Re-Platforming Your Tech Stack
speakers
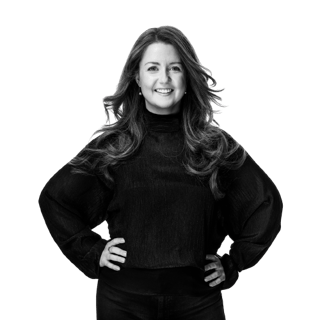
As an Irish woman who relocated to London after completing her university studies in Dublin, Michelle spent the past 12 years carving out a career in the data and tech industry. With a keen eye for detail and a passion for innovation, She has consistently leveraged my expertise to drive growth and deliver results for the companies she worked for.
As a dynamic and driven professional, Michelle is always looking for new challenges and opportunities to learn and grow, and she's excited to see what the future holds in this exciting and ever-evolving industry.
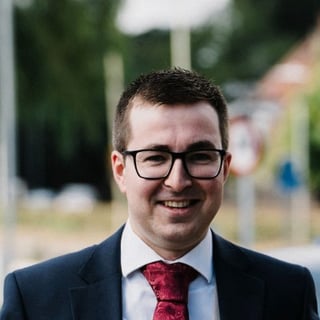
Andrew graduated from the University of Birmingham with a first-class honours degree in Mathematics and Music with a Year in Computer Science and joined Lloyds Banking Group on their Retail graduate scheme in 2015.
Since 2021 Andrew has worked in the world of data, firstly in shaping the Retail data strategy and most recently as a Data Science Delivery Lead, growing and managing a team of Data Scientists and Machine Learning Engineers. He has built a high-performing team responsible for building and maintaining ML models in production for the Consumer Lending division of the bank.
Andrew is motivated by the role that data science and ML can play in transforming the business and its processes, and is focused on balancing the power of ML with the need for simplicity and explainability that enables business users to engage with the opportunities that exist in this space and the demands of a highly regulated environment.
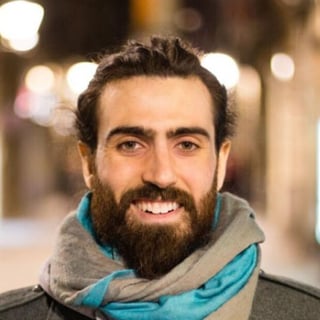
At the moment Demetrios is immersing himself in Machine Learning by interviewing experts from around the world in the weekly MLOps.community meetups. Demetrios is constantly learning and engaging in new activities to get uncomfortable and learn from his mistakes. He tries to bring creativity into every aspect of his life, whether that be analyzing the best paths forward, overcoming obstacles, or building lego houses with his daughter.
SUMMARY
Lloyds Banking Group is on a mission to embrace the power of cloud and unlock the opportunities that it provides. Andrew, Michelle, and their MLOps team have been on a journey over the last 12 months to take their portfolio of circa 10 Machine Learning models in production and migrate them from an on-prem solution to a cloud-based environment. During the podcast, Michelle and Andrew share their reflections as well as some dos (and don’ts!) of managing the migration of an established portfolio.
TRANSCRIPT
Michelle Marie Conway [00:00:00]: So, hi guys, I'm Michelle Conway. I'm a lead data scientist for Lloyd's Banking Group. I work in the consumer lending data science team and my coffee choice of the morning is always the flat white.
Andrew Baker [00:00:11]: Hey there, I'm Andrew. I'm a data science delivery lead in the consumer lending data science team at Lloyd's Banking Group. For my coffee of choice, it's always a cappuccino. Whole milk and chocolate sprinkles on the top are a must.
Demetrios [00:00:26]: Remember that time Michelle came on here and talked to us about how Lloyds bank was moving from on Prem to the cloud and it was going to cost them something to the tune of $5 billion? Well, they've made some progress and this conversation was all about the sticky situations they got into, some lessons learned and how that shift in migration is going. She brought a friend along, which is also always fun. Let's get right into it. And as always, if you enjoy this episode, you probably can think about how you heard about this podcast and I'm guessing it was because somebody told you be that somebody for a friend and tell them about it. Let's get into it. Michelle, your veteran on the podcast. Andrew, this is your first time. It's great to have you here.
Demetrios [00:01:33]: I was reading through some of the fun facts about you all and Andrew, you're a classical guitarist. I am a fellow guitarist myself.
Andrew Baker [00:01:42]: Sounds pretty cool. Classical, electric.
Demetrios [00:01:45]: Yeah, I just make noise. Really do much of playing music. Yeah, I just bang around on it.
Andrew Baker [00:01:52]: Yeah, no, it's. To be honest, I don't get to play as much as, as much as I would like to anymore. It was, it was something that I've been doing since the age of 8 years old. Did it, did it university as well alongside, alongside some maths. So I'm not, you know, I'm not a complete, you know, kind of, you know, kind of artist musician. It's. There is that. There is some technical stem, stem background there.
Demetrios [00:02:14]: Real life took a hold.
Andrew Baker [00:02:15]: Absolutely. Yeah.
Demetrios [00:02:16]: Yeah.
Andrew Baker [00:02:16]: Guitar playing doesn't pay the bills, unfortunately. So here I am.
Michelle Marie Conway [00:02:20]: You did just out the guitar stills last week, Andrew. We had our Christmas party in Chester for the department and there was a pink guitar in the hotel and out it came and it was played.
Andrew Baker [00:02:30]: That's like riding a bike though, isn't it? It's one of those things, once you, once you've got it, never lose it. So.
