Streaming Ecosystem Complexities and Cost Management
speakers
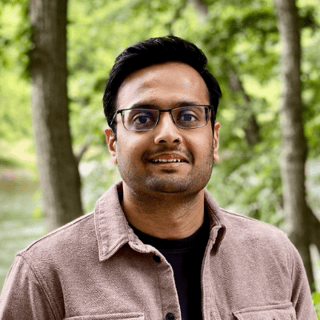
Rohit Agrawal is an Engineering Manager at Tecton, leading the Real-Time Execution team. Before Tecton, Rohit was the a Lead Software Engineer at Salesforce, where he focused on transaction processign and storage in OLTP relational databases. He holds a Master’s Degree in Computer Systems from Carnegie Mellon University and a Bachelor’s Degree in Electrical Engineering from the Biria Institute of Technology and Science in Pilani, India.
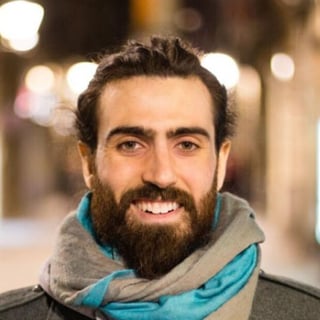
At the moment Demetrios is immersing himself in Machine Learning by interviewing experts from around the world in the weekly MLOps.community meetups. Demetrios is constantly learning and engaging in new activities to get uncomfortable and learn from his mistakes. He tries to bring creativity into every aspect of his life, whether that be analyzing the best paths forward, overcoming obstacles, or building lego houses with his daughter.
SUMMARY
Demetrios talks with Rohit Agrawal, Director of Engineering at Tecton, about the challenges and future of streaming data in ML. Rohit shares his path at Tecton and insights on managing real-time and batch systems. They cover tool fragmentation (Kafka, Flink, etc.), infrastructure costs, managed services, and trends like using S3 for storage and Iceberg as the GitHub for data. The episode wraps with thoughts on BYOC solutions and evolving data architectures.
TRANSCRIPT
Rohit Agrawal [00:00:01]: So my name is Rohit, I work at Tecton. My title is Director of Engineering. I essentially just lead a few engineering teams and for coffee, I'm a huge fan of Cometeer. Actually I like my coffee, like basically how easy and simple I can make it. So I started using Cometeer back in Covid when like all the cafes had shut down and I became a user then and then I've just since then, like I think it's one of those Covid things that are just stuck probably maybe the only thing. And I still drink comedier essentially.
Demetrios [00:00:34]: People of Earth, today we get into it with my man Rohit all around streaming data. This real time, low latency use case that can be associated with traditional ML. Now I want to tell you a quick story before we get into it, because over the holidays about two months ago, I asked everyone in the community what they wanted to see for the New year and many of you said, I want to see some traditional ML stuff. It feels like we've been talking about the AI and LLM booms too much. So this episode right here is for your viewing pleasure and if you are listening on podcast stations around the world, we've got a recommended song, recommended listening, which comes from a fan, Amy or Ami, because I've been asking folks what their favorite music is when they join the community in this one is a gem that I did not know about. It's Gibran Al Kosr and the song is called Idea 10 Test in production. That's how we're running with this. Basically this conversation came about because I was on a call with you and Kevin, the co founder of Tecton, and you guys were explaining to people all about streaming and the situation that the data streaming and streaming ecosystem looked like.
Demetrios [00:02:55]: And I thought, man, that is such a cool topic for a podcast. I would love to talk to you more and dive into it. So we should probably just start with like what is your day to day? You're working at Tecton? What are you doing there?
Rohit Agrawal [00:03:13]: Yeah, absolutely. So I joined Tecton about like four years ago. So I joined as an ic. We were pretty small back in the day, so I worked on pretty much everything, but I focused mostly on the real time pieces. So for example streaming as you mentioned, and a lot of challenges around that. My role now has changed a little bit. So I am more in like a managerial role right now. So I manage three different teams that focus on like streaming data, batch data, online inference, offline inference, et cetera.
Rohit Agrawal [00:03:41]: So kind of like the core data flows and infrastructure that Tecton provides as part of its core product. So in short, my day is a lot of meetings.
Demetrios [00:03:50]: But yeah, we should probably state for everyone because we can't take it for granted that everyone knows what Tecton does.
