Responsible AI in Action
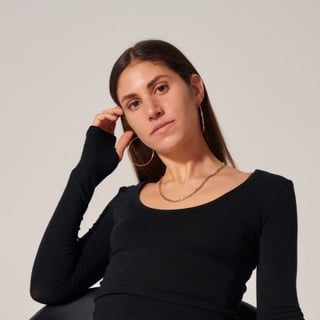
A documentation-loving, data team advocate. Allegra enables technical programs and platforms through a dedication to productivity, transparency, and long-term focus. She is driven by empathy and a never-ending desire to learn.
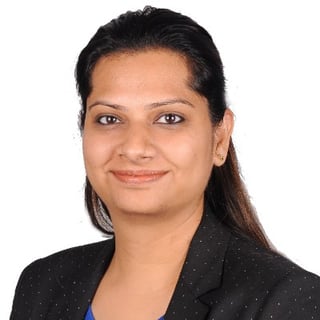
Seasoned data science professional with 10 years of experience in machine learning and data analytics.
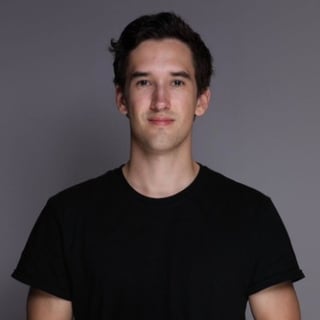
Stefan is a product manager at Mozilla.ai, focused on trustworthy AI, with a background in Data Science consulting across the Government.

I'm João Silveira, a Big Data Engineer at Microsoft with expertise in Spark and Cloud. I excel in designing scalable data processing systems and optimizing pipelines for efficient data management.
With extensive experience in Spark, I've been helping closely my customers in building and optimizing large-scale data processing pipelines. My expertise spans various industries, delivering data-driven solutions that drive business growth and data-driven decision-making.
In addition to Spark, I have a strong background in Cloud administration, particularly with Azure. I excel in configuring scalable computing resources and optimizing data processing workflows in the cloud environment.
For inquiries or collaboration opportunities, please contact me at [email protected]. Let's connect and leverage the power of data for innovative projects.
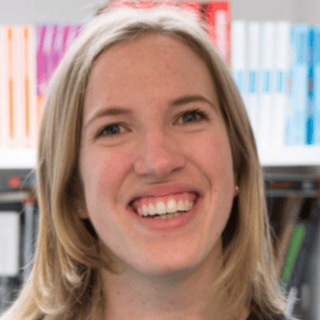
Sophie is a Senior TPM with over 5 years of experience managing machine-learning projects at Onfido.
Catch a panel discussion on the tactical side of responsible AI. They discuss what it takes from the engineering perspective to put theory into practice.
Anjali Agarwal [00:00:01]: Hi everyone, my name is Anjali Agarwal and I'm originally from India. I started my work 13 years back and I started with technology. I worked in technology for initial four years and then I slowly moved towards the data teams. I started working in data analytics, some part of doing a lot of dashboarding and visualization in tableau, etcetera. And then I moved towards machine learning and AI completely. So in data, I've been there for like ten plus years now and I was lucky to work on a lot of end to end machine learning projects where I did cover a lot of aspects on responsible AI, validations of models, governance, etcetera as well. Six months back, I got a chance to move to Lisbon. So after moving here, I joined Accenture as a associate manager in AI and LLM space.
Anjali Agarwal [00:01:06]: And it's been great so far. It's a lovely city and I'm looking forward to share some knowledge here to my fellow panelists as well, and talk to as many of you as I can.
Stefan French [00:01:19]: Hey everyone, I'm Stefan. I'm a product manager at Mozilla AI and we work on a product that makes it easy for the user to select the right language model for their use. My background is in data science. I spent just over like six years working in UK government on different AI projects and in the finance sector as well. And I'm based in London. Nice to meet you all.
João Silveira [00:01:46]: My name is João Silveira. I'm working as a data and AI support engineer basically at Microsoft. Basically, my day-to-day life is to help customers in using the Azure big data technologies. I'm SME into spark technologies mainly, maybe the ones that might have words about our Databricks fabric right now, which insights synapse for the data guy? Think for the data engineers in years, they're most likely are comfortable with these technologies, and well, that's pretty much thank you for.
Sophie Dermaux [00:02:19]: Hi everyone, I'm Sophie Dermaux. I am originally Belgian and gas by accident, but I grew up in London and I joined Onfido, which is an online verification company, about six years ago as a backend engineer, and then I moved as a technical program manager about four years ago. And my main two areas of specialism in terms of my day-to-day role is relating to machine learning infrastructure and then also data privacy and how to use very sensitive data as you can imagine for a verification company in an ethical manner. So that is me. Amazing.
Allegra Guinan [00:02:56]: Thank you so much for these introductions. A little bit about myself. My name is Allegra, I am based in Lisbon. I'm currently the CTO at Lumiera, which is a boutique advisory for the big organizations with responsible AI strategies. I have a background managing data engineering and enterprise engineering portfolios, and I'm really excited about responsible AI. I have a lot of thoughts on it, and I'm sure everybody here does as well. I have gone to a few meetups and conferences that cover this topic, but it's really in the theory and principle lens at level, and it doesn't go into what it actually takes with this into practice. What do the engineers, what do the data engineers need to do in order to take what you're saying you want to happen and make that a reality? So that's what I want to cover today.
Allegra Guinan [00:03:48]: The 2024 Harvard AI index report came out this year. You didn't get a chance to read it. Okay, it's quite long, but it defines four key dimensions of responsible AI. So privacy and data governance, transparency and explainability, security and safety and fairness. A few of these things are really present, I'm sure, in all of your work. Maybe it's not through the direct lens of responsible AI, but you can pull some examples that you've seen right now and tied them into this discussion. So just a general question to anybody, I'll let anybody take it. So have any of the dimensions that I mentioned so far found their way into your work? Does somebody start coming to you and say, we need to implement AI? Do they say, we need responsible AI? Has anybody ever mentioned it in your workplace before? What's your experience thus far?
Stefan French [00:04:43]: Sure. I mean, I'm so. I mean, I guess there's kind of two ways I can ask. This is one, which is working out Mozilla AI, like responsible AI, is kind of the core mission the company. So a lot of the principles about how we work, how we develop, and how we put products out there is based on transparency and trustworthiness. So things we think about, when we think about responsible AI is like developing in the open and making sure we put that out there are open source, and that helps with transparency. Look at the code. They can challenge things if they think things are wrong.