Demetrios [00:02:36]: Yeah, yeah. And it makes sense you, you enjoy math because music is so much math.
Andrew Baker [00:02:41]: Yes. They are intrinsically linked, although there's not that many Places that actually kind of offer joint courses. So I was, I was pretty lucky to be able to, to study both of those things and enjoy it as long as I could. And then like you say, reality set in and you gotta find a job. And as I say, I found myself in the, in, in the corporate world.
Demetrios [00:03:00]: Yeah. Happened to me too. So I feel for you. So, Michelle, last time you were on here, you said something in passing that was something along the lines of, let me see if I can remember it correctly. It was like, yeah, we're moving to the cloud. We've budgeted like five years and $3 billion for it. And I was like, oh, three with a B. And you said, yeah, yeah, B.
Demetrios [00:03:24]: So fact check me on that. Is. Is that right? Five years and $3 billion later?
Michelle Marie Conway [00:03:28]: Yeah, that is right. That is out in a press release. We did that. I think we launched it two or three years ago, but it was definitely 5 billion into cloud services to help us move. I think the main strategic one was Google Cloud. So we've been on a journey with that since we last spoke.
Demetrios [00:03:47]: Wow. So the both of you, what do you do at the company? For the listeners who missed maybe our first podcast, so they can orient themselves, certainly.
Michelle Marie Conway [00:03:59]: So we're both senior managers on the same ML ops team. I operate as a technical lead on the team, so lead data science scientists and we have a portfolio of around 10 live production models that do various different things for the business. So we've been on a journey helping to move that onto the cloud platform and away from our on prem tech stack. And we've had a lot of learnings experiences and we're happy to say we successfully moved them.
Demetrios [00:04:26]: Yeah.
Andrew Baker [00:04:26]: And I think it's fair to say Michelle, I mean Michelle is definitely the more technical out the two of us. My background's primarily in business, so I'm kind of the sort of manager of part of the team. So I've got a team of, I think it's about 10 now, data scientists and machine learning engineers. So we've kind of built that up from humble beginnings and yeah, into the team that we've got today who have, as Michelle says, been on the journey with us to sort of migrate everything.
Demetrios [00:04:52]: Across to the cloud and working primarily on the platform. Correct. Or is it also use cases and all that.
Andrew Baker [00:05:03]: So that's, that's primarily what we do. So I mean historically our team was kind of set up to take on models that had been built elsewhere, so by central, central team centers of excellence. And our skill set is primarily in maintaining live machine learning models in production. So there are, I would say there are very few teams, Michelle, keep me honest here, but very few teams in the organization that I think have got the breadth of experience when it comes to the MLOPS side of things, as we do. Over the last 12 months or so, we've been sort of pushing further back in the development journey, if you like. So a lot more focus now on the end to end. So from inception of an idea right through to developing that, getting your business stakeholders and your risk stakeholders comfortable with things and as I say, doing what we do best, which is deploying things into, into production. And then of course, as everybody knows, you know, the journey doesn't stop there.
Andrew Baker [00:05:58]: You've got to work with the business to continue to improve things. And actually, you know, I look at it and say, project's never truly finished. There are always things that you can be doing. So. Yeah, there you go.
Michelle Marie Conway [00:06:09]: Well explained.
Demetrios [00:06:11]: I was expecting something else to come there from you, Michelle, but I will keep it rocking and rolling. So I think I understand you right now were like the tail end of the machine learning productionizing cycle and you're starting to move upstream and more and more upstream you. And before what was happening was you had modelers that would create models and then they would say, all right, this looks good, and they'd send it to you in an email. It's like they'd send you an Excel sheet or what.
Andrew Baker [00:06:45]: It's not quite, not quite that bad. But there was definitely, definitely a sense of, you know, we've got to find this model a home now we've built it. And people sit there and say, oh geez, we've actually got to run this thing now. What are we going to do? And they said, well, we're not really equipped to do this. So that was, that was kind of where our team came from, if you like. So it started very small, from humble beginnings, I think there was a senior manager, a data scientist and one laptop and kind of off you go and you, you know, over time we would take more and more of these models on. We've had to, had to establish some pretty robust handover processes. I think we, it's fair to say, Michelle, you'll know this.
Andrew Baker [00:07:26]: We, we kind of learned, as learned as we've gone along. Michelle found herself on, on the other side, so on the kind of the handover side to begin with. So she's had the experience of, I'm going to say, tossing things over the fence. That's probably a little bit unfair, but you get the right, we just sort of get rid of this, off you go. And we'll sit there and say, hang on, what about, what about the monitoring? What about this? What about, what about that? What about the risk angles to some of this? So I think what I would say is our team probably thinks very differently about machine learning models and projects and almost we will come at things thinking about the longevity of them. There is always a danger, I think that once you've built something, it's onto the next thing and you can leave things. And if they get left, as we know, they'll start to drift, whether in terms of performance, in terms of stakeholder engagement and interest. So actually the role that we play at the back end of that cycle is super important in terms of continuing to realize the value that can be sort of gleaned from these models and sort of make the upfront costs and investment all the more worthwhile.
Michelle Marie Conway [00:08:31]: No, 100% because like, as well as Andrew said, I've seen it from when you go from the proof of concept to the MVP to the build to the deploy and like live in production, there is definitely something about being at the other end when you're in the production end because like you see the engineering standards, you see what's good and what's not and our portfolio, I have to say like the engineering on it is pretty epic. Like even after replatforming other areas of the bank, we're like, wait a minute, we want to stay close to your team because you have these epic code basins that are locked down and locked away their production. And it's. So you do see some really good stuff when you are on the NL op side of things.
