu enjoys suspending all logic to watch Indian action movies, playing chess, and writing.
SUMMARY
The "Practitioners Guide to MLOps" introduced excellent frameworks for how to think about the field. Can we talk about how you've seen the advice in that guide applied to real-world systems? Is there additional advice you'd add to that paper based on what you've seen since its publication and with new tools being introduced?
Your article about selecting the right capabilities has a lot of great advice. It would be fun to walk through a hypothetical company case and talk about how to apply that advice in a real-world setting.
GCP has had a lot of new offerings lately, including Vertex AI. It would be great to talk through what's new and what's coming down the line. Our audience always loves hearing how tool providers like GCP think about the problems customers face and how tools are correspondingly developed.
TRANSCRIPT
0:00 Vishnu That was a really cool conversation, Demetrios I'm glad that we were able to get Donna and Christos on and learn so much from them. 0:07 Demetrios Yeah, for sure. So, for those who don't know, Donna and Christos are working at Google – they're working at Google Cloud – and they are behind some of these papers and blog posts that have been circulating. Most recently, there's like a “best practices of MLOps”. I get titles wrong so much, Vishnu. [chuckles] And I know that's not the title. What is the real title of that? It's like an ebook. What's the real title, man? Help me out here. 0:35 Vishnu It's “A Practitioners Guide to MLOps,” which is a really comprehensive and great overview. If you're an ML engineer, ML platform engineer, software engineer, machine learning – whatever you might be, it is a great hands-on guide to how to assess what capabilities you need, where you might be on the different maturity levels, and how to assess what investments you want to make, and what portions of your tech stack. Really cool. We had some of the authors of that on – Donna and Christos. 1:06 Demetrios Yeah. Obviously, we'll link to that in the show notes if you want to just jump to it and read. But what did you think? How was the conversation? What were some key takeaways for you, Vishnu? 1:16 Vishnu So, we've had a couple people on from Google on this podcast before, but the Google-verse is so big that you can have people on that are technically from the same company and have very different perspectives. We've had on Todd Underwood, who is a Senior Technology executive focused on SRE. We've had on Lack we've had on D. Scully – so many people. But what Donna and Christos focus on in particular, is really solutions engineering and focus on external customers for Google Cloud and building for their needs, and then turning that into capabilities on the GCP platform. It was really interesting to get their lens on how to think about customer needs in ML, how structured their thinking is, and then how they turn that structure thinking both into best practices and knowledge for the community through thought leadership, and also into real products that end up becoming part of the GCP platform. I thought it was cool to get an inside look into that. 2:22 Demetrios That's a great point. That is so cool. So let's just give a quick intro and read off their bios and then we'll jump into the full conversation. Donna Schut is a Solutions Manager at Google Cloud, responsible for designing, building and bringing to market smart analytics and AI solutions globally. She's passionate about pushing the boundaries of our thinking with new technologies and creating solutions that have a positive impact. Previously, before Google, she was a technical account manager overseeing the delivery of large scale ML projects and a part of the AI practice developing tools, processes, and solutions for successful ML adoption. She managed and co-authored Google Cloud's AI Adoption Framework and Practitioners’ Guide, as we just mentioned. What about Christos? You want to give it a go? 3:15 Vishnu Sure. Christos is a machine learning engineer with a focus on the end-to-end ML ecosystem. On a typical day, Christos helps Google customers productionize their ML workloads using Google Cloud products and services, with special attention to scalable and maintainable ML environments. He made his ML debut in 2010, while working at Digital MR, where he led a team of data scientists and developers to build a social media monitoring and analytics tool for the market research sector. Some very cool people. 