Stefan French [00:05:19]: So that's really important point of transparency perspective. And then we also think about making sure that our products are accessible and that anyone download the product and deploy on their own infrastructure. I think this helps a lot from perspectives of data privacy, and then the user and the customer can own the product and deploy on their own infrastructure and have control over their data and the models that they use.
Sophie Dermaux [00:05:49]: My experience has actually been a little bit more client led. So Entvedo is very much a european firm. We always have european clients, old north american clients, often kind of in the fintech and financial services space. And due to regulations, they have forced us to, which is a good thing to start understanding the bias in our technology. So as you can imagine, if we have mainly us and european clients, they're often us and european users in the end, who go through our system. And there's been a huge push in the last two years by a lot of our clients to have to try and start proving how, if we do and don't have any bias in our systems and how we're trying to mitigate it. It's been a huge challenge because to be able to go into these new markets, we have to show people performance, but then without going into those markets, we can't get a good performance. And so it's been a quite vicious circle in terms of the data that we need to ensure that we don't have the bias, particularly when it comes to biometrics and so taking pictures of people's spaces, because if we, as you can imagine, certain people conquer access to core services that we then provide access to.
Anjali Agarwal [00:07:02]: I think in the past few years that I worked in, I mainly worked in a financial services firm. So all these aspects of fairness or security or transparency have been like the pillars. So you can't just go on and create a model in a silo. No, that would never go to production just like that. So there has to be a proper ethics form. There have to be, you know, some rules. You have to clearly abide by those guidelines and see where you are pulling that data from which you're going to analyze. What, what challenge is it solving for the business? And are you taking care of those security measures related to the data? Let's say you are using healthcare data.
Anjali Agarwal [00:07:53]: So is it certified by HIPAA or something? Let's say for America. Right. So it is very important to understand about all of these aspects, especially in these forms. But I think overall also, it is very important that all of these models or any teams that work on these things should, from the beginning itself, when they start with the project, they invite these things in the development process, not just at the end, but in the.
João Silveira [00:08:26]: Starting itself, both regarding data privacy and security. One thing that across the years has helped a lot, and that right now I see that the organizations that Microsoft works with and their data platforms best suited for is that, well, a lot of times the data was split into different places. And right now, all organizations are working to have the data secured into the same place and having the data into the same place, it's really good because it will be easier for use and it will be easier to manage. Right now, across the last two years, I've been seeing an increase of customers of Microsoft asking about data governance, data privacy and. Well, fortunately, I believe that the way that the data worlds has been involved in the way that the data platforms the organizations use, they are more well suited to use them and to make sure that these data can only set of people access the traffic, Google, private, etcetera. I feel that that's a topic that I see more and more times, not only because of the hype of AI, but as well it's driven maybe due to government requirements like LGPD or even like the laws. So I think that the companies are more suited for them for. But each more and every time I see more companies with specific people, like two, three people only responsible for the data governance, to make sure that the data is only accessible to those ones that are supposed to and that is safely.
Allegra Guinan [00:10:13]: Thank you. This actually brings me to my next question around who should be setting the requirements and if you're getting those right now. So Stefan and Sophie, I'm curious, from your perspective as you're a bit like further upstream in this, are you setting the requirements when you're thinking about something that needs to be prioritized and it's going into the roadmap and you're going to start pushing for it? Are you thinking about the ethical side of things or any of these other.
Anjali Agarwal [00:10:38]: Dimensions that we mentioned?
Allegra Guinan [00:10:40]: And are you explicitly writing them out so that people can follow it on the data and on the downstream side?
Stefan French [00:10:46]: Yeah, sure, I can go first. And actually I'll probably draw a little bit more from my experience as a consultant in government, which I think has been more relevant. So I was working at a kind of large UK government department and there was definitely a fear, it was this fear of not doing responsible AI because they're under a lot of scrutiny, particularly from the press, about any decisions made by AI. So there was definitely actually a top level stakeholder fear that they had to do something about it. Our approach that we use is actually following quite good framework that's available publicly, I think was published by the Alan Turing Institute, which is around the ethical way of implementing AI. And our approach was trying to think about it on three levels. One is taking a bigger picture, step back about what you're actually trying to do and thinking about how it's actually going to impact the people or the customers it will affect before actually starting and building a feature or a model that's going to impact people thinking, actually, is this the right thing? If that's still okay, then once you're actually developing it, looking closer at the data and saying, what data are we actually using? And is there going to be any bias and discrimination in this data? And if you're building a model, you can use things like Shakti analysis, this kind of thing. Then the third one, which is, I'd say the most important, is while you're developing it, not siloing yourself as a data science team, making sure that all the documentation of how the model works and what data is being used is super transparent, not just within the team, but also for the senior level stakeholders.
Stefan French [00:12:31]: And this is actually quite okay to do within the team because everyone speaks the same language. Data scientists understand each other, but then when you're dealing with senior level stakeholders, a lot of them don't know anything about data science and MLK. So you really have to make sure that you translate it into a very easy, understandable way so that top level stakeholders are very much aware of the risks that they're taking. And I say the final thing is making sure that it's not just the accountability of the program manager or the product manager to implement responsible AI. It goes to the level of the data scientist. So it's very important that the data scientist is conscious about these things in terms of thinking about what data they're going to use, even like what model they going to use. Should they use a neural network when actually something is much simpler and more easily explainable, like a random forest classifier would do better. So that's the kind of thing that we would use.
Sophie Dermaux [00:13:29]: Yeah, I can add on to what Stefan was saying also kind of slightly disagree in a way, based on my personal experience on FidO, sadly. But that's just the way of the world of work. I think that what I've seen, a lot of the conversations around responsible AI is kind of pushed onto the company, like I mentioned earlier, with the client's expectations. But recently there's been some restrict biometric laws that have been put in place from the United States onto company, and there is actually quite a lot of friction internally versus compared to what the law states. It wasn't very well written, incredibly confusing what it is up to us. It was so up for interpretation, and I really loved Stefan's approach of being thorough and really thinking through. But unfortunately, in our case, we had to put something rapidly into place and it made that there was actually very difficult from like an internal perspective because it made for a very clunky UI. We had clients that complained, but it had to be done that specific regulation, a specific time.
Sophie Dermaux [00:14:32]: We didn't make lots of friends at that moment. But one thing I do think is really cool is that from like a team perspective, so that's kind of imposed onto us. But I do think as like a research and kind of machine learning department, the team are very proactive and trying to understand what they can do to be more ethical themselves. So that point around like transparency of documentation, we work really closely together in understanding what we can and can't use and consider things from the end user specifically. So my experience is a bit contradictory.