Demetrios [00:09:13]: What are some questions that you feel like your team asks because they are at the tail end now when they are engaging in different ML projects.
Andrew Baker [00:09:24]: So, so I've got a, I've got a great example of this. So over the course of this year we have worked with a third party consultancy firm on a new project and it was through that build process that they'd got their solution design that they were bringing in. And we knew that the end, the end game was us taking ownership of that, of that model. So we said, right, we need to be involved right from the get go in the build, in the design choices that are being made. And I remember this because it sticks in my head. The consultants had said, right, we want to use this particular library, this particular package. And we sat there and said, okay, we've never heard of this, let's go and Have a look and see what it is. Turned out it was something that a PhD student had built for a thesis and hadn't been touched for about 18 months or two years.
Andrew Baker [00:10:10]: And I sat there and I said, absolutely not. Because, you know, you get into a position where the thing suddenly becomes unsupported. It, you know, you get, I don't know, I'll pick on Python because that's kind of the primary programming language that our team uses. I don't know, you get a Python upgrade that comes along and all of a sudden this package is no longer compatible. What's the next nearest alternative? Well, who knows? And actually, if that's a package that is fundamental to your modeling methodology, well, now you've got to go back to the drawing board. So, like, actually we, I'd consider that a small win for our team that we managed to get them to change their mind and actually adopt something a little bit different. It didn't quite have all the bells and whistles that they were looking for. But again, what we would look at is we say we value the longevity and the robustness of a solution over the, you know, the perfection or the, you know, that, that pursuit of accuracy, which I think, you know, intrinsically, every data scientist wants a perfect model.
Andrew Baker [00:11:08]: And my experience is that in the real world, when people are trying to use these things, I would say robustness, explainability, simplicity, the ability of users to understand it trumps accuracy time and time again. Now, when I say that, I don't, I don't mean let's let the thing be inaccurate and put out terrible performance, because at some point the business users will come knocking and say, well, that doesn't work for me. But there is definitely a trade off there that I think we appreciate more than perhaps some of our colleagues in other areas of the bank.
Demetrios [00:11:41]: You're looking for a Toyota, not a Porsche.
Andrew Baker [00:11:45]: I mean, I don't know about Toyota. I go for Ford. I find my, my Ford Focus pretty reliable. So if that's what we're going for, I'd say reliability over.
Demetrios [00:11:56]: Yeah, yeah, yeah, exactly.
Andrew Baker [00:11:58]: Porsche or your Ferrari.
Demetrios [00:11:59]: Exactly. I'm good. Exactly. You don't need that Ferrari, the, the Toyota or the Ford that can go couple hundred thousand miles and you don't need to replace the engine. You see, that there is the longevity in it. You recognize, at least in all of the known risks, you recognize you're covering your backside, right. And who knows, maybe something comes up that you weren't really aware of, but you do everything you can to make sure that you don't have those surprises.
Andrew Baker [00:12:35]: Yeah, I, I think that's, I think that's totally fair. I mean, I, I don't think you can ever be immune from the unexpected. There's, there's always going to be. I mean, things evolve at such a pace, right, that actually the most important thing in my mind is having a team who is resilient, who is adaptable, who can react quickly to some of these things and effectively problem solve. It's less about the situation you find yourself in and it's more about, okay, how do I get myself out of this into a place where we can be happy with what's happening, our risk teams are happy with what we're doing and ultimately the business users who are, you know, the people that we're serving when we're, when we're maintaining these models, they're the people that have got to be happy with this. So, you know, where we find ourselves. Okay, let's, let's just, let's just deal with it and see where we can get to.
Demetrios [00:13:29]: It makes a lot of sense that you are moving upstream because I'm sure there's hard lessons learned that you've had over the years of being so close to production that when you do go upstream, you can nip something at the bud so much faster. And just small things, I imagine with security and compliance that you have to think about when you're trying to take something to production, it's second nature to you. And then the teams that are in that AI center of Excellence, they're living on Cloud nine, like, oh yeah, this will get through, or our productionized team will figure out how to get it through. So the I, I can see how it can be more efficient in that regard. But let's talk a little bit now about what the situation looked like before as far as the infrastructure and everything. And then how did the idea of going to cloud even spur? Like, it's a big project and I'm sure it was painful in many different ways, so why put yourself through all that pain? Paint the picture for me maybe.
Michelle Marie Conway [00:14:42]: Yeah, we can definitely give examples. So, like, we had loads of models that are on our, on prem textract bells and whistles. And we went through the journey of moving them to the cloud and we noticed like one of our pipelines used to take five hours to run. When we moved everything on cloud as is, we didn't change anything in terms of the way stuff was laid out. It was like a lift and shift as much as possible for these models. So the pipeline went from five hours to 20 minutes, so we unlocked a lot of capability. Just being on cloud with the same portfolio, yeah, it's crazy, but a massive benefit and that's a massive win. Obviously there was other things, but that was the one that definitely stuck out.
Michelle Marie Conway [00:15:24]: We were all kind of like, oh, okay, this was a good thing we did.
Demetrios [00:15:28]: Do you know why it was so much different?