3:41 Demetrios Amazing. Yeah. And that's true. He hit the nail on the head when he talks about “On a typical day, Christos helps Google customers productionize their ML workloads,” that's pretty obvious from the conversation that we had. He's knee-deep in that and trying to help them so – awesome, man. Last thing I'll say before we actually run the conversation. We are looking for people to help us edit these videos and podcasts. What does that mean? You probably are thinking “I'm not an editor. This can't be me.” We've got editors. That's not the problem. We know how to use the software to edit – the tools that we need. That doesn't matter. We just want to hear “What are the gems of the conversations so we can make highlight reels,” and you're probably thinking to yourself, “Well hire somebody like an editor to do that.” The problem is – video editors and producers of podcasts don't really know much about machine learning. So we've had some trouble finding people that are capable of finding the gems and finding “Oh, wow! That's a great little clip. You should probably make that into its own standalone clip or throw it into the highlight reel.” And that's because it's complicated stuff. The normal editors and producers think that everything is… they're either too bullish or too shy. When they are editing, they'll either cut out stuff that is actually important, or they'll be too shy and they'll just leave everything in. And we want to try and make those highlight reels so people can digest a 10 minute version of these conversations – if you don't want to listen to Vishnu and I chit chat for the first five minutes of the conversation and you just want to get right to the learnings. I mean, some people, I guess, don't want that. So we're trying to serve all these needs. We're thinking about you all. So help us out. If you want to volunteer and help us with this editing – all you’ve got to do is say “from second 45 to second 55, that was a good little part – you should add that,” and then we take care of the rest. It's that easy. We just want to hear from you listeners. Help us source the best parts of the episodes. Alright, that's it. Let's get to the actual conversation. 5:52 Demetrios [intro music] I want to start with why you all created the incredible – it's an ebook, it's a paper, it's… I don't know exactly what you want to call it – but where did the inspiration for the MLOps Best Practices come from? 6:08 Donna Yeah, that's a great question. We're always inspired by our customers. I think the customers are, really, at the heart of everything that we do. Based off of a lot of customer conversations, we found a lot of asks around this, but more around the focus on the processes of MLOps. Prior to that, we had kind of two guides on more of the technical architecture side. We published a broader AI adoption framework as well. As we followed along on conversations, as we were having them, we were finding that this is where customers were asking the most. And so, as we were going through this process, we decided to share this more broadly. 6:56 Vishnu Got it. Thanks again for joining us, Donna. We really appreciate it. I want to start with – your focus is, you’re Solutions Manager at Google Cloud and I'm interested, maybe you can tell us about what your model is for working with customers and how you guys get that feedback in the process of your daily job? 7:20 Donna Yeah, that's a great question. We work with customers, typically, in kind of the “incubation phase,” as we're starting to design and build these solutions. We meet regularly with customers to understand what their requirements are, where they're facing challenges, and then working with them to pilot some of these solutions, having this iterative process of constantly improving it with input from a variety of teams, internally as well. We get a lot of great input from all the cross-functional teams across Alphabet, who are working on this and then work with customers to land it. Once we've seen that repeatedly, then we'll typically also publish something. 8:12 Vishnu Got it. So, Christos, you're an ML engineer and you've worked with customers at Google Cloud. And I must say, in our conversations, we hear very positive reviews about how Google Cloud is enabling MLOps and allowing companies to move a lot faster with the entire stack of services that you guys offer for machine learning. From your standpoint, working with external companies, but also sitting internal to Google Cloud – can you tell us where we're at with MLOps? 8:45 Christos Yeah, of course. We do, as we said, work with a lot of customers and we try to adapt to our customers’ needs. Maybe three years ago – we were talking to customers about, “Hey, what is machine learning and how can we productionize a single machine learning model?” And then those conversations transitioned to perhaps spending the last two years talking about them a lot, right? Because, “Okay, how do you do that at scale? How do you do that in a way that helps the whole organization and not individual teams?” And then only in the last year, we've seen big organizations trying to really feel those MLOps platforms and try to bring this to life. Our role is to help them achieve that. Because you mentioned, “How I see it within Google, but also working with customers,” it’s very important to mention that. We are touching on two different worlds and we learn from internal teams, but also we are very conscious that not everybody's Google and not everybody operates on Google’s scale. And, we don't want to kind of impose that everybody has petabytes of data. Therefore we try to adapt our solutions in different scenarios, depending on different customer needs. Again, the point here is that we do get inspiration from within, but we listened to our customers in order to bring things to the market – things that help them solve the problems they have today. 