Allegra Guinan [00:15:03]: I guess I'm curious on the data side, if you're facing new challenges from these kinds of regulations that are coming through, or maybe you get a set of requirements that the expectation is that you are going to be compliant at the end or something, it's coming up super quickly that you need to act on. Are you seeing new challenges in this space as far as data governance goes?
João Silveira [00:15:24]: I don't build the product itself, but I have a lot of customers from different segments. And yeah, I can say that for example, usually financial institutions are the ones that are more strict with it. And for example with LLMs, when saying that has been brought, that I've already worked, I'm still ongoing. For example, with a customer that is like, we are used to work a lot more with Tumblr data and with tableau data, it's quite easy to see OSX table. It can even be done at column level or at row level, those kind of things. However, one problem that sometimes they came with is like when using LLMs, because the LLMs are trained upon data and then they might have answered to everything. Or another way that they can do it is to build racks upon others. So it fetches the date, it searches the data, and it builds the response based on the data that they can see.
João Silveira [00:16:31]: However, in those regards, there is not much. It's less straightforward, it's more tricky. The way that you can say, hey, these users can have access to these documents, these users can have access to this. It's more tricky. And I felt that there are some projects that were delayed for these kind of situations, even like with post live, that they were supposed to go to production for some customers that were like two, three months, these kinds of things.
Allegra Guinan [00:17:00]: Yeah, im actually really curious about that on the project management side and from all of your perspective, like, what have been the things that are the most challenging as far as, like, resource allocation or the amount of time things take. Like, obviously, things get reprioritized all of the time, and delivering fast is everybody's first goal. You know, they want to push things out as quickly as possible. So, yeah, I'm curious to build on that. What other challenges you guys are seeing specific to this area?
Sophie Dermaux [00:17:31]: In my experience, one of the biggest challenges has been relating to what data is used among different engineers. So we have to kind of segregate our data sets dependent on what's being. What it's being used for. So which department, what type of model it is, if it's used to combat fraud, or if it's for other purposes. And that's actually very contradictory of speed because engineers want to be able to share the data among themselves. Imagine you're working together, and you've got the perfect data set for. In our case, we do biometrics, but also we check your documents. Imagine you have a perfect french passport data set that you've gone through and you've labeled, and you see that, and it's great, but you can't actually share that with another engineer to be able to be used, because they're actually using it for a completely different model.
Sophie Dermaux [00:18:19]: And that at this moment in time, Amphido is still incredibly manual. So we have to discuss it as a team, what we can and can't use. And that causes a lot of delays about the challenges.
Allegra Guinan [00:18:32]: And from the ML engineering perspective, let's say you're working on something and you're being asked for it to be, like, transparent, but also super accurate and explainable. And I needed to be done, like, today. What kind of challenge are you facing there? Is this possible? Are you seeing it being possible?
Anjali Agarwal [00:18:52]: Yeah. So there are challenges at various levels. So one thing is, when we are asked to, let's say, do an analysis, first thing is these companies have so much data now, it's difficult to find out where the data is which you really want for this analysis. So it's like a haystack, and you're trying to really find out, what are those things that I want. Right. So that challenge starts from there, and it takes the maximum time in finding out the actual data that you want. Once you find out the data, then you start to clean it, and then you find another challenges seeing that, okay, this data is not even complete. Oh, half the data is coming or recorded from 2022, but other data is recorded from 2020.
Anjali Agarwal [00:19:44]: So how do you bring them on the same level, right? Because you either get the data for the same time frame, right? So the challenge starts from there. And then when you are developing the models, then you need good computing. So if you are going for a higher computational kind of model, like a deep learning, or you're going for something which needs more computation, then you need to work with the other teams, like data engineering teams, etcetera, where you tell your use case, you demand for more computational machines, and then you build on it. Now, your model is built, then you work with the production teams, or again, the data engineering teams who help you move that code to production. So now you have to be in sync with the data engineers. You explain them what you have done. This is your model, and how do you what needs to go in production and whatnot, right? So that is one challenge in the development cycle itself. Now, another check comes with the governance side, right? So, in bigger firms, there is a yemenite separate team for governance.
Anjali Agarwal [00:20:56]: There is. It's like a jury. So there are three steps. There is a governance team, which has given you a form of, like ten questions where, like Stepan also mentioned, you have to put everything about the model where you get the data from, where you're using it for, which is your affected audience. Right? Like, when you implement this model, what is it gonna affect? So all of that is mentioned there. And then you have to answer and convince the jury that this is what my model is going to be used for. Then the other part comes the bigger part, which is the ethics part. Now, in ethics, you have to explain them that why is your model fair and not biased? Right? So you show them by doing various checks, using jurity, etcetera, showing that my model is not biased towards any gender or race or ethically or all of those scenarios.
Anjali Agarwal [00:21:53]: So this is a long process. Right? And at each step, we have to be very careful. We have to be very much presentable of what we have done and why we have done. And at a broader level, all the models are categorized in green, yellow and red categories. The rent categories are the most critical models. Critical models means they will impact the organization much more than green and yellows. So that's where all these security and lot of questioning and a lot of audits also happen, specifically for those models where we have to be very, very clear of why we are building, what we are building, how is it going to affect, and it's not biased in any way, etcetera. So you might have seen some of the real life examples as well.
Anjali Agarwal [00:22:49]: Like one big firm was hiring a couple of years back, and they were using their own historical recruitment data to find out whom are they gonna recruit next. Right. So that's what they were putting in the model. And their model was biased for male employees because in historical data they had more male employees. So now their model, this model was biased. Right. So something like that should not be put directly in the market or in production because that will really, that can tarnish the, you know, the reputation of the company as well. Right.
Anjali Agarwal [00:23:31]: So there are measures that have to be taken to fix such things.
Stefan French [00:23:37]: Yeah, I would say. I wouldn't allow that, I think. I mean, I agree with all of that. For me, from my experience is actually sometimes, often just like the wrong incentives. When you're working on a project, like you were saying, having to deliver something quickly or deliver value quickly and, you know, responsible AI is important and maybe even talk, come up with some ideas, but then it becomes like an afterthought because you're kind of heads down, focused on delivery. And one time this was extremely apparent when we wanted to improve the performance models we had in production. So we decided to run a hackathon to be like, k, yes, we're going to make this way better. And everyone was super excited and we made the goal just about model accuracy, which was super fun for the data scientists.