Michelle Marie Conway [00:15:31]: There was something to do with the on PREM configurations and infrastructure was just so restricted and locked down that you couldn't unconfigure it. And it was so limited that when you went to cloud you could like optimize it with like more nodes and computing power and it was like so much better. And then I think I spoke to an engineering lead recently and he was just like, oh, the signaling and the setup for like on prem was just. It was blocking everything. Like, the only time we could really use our old platform was in the evenings when no one was on it. It unlocked really good capability for us and we did move.
Demetrios [00:16:05]: Yeah. So people would just click train model and then go home and who knows what happens. Hopefully when you show up to work the next day, it's all ready for you.
Michelle Marie Conway [00:16:17]: Yeah, it was a little bit like that. It was a little bit like, fingers crossed. If I run it now in the evening, like, everything would be like, no one would be using it. And I've a few times done that. Like, okay, if I hit it off after 6pm, it's more likely to survive. And loads of people in group have done that. So now it's brilliant. That stuff works during your working hours.
Andrew Baker [00:16:37]: Yeah, I think that's really important actually. The point Michelle was making here, the scalability is a massive piece. Right. So, I mean, as Michelle's kind of alluded to there, there was kind of an infrastructure that is predominantly on prem. And you know, I think certainly over the last few years and more so now with the explosion of gen AI, I think there is a real thirst for organizations to do more with the data that they've got. And certainly in terms of the focus that Lloyds banking groups put on recruitment in the sort of the data and tech space, I think they want to sort of use certainly the vast kind of amounts of data that we've got at our disposal together with the latest thinking and the brightest young minds to sort of think about things that we can do. Now, of course, on PREM infrastructure has its limitations, so I think the scalability that cloud offers in particular is, is vital in being able to just, just unlock the number of different use cases that, that people can dream up. You know, it's, you know, it's, there are so many people, I think they've got great ideas and things that can be done and what you don't want is your infrastructure to be the thing that holds you back.
Demetrios [00:17:48]: And do you think it was just years of using On Prem so that there was this very solidified process and structure around things that you could potentially run into that same five, 10 years of being on the cloud and solidifying things and having more and more restrictions every year, or is it more of an underlying infrastructure that's ideally not going to happen now on cloud because of the way that the design of the system is.
Andrew Baker [00:18:23]: So I think what I would say to that is there is less, in my mind there's less chance of that happening. And I think partly because moving away from On Prem and towards a cloud based infrastructure means that, you know, the organization is learning about how this works and it works in a very different way to the way that we might think about on On Prem. Whether that's, you know, the technical aspects of it or perhaps some of the risk aspects of it. I think everybody, no matter what part of the organization they're in, when you talk about a move to cloud, they're having to upskill and think slightly differently about things. So all of those old press, old processes and established ways of working, I think, are being thrown up in the air and people are ripping up the rule book and saying, right, okay, we've got this great opportunity at our fingertips. How do we need to think differently? Because those old ways of working just don't make sense anymore.
Demetrios [00:19:18]: So, okay, you were having this scenario where you recognized things were getting way too entrenched. You're On Prem, you're probably not the one that made the decision to go into the cloud. Or maybe you do have 3 billion in budget or 5 billion in budget that you can throw around.
Andrew Baker [00:19:38]: I think I could have a much bigger team if that were the case.
Demetrios [00:19:43]: How did the transition happen?
Michelle Marie Conway [00:19:46]: As in when was it decided? Or how was the journey going from On Prem to Cloud?
Demetrios [00:19:50]: I, I want to know how was the, how did it come down to like being sold on this idea of changing from On Prem to cloud? How did the, how did you all get this information? Like, this is very, it's a very big decision, right? And I know like I said, you probably weren't the ones that made that decision, but what was that like? Like, how did, how did you experience it? You were just told, hey, we gotta go to cloud now. And you're like, all right, well let's go about it. And how do you even like, prepare for such a big project? How all of these, these ideas of what did it look like from your side of the fence, of, okay, now we're gonna make this shift.
Michelle Marie Conway [00:20:43]: It was definitely. We had been trying to move to Google Cloud over the last few years and then we got new leadership in the last two to three years where the very clear strategic direction was data is going to drive our organization now. Like, we need the best cloud computing to go with that. So there was very good messaging before all this started. And then I'd say it would happen as a waterfall effect. Like we would have our cloud services department who were basically taking the Google Cloud platform, like going through it with a fine tooth comb to make sure everything was secure and able. And then that would come then into our like, analytics and AI platform. The engineers would look at like, what products they needed to do their specific jobs.
Michelle Marie Conway [00:21:30]: So we worked really closely with those AI engineers because they were like building out the infrastructure that we needed to sit our models on top of. And we kind of found that sometimes they're excellent engineers. But the engineers within our small little ML ops team were just as good, if not better when it came to the technicalities of things because they would see us as the customers and they would build something for us as customers. But what they would build, we would be like, no, send that back. It's not good enough for what we needed to do. And it wasn't out of like egos or anything like that. It was more out of like the technical details we knew and we knew exactly what we needed. So it ended up being, we were more peers building together rather than being a customer that was being served.
Andrew Baker [00:22:18]: And I think just.
Demetrios [00:22:19]: Did you.
Andrew Baker [00:22:20]: Sorry, just to, just to build on what Michelle saying, I think it links really nicely back to what we were talking about earlier. I actually, as a, as an mlops team and as a team that plays at that sort of back end of this lifecycle, our views on what is needed for a machine learning platform is probably different to a view that you would get from a team that is purely there to develop models. Right. I think there are a lot of things that we have to consider from an ops perspective that if, if your job is purely to build something and get it signed off and, and live, there's, there's, there's definitely a lot less that you would need to think about if, if you restrict yourself to that part of the life cycle.