10:19 Demetrios Can you talk about some of these different things? Like, what does the process look like? You sit there and you hear from people that they need XYZ, and you hear it enough times, and then you go and build it? Or you ask for a PM to build it? What does that look like? 10:35 Christos Yeah, that's a good question. So, we do work with customers and we do understand the needs, the frameworks that they are using, how they're using them, and the scale of data they have. Of course, they are testing and trying our products. And from that we get feedback. That feedback ends up with a product team. Of course, the popular ones get priority. Again, it's not like we’re following feedback and compliantly building a feature – we really need to understand the need for the feature, how it helps all over the customer portfolio. But we also need to know, “Are there any workarounds? Any quick wins? Anything else that they can benefit from using the product GCP (Google Cloud Platform) ecosystem and not just from the vertex AI products?” 11:30 Vishnu I really appreciate the customer centricity that you're sharing. It’s clearly a core value of how both of your teams work and how you both approach your work. I also really appreciate your comment, Christos, about how not everybody is Google. There's a famous blog post about it from a Bradfield CS School and we've all talked about it. 11:52 Demetrios We reference it so much. Yeah. 11:53 Vishnu Yeah. It's great to read all the content that comes out of a company like Google in terms of how ML is applied, but most of us aren't working at that scale and I think it's important that you have highlighted that. What I want to ask about now is your white paper that Demetrios referenced, the “Practitioners Guide to MLOps”. I thought it was really excellent. And it gave a lot of frameworks for how to think about the field. Could you provide a brief overview of the processes and the capabilities, Donna, that are outlined in that white paper? Also, how have you guys seen that advice applied in the real world ecosystem? 12:31 Donna Sure, yeah. I’m glad you found it useful. I think that we always – we try to create content and we hope that it's useful for others, so it's great to get that feedback. In the framework, we outline six integrated and iterative processes. Starting with ML development – experimentation and prototyping. Training operationalization – automating the process of packaging, testing and deploying training pipelines. Then continuous training – repeatedly executing the training pipeline, and that can be, for example, in response to new data or on a schedule. Model deployments –packaging, testing and deploying a model to a serving environment for online experimentation and production serving. By “online experimentation” we mean production testing. Prediction serving – serving the model that's deployed in production for inference. Continuous monitoring – identifying and predicting model performance degradation, data drift outliers, for example. Then at the heart of these processes, there’s data and model management, which is a central function for, essentially, governing ML artifacts. That supports audibility, traceability, compliance, but also, shareability, reusability, and discoverability. And then we outline the capabilities that are necessary for these processes. One of the things that we emphasize as well is that many organizations already have existing investments in infrastructure and security, CI/CD – and those can be leveraged for these ML workflows. Then there are the core MLOps capabilities on top of those – ML metadata and artifact tracking, ML pipelines and so on. Yeah, to your question of how it’s applied, I think, typically what we see is that these are being kind of deployed in stages all at once. So organizations tend to initially kind of focus on ML development, model deployment, prediction serving. I think, as Christos also mentioned earlier, it kind of depends on if you just have a few ML systems, you may not need the continuous training and continuous monitoring. We've worked with customers in two ways. I think one is actually building the platform. So for example, we worked with a Telco where they’ve had a variety of regional teams running similar models. For example, you could say you have a team doing a propensity model for marketing campaigns in the US and in the UK, and they have different local requirements, but at the same time, that work can be leveraged. So creating these templates saves time, but at the same time, they were able to make the adjustments that they needed. The other would be on the use case basis, where, for faster time to market, some companies opt to adopt these capabilities by use case. We have, for example, a media company that we worked with that really worked on particular use cases, like recommendations or audience segmentation. I think one of the most common questions that we got as we were doing this is really, “Do you need all of these capabilities and all of these processes?” So that's actually what led us to write that other article around, “How do you select these MLOps capabilities by use case?” 16:16 Demetrios So, there's something interesting there and I love all of the, basically, knowledge sharing that comes out of Google, really – especially in the ML and MLOps field. It seems like all of the classic papers have come out of Google, whether that's the high interest credit card debt – which I can never say that title