State of AI Report 2024 // Nathan Benaich // Agents in Production
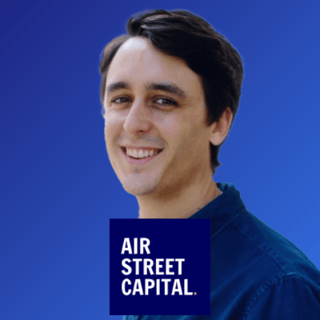
Nathan Benaich is the Founder and General Partner of Air Street Capital, a venture capital firm investing in early-stage AI-first technology and life science companies. The team’s investments include Mapillary (acq. Facebook), Graphcore, Thought Machine, Tractable, and LabGenius. Nathan is Managing Trustee of The RAAIS Foundation, a non-profit with a mission to advance education and open-source research in common good AI. This includes running the annual RAAIS summit and funding fellowships at OpenMined. Nathan is also co-author of the annual State of AI Report. He holds a Ph.D. in cancer biology from the University of Cambridge and a BA from Williams College.
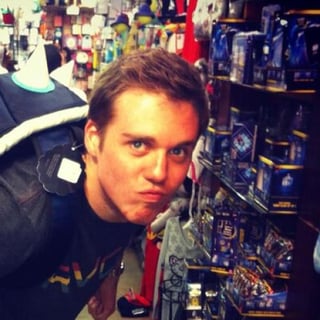
Data is a superpower, and Skylar has been passionate about applying it to solve important problems across society. For several years, Skylar worked on large-scale, personalized search and recommendation at LinkedIn -- leading teams to make step-function improvements in our machine learning systems to help people find the best-fit role. Since then, he shifted my focus to applying machine learning to mental health care to ensure the best access and quality for all. To decompress from his workaholism, Skylar loves lifting weights, writing music, and hanging out at the beach!
The State of AI Report analyses the most interesting developments in AI. We aim to trigger an informed conversation about AI's current state and its implications for the future.
Link to Presentation: State of AI Report
Skylar Payne [00:00:05]: So Nathan's here from Airstreet Capital. If you haven't been living under a rock, you've definitely come across the state of AI report that he has been putting out since 2018, I think.
Nathan Benaich [00:00:17]: Yep.
Skylar Payne [00:00:18]: And yeah, a lot of great information. He's gonna walk us through it, something you don't want to miss, so definitely get ready. Just want to point out for those of you who are on, there is a Q and A tab. So if you have questions as we're going along, feel free to drop them in there and we'll get to them at the end. But with that, I'll let you take it away.
Nathan Benaich [00:00:38]: Sweet. Thanks for having me. It's a pleasure to be here. I'm going to spend a bit of time walking you through an executive summary kind of Editor's cut of the State of AI Report the goal with this study is to focus on a couple of different angles around AI. So we cover AI research, like what are the most important papers and breakthroughs that have happened in the last 12 months. We look at industry trends, politics and safety, and we make a couple of predictions every year as well, and we review them in the subsequent year's report. And the goal we have with this is to try and inform conversation around AI and really, like, what does it mean for the future? Where are things going? It's all freely available online at State of AI and we have a bunch of reviewers who are active participants and contributors in AI who help keep us honest and review the content for accuracy. So the whole report is around 200 and something slides.
Nathan Benaich [00:01:37]: It grows a little bit every year. And I'm just going to present to you a snapshot of my favorite pieces across this report. So we'll start with research. The main story here, I think, over the last 12 months was that OpenAI is clearly number one in terms of producing models with great capabilities. And it seemed like they had this kind of reign of terror that was never ending really. And in the last couple of months we've now seen new systems coming out from Anthropic, from Google and XAI and others at the frontier model scale, and the performance deltas between these different systems seems to be eroding. So perhaps like this reign of terror is ending. And then just a couple of weeks ago they dropped a new model focused really on complex reasoning.