Demetrios [00:23:02]: What did you end up going with? Is it off the shelf, vertex, AI type of thing? Are you able to like grab pointed solutions when you need them?
Michelle Marie Conway [00:23:15]: I would say we definitely use some aspects of Vertex because that's where machine learning sits within Google Cloud. We did heavily use the Python APIs a lot within our code bases and I'd say that's something that we learned about. So when it comes to moving models that are already live, to make changes to them, you have to go through a lot of governance and like work with your risk department. So our goal was to move them without making too many changes because otherwise you have to explain your changes, you have to explain your commits, your pull requests, the reasons for this, the reasons for that. Like our release notes are epic. Like they're not a one pager, they are like absolutely epic when it comes to explaining everything. So you don't want to be like ripping loads of stuff in and out. So we were very clever with how we used our models and changed the infrastructure points that it touched.
Michelle Marie Conway [00:24:06]: And so we used a lot of different ways around that by using Python based APIs rather than just the GUI system of products. And that enabled us to move it and lift it nicer and easier, I'm sure. Andrew, you remember all the lovely releases that we had to do?
Andrew Baker [00:24:26]: Yes, yes, there were many of them. I think just to add to what Michelle said there as well. I mean, like, I think we are probably one of the few organizations that as a, well, as a financial services organization, as Michelle said, we, we kind of use aspects of it. But there is an awful lot, as we've kind of alluded to, that has to go through kind of cloud risk partners and they'll look at actually from a risk perspective, what are we comfortable with as an organization. Now different organizations will have different levels of appetite for some of this stuff, but we do kind of have to consider the fact that we're in a highly regulated environment. So I think some of those considerations, we've got huge teams of people that are looking at that and thinking about what can we use and actually where do we perhaps need to adapt things to remain compliant either with broader regulatory kind of pieces or our own internal risk appetite.
Demetrios [00:25:24]: So everything that you did behind the scenes of the model, you didn't necessarily need to give a big description, Iliad and the Odyssey type of description. You could just do it. And it's only when that you update that model that you need to go and write this compliance report. Is that how it works?
Andrew Baker [00:25:49]: Yeah, there's kind Of, I guess there's two strands. So there are the team that are responsible for building out and implementing the platform as is. So that's not us. As Michelle said, we worked very closely with them. So when they are designing capabilities and making those available to us, that was the process of kind of review and feedback to make sure that it worked for us. And then when it comes to each of the individual models, you know, a lot of the time, fortunately, there were changes that were not unique to specific models. So you're able to make a similar sort of change across. Across your portfolio.
Andrew Baker [00:26:23]: And of course, then you can sort of explain those changes once and everybody gets comfortable, particularly your risk partners, with the idea of what you're doing. Where it gets more fun and more interesting is where you have models that do something a little bit different. And kind of, as I'd said earlier, our portfolio was predominantly built by other teams of people. And I love this analogy of if I asked five different people to write chapters for a book, would I get a book that's coherent? Probably not. So you end up with all of our models being a little bit different and you having to sort of adapt your approach depending on specific patterns that a team may have used. So it's. It all comes down to kind of individual team preferences as to how they've done things. One thing that we really push for, and actually I'm a really big advocate of, is the more consistency you can bring to your portfolio, particularly from an engineering standpoint, the better.
Andrew Baker [00:27:17]: You know, how much easier. Easier is it for somebody new to the team to upscale on ways of working and understand how you go about maintaining code bases, if you can keep components and elements of that consistent. Right. Right across the board. So that's definitely something that we used the replatforming journey as an opportunity to have a think about. I think there are still things that we can do, but it was definitely an opportunity to take that step back and just look at the portfolio in the round.
Demetrios [00:27:47]: Yeah. Did you do any type of. I've heard of model cards being popular, or do you have a standard way of doing it now, since you've gone and you had the whole process and you saw like these five different people or five different teams that wrote five different models? Wow. We might be able to make this a little bit more standardized across the team so that we're not having to do all of this different, like Game of Twister to support these models. Yeah.
Andrew Baker [00:28:25]: So I would say certainly in terms of. In terms of our team pushing earlier in that journey. That's part of the reason for it, right is that actually now that we are established and we've been around for a while and people can see the capability, the ability that we've got to take an idea and turn it into something and sort of maintain it at scale. The more new business we can put through our doors, the more control, creative control we have over designs. And I know Michelle has been involved in sort of trying to design templates and cookie cutter type approaches for certain things and that's something we take quite seriously. I think there will still be circumstances where others have built models and we have to, and we have to take them on and whilst we will try and get in there as early as we can in the process to influence their decision making, I think it's fair to say we don't always catch everything. So there are, there are things where I suppose you just take a materiality based approach, right and say can I, can I live with this? Even if it's for a sort of short period of time until we, until we've got some capacity to then go back and perhaps re engineer this to, into a format that suits us?
Michelle Marie Conway [00:29:34]: No, we definitely do and we do kind of when it comes to like patterns and standards, like every business problem will be different so your data sources will also be different and then how you're building that data science model will, will be different but the infrastructure that it sits on, that's something you can give consistent patterns and like the data sources that it's coming in and out of, like you know, if you're going from like a SQL server database, like maybe mapping to like cloud, SQL might be more optimized than going straight into BigQuery because you have less changes in terms of your schemas and things like that. So, so there is certain patterns that you can align and make similar but obviously machine learning for different business problems will be different.