Stefan French [00:24:22]: And everyone went around looking at the data, came up with a bunch of new features, and the model got a lot better. But then actually we had to present back and make a justification for releasing this new model. And some of the actual more senior stakeholders actually challenged a lot of the features that we used and at this point basically said, we've got a doubt about whether this one is actually going to be biased. A lot of that hard work got on done because we just didn't have it as an incentive. So, like, I just think, like, it's important for the idea that, like, responsible AI and doing things with an explainability angle is there from like, as like a target as well and isn't like, not at all.
Sophie Dermaux [00:25:08]: Yeah, because I can, I can add to that thinking about responsible AI and obviously mentioned as data scientists, have a lot of expertise in the space, but that's where it's good to have varied profiles involved in who you're working with. Like, an example is we have, like I mentioned already, the biometrics base, but we have a lot of data related to your documents, an IoT document. And we had a period of time where we were really trying to grow an AIPAC. And there's certain countries, AIPAC that require for governmental purposes to extract your race, your gender, and if you're married or not from your identity documents. There's certain countries still that have your marriage, who your father is, et cetera. And then those people would then get access to services dependent on what's in that document. And for a long period of time, the team were like, okay, cool, we can extract that. There's an XYZ sale.
Sophie Dermaux [00:25:58]: We'll get this money from it. And it fall, actually, from a product perspective, from an engineering perspective, from like, a sales all made sense. And then actually, it was only when we started having to get involved with the legal team, who then were like, we're a european company. We cannot extract and store that information. And it put everything to a halt. And then now I look back on it and I'm like, so she like, what? Yeah, like, what? But I think sometimes when you go onto the, we go with momentum, and you see the importance of something from, like, you know, like, in terms of, like, a teamwork perspective. You're like, okay, you're great. They great, great.
Sophie Dermaux [00:26:34]: It's very similar to this hackathon idea. It's only when someone who's completely extracted from the conversation, simply put, goes, you're working on extracting this information, you're like, you're allowing that. It's only then when you're then taking a step back, which is why I think it's always good to have very profiled knowledge, skill set involved.
Allegra Guinan [00:26:53]: Yeah, that's really good point. I'm curious on the retraining or pivoting after something like this happens, right? Like, you spend all this time, you build something you're about to put into production, or you do maybe, and then you realize, oh, wow, that was like a poor decision. Or it's actually biased and realized because we weren't checking for that previously, have you had to deal with that situation or thought, what would you do? What's the process now for fact tracking? It's the public now, right? Like, you're using it in your product. What are you going to do to handle that?
Anjali Agarwal [00:27:27]: Yeah. So now that we have discovered that there is a bias in this model, now what do we do? Right, so now from at each step, you want to really be careful.
Sophie Dermaux [00:27:39]: Right?
Anjali Agarwal [00:27:40]: Now, as a data collection itself, suppose we have to really check, let's say gender is one feature that we are using for our model. We have to make sure that we are using equal quantities or the data for both the gender, considering there are two gender categories there. So we mentioned both in equal proportion, that is very important, right? Otherwise, in the later part, you will find that, oh my God, 70% of my data was about men and only 30% about men. So obviously there could be a, there's a high possibility that there is a biasness in men, right? And even if it is not discovered now, it will discover later in production when next year's data. So it may pop up later. So you want to fix it before going to the production itself. Right? So first is at the time of data collection itself. Now, the second thing is, sometimes there are some proxy variables which do not directly talk about those specific variables, but they look like that.
Anjali Agarwal [00:28:42]: For example, occupation, right? Like occupation. In occupation, I have mentioned housewife, housewife, housewife, etcetera. Now, it's, it's not directly saying a gender, but housewife is mostly a vib, right? So you have to be careful about these variables as well. That is so exploratory. Data analysis is very important and we need to keep our eyes open when we that, right. We have to make sure that we are not imbibing the bias from there, that point itself. Now, while we are treating our model, then we can use regulation techniques to add penalties. Then while validation, we do a manual check where we try to plot our graphs in such a way that we compare the model variables like gender, race, etcetera, with the target columns or whatever we are trying to achieve with how is it impacting.
Anjali Agarwal [00:29:38]: Right. So all of those measures we do. And then we also do fairness and bias tests, which are like durity and some other tests, they clearly show you disparity or if there is a bias on the location, location wise bias, etcetera. So all these things will be evidently shown using these libraries. You can get drafts, so it will tell you that there is a bias or a. So basically you find a bias, you go back, fix at every step, you come again to the final step until you minimize the buyer as much as possible. And if sometimes they say that it is impossible to remove 100% of the bias at times, and that is true, but then again, business has to take a call and we have to understand that is it. Okay, so depending on the model criticality.
Anjali Agarwal [00:30:39]: And so for example, we are giving more loans to someone who is from a specific race, right. Then that is really wrong for a bank to do such a thing, right? So that is something that you should not push to production, as in you should not. So we need to be careful where we are using what features when we are training our model. Right? So those are some of the things.
Stefan French [00:31:09]: Yeah. We did pretty similar things, actually. It was two things really. Like. One, whenever the model is retrained with new data, we have like automated explainability report that was generated in review. And then the second thing is just having like live power bi dashboards that we had, which was just like characteristics you were looking out for in terms of discrimination, like gender, nationality against the target variable of the model, and then just monitoring that. And if it hits the summit threshold, we would just go back to look at the data and get people who actually knew about the space to manually review cases and then update the data.
João Silveira [00:31:44]: Yeah, one thing that I've started seeing for the last month that's, well, for regular data science, let's go all. It's really difficult to do the typical data exploratory analysis and then cleaning up the data, transforming it, etcetera. However, one question that usually raises that I believe that the companies are more used to do it, is that, well, if you are using LLM, you can just throw basically all data there, because it's only text data after all, and then it will be able to search either so the data or train on top of that. However, though, I believe that out there, it's really hard to do some kind of exploratory data analysis to understand the people that were described, for example. And one thing that I've started seeing from some big corporations is that starting to doing a mix of data processing with NLP on huge data documents to remove names. I think that for removing genders, it will be a really good approach as well, because I think that data scientists, AI engineers should be really used to remove genders from, from, from a table. It's really simple just dropping out the table. However, if you are working with texts, it's something that requires some extra work.
João Silveira [00:33:04]: And I've started seeing some of that. Some big corporations that they process the world data and just like leave the, just like solid, the important tokens that are text data that is used to train the models. But something I believe that a lot of companies might skip the best and just give all the files to the model or just put them into the vector database that the models are built on top of. And they forget that sometimes in these cases, on bots and on LLMs, I believe that it might be way more disguised than people might be able to understand. Whereas for example, on data, it will be easy to see if we have 70% of this or 30% of this. And on both side, I believe that even the users will need to be more accurate and to understand better on where's the bias and the fairness or not, because it's not statistical.