Nathan Benaich [00:02:29]: One of the main critiques that a smaller and smaller group of critics have about AI systems is that they're kind of stochastic parrots. They learn statistics, but they can't really do fundamental reasoning with this new system, dubbed 01, the company really showed that AI systems can pause for a while, really try to understand a problem and go through stepwise reasoning and perform pretty well on math and complex science and coding problems, in fact, even replicating some of the strategies of certain PhD students. And this is down to this idea of inference scaling. The other major discussion in research has been this closed and open argument. Of course, over the summer and towards September, Meta released its bigger family of Llama 3 models and updated them several times over the year. And again, like these systems are performing pretty incredibly for their size and has really spurred a lot of innovation. And forks on hugging face. I think there's about half a billion LAMA derivative models that have been created.
Nathan Benaich [00:03:41]: And one of the other Meta themes that's been really interesting is this idea of models having to become very large first so we can kind of understand their capabilities, how they work, but we can then use them to curate training data to understand how best to optimize training, such that we can produce models of a similar intelligence, but that are smaller. We've seen this happen with Gemini Flash, where one large model was used to help the training of a smaller one through methods like distillation. And Nvidia and others have also been working on this. Pretty much absent a year ago and now really almost at the top of leaderboards has been Chinese AI company models, particularly those of the vision and language flavor in particular, like Deep Seq and Owen, Alibaba and Quinn have constantly been pushing out new systems and these are remarkably open source with a focus on coding, and they challenge some of the bigger vendors in the US and one of the kind of interesting things with this report is to look back over a couple of years and consider how far has progress really come. Because oftentimes I think in this era of like new models and new data sets and capabilities getting published almost every week, we kind of become numb to these sort of progress improvements. And it's really not that long ago in 2018 when we started doing the report that most vision systems would be incapable of producing appropriate question and answering completions for a picture of the baby holding a toothbrush and for example, outputting that it's a young boy holding a baseball bat. And nowadays we have these systems, whether it's a quand or others, that produce pretty amazing vision, language, reasoning capabilities on PDFs, on charts, and it's kind of table stakes. The other beautiful thing with these large models is that they're diffusing across different modalities, not just like Image and text of kind of formats that humans are used to reasoning with.
Nathan Benaich [00:05:52]: But they can go to other domains where different languages exist. And here we show you examples in biology where a company called Profluent has trained large models on protein sequences, amino acid sequences, and use that to basically learn the grammar and the structure of how sequences produce functional proteins. And excitingly, they've used this to develop brand new genome editors which have sequences that are vastly different to naturally occurring genome editors and demonstrated that they're actually fully functional in human cells for the first time. Robotics of course, has gone from in vogue a couple of years ago to totally out of vogue to now back in vogue. Teams have been fired and rehired. And if you were to sample a venture capitalist today for one of the most exciting industries, they would certainly pick foundation models for robotics. And general purpose embodied AI is as one of the themes moving into industry. It's of course no secret that Nvidia is now probably the biggest company in the world, depending on its stock price, which keeps appreciating.
Nathan Benaich [00:07:00]: And it's just been like astonishing to watch the progress of this business. And we have quite a number of slides that go into just how it's done this. We produce this compute index every year where we're effectively tracking the size of compute clusters that are being created and launched either on the government side or private cloud or public cloud. The main difference we see is just kind of an order of magnitude larger systems being built today than about a year ago. Famously, XAI brought on their 100k cluster in about 122 days, which is arguably three to four times faster than industry average. And also companies that are on the smaller side have started to build up GPU capacity. And the first GB200 clusters are starting to go live. And many more will happen next year.
Nathan Benaich [00:07:53]: Like doubling down on this theme of like how dominant is Nvidia? We look at all open source AI literature and count the number of papers that mention the use of a specific chip in there. Research experiments and this graph we show you on a log scale just how many total papers use Nvidia versus every other chip. And so this past year there's been around 35,000 papers that use any Nvidia chip, which is about 11 times more than all Apple Big Six startups, Huawei, FPGAs, Google's TPU and ASICS combined. On the Nvidia side specifically, the most popular chip in AI research is still the A100 which came out a couple of years ago. Last year, the most Popular One was the V100, which is now seven years old. And what's really interesting with this chart is just to show you how much time longevity each of these chips has. So on average, it looks like a chip can be useful for AI research for almost a decade. And on the AI chip startup side, really, like, there's not much activity, I would say, in terms of usage in AI research, the sum of all papers is on the order of about 400 or so.