Demetrios [00:30:17]: And are you. Oh man, there's so many, so many ways that I want to go here but because the standardization in all of the just optimization for these different teams has got to be like a never ending puzzle that you can constantly try to figure out better ways and, and I imagine it's in phases also as you've, you see, huh, this might be better XYZ or done like this or these capabilities could give us a bit more efficiency gains. And I'm just thinking about that like 5 hours to 20 minutes type of pipeline. Were there other areas that you saw that kind of lift? Maybe not that drastic but things that you recognized whether or not it was just for moving to the cloud, or maybe it was because you got the chance to move to the cloud and use some different tool or have a new design pattern, you got to see this works out so much better for us.
Michelle Marie Conway [00:31:29]: There's definitely, I think, two things come to mind. One was the on prem and we try to spin up workspace and stuff like that. Sometimes the connectivity would just drop and it would not be there and it would not be consistent. And then we moved to cloud. I'm not saying we didn't have challenges with connectivities, but the connectivity was much better when it was up and things were smoother and there wasn't lags and like, it was better. And then when it came to like doing patterns, like being able to massively influence the platform engineers with like, hey, you're building this cool thing, but actually this is how we're using it. Can you align it this way and this way? Because when you're building something generic, that's fine, but without. Actually the use case that has been built for you might be missing a few functionalities.
Michelle Marie Conway [00:32:17]: We were able to like tap into that to be like, hey, here's the bits we need to configure to make it better. And I did find our engineers were great at highlighting those bits and pieces and twigging it to be like, hey, change it. And it makes it much more robust for everyone using it, but it comes from the users using the toolkit rather than the engineers of the toolkit.
Demetrios [00:32:39]: Oh, very cool. And do you have some, some different ways that you were able to configure it? Like, was there any, any tricks that you found or anything? Maybe it's that you were using a database in, in a different way, or maybe it was that you just optimized one database towards a certain.
Michelle Marie Conway [00:33:04]: We definitely tried to tweak like, for like, because we were mindful of. If you move on to certain products that are completely different to what you're using now, that's a lot more changes that you need to be more mindful of as well as you need to be mindful, like what libraries are in your ML models to begin with. Like, do you need to strip out those Python libraries in order for it to be compatible with something new? Sometimes we did have to do that because we were like, okay, we're no longer using this product, therefore, like, these files that relate to the config of that product must be removed. But other times it's like, oh, actually if we strip this out, like, if you're removing Alembic or SQL alchemy. Like, that's lots of Python code. You need to find ways of keeping it compatible because it's not that you shouldn't upgrade these things, but do it incrementally. Like, don't do it as one big bang. Because if you do a massive release with loads of changes, you don't know what's gone wrong on those changes.
Michelle Marie Conway [00:34:00]: Whereas if you do it incrementally, at least you'll be able to be like, right, okay, cool, we change just the product type here, change the data source there, or we change new infrastructure thing over here. And if you do that into like three different releases, at least you know where you can pinpoint. Whereas if you do it all in one bundle, it's like, best of luck, buddy. Figuring that out.
Andrew Baker [00:34:19]: Yeah. And I think actually Michelle. So there's two things I was going to say. So Michelle's touched on an important point. I think just more generally when you're maintaining a portfolio of models. Right. Is the little and often approach, I think on reflection is probably a better way to go. There's always a danger, I find that because business users are so focused on value that they can see you don't spend the time on the, oh, I'm going to go from this package version to that package version.
Andrew Baker [00:34:50]: You know, the thing's working, let's just leave it alone. Let's go and spend our time on something that's going to deliver value for the business. Well, the problem with that is you might find something creep up on you where all of a sudden the model that was working yesterday no longer works and I've now got to pull three people off what they were doing to try and find out what the hell happened and spend, I don't know, a week, two weeks trying to then figure out what we, what we do. And of course it's then the knock on impact on, you know, on other packages that may be dependent on the package that you were using. So it can be a real minefield. I wouldn't, I wouldn't say that's something that as a team we've necessarily cracked in in the past. And I'm not sure there is ever a, a one best way of dealing with that. But I do think, you know, for anybody who is aspiring to have a team of sort of data scientists and engineers maintaining a portfolio of models, thinking about your approach to ongoing maintenance is super key.
Demetrios [00:35:47]: Yeah, it's that classic tech debt can come back and it can get you in the end and it's never when you want it, you're never ready for it. It's not like, oh, cool, we just happen to be doing a hackathon and things broke. It's like, when engineers are out, they're out of office, it's summertime, nobody wants to actually work on anything, and boom, what can go wrong will go wrong. And speaking of things going wrong, tell me about what hit the fan when you were making this shift. What are some huge challenges that you had and how did you overcome them?
Michelle Marie Conway [00:36:27]: I think because we're so large as an organization, like, I think we had a total about maybe 16 or 18 live NL models in group to move and the logistics of figuring out who's moving what and with who, because you need the platform engineers who know the infrastructure, and you also need the SMEs that are like, using the models on a daily basis. And you need to form little squads of everyone. And like, we had scrum of scrums across all these little sister teams and trying to organize this as like an ML ops team where we owned 10 of the models and we had like a massive dev team to distribute. And as well, you have to think about all the models are different. Like, some might have 10,000 lines of code, some might have 40,000 lines of code. No model is the same. And so some models might need, like, more months of work on them to move them than others. So trying to, like, do the planning and logistics around that was like, it was massive.