Rohit Agrawal [00:04:00]: Yeah, absolutely.
Demetrios [00:04:01]: They're one of the original OG's feature platforms. So a lot of dealing with your features, transforming your data, making sure that you can do use cases like fraud detection, recommender systems, loan scoring. I mean, are there other stuff that you see people using Tecton a ton.
Rohit Agrawal [00:04:22]: For also for like insurance claim processing is also like a big use case for us. Yeah, recommenders are like in many different shapes and form and then broadly fraud and risk applications, I would say is the biggest like use case for us.
Demetrios [00:04:36]: Yeah, yeah, that those use cases that you just need really fast responses.
Rohit Agrawal [00:04:41]: Correct.
Demetrios [00:04:41]: Yeah, yeah. So that's why we wanted to talk about streaming, man. That leads us right into the whole streaming ecosystem, like break down. What is it? What does it look like right now? What are some things that have changed since I was on that call with you, like a year and a half ago? Like I can't even remember.
Rohit Agrawal [00:05:00]: Yep, yeah, absolutely. So I think like when we look at customers, most of them have, you know, invested in like a streaming solution which can like stream data at low latency. So for example, this is like Kafka, Kinesis, et cetera, but that's kind of like a data delivery mechanism, but they kind of fail when it comes to using that data effectively for applications. So a lot of app companies we talk to have data in these Kafka streams, Kinesis streams, but then they fail in making machine learning models or even analytical use cases use this data effectively.
Demetrios [00:05:42]: Wait, why is that?
Rohit Agrawal [00:05:45]: Yeah, I mean, I'll go into it. So I think fundamentally the ecosystem is extremely fragmented in terms of several different tools and technologies. And so you have to string together a bunch of these things, hire experts for each of these things, and then once you can even do these things, it's not a one time cost to actually build it out. But these systems need resiliency and reliability. And so it's like an ongoing effort to basically maintain these systems. So the simplest solution we see is people have like a Kafka stream and they would have a stream processor which can either be like a Spark or a Flink. And then they basically connect that to then storage, like could be key value storage, could be offline storage like Iceberg, and then build a serving layer on top of it for applications to consume this data. And each of these different steps actually requires like different skill sets.
Rohit Agrawal [00:06:34]: So building a team to actually do all of these things is very difficult. And the tools inherently that are available from the ecosystem are also like fairly difficult to actually use, especially if you're trying to go from 0 to 1. I think if you have a very large team and you're running flink at, let's say, you know, Facebook or meta scale, you know, it's maybe somewhat manageable. But for like smaller companies and teams that just want to still leverage the real time data, the ecosystem doesn't really have like these simpler tools available for people to use.
Demetrios [00:07:09]: Yeah. And I know that DynamoDB plays a huge part in this. Where does it plug in? Is it on that serving layer?
Rohit Agrawal [00:07:17]: Yeah. So Dynamo is basically storing records coming in from a stream processor and then making it available for high throughput, low latency serving. So essentially something needs to write to Dynamo and something needs to read from Dynamo. And that writer and reader is something that each company kind of builds it their own way. And even maintaining that is a challenge because you need to think through what is the schema which favors read latency versus write latency and which one is more important to you and how do you evolve that over time. And all these are challenges that people who are building all these pipelines have to think through very deeply when they are building these systems.
Demetrios [00:07:56]: Yeah. Because you are trying to just keep things super fast, as we said, and make sure that your use cases like the loan scoring or the fraud detection or recommender system, whatever, is just blazing fast. And so you need to think through wherever you can shave off milliseconds.
Rohit Agrawal [00:08:15]: Yeah. And there's this also additional dimension of cost because I always think of these are the two sides of the same coin, which is how fresh do you want your data and how much are you willing to pay for it? Right. And ideally they should be in sync with each other. Meaning that if I want data at millisecond level granularity, I'm willing to pay more money for it. And if I am okay with an hour worth of freshness and I'm willing to pay less for it. Right. And you ideally want a system that has the right levels of knobs built in which can give you this trade off and you can actually play with it. For a lot of systems that we see in the real world, this is not present, meaning that they either have a system that's built for very low latency and there's a very high cost of the system, or it's the complete on the other side where the cost is Relatively low, but the system cannot afford freshness that's pretty low that's required by applications.