Allegra Guinan [00:33:56]: On looking to robustness reliability outside of the dimensions. Are you facing any challenges right now with this for like being innovative, which is again something want to push for. They want to move fast things out there. And maybe you're creating some models or you're working on projects that are lower stakes, but it needs to move into like a higher risk situation or more critical. You know, you're describing the different levels, yellow, green, redh. Have you run into a situation where you're being into a more critical space and you need to think about scaling now. You need to think about the reliability and how do you make sure that that gets built into the process?
Anjali Agarwal [00:34:44]: Sure. So there are two aspects in AI now. One is either you use the models that are trusted now, let's say random forest or XGBoost. There is a lot of trust in these algorithms now. But on the other hand, there are new algorithms being built every few days now. They are coming up very fast. It's a very evolving field. So do we use something which came in the market just last year? So there's always a, a tussle.
Anjali Agarwal [00:35:23]: So what the big companies do is they, they experiment with the already set algorithms and the new ones as well, and then they figure out which are giving me what kind of results. Now, if there is a slight trade off of trust, as in if the results are very close, then they will go with something which is more explainable and which is something which is more reliable. Right. Because they have seen in many cases that decision trees or let's say xgboost works very well. So we'll go with that rather than experimenting with something which is just came in the market last year. Because in the new one, you cannot custom a lot, there will not be much documentation available and not much people will know about it. It has not been explored to the depth. Right.
Anjali Agarwal [00:36:18]: So we will. So depending on what the situation is, if it is something where we are okay to experiment, then maybe we are okay with going with the recent algorithm. But in most cases, if something is going to directly hit our bottom line or top line, we will go with something which is stable, known in the market for years and we are reliable about it. Also, in terms of reliability, there is a very big say of explainability, because any team will have to justify to their board members or senior leadership, why are they using this model and why should they trust this model results. Now, how do you build that trust? They will only trust it when they understand the model. Now, to explain, to increase the explainability, it can be done in two ways. Either you use a model where the explainability is inbuilt, for example, linear regression, or like a decision tree, like a linear regression, you already know that coefficients are directly telling you a story about which features are more important and which are affecting your final outcome. Let's say sales more, right? And in decision tree, you can see the flow, okay, this way it is going.
Anjali Agarwal [00:37:43]: And because of these rules, you are finding you are coming to this conclusion. So you can tell a story to the business partners. But on the other hand, there are a lot of black box models where you cannot tell or cannot explain it that well to the senior members. Now what do we do? Right. Then we use things like sharp curves or line curves. These are some of the libraries available in pandas, in Python, which you can use for explainability. They clearly show you which features are impacting the model, how much impacting the outcome, how much. So you can clearly show them with the graphs that, okay, this is why I'm saying that you focus on these features rather than the other features.
Anjali Agarwal [00:38:31]: Right. Then there are something called as PDP's, which is partial development dependency plots. They give you results on each feature level. So that way also you can very well explain the senior management that, okay, these are the features why I'm using for this reason. So when this trust builds, you can talk in business terms, you don't have to really talk in data science terms and you can still get a buy in. And that's when I think the trust and reliability for your outcomes build, because many times when they don't understand, they are not convinced to move ahead with the business decision. And they are the business decision makers, to be very frank. So if they don't give a buy in, we are not going to move it any further.
Sophie Dermaux [00:39:19]: Yeah. To add to what Andre said, this whole like business maker decision makers, they're often actually not those that are involved in the implementation. And so there's the elements of robustness, but also like proving the robustness per se in an explainable way to someone who doesn't want to know the details, but just wants to know that the company is not going to go on fire. And so what we've had to do on Tito is we actually, as a team, decided to like generally from a decision made from the research and machine learning team to then on to actually implement something in production. We have about like a week to a week and a half of like a testing phase. So where we replicate in our testing environment, our current production environment. So we can actually then see what clients will be able to experience once we ship something. And then we have a dedicated team.
Sophie Dermaux [00:40:12]: They say team is generally one person who reviews and tries to understand the depreciation. I can never correctly say this word, depreciation. Depression. I know when it goes, sorry, depreciation.
Allegra Guinan [00:40:25]: Thank you.
Sophie Dermaux [00:40:26]: It's always good to have a non native english speech. The piece of this, on the, on the analysis of what our clients will experience, because what Andrew was saying is, it's so true that there are so many implementations that you continue as an engineering team, but then no matter the level of granularity and documentation that you put, if then in the experience of the client, it's completely different to what you expected, then all those details of the documentation isn't really worth anything. So we've actually had better lag in terms of implementation. When you say the clients, and I.
Allegra Guinan [00:40:59]: Mean, you're obviously speaking from your perspective, you have a lot of background on me, able to choose like what the best solution would be. Are you finding that there's a lot of trust put into engineers to come up with the best option out there and also then an expectation for them to be continuously learning and aware of what any new model might be that they work with or. Yeah. How much trust is put onto the engineer from your, um, experience right now. And I want to take responsibility in a different direction after this one, but I'm curious what your experience has been so far.
Anjali Agarwal [00:41:35]: Yeah, I think data scientists have to have a combination of a lot of things, right? Like they need to understand the business. They need to understand maths, statistics, as well as the, the project, right? Like they business, technology and maths, all three. So they are present in the meetings with the senior managements as well, where we understand what we are going to build and why we are going to build. Then we give our suggestion, come up with a project plan of what we can do for a thing given to us, right? So like business will just tell us, my revenue is right now going 18%. Can you do something to make it 20% by next year? Just a business problem in one line. Now we have to break it down to every possible solution that we can think of using whatever. They don't even tell us that use data science or use data analytics, right? It's just a problem now, how will you solve it? So then we break it down in thinking that what are the parameters that affect their revenue directly or indirectly? So that's how we break it down. We create that solution, we propose that solution.
Anjali Agarwal [00:43:04]: There are a lot of to and fro meetings that happen where we propose case one, case two, case three, we go with different kind of answers and convince them why, what we feel about the different solutions that we have proposed and what are the techniques that we are going to use, how we are going to use it, and then when they approves, then we actually start building it. So it's a whole process, end use agile. So every two weeks we are in sprints. So it's a fail fasten kind of method. Sometimes we know in the usage itself where we are going to break it and we fix it right then, but sometimes we are not able to do as well. There are lots of dependencies, there are lockers at times, but we keep the management informed about all of that. Also, when we start right at the starting itself, we do a pre analysis. Now, that's something that we have recently started after so many years of our experience in data.