Michelle Marie Conway [00:37:25]: Like, it ended up needing like three senior managers from our team, like me, Andrew and another, like, working on it. I ended up getting pulled into the central engineers teams because they were like, well, we have to move 18 models. Your team has like 50, 60% of those. You're coming in here. So we want someone who can read the code bases with us. And I was kind of like, what the hell am I doing here? And being on calls or in going through the 40,000 lines of code for 10 different models and explaining where the infrastructure is hitting and upskilling the engineers and the ML pipeline of each of the different ones. It was a massive logistical operation. I'd say it went on for like, Andrew, I can't Remember, was it 12 or 18 months?
Andrew Baker [00:38:05]: I don't know. It feels like forever, to be honest. I think also, just as Michelle was talking, I was sort of reflecting on a couple of things. Like, it's kind of implicit in what we've already said, but I guess just to be really clear, we were having to give feedback on some of the tooling and the capability that had been built, or perhaps as we've kind of said, adapted so that it's compliant with our kind of security policies and risk appetite. You only really find out what works and what doesn't sometimes as you're in the process of moving these models. So there were definitely moments where you're trying to prepare the groundwork for the model to move and at the same time saying, right, can you just go and have a look at this capability that we don't think is quite hitting the mark in terms of what we need. So, you know, you might think that you're going to have a, you know, fully established, perfectly working, all bells and whistles, machine learning platform there and. Right.
Andrew Baker [00:39:01]: Okay, now I can just get on with the process of moving the models. In reality, I think it's often you are, you would be very, very lucky, I would say, if you ever found that scenario in an organization anywhere. If anybody has seen that somewhere, I'd love to hear from them. But I think there's definitely something about that process of. I mean, I sort of talk about hitting a moving target as you're kind of moving things. Like Michelle's laughing because she knows exactly what I mean by that. But it's, yeah, you kind of have to roll with the punches a little bit, right. It's an imperfect world.
Andrew Baker [00:39:35]: And actually by starting the process of moving some of these things, you figure out what will work and what won't. What I would say is, I mean, it would be great if anybody were doing this in the future, a guinea pig project that you can sort of throw at your platform to say, what are the pitfalls? What are the things that need to be adapted? What do we need to think about when we get to the rest of the portfolio would be great. Now it's you. You then get to a weird space of do I pick the easiest one to move? Which you could do. But the easiest one isn't necessarily the one with all the different capabilities that might be required by your portfolio. And as Michelle rightly said earlier, no two models are the same. So in some ways I get to a space that says, you probably want to start with the most difficult one first. But yeah, you might, you might find that you sort of turn your developers off in the process by saying, right, let's, let's start.
Andrew Baker [00:40:28]: We don't, we don't really know what we're doing, but let's start with this complicated one here and, and figure it out as we go.
Demetrios [00:40:34]: All of a sudden you stop Getting invited to the work birthday events and you're wondering why nobody's talking.
Andrew Baker [00:40:42]: I've got no work friends anymore and I don't know why.
Michelle Marie Conway [00:40:46]: Yeah, we definitely got invited to the Christmas party last year. Not this year, but no, even like there was stuff we realized on the way, like, obviously Google Cloud are going to be really smart with how they do things and they're going to like, move with the times. And we may have discovered halfway through that the minimum version of Python that they Support is Python 3.10.
Demetrios [00:41:10]: And you weren't on that.
Michelle Marie Conway [00:41:12]: Some things might not have been on that, but they were swiftly moved onto that and we discovered that on our journey. So there was lots of interesting insight to be done.
Demetrios [00:41:25]: And that's the stuff you discover in flight, I imagine, where you're like, why isn't this working? And then it's one of those where it's really fundamental and you go, oh, okay, we gotta spend a few more weeks on this.
Andrew Baker [00:41:37]: Yeah. And as we said earlier, right, it's not just, it's not just the Python package, it's everything else that depends on that Python package. All of a sudden you've opened a massive can of worms. And what sounds like a simple change when you articulate it like that, you know, just move from this Python version to that Python version becomes a. Right now I need to go and check all of these libraries and see what needs to change. And you know, if you, if you move this here. Okay, well, that breaks that thing over there and it's. It can become a bit like whack a mole.
Andrew Baker [00:42:04]: So it's. But that's, that's part of the fun of it, right? Is problem. Problem solving is the name of the game.
Demetrios [00:42:10]: Yeah. 100% feels like dominoes. Have you heard of this? There's a while back I had this guy named Benjamin, who's the CEO of steadybit, come on to the podcast and he is more in the DevOps realm and they do like chaos engineering and their whole thing is we're going to stress test the systems by almost turning things off. And it comes from the idea of chaos monkey, where I think at AWS or at Netflix they had this where they would let a monkey loose among the system and it would just go and turn things off or increase this or decrease that and see what happens. See how resilient the system is. And chaos engineering is the next level of that where it's. You are having a thesis in mind. Like if our Traffic suddenly spikes 10x, are we going to be able to handle it with our current infrastructure and how does that look? Or if we lose signal from this database or that database, do we have enough replicas in place to be able to get that database, get that data back? And in that conversation he talked about how.
Demetrios [00:43:30]: Well, I asked him. Some things that I've heard from DevOps folks are, yeah, I don't really want to know how brittle my system is. I don't like your tool because I know it's brittle. I just don't want to know how bad it is. And it kind of feels a little bit like that where you had to figure out how brittle it was and then you had to go and fix it and you almost didn't realize it, or maybe you did realize it to a certain extent that what you were building your foundations off of may have been false security in a way because you thought the foundation was strong. And then when you go to change it, you're like, actually it's not as strong as we originally had the idea.