Nathan Benaich [00:09:10]: And of all of them, Cerebra seems to be upticking more than most. So if you were to make this whole comparison of, you know, should we really be supporting, you know, new startups to try a challenge Nvidia, or should we just invest in Nvidia? We looked at your, the value of your portfolio. If you would have taken all $6 billion that were invested in AI chip challengers since 2016 and how much that would be worth today based on valuations in public markets, and how much that portfolio of 6 billion would have been worth if on the day that the announcement of fundraising for each of those AI chip startups was made, that you just put that money into Nvidia and it looks like as of a month ago, that 6 billion would be worth 31 billion into the challengers, half of which is in a publicly listed Chinese company. But if you put all that money into Nvidia during that time, that 6 billion would be worth 120 billion. There's been a lot of buzz around vertical applications of generative AI for lots of different use cases. You know, many companies have raised huge rounds, whether it's for sort of custom chat bots like with character AI or image generators or Stability or Black Forest Labs or more enterprise products like Cohere and Perplexity. Valuations still look quite spicy, but, you know, it's still very early days for monetization. Still some questions around margins, but I think a lot of these discussions are starting to get worked out as there's a ton more work that's going into efficiency of inference and training.
Nathan Benaich [00:10:52]: But at the end of the day, perhaps it's really, you know, just an industry that's dominated by vibes, just like public market seems to be. The most obvious example of this would be the turnaround for Meta, which, you know, basically like lost over half a trillion dollars of market cap in its foray in Metaverse investing. I want to turn that around in early 2023 with a focus on AI instead of Metaverse. It added, by today's count, probably closer to $1.3 trillion of market cap and to the point of making these systems cheaper. It's been astonishing to see the cost differential just a year ago to what it is today for systems of equivalent intelligence. And we see drops of one or two orders of magnitude across providers, which is going to be a mix of improvements on the technical side and cloud hosting side, but also good old fashioned price wars. These companies like OpenAI, Anthropic, Vercel have started to launch very popular coding experiences. One of the ones over the summer was this kind of interactive developer Sidekick UI where a developer could ask a bot to make certain programs and it would launch basically part of the screen which would start to actively create what they asked for.
Nathan Benaich [00:12:14]: But not everybody is super happy in this industry. There's been a huge backlash from content owners around copyright, particularly for image and audio, with a slew of lawsuits actually that were filed earlier this year, and several examples of leaked Google sheets and other databases with pretty explicit call outs for scraping content that actually needs a license. But it's probably going to take a bunch of time to see these cases run through the court system. And in the meantime, these companies will become pretty large. We also look at a couple of examples of themes that for many years investors in the market would say like, you know, lots of money going in, lots of money going in, nothing is delivering, everything is delayed. And the prime example here would be self driving. But for anybody who spent time in San Francisco, Louisiana or Phoenix and had the opportunity to try Waymo, I think it's probably one of the most magical consumer experiences you can have. And it looks like the product is pretty ready.
Nathan Benaich [00:13:21]: Today we look a little bit deeper into just how consumers and enterprises are engaging with AI first products. Here we show you some data from ramp, which is basically a credit card company for enterprises. And so they can track the transactions that companies are making with the card. And so they look at a basket of AI products and the users of those products in 2022 and 2023, and just how many customers are still buying those products that they did at the start of the year compared to the end. And therefore we see retention grow from 41% to 63% year on year, which is pretty exciting. And companies are spending more money on AI products than they did just a few quarters ago. Actually, it's growing 3x. And the other remarkable effect here is just how quickly companies that are AI first with generative products are growing their revenues.
Nathan Benaich [00:14:19]: This graph on the right I think is particularly striking. Where Stripe looked at the 100 most promising SaaS companies and the 100 most promising AI companies and showed that actually AI companies took just a little over a year and a half to get to over $30 million in annual revenue compared to their SaaS peers which took almost five years. Some killer apps have really started to emerge, particularly text to speech and speech recognition where I think we've clearly crossed the uncanny valley and have this amazing capability to do multilingual lifelike speech in lots of different sort of styles and Personas. Over on the biotech side we saw one of the biggest transactions come together with recursion and exientia, one being a powerhouse on biology based drug discovery and the other one on chemistry come together in an M and A that's worth almost $700 million. That creates a very compelling clinical pipeline and and a business that's combined has the largest GPU cluster in biopharma. Video generation has been insanely hot as more companies are pushing the envelope of long form coherent physics consistent video generation and we think there's going to be a lot more innovation here coming out soon. On the consumer side there's been some have and have nots with regards to new hardware products that have AI embedded in them and trying to reinvent the kind of user interface towards AI in the environment. On the one side, Meta's Ray Bans have been a smash success, perhaps more to the form factor and the embedded audio than the AI for now.