correctly, but I think everyone who's read it knows exactly what I'm talking about – or the ML test score, and then even the continuous training paper – which again, I'm probably not getting the name of that correctly, but we'll link to all of those in the description. One thing that I wonder about, when you're creating these frameworks, in this continuous training, and hopefully you guys know which paper I'm referencing when I'm saying the “continuous training blog post,” it's really cool to see the different maturity levels. You have maturity level zero and what that looks like – maybe what some architecture choices can be. Then, when you want to go to maturity level one, maturity level two – what those can look like. The thing that I wonder about on this is, because ML is so vast of a practice and when you're looking at these different maturity levels, some of the things that you reference in the different phases may or may not be useful for certain types of ML applications. So how do you look at these ways of doing it, where you say, “Okay. Well, maybe for structured data, this is a really solid architecture design.” Or “This is a really solid way of choosing how I'm going to basically set up my system.” But then if it's a different use case, like you were mentioning, like, “Oh well, maybe now we have a computer vision use case and we need to take different things into account like with looking at unstructured data,” or however it may be. And then on top of that, even going a layer deeper, you can say, “We really value low latency.” Or “We really value the accuracy,” or whatever it is that you're valuing there. How did those architectures come into play? And then, I think the real question here for you is – how do you synthesize all of that data, and then try and create something that you can package up and give to people so it can help them along on their journeys? Maybe Christos, I'll throw this one over to you. And, Donna, if you have anything that you want to add after he chimes in, then feel free to. 18:57 Christos Yeah, that's an excellent question. It is a challenge to just create something that serves everybody, right? And that's why we try to generalize a bit and provide some guidelines when we create either an architecture, or a guide on selecting the right capabilities, for example. I will talk a bit more about the blog posts around selecting the right capabilities because it's very related to what you said. That blog post, by the way, was co-authored with Donna and Lara Suzuki – so big shout out to them. Essentially, it explains how the journey should look like for organizations when they have use cases in mind. Creating an MLOps platform is a different beast than creating a few ML capabilities. It's easy to say “Okay, I will set up a feature store and use it.” It's easy to say “I will have a training service.” But once you start creating a platform means that, “Okay, that platform should use a lot of capabilities, (maybe not all of them, but a lot of capabilities) but you should also take into consideration access to the platform, access to data, security. What can data scientists do on the platform? And what can ML engineers do on this platform? And how do you escalate assets from the development stage?” So, it's a beast. And it takes a lot of time to build, let's say, the best possible ML platform. And we don't advise people to try and land there, we say, “Okay. Take your use case, or a few use cases, and think of what you need to solve those use cases.” Perhaps an organization might be dealing with, let's say, 70% of the time with structured data, and therefore, now we can narrow down the priority – to solve for this structured data. What we do from there is we say, “Okay. So, what capabilities do we need to solve for this structured data? Or, if it’s critical workloads that we're dealing with, what capabilities do we need for that?” The blog post explains how you can pick those capabilities based on the use case, and build them as part of your MLOps platform. So you prioritize based on that. And in the future, when you will have a new use case – maybe now I'm dealing with images, and I need different capabilities – then what you do is, you go when you build those additional capabilities that you might need. And slowly, you start from a kind of “basic” MLOps platform that starts becoming more transformational because you start building good things and better things and more capabilities are building up. Yes, maybe some use cases don't really need a feature store. But once you’ve built it, you might as well use it – if it's a good idea. So things like, “Okay, I don't really need continuous training, but now I have it. It's a click of a button. I can use it. Why not use it for a use case that might not need it?” or “Why not use continuous integration delivery, even if I don't change the base of my code, which means I don't get a big advantage.” But again, if you reach the stage where you build that capability, you can as well use it for all of your use cases. Again, back to your question, I want to highlight that it's this incremental process. Like, don't try to build the best – just try to build for your use case and have your use case as, basically, a guide on what to do next. 22:38 Vishnu In our community, we have a lot of avid