Rohit Agrawal [00:09:17]: And most companies have a very varied set of use cases. Some need very low latency, some are okay with slightly higher latencies, et cetera. And so I think having a system that can manage the cost for that freshness is also very important as opposed to building it. The simplest analogy is people are not just looking for a Ferrari because it's fast, but it's also very expensive. And then what people really want is that they want a system that's fast on some days when they need it to be, but can also act as a Ford SUV when you want to go to the grocery store and buy some Wilkin eggs.
Demetrios [00:09:57]: Yeah, if you're with your family. And that makes total sense because you have different use cases that have different needs and different fresh and different variability of how much you're willing to pay for that. So for each instance of these, you want to be able to configure it to those different constraints that you have.
Rohit Agrawal [00:10:20]: Yep, absolutely.
Demetrios [00:10:22]: And the other thing that I was thinking about, because you were talking about how folks are using Flink or Spark, I think I remember DuckDB being plugged in here in some ways. Can you talk to me about that?
Rohit Agrawal [00:10:38]: Yeah. So on the batch side of things, we do provide a solution called rift, which uses DuckDB as an alternative for some of the batch pipelines.
Demetrios [00:10:49]: Okay.
Rohit Agrawal [00:10:50]: So that's kind of like the equivalent of like Spark batch. Right. But on the streaming side of it, we, we don't use it DB There. We have our own like stream engine there essentially that we use.
Demetrios [00:11:00]: Yeah, that makes sense. Okay, so basically that is the scene you have set is that there's a bunch of disparate pieces to the puzzle. Mostly everyone builds one size for all of their use cases in their organizations, and they don't think about how to service each individual use case through. And then you've got reliability or maintenance of these pipelines and of these platforms that come into play. How do you see teams dealing with that maintenance?
Rohit Agrawal [00:11:43]: Yeah, I mean, honestly, it's like very challenging because a lot of the times the teams that are building these are sometimes like machine learning engineers or people who are more focused on like the data layer of it. But the production experience of maintaining the system typically falls to a different team. And this silo between the teams often is a huge challenge for a lot of our customers that we see. And on top of that, users care about end to end reliability as opposed to whether my Flink went down or my worker went down, et cetera. And they were looking for solutions that can offer end to end reliability across the entire pipeline. And that's something that's just very, very difficult to build because a lot of the on call production team thinks of like one service in the individuality of it and a lot of the machine learning teams care about like the end to end reliability of the entire pipeline. And this mismatch often is like a huge concern for a lot of our like customers that we see.
Demetrios [00:12:41]: So wait, this means basically the SRE who's getting pinged at 3am is getting pinged for one particular piece. Like the flink went down.
Rohit Agrawal [00:12:50]: Exactly.
Demetrios [00:12:52]: The machine learning engineer is thinking about well I've got Kafka and I've got my Flink and how are they looking at it? That's not just like that one service went down.
Rohit Agrawal [00:13:02]: Correct. Like for example if let's say like your flink went down or like your spark structured streaming engine went down, then probably now messages are being backed up in like Kafka. And now the machine learning applications are seeing like high freshness basically like they're not processing any more real time data. And so the repercussions of each of these are like so far and beyond that a lot of the if you're building a solution that requires stringing together a bunch of these disparate tools, then it's like a never ending game of like trying to build end to end reliability because it's just very difficult to do that for like individual components.
Demetrios [00:13:38]: And then you start to see that's where you can make the case for a lot of money being burnt on fire.
Rohit Agrawal [00:13:47]: Yeah, I mean we constantly come across customers who are spending like you know, tens of thousands, if not hundreds of thousands on like just simple like streaming pipelines. And I'm just talking about the infrastructure cost of it. There is the other cost of it which is like the SRE cost of it and the operational cost of it on a day to day basis. But just like pure AWS infra bill for all of these pipelines is like pretty high and like optimizing it is very, very difficult given their stack.
Demetrios [00:14:15]: Have you thought about different best practices on how to optimize that besides like okay, just plug in Tecton here. Right. I imagine that you have seen teams that are doing this well and what does that look like?