Anjali Agarwal [00:44:10]: We do a pre analysis where we previously only look at certain features and we look at their dependency with the target vehicles or the outcomes. So just that it's like, it's like a mini project before the whole project, you know, MDP sort of. So we just showcase them with a smaller stop the data that is it possible or not? So if it is possible, then we go ahead and build a full fledged solution. So business is so much involved with us at every step in this whole lifecycle because it's crucial, right? It's something that is directly going to affect their business. It's going to impact their I revenues or profits. So, yeah, we are all within goal.
Allegra Guinan [00:45:00]: I'm curious on the product side, how much you're putting onto the engineers to figure out the solution here.
Stefan French [00:45:06]: So I would say, like, from my experience, practically speaking, I think a lot of the responsibility lands on the data scientists and engineers. But I think fundamentally they have a lot of, they're the ones with the knowledge and the knowledge of different algorithms. You can use different models, you can use the latest models and a good sense of which ones are more explainable, which ones are less explainable, which ones are going to be more of a black box. So I think, frankly, realistically, I think you have to put a lot of trust into your engineers and data scientists and also ensure that they are very much aligned with your values and principles. And I think there's, that's kind of the reality from my perspective.
Allegra Guinan [00:45:50]: So knowing that, are you seeing and does it have to be in your company, be another, it's like continuous learning being prioritized for engineers or, you know, we're talking about timelines, working sprints. So are you making sure that you're setting aside some capacity allocation or learning, or being able to, you know, try something new for a couple of weeks in our determine if it's the right path for you? Maybe that's part of the lives that you're mentioning for some of these projects. I'm curious about people understanding what it takes to have that knowledge and to stay on top of all the latest innovation, which is a lot of information and a lot to learn, especially if you're trying to put something into practice, into production, and test it, make sure it's soluble for your customers, et cetera. It's a lot of pressure. Or you see people prioritize that on the chance that.
Anjali Agarwal [00:46:44]: Yeah, so I think we are sometimes so involved in our project work that, I mean, if it is totally left on us, we will probably put the training part on the last part of the year, but our curriculums now, or our goal sheet itself saying that 20 hours of training mandatory. So we do mandatory, the training which is on all kinds of technologies, so that we are aware of what's going on. But in our space in itself, we have to do continuous learning, because if we won't know what is new in the market or new LLM version has already come up, then how will we compare? So it's always, I mean, we have no other choice, only we have to read about it, so only then we can implement. And there are, I mean, there are a lot of algorithms which are still developing. There are some version of it available, but there are continuous discussions that keep happening on stack overflow or quora, or some other sites where we keep learning. How did they solve this particular problem that they changed while we were doing this algorithm? Right. Almost every day we see ten tabs open just to understand. Oh, I'm stuck here.
Anjali Agarwal [00:48:05]: What? Okay, I understand this new thing now. Okay, how do I set this hyper parameter? Okay, like this. So sometimes we fall into the problem and then learn, and some, some of the training we have to mandate and some of the learning we have to do, because we have to keep up in this data science world, because it's evolving so fast that it's. It's almost hard to catch up on all the feeds that come in from towards data science, or so many portals sometimes just read the headlines, but it's important to keep a tab on these now more than ever, I think.
Allegra Guinan [00:48:43]: Quick plug for mlops community slack questions on there. People are always checking.
Sophie Dermaux [00:48:49]: That's also the point that Anjali mentioned around, like having to stay fresh is that one thing I think that's awesome about on video is that we do some voyages. I don't know if that's common at other teams, like where someone will go and work for like a month in a competitive department, because maybe I'm speaking just for myself. Some people love training, but when someone like makes it obligatory for you to join a training, automatically it kind of puts me off. Whereas if you're going into a completely new situation, which means you're going to have to learn and see something in the new mindset, I think you're then more likely to adopt these things. And so we found that people actually introduce a lot of new ideas by having to meet completely in a different setting and encouraging you then to look into things and try out different things. And so it's like months, sometimes two months of kind of rotations are a really good concept.
Anjali Agarwal [00:49:37]: Interesting.
João Silveira [00:49:38]: Well, on that I cannot complain because my company I provided with some days for studying, etcetera, but I believe that's everyone that wants to strive on these worlds needs to do their own thing, keep connected on LinkedIn. And I think it's 2 hours past my work shift and he and I are still here talking about work related things. So I think that pretty much everyone that works in the tech world needs to stay connected. And even we don't call this work, but it's. I think that everyone tries to be up to date monster based technologies as well.
Allegra Guinan [00:50:17]: We were just talking about this before it started. I wanted to see if this direction after asking this because it is such, it's a high expectation, it's high pressure. Things are moving really quickly. I know from the program, technical program, you see the high level, you see how people are to bring in new things and deliver value really fast. Do you feel like as people, humans, not machines, that are expected to work and deliver. Thank you for sending two extra hours of money, but do you feel like, okay, I'm working on responsible AI, but management is not giving me the time and space that I need to develop, choose the best thing. And they're not really respecting me as a person necessarily because I need to push something out at like is that expectation aligned with what they're asking? I'm really curious about that.
Anjali Agarwal [00:51:13]: I think in my space definitely there is. I mean, it's difficult tell the business that I'm going to deliver this in four months. I know we can tell this for sure. Because if my model is not going to work with the data that is available for this task, I will say that not every data science project will actually come to a finish line. Right? So many times you will see that the accuracy is not that great. I'll probably try something else, then retrain the model. There are a lot of iterations that are happening, so the best way is keep your stakeholders involved at all times. Like, keep telling them that I'm at this stage, I'm facing these difficulties, and this is what is slowing me down.
Anjali Agarwal [00:51:58]: Is it computational problems, is it data related problems or whatever? Because then only they will understand that, where is she? Right? Otherwise they'll always think, oh, last month ask for an update, and now I'm seeing that it has only reached here. So that expectation setting is very important because unlike already set environment, like, I would say, when something like a migration project in a technology or something, where you know that, okay, this is the way it has to be done, right? It's a structure and you know what is to be done. But here, you don't know if it's gonna succeed or not, right? So it's very important to keep them informed and let them know. It's, it's stressful, I would say, because we don't know the outcome yet, right? So it's, it's always like we ourselves don't know, what do we tell them? Right? So, so there's always this tussle. So I would say it's difficult to even say that I will complete the data acquisition part in one week. Even telling this itself sometimes is difficult because I can only do certain part. But maybe something is left, maybe some things are left for. And I later discover that this should have been involved.
Anjali Agarwal [00:53:16]: So it can happen in phases as well. Like, some teams just follow the sprints and they say, okay, you go with what you have collected till now. Okay, so set a timeline. One week take for data acquisition. You got it? You move forward, you move forward in model building. Move forward, move forward, move forward. Now, at the end, when you see that, okay, then you list down what are the things that you can apply to, you know, better, mend the model. Then you go back and create it as phase two.