readers of content like yours. And what traditionally happens is someone – honestly someone like me – one machine learning engineer sitting in a company or startup says, “Hey, maybe there's ways that we can be doing things a little bit better.” And they read a Google Cloud blog post or two and they say, “Hey, I'm at MLOps maturity level zero, (or one). These are my use cases. And these are the capabilities I need.” Then they come into the community and say, “This is what I'm thinking, how are you guys thinking about it?” And we end up having a lot of great discussions from that. That is how we have learned that your content and your thought leadership has been really invaluable to the overall state of the art. I want to flip that sort of question back to you and say, “How do you see your customers read and learn about their level of maturity, let's say, or what level of capabilities they may have or need? And what does the professional mix look like? Are these engineers? Are these project managers, product managers, or more senior technical executives?” Donna, can you give us a little bit of context about this discovery process? 23:53 Donna Sure, yeah. Maybe, first of all, in terms of who's the audience – I think that really depends on the type of content. We created the AI adoption framework, which is more for technical leaders to think about “What's needed to build an AI capability?” That goes across also to “What kind of people do you need to hire?” The sponsorship – there's a lot outside of it. The Practitioners Guide was more oriented, [laughs] as the title indicates, towards practitioners and architects and engineers. And I would say that the article around selecting the right capabilities for your use case is also more oriented at the practitioners. But we see interaction from kind of across the board. I think in terms of the way that we interact with customers, it would be really with a variety of roles. Having a cross-functional team is really important in a lot of these endeavors and that's actually where I think we see one of the main challenges as well, in terms of “What kind of skill set should be hired? What does that team composition look like?” And so, I think that's the first part of your question – around who we are interacting with. Does that answer your question? Or did you have more specific questions around the process? 25:21 Vishnu I think that does answer the question. It seems like cross functionality is really important. I'm curious what level of disciplines and seniority mix you tend to see. Is it usually more senior people that are coming and starting these conversations? Or is it more of a bottom up movement? 25:40 Donna I think it really depends on the organization. Yeah. 25:44 Vishnu And that makes sense. That makes sense. That makes sense. Yeah, I think in our experience, we tend to see a lot of the bottom up discussion happening. You know, someone who wants to be an evangelist within their company. We don't see as much of, maybe a senior executive saying, “Hey, this is the broad enterprise little strategy that I want to adopt.” I think Demetrius has a question, so I'm gonna kick it to him. 26:12 Demetrios So I want to… [chuckles] Yeah, I want to keep going back to these blogs and papers. If you want to move on, and you're like, “I'm sick of talking about this!” Tell me. But there's something super interesting in my mind, knowing about the papers and the frameworks. Since it came out a few months ago, right? Well, the blog came out – I don't know if it was a year ago, but it feels like it may have been a year ago, correct me if I'm wrong, Donna. A little less than a year ago, probably? Because I feel like I was talking about it with David on here. We did like a whole series, breaking it down on this podcast when it came out, which I feel like was last March, or maybe February. I can't remember. Anyway, the question that I have is really around – and this might be totally ridiculous. I'm just gonna preface the question with that. Is there a next level up – since you wrote that paper or the blog – is there like a level three? Now that you would say, “Okay, since I've seen more and I'm starting to see that as an industry in general, we're maturing. Now, I would say that there are probably these other things that I would have added.” 27:31 Donna Yeah. It's a great question. I think to your point earlier, in terms of where we're at with MLOps now, I think that initially there was this first wave of companies that built their own in-house capabilities. Then, now, we're actually because of new tooling that's become available, it's making it much more accessible and it saves a lot of other companies time to do the same. So what we're seeing is that there's a lot more companies starting to adopt them a lot. And then, at the same time, we also see that the first wave starting to maybe modernize their platform, adopt some of that tooling. I think lately, there was an article by SP, which was a great example – I can send that to you as well – where they start to adopt some of that tooling, and maybe shed some of the technical depth and they moved to more of a self-service model, because it was more familiar to their users with great results. I think that's kind of how we're seeing it evolve and it's still very much a dynamic and evolving space. So, yeah, I would say that that's kind of what we're seeing in the landscape at the moment. 