Nathan Benaich [00:16:06]: And on the other hand the rabbit R1 and humane pin have just not managed to find many believers. Investment dollars keeps really flowing into the space. It's been enormous funding rounds of billions of dollars into generative AI and largely if you were to pull out all the AI financing from private markets, I think the market would be generally flat or down and same would probably go for Publix. Huge rounds tend to be the dominant sort of paradigm in the last two, three years, particularly in the post GPT4 era. And public companies just continue to rip. I think these are almost like $10 trillion in value that put together for companies that are based on AI and Publix. Meanwhile IPOs remain pretty frosty and same goes for M and A. By the end of the day perhaps, you know, attention is was all you need to start a company and you know, we made this slide a couple of years ago showing all the authors from the original paper that have gone on to form startups that have been, you know, more or less successful large businesses.
Nathan Benaich [00:17:11]: And today it looks like many of those businesses are starting to find exit routes through increasingly creative Corporate structuring, particularly for inflection and character and others where the team ends up being rehired and models are licensed by the buyer and the shell company remains which has to continue on a go forward basis. Over in politics of course like the last like 10 days or so has seen some major changes so all this is probably up for being rewritten. But the US introduced quite limited frontier model rules via an executive order which is really opt in from major companies on the large model side. And in the absence of federal legislation which is still going to be a bit TBD, states are pursuing their own. For example SB 1047 was overturned in California, Milan, Colorado. They've implemented their own, their own regulations in Europe it's been really tricky for US AI labs to push forward their products. As an example, Claude was not available in the EU until May this year. Apple had issues and still has issues rolling out Apple intelligence and Meta also limited the ability for consumers in WhatsApp and Instagram and Facebook to get access to LLAMA 3.
Nathan Benaich [00:18:36]: Governments are also not very happy also particularly in Europe around usage of citizens data on social networks for AI training. X had real issues with GDPR complaints in Europe after using models after using consumer data on models. Meanwhile consumers have the ability to opt out of getting their Facebook data used for meta model training as well. And you know just a few years ago big tech companies had a great commitment towards net zero in 2020, sorry in 2030 Microsoft even said that it would be carbon negative. But in the backdrop of this voracious demand for build out of GPU computing clusters, it looks like all these net zero commitments are just not going to not going to be anywhere close to being passed. For example, Amazon's done deals with nuclear companies. Microsoft has done a deal with Constellation Energy to revive one of the Three Mile island nuclear power plants. And we expect more of these sorts of deals to happen over in safety.
Nathan Benaich [00:19:45]: I think this has probably been one of the biggest vibe shifts that has happened in the last year. 2023 the narrative was AI can be extremely dangerous. And we saw many of the top executives testify in front of nation states in the U.S. and in Europe to that end asking for regulation to come into play. And fast forward to today, it seems like it's all systems go where our companies are trying to continue to raise huge amounts of money, get consumers to use their apps and enterprises to pay for them. And you know, model builders and red teamers are now competing against each other, trying to break each other's systems and defend against each other's. Hacks and for now, Red Teamers have managed to defeat all the jailbreaking safeguards from big labs. It's a bit of a whack a mole situation, but at the end of the day it looks like so far the kinds of harms that are really presented by big models tend to be much more prosaic.