Anjali Agarwal [00:53:49]: Right? Now, in phase two, I will add these features. Now in phase two, I will do hyper parameter tuning. So things like that. Otherwise, in data science, it's very difficult to push. And I remember in my last project in last year, the time to market for a data science project was, I think, eight to nine months for one project to go from the scrap from the beginning to production. It was nine months on an average. And if somebody could do it in like seven months or eight months, it was considered like an achievement. Wow.
Anjali Agarwal [00:54:27]: Because there are so many aspects, right? So it is definitely very tricky, but the only, only thing is keep your management on board. If they know that this is how it is, then they will understand it and, and the expectations and, you know, their, their thoughts about where to use that outcome will, will be on time. Right. So they should not expect like four months will implement this? No, it cannot be done in four months. Why? To set incorrect time.
Allegra Guinan [00:54:54]: Do you think companies would allow nine months if you gave them that timeline or. What's your thought on this?
Sophie Dermaux [00:55:02]: So, I mean, my thoughts. Sorry. Just in general, on this topic of.
Allegra Guinan [00:55:07]: Like, expectations around engineers and, like, being able to deliver on, I think they.
Sophie Dermaux [00:55:14]: Would allow nine months if they, if you really pitched that, it was incredibly complicated. And so they'd be like, okay. But generally, I've never seen anything other than two, three months.
Stefan French [00:55:26]: I think, like, when it was at its worst, I think for responsible AI, like, this was at my previous company where I had the client who had very high expectations on whether they're bringing value early. It was during the time of the peak LLM hype, and they really wanted an LLM solution for one of their business problems at all costs. And in that scenario, when a client wanted something, we built something that ended up being some kind of enterprise search with an Llmdeh. But at that point, the responsible AI side, which they had been very receptive to before, just got thrown out the window. And even in the solution, I wanted to put a little bit of a warning label saying, these answers are not very reliable. But they were just like, why do you have this label? And we're like, well, we need to be responsible about this. And I think in certain scenarios, I think especially when stakeholders have, like, top down pressure to really deliver something, that is the situation where it's actually sometimes very difficult to push back. But I think with more maturity, and if you can really show the value that responsible AI brings, I think that conversation can be a lot easier.
Stefan French [00:56:37]: But I think that takes a lot of kind of maturity and experience and being able to kind of show some of the positives it brings as well.
Sophie Dermaux [00:56:46]: Thanks. May I add to Stefan's point around, like, bringing value? I mentioned earlier this bias testing, this was something that, honestly, it was between me and two data scientists that were quite interested in it. We were looking at how we were performing at Fido we were like, oh, okay, okay. But there wasn't any, like, business momentum behind it. So we were like, okay, how can we go about doing this? And I was like, you know what? Like, I think I'm good as a TPM, so I speak to lots of teams. So I then went and spoke to the different clients and what was the like and different client managers and said, like, you know, this is what we're thinking. Have you noticed that clients said anything about it? And they're like, no, no. I was kind of going around trying to find the answer that I wanted, and then it was like, the holy grail was that we were onboarding a bank, and they were simply saying that we, that their competitors, our competitors were starting to showcase slide decks about the topic, et cetera.
Sophie Dermaux [00:57:43]: And so that was like, you can say, like, blood for a shark, you know, something. Okay, perfect. And so we then actually got, like, capacity and also budgets to create the datasets because we had to work with external testers to be able to get us, like, 60,000 different types of faces. So, as you can imagine, we didn't have that internally. It was very expensive, but it was only really through that business incentive that the project came to fruition.
Allegra Guinan [00:58:12]: It always comes back to the business.
Sophie Dermaux [00:58:14]: Yeah, it does. Yeah. Sorry.
Allegra Guinan [00:58:17]: Well, I want to be focused at the time, so thank you so much. I want to open it up and ask the audience if there are any questions for the panelists. Final thoughts.
Stefan French [00:58:27]: Hi. Thank you very much. I'm Bilal from unwavel engineering, manager of the AI team. So I was curious if, how many of your companies are using LLMs? Because as Joel pointed out, that it can be a lot more tricky with LLMs to measure fairness, bias, other things. So I'm curious if you're using LLMs, first of all, and if you are doing anything about all these aspect of things that are related to responsible aihdem, especially when it comes to fairness and biases and toxicity and all the other stuff.
Anjali Agarwal [00:59:06]: Yes, we are using LLMs, but we are very careful about where to use and how to use LLMs. So if it's a financial services firm or any, any such firm for which the data is very, very crucial, we do not want to fine tune LLM as such because there's a lot of cost involved. Also, we do not trust to give the data to it so that it trains. And we do not want to put that much money to fine tune it because we really have to first find out the business cases where it is really going to be beneficial for us. Rather, how we are using LMS is we are creating some development projects where we create like a in house tool, where we are using like a chat option or Q and A's or translations, etcetera. Basically it's a version, inbuilt in house version, but it's only for the development projects. But we are not trying to fine tune it or because we don't want to probably put that much budget on it and not get the real ROI. So this is now happening a lot in the AI space that how much resource are we putting for which project and how much outcome it's going to generate, right? Because now there are too many teams, too many resources working on AI.
Anjali Agarwal [01:00:50]: But if these models are going to run in silos and not really generate some revenue or profit to the company, then it's not really of a lot of things, right? So that's why we are still waiting kind of, and we are fully our position, I would say in terms of LLM, we are not full cats. We are not going to production with LLMs yet. We are just being easy on it. One space where we are using is like on Google Cloud, we are using a lot of vertex AI, etcetera. And because it comes as a packet itself, when you buy, let's say a lot of licenses of AWS, then a lot of our development folks can make use of those LLMs in their coding or finding out bugs, etcetera. It speeds up the development process. So I think that's the biggest use where the developers are using it, but nothing in production for now, you're more cautious than that.
Sophie Dermaux [01:01:57]: You can tell the difference in size, company size, and they wouldn't say where I work there is these types of consideration specifically for LLMs.
Stefan French [01:02:04]: That's my so we use, we use LLMs. We are building a product that is aiming to make it easier for end users to select the right LLM for their use case. From a responsible AI perspective. Product is going to be open source, so anyone can look at how it's comparing and judging which LLM is going to be best for that use case. We're also making sure that people can deploy this on their own infrastructure so they don't have to actually send their data to OpenAI or claw or whatever. So they ensure the data stays in their infrastructure. From a explainability angle. The LLM field is still very immature compared to explainability.