Andrew Baker [00:44:21]: I think that's fair. I mean, I'd say it's ignorance is definitely that regard. But like, like you say, I think, I think the, the journey that we've been on certainly opened my eyes to some different challenges, but I think with that is a massive opportunity, right? It's an opportunity to take that step back and look at the infrastructure, as you say, and if there are things that you thought were safe but are not, how do I think about improving them? I mean, it's really interesting actually, you talking about chaos engineering. I sort of think our friends in the risk teams probably wouldn't be massive fans of any of that, to be honest. But there is definitely be something in a, almost a stress testing the system to sit there and say, you know, if this were to happen, what's going to happen to my portfolio now? I think we're probably fortunate in that the vast majority of the models that we look after are not time sensitive or time critical. But there are definitely there will be use cases across the bank. I am sure where that is, that is not the case. So of course, as you would expect in a, in a highly regulated financial services organization, anything that is considered critical in terms of a process has, you know, very strict controls around it and there are regular sort of stress tests.
Andrew Baker [00:45:38]: I think the increasingly as we make more use of data science, machine learning, gen AI and some of those use cases become more entwined with business processes. I think we will fundamentally see, see a shift, as you say, to, to far more focus on stress testing. Of some of these, these tools, platforms, the infrastructure, and, and kind of think about some of these things because it's all too easy to forget about it until it doesn't work.
Demetrios [00:46:10]: Exactly. Well, Michelle, I would love to know in those weeks or months, I don't know how long it was when you were actually. It was, yeah, what, nine 12 months of you being on the other team with the platform team, what are some things that you've learned on how to best interact with these platform teams? And the reason I ask this is because in the ML ops community, the question comes up constantly, how much ML do I need to know if I'm trying to build an ML platform? How? And on the other side, someone that's coming from the data science aspect of it, it's how much platform or how can I better relate with my DevOps team? How can I better help them to understand what's going on? So did you learn Anything in those 12 months of working very closely with the platform team?
Michelle Marie Conway [00:47:09]: I learned loads. I learned I was a terrible spy, I'm a terrible liar in general. So when I devoted 50% of my time to be part of the platform team engineer squad that was moving everything, and then also be a tech lead for our team, it was like I couldn't lie to either side. It'd be stuff like, oh, I can't tell you this, but I'll show you anyway. I did learn, like, obviously my data science is the strongest. My software engineering, not so much. I do think I massively upskilled in software engineering because I was plugged into a core infrastructure library where I'm like, right, okay, I need to massively learn how to upscale here and how to do it. And I'm learning bits and pieces and I'm being thrown into scrum of scrums and really technical meetings where I'm like, I am out of my depth, 100% happy to hold my hands up to that.
Michelle Marie Conway [00:48:03]: But I do know, like, my verbal skills, my people skills definitely helped because people are more likely to help you if you're like, hey, you do that really cool thing over there. Do you want to show me for just 10 minutes and help dig me out with this massive hole I have over here because I have no clue what's going, going on. And like, I was so uncomfortable during that time that I just got used to being uncomfortable. Like, everyone talks about this, oh, imposter syndrome. Like, imposter couldn't exist during that time. It was like, it just wasn't allowed. It was like, we need to put on the big girl boots and get on with it. So I learned loads, but I definitely think I added value to certain blind spots that the platform might have had as well as they teach me so much in engineering that I have a massive value for it and I think it's enabled us massively because I now understand the platform like the back of my hand and we are looking to expand our portfolio into more Gen AI and we're building that on top of our existing platform.
Michelle Marie Conway [00:49:03]: So the fact that I understand it really, really well means I'm now dancing circles around different proof of concepts that are coming out on Genai. Because I'm like, no, no, that won't work. No, that might work. And oh, I like what you're doing here. Can I have that? So it means now we're cherry picking and we have a lovely working group with the right technical leads across the group, which I think people don't like because we're advanced users. But it's not our fault for wanting to learn, grow and develop and having a really good portfolio that we care about.
Andrew Baker [00:49:34]: Michelle's been quite modest there as well. She was, she was so enthused with the platform that she, she also helped our risk partners on their journey to kind of understanding, understanding how the platform works. So when we were trying to describe some of the changes we've made to the models, in fact she made them so helpful that the challenges that I then got back about why we were doing certain things made my life a little bit painful, shall we say, for a few weeks. So thanks for that, Michelle, but no, it was absolutely the right thing to do. Right. I think we're all going on a journey and actually us being able to take what we know and help, whether it's the platform team in terms of understanding our requirements, whether it's the risk teams that are there to keep us safe, helping to equip them with what we know so that they can effectively challenge how we've thought about things and then genuinely be a partner in working through things and keeping us safe. I think that was super important. So, yes, as I say, I made my life hell for a couple of months, so thanks, Michelle, but we got there early.
Michelle Marie Conway [00:50:36]: I was asked just to talk to some of the risk team and I was like, oh, they weren't too sure how to use the platform, so I did hours of pair programming and I made them a little bit too good. Like they're very good now. Like they could, they know how to use that platform and how to pick it apart they're an excellent Rex department. But I did make Andrew's life a little bit difficult when it came to releases, and I totally forgot about them.
Demetrios [00:51:01]: Incredible.