Nathan Benaich [00:20:50]: For example, you know, Arup and some other big customers lost money over deep fakes, but it's not like the issue of AI taking over their systems and having a volition of itself. On the prediction side, we got a couple of them right from last year, for example, that a Hollywood great production would make use of generative AI, which has been the case in Netflix and HBO Productions that a regulatory agency like the CMA or the FTC would investigate Microsoft and OpenAI, which certainly happened, and that generated song from AI would actually make its way into a billboard. And this happened in Germany. We actually predicted that financial institutions would launch GPU debt funds, and at the time of writing this report it had not happened. But about a week or two ago, Wall street announced about 11 or 13 billion dollars worth of debt financing for building out GPUs. So what do we think is going to happen in the next year? We made 10 predictions. I'll just highlight some fun ones, like an AI research paper generated by an AI scientist will be accepted at a major machine learning conference that some developer is going to use generative tools to write an app or a website without having very much coding ability, and it'll go viral. And my third interesting one is that as big labs start to have to raise tens of billions of dollars from sovereign states, we'll get into a position where we tend to take some serious national security review to determine whether this is a state of play that states will accept for such a critical technology.
Nathan Benaich [00:22:39]: With that, just my editor's cut of this year's narrative. There's 200 or so more slides to go, so I invite you to go to State of AI to have a read. Thank you for your attention and thanks for those of you whose work we profiled in the report, so it's been a pleasure to share it with more folks.
Skylar Payne [00:22:58]: Awesome. Thank you so much. We got some questions in the chat. I'm going to go through and flip through them. So somebody asked bottles keep getting cheaper, but at some point that has to stop. Do you see a world where we pay.000002 cents per token or where's the realistic limit in your eyes?
Nathan Benaich [00:23:21]: I think it depends on the value of the, of the, of the task at hand. So there's going to be, you know, some work which is super cheap and a model is going to have a hard time comp. Competing against human labor. But there's lots of human labor that's incredibly expensive that, you know, even a model that costs $10 or $5 per million token is probably more efficient at. So my sense is going to be much more like a task based segmentation. Like you're gonna, you're gonna either hire a model alone or a model plus a human to solve a task and do the kind of ROI yourself.
Skylar Payne [00:23:57]: Awesome. Totally makes sense. So given your position as both an AI investor and somebody who's putting out this report year after year, you have a pretty broad view of a lot of what's happening in AI. Are there any areas you feel like are not getting enough attention or deserve more investigation?
Nathan Benaich [00:24:16]: I still think we have so much more to go in AI for science. It's been like, it's very encouraging to see that a week before the report, both basically deep learning and protein folding won the Nobel Prize. So I hope that vindicates a lot of researchers efforts to evangelize those two areas. And I think biotech is going through a pretty tough time at the moment and so is just science progress in general, whether it's materials or energy or whatnot. Um, and just like AI has such a huge role to play there. In fact, there was even some work from MIT yesterday or two days ago that looked at the productivity of a major American R and D firm where they introduced AI tools, particularly a graph neural network that can help predict material properties and looked at the efficiency of workers. There's a thousand workers, they either had to tool or didn't. And you see material uplift and like new materials getting discovered, better quality, more patents filed, more productivity.
Nathan Benaich [00:25:19]: So I think it's still, it's still super early days. I think we should be like accelerating far faster on the science side or AI for science side. Yeah.
Skylar Payne [00:25:31]: Is there maybe time for one more question? Oh, actually there's a couple more in this chat. What's the best use of an LLM you have seen so far? Outside of obvious things like ChatGPT.
Nathan Benaich [00:25:48]: Question, I think, I think the best one is probably as a coach, like just, there's, there's just some stuff that you want to talk to somebody about and it just looks like actually these agents are pretty good therapists and like thought partners.
Skylar Payne [00:26:08]: Yeah, yeah. It's interesting you say that, especially with voice mode.
Nathan Benaich [00:26:12]: I think voice mode is, like, the killer.
Skylar Payne [00:26:15]: Yeah, definitely. Yeah. Most of my background has been applying AI to mental health, and one of the things we often saw in transcripts from therapists was, like, a lot of the time is not really spent on therapy, it's spent on companionship. So it's not surprising to me that coaching can be done by AI. Awesome. Well, I think we're at time here, but thank you so much for your time. This was amazing. And, yeah, everybody go to the airstreet website and you can find this year's State of AI report and also go and look at all the past.
Skylar Payne [00:26:48]: But, yeah, thank you so much for being here.
Nathan Benaich [00:26:50]: Thanks for having me.
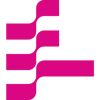