Stefan French [01:02:54]: If you want more traditional LLC machine learning models like Angela was talking about sharply values and that kind of thing, I think we're not really there yet in terms of explainability, LLMs, what we are trying to do is show and guide users that smaller language models can actually often perform as well as to large language models. And I think explainability is easier when you're going from billions and trillions of parameters down to much lower level of parameters. So guiding people to realize that smaller language models can be as valuable and as performed as larger language models will actually, I think, hopefully help long term in terms of sustainability when deciding what language model to use. Like Angela was saying before, when you're making a decision and you're like, oh, I could use XgBoost or some neural network, and I get 1% improvement in accuracy versus like a random forest or linear regression, it makes sense to use random forest and linear regression off because then you can explain it much easier to stakeholders. And I think there's, I claim there's value to going with smaller language models for the same reason, even though I think the explainability isn't quite there yet.
João Silveira [01:04:14]: My company uses LLMs. I think that, you know, it's called Microsoft, and the information that I have about it, well, internally, what can I say? It's like internally I have the opportunity to work and to be part of the test of them, because, for example, Microsoft 365 copilots, we use it a lot. And as inside Microsoft, as the employees are part of the program that is supposed to train the model as well. And we are the ones that are supposed to provide the feedback on that. Paul? No, I think that if you look at the Azure OpenAI european AI products that you'll see, you will see that there are some filters there that were built by the engineering team. I don't have much information on that, but I know that they have some fairness already implemented and there are even some triggers that you can even put for the aggression. I still know that there are a couple of filters that you can even stop the Azure open AI itself from, but I think that's the information that I can provide you on that. Maybe you might even know more than me, I would say.
Allegra Guinan [01:05:41]: My name is Sarah, I'm a chief.
Q1 [01:05:42]: Policy officer at Lumiere. Together with Allegra, I'm thinking about the concept of maturity. So you all mentioned the difficulties and challenges of convincing from a data engineering perspective, to business leaders that don't necessarily have tech background, and they also just want the easy reason to how they can increase their ROI. How about maturity on the end user perspective? So the customer or the client, how much do you think about the person that's using the service and their maturity and understanding because they may have questions around like, oh yes, I want you guys to do responsible AI, but they might not really know more than that need. How much do you think about that maturity level? And do you take that into consideration when you're talking to business leaders or do you think about it just on your own in your chamber?
Sophie Dermaux [01:06:31]: I can kick off. So in terms of the client perspective I mentioned earlier, there was generally, I found with clients pushed by regulations and then making them having to care. That's generally the experience that I've had working with specific clients. And from the end user perspective, as you can imagine, people are very sensitive around on video owning, having photo of their face and then their documents. But then there's actually very rarely do we actually get direct feedback. Under our terms and conditions people can contact us. And in my six years I would have been part of the team to handle that. It's only been one query directly from the end user.
Sophie Dermaux [01:07:10]: So I think there's a lot of, obviously that's not like maybe across the whole of the tech industry, but just in my experience. So I think there's maybe a lot of questions about it. But I wouldn't necessarily say that you've seen end users kind of question the process as much in terms of us using their data and how we do it. I haven't really seen that come to fruition. And what's interesting is that the considerations of trying to make it more protective for end users has, like I mentioned, like a real impact on our UI. We've had to include incredibly boring tests that they have to tick off, and then they've just seen a huge drop of people not wanting to use the service at all. So that's also then consequently meant that they've had to try and find other solutions or maybe go to a competitor. So it sometimes can be a bit of a clash.
Stefan French [01:08:04]: Amazon AI with our products, our users are developers, so they definitely have our level of maturity and understanding of these things a lot of time. Our advantage is that web sites are open source, so if they have concern feedback, they can go on the GitHub repo and they can collaborate with us and they can make suggestions or improvements or ask questions. In terms of how we think about it, I think it's more from a collaboration perspective than necessarily thinking about collaboration is the key on site.
Anjali Agarwal [01:08:45]: I think when it comes to maturity from the client side, many times we see that client is not mature enough to even know of the consequences of responsible AI. Frankly, many times they think that it's just a check that you have to do at the end and you will do it and it's done. But it's not really just this, right? It is, it is something bigger. Think of a example where it happened in the real life, right? Like some time back, I think it was with Apple, the credit limit that they were giving to. I think it was a case where there was a credit limit given more to a men as compared to women, even when the women earned more as compared to men, right? So again, on the historical data, they did this analysis, but they did not probably do the proper checks and everything. And there was a bias in the model. So it can backfire really bad if they take it lightly. And a company whom they are working with to give them an AI solution, it's their responsibility as well to make them aware of what responsible AI is.
Anjali Agarwal [01:10:05]: So they should talk about all these aspects that, I mean all these risk assessment checks, right? Like where is the data coming from? Is it compliant or not? And what are you going to use it for? And is it not biased for any race and color and gender etcetera so many a times? It's not that they are doing the mistake knowingly, right? They probably don't know about it. Like the client is new in this whole AI space and they want to implement a solution. Now, whichever company is helping them create that solution can probably tell them that this is an important thing and probably these are the consequences that might happen if you do not adhere with that, right? So you can get a mix back. Sometimes the client itself is mature enough to have another set of risk assessment form itself. I mean they have their own set of rules. So sometimes you have to work with them to come to a common point where you provide that. Okay, we are checking these already. We are good things like that.
Anjali Agarwal [01:11:17]: But I think this is something important. Whoever is building a model or any solution, I think it's important to have this governance in place because later when we see after one year at oh, this, this is a problem, then it can risk by the reputation and it's a lot of loss for the resource money. The company has put so much effort into it. So why not take it from the beginning, right? So I think now the industry is becoming more mature and now they are understanding these consequences and moving towards a mature AI world altogether.
Allegra Guinan [01:11:54]: I think the more that companies make these things transparent and talk about the bulkly out of their desire to do so, then other companies see that and clients and the audience will say, oh well, this company said this thing, so now I can apply it over here. So that's a really good point. Well, thank you so much to all the learners. I really, really appreciate it. Such a good discussion. I learned a lot. It's really valuable. You all join it as well.
Allegra Guinan [01:12:20]: I want to give a special thanks to Mozilla AI for sponsoring this event and El Pal for hosting us here, and the rest of Mozilla team that I know is around and came from various places. Thank you all for sending your time here after your long work day at the end of the work week. So it's really appreciated. We're gonna have some pizzas and drinks outside the door here as soon as we're done. Also, feel free to stick around. That's when you eat and talk to everybody, and then I'll put you at the next ML you.