28:50 Demetrios Yeah. And I love that you mentioned that. I think [chuckles] it's really funny, because I was thinking, “Oh, well, maybe there's some kind of new store that Donna's gonna tell us about, that we need to put in our architecture diagrams as the ‘new hot thing’.” But it is true. I think, actually, the person who wrote that – Kyle, from Etsy – he was on here. And he was talking about that. It parallels what we were talking about last week with Jesse about how you see that pattern, where there are those people that were starting ML a few years too early? Well, not too early, but just a few years ago, before there were all these offerings out there and they had to build it themselves. And then, they're reaching a point where they're saying, “Wow, maybe this is easier to just go and buy something,” because the offerings are now reaching the maturity level. So yeah – that's really cool to see. Vishnu, I know you have one. Hit it. 29:50 Vishnu Okay. So Christos, I want to talk to you about – maybe it's real, maybe it's hypothetical – but a sort of case study, or a company case study that you've worked on, where you had to talk to the customer, understand their needs and apply some of the ideas that are in the Practitioners Guide to MLOps and any of your other blog posts or papers. Can you tell us about maybe one example that stands out either for its success or for its failures? 30:21 Christos Yeah, of course. I mean, when we work with customers for building MLOps platforms, when we do use the Practitioner's Guide to MLOps as kind of the starting point, because that helps understand what needs to be done in the landscape. I think that on the back of that, selecting the right capabilities is kind of a super important piece, because it helps you scope the piece of work, as we said earlier, on what you need to do first. And I can give an example – it can be a hypothetical, but it helps you understand how you go about picking the right capabilities. So let's say that you have a use case where you want to analyze text from a call center (maybe it's transcripts) in order to understand how customers complain, perhaps, but also how you can improve your customer service. Say we take that as a use case. We need to kind of start going through each characteristic for that use case that applies as described in our blog post. Let's say, first of all, is that use case mission critical? Analyzing call center data for internal reports, right? Let's say the manager of a call center wants to see a report. Is that mission critical? Well, I would say it's not, because the model is used internally within the organization. It doesn't expose anything to our customers. How we classify ‘mission critical’ is basically “Would it have any financial or reputational impact to my business if there’s something wrong with this model?” I don't think that will be the case because, again, it's a report that the manager will see within. If they see the report that is very odd, they will say, “Hey, there's something wrong with this report.” But it's not a big deal. Right? So the fact that it's not mission critical, it means that “Well, okay – I don't need to worry about tracking all the metadata for this model and do artifact tracking.” And that might sound weird. But again, it's a guide. 32:41 Christos We don't say, “Hey, it's actually useless to have metadata and artifact tracking. What we say is, “Well, maybe it's not your number one priority, so just push it back and we can revisit that later.” Then we say, “Okay, does this use case need to have reusable and collaborative elements?” In other words, “Do other pieces in my organization – other parts of the organization – want to use this model?” And then maybe the answer is ‘yes.’ Let's say it’s a language model. It does sentiment analysis to understand complaints with customers. So it can be a bit generic. And therefore, it's nice if someone else and a different department that also has a call center capability can take this model and then utilize it. So if I want it to be sharable and reusable, I need capabilities like model registry so that I can log my models, I can tag the models with parameters, I can track them with a description – what the model does, how it can be used, and the performance of the model. And therefore, when my colleagues in another department want to borrow that model – download the model and use it – they have all the information needed. Of course, now you say “Okay, now, actually, I also need metadata tracking and artifact tracking.” Right? Because now others depend on this model and the training process of this model, it's good to provide them with all this information so they can know exactly how I and my department train this model. And then you move on into the training. “Do you need to retrain this model frequently or only when it degrades? Well, it’s a language model. So maybe I don't get any benefit if I do training daily or weekly – I will only need to retrain it if the language changes – so that's kind of in a 100 years, perhaps? Or I might need to retrain it if there are new products introduced in my company, and therefore my call center will be discussing these new products. So I don't need to retrain and therefore, the most important thing here is – I do need my training service because I still need to train it at least once or, every time I see the model degrading. But that's kind of the key element – the model degrading. If I don't retrain my model frequently, I need to make sure that it doesn't erode in production – that it doesn't become so stale and becomes, basically, inaccurate. So that model monitoring element is very important for ad hoc retraining of models. 35:15 Christos Then, we think of implementation of, basically, code changes to the code of the model – we want to change the architecture of the code of our pipeline. Do I want to keep doing that frequently? Again, the answer is, “No, perhaps not.” Because if you have a model – a language model that is accurate enough – it doesn't matter to get another 2-3% of accuracy. However, if that was a model that was client-facing, and that was my competitive advantage – maybe I just tune this model. Maybe that’s kind of that's my day-to-day job is tuning this model and squeezing any percentage of accuracy. That means a lot of changes in my code. And if I do a lot of changes in the code of the model – in the algorithm in my pipeline – it means that I need continuous integration and delivery. So I need to have this prove that things are getting shipped into production. In this case, I don't need CI/CD. Again, there are lots in the MLOps community, so people will be like, “What? We don't need CI/CD?” It is nice to have, right? So, add it when your use case needs it, but it's not a priority. Still a nice thing to have. I mean, I love the concept and I love to use it whenever I can, but if you want to prioritize, just push it aside for the time being. And then you see, “Okay, the batch is online serving. It’s batch serving, and therefore that means that we need to have a model serving capability for batch loads. But I don't need A/B testing. I don't need online experimentation, because it's only the API that I need to keep an eye on.” So this is kind of a very quick scenario of how you can use these capabilities in that context. And I think the MLOps Practitioners Guide is that in great detail. Of course, what I like about that is that it focuses on the processes and doesn't really describe specific products. That's kind of a great educational piece, but then paired with selecting the right capabilities, I think that basically brings down to Earth and say, “Okay, this is great. This is a really good platform that you can build. And when you get there, here's how you can prioritize.” 37:36 Demetrios Excellent. Yeah, it's almost like, “Go through this and use your common sense, (which is the least common of all the senses, as they say) and really try to look at what you need the most and what you don't need. Then you can be a bit more patient in trying to implement or just scrap it all together,” right? So, there is one thing that I love to ask people. Of course, for you all, this can be very hypothetical, we could say – just to cover yourselves. But I would love to hear about war stories. Maybe it can be someone else's war story, or even better, it can be yours and what you learned from it, what you were able to take away from it. It doesn't have to be right now, when you're working at Google – it can be in your last job, or the job before that, or back when you were in college, I don't care. I just think that people have written to us (actually, I just got one last week) where someone wrote to me and said, “The favorite part about the episodes is when we have war stories.” So maybe, Donna, do you have a war story that you could tell us? And what you learned from it, what takeaway do you have? 38:50 Donna Sure. Well, I don't know about war stories, but maybe I can talk through some of the challenges or pain points that we see going through engagements with customers. I think there's a variety of challenges across different areas. For example, one of the first ones that we typically see is that probably the most successful undertakings are typically when there is a pull from the business and also a push from a platform team. I think that getting that buy-in is probably one of the main pain points that we typically see working with customers. Another one is, I guess from a technical standpoint, interoperability. I've worked with customers where they have a variety of different tooling, or maybe they're using different vendors, and there's been some kind of technical challenges from that perspective. And then another one is – we work with a lot of customers that may, for example, start embarking their journey to Google Cloud and it also comes with a completely different mindset. So I think where, for example, the way that you would manage costs is very different – moving from what was maybe a central team that owns that budget to actually being able to spin up and down resources really easily. I would say that maybe another “watch point,” as you go along this journey is to think through that, in this culture of everyone being an owner – what are the mechanisms that you can put in place? So, for example, having labels, making it transparent, this whole culture of spin offs, and there are people who are probably better specialized in spinoffs that can talk about that. But I think that that is definitely something that applies here as well. We also wrote a Best Practices Guide, specifically, because we have seen this go wrong and that's not an experience that we would want. So I think those are maybe a few points, but I'll let Christos talk about this. He's worked with several customers as well. So I'll give him the helm if he wants to add anything. 41:08 Demetrios Yeah, I want to hear the juicy stuff, Christos, about blowing hundreds of thousands on a model or something, where they left on – or whatever. [chuckles] But if you don't have it, it's alright too. 41:21 Christos Yeah, I mean, you often get scenarios where things are overlooked and products might be misused in terms of maybe not the right governance is in place. But, of course, we do have ways of controlling that. It’s just sometimes people get too excited to jump on GCP and use things without thinking of, “Okay, what's the impact of that?” Just to kind of expand a bit more on what Donna said, I think big organizations – global organizations – it's a bit of a greater challenge when we think of MLOps because – small teams and small organizations, they're very agile. It's very easy to agree, “Okay, this is how we’re going to build MLOps. This is how we’ll build the process of productionizing – building code, testing the code, productionizing everything.” When it comes to big organizations, since they operate on a global scale, the challenge is building the right tools by listening to each local entity – for different teams within the organization – building something that then can be shared and used in a standardized way across the whole organization. And then convincing them to use a specific pattern, or architecture, or platform – basically, it's a hard thing to do. Because you cannot just enforce it in that scenario, you just need to deliver the right things so that people themselves can say, “Actually, it's much easier to build my models this way, or my pipelines this way.” We're getting there. But I think that's a bit of a challenge still. 43:17 Demetrios Excellent. Well, thank you both. Go ahead, Donna. 43:20 Donna No, no. I was just going to say, actually, to add to what Christos said – we actually did an internal study looking at “What qualities do the best ML engineers have?” Then, we have the technical skills, for example, distributed systems like, knowledge and understanding of testing, security, and so on. But also, one of the things that came out were the soft skills – strong communication skills, because you're working at this intersection of so many different teams. And, for example, having a humble approach, because this is a dynamically changing landscape. And also really knowing “What's good enough?” So I think that that kind of points to what Christos was saying. This ability to work with so many different cross-functional teams. 44:08 Demetrios Oh, I love that. Is that out for the public to see? Can we link to it? 44:14 Donna I think it might have… Yeah, let me check. I think there was an article published on that as well. So I'll try to find that for you. 44:21 Demetrios Yeah, that's so cool. Sadly, we’ve got to wrap. But I really appreciate this conversation. I appreciate you sharing these insights. More than anything, I think, I super appreciate all the work you're doing in this field and helping us to understand and helping us to see how you think through problems. I mean, the work that you're putting out is so beneficial for myself – I'll just speak for myself. I know Vishnu likes it too, but I'll let him talk about it on his own. So I thank you for that. I also think that you could write a whole blog post or book about managing dependencies between different tools, as you mentioned, that was one huge takeaway. And I would love to see that. That's a question we get quite a bit in the community also. But that's it. That's all we've got for today. Thank you again for coming on here, Christos and Donna. This was incredible. 45:18 Donna Yeah, thank you as well for having us. It's great to see the fantastic work that you're doing as a community. We enjoy seeing that, too. I know there's actually a lot of Googlers who are also listening to your podcast. So keep up the great work. 45:32 Demetrios Oh? Uh-oh 45:33 Vishnu See, that's news to us. 45:35 Demetrios Yeah, I better stop talking [expletive] about Google. 45:37 Christos We are watching.
45:41 Demetrios Oh, no. [laughs] 45:43 Vishnu Just a final comment for me. The thought leadership that you guys put out, I have applied it in my company. I’ve taken – read some of these papers and said, “Hey! We're at level zero. We need to get to level one.” I just do want to second what Demetrius said in terms of that. It is usually useful to the community. Thank you for sharing and thank you for coming on. 46:03 Donna That's great to hear, thanks. 46:04 Demetrios Are your teams hiring? I mean, Google's always hiring, right? But are you all looking for people? 46:10 Donna I do think there are within solutions engineering, there are some job openings at the moment. 46:16 Demetrios Cool. Cool. So in case anybody out there listening wants to go and do some cool stuff with these people. You know where to go and find it. That's all we've got for today. See you all later. 46:29 Christos Thank you.