Rohit Agrawal [00:14:29]: Yeah, I think like to be honest a lot of like newer age technologies that are coming in are actually aimed at bringing down like the operational and one time cost of setting these things up. I do think it requires a departure from just like a mental thinking model of like I want to use what Google and Facebook are using, which typically could be flink, et cetera. And I think it requires you to be open to some of the newer technologies that are coming in. So I'll give you one example where we're seeing a lot of like streaming solutions not use like local disks attached to nodes, but use S3 as like a storage service and stream all that data through S3 essentially and kind of build on object storage. So one good example is like Warp Stream for example, which kind of is like a Kafka on S3. Basically they got recently acquired by Confluent and we're seeing a lot of these newer age real time operational systems that are truly building for the cloud and leveraging object storage. And I think that's a huge way that huge lever that customers can pull and see like where are we using cloud resources effectively in our stack or not?
Demetrios [00:15:40]: And is that just because it's much cheaper to do it through S3?
Rohit Agrawal [00:15:45]: It is cheaper, but also like the, it gives you elasticity. So I think like the biggest way you can think of it is you can start to scale, compute and storage independently as opposed to, for example, if you are using like disks that are attached to your server, then every time you add a new server, you're also adding more disks to it. And so it's very difficult to decouple the two. Whereas some applications may require more compute and in those cases you're still ending up paying for more storage. And I think the cloud gives you this elasticity of being able to scale different layers independently and it leads to not only reduced cost, but also just reduced operational overhead.
Demetrios [00:16:24]: Yeah, that is cool. What other tricks? Because I hadn't heard about these guys that just got acquired from Consulate. What was their name?
Rohit Agrawal [00:16:32]: Warp Stream.
Demetrios [00:16:33]: Warp Stream, yeah. Fascinating.
Rohit Agrawal [00:16:36]: Yeah, I think like there are other like in other interesting tricks that like we see some of our customers use. For example, like a big cost of like streaming workload is typically checkpointing, which is like how frequently are you writing to storage in case your streaming application, in case your streaming pipeline fails, you can recover from the latest checkpoint. And typically the more you are checkpointing, the more you are paying for this checkpointing cost. But the benefit you can get is that if you, let's say went down, you can pick back up from the most recent checkpoint, which maybe just be a few seconds ago. Right. Because you're checkpointing so frequently. And I think for A lot of our customers, the default checkpointing settings for a lot of these streaming applications is just, like, very, very high. And they're often surprised to see just, like, the cost when the bill comes up.
Rohit Agrawal [00:17:25]: And for a lot of our customers, like, we can just look at, like, it's just like one of those things where when most customers ask us, like, why is my streaming bill so high for my existing stack, like, the first place we would look is, like, how frequently are you checkpointing to disk, et cetera? And I think, like, a lot of our customers are just surprised by, like, okay, I didn't even know about this.
Demetrios [00:17:48]: And what is it that the majority of the time you don't need to be checkpointing as much.
Rohit Agrawal [00:17:53]: So I think, like, it's. I think checkpointing is usually, like, very important and it should be done. It's a question of, like, do you want to do it every second, every 10 seconds, every 30 seconds, every minute, every hour? And just the default settings for a lot of the streaming workloads is, like, very aggressive checkpointing. And even if you can tune it from the one second to, let's say, 10 seconds, and if your application can allow that level of, like, recovery point objective, then you can save, like, pretty significant costs for a lot of our users.
Demetrios [00:18:24]: Yeah, that feels like a huge difference from 1 second to 10 seconds. Yeah.
Rohit Agrawal [00:18:29]: I mean, as I said, like, it's a lever of, like, how much cost are you willing to pay for how much freshness and these knobs are present in some systems? But you need to know of, like, what these knobs are and how to tune it effectively. Otherwise you will. You might just end up spending a lot of cost for workloads where you don't require that level of, like, freshness.
Demetrios [00:18:47]: Well, it goes back to your whole thing at the beginning where you were saying when you have to patch together so many disparate tools, you have to have people that understand and are experts in all these tools so that they know, oh, it's possible for us to just checkpoint every 10 seconds. Yeah.
Rohit Agrawal [00:19:06]: And they also need to reason about the end to end pipeline and understand what this one thing will lead to the end to end pipeline, because most application teams are concerned about that as opposed to, like, this other objective, for example, and it's just like a very hard problem to solve.
Demetrios [00:19:20]: I know you talked about the way that you look at the shape of the data as being a lever that you can pull. And so it is. This picture that you're painting in my mind is just like, there's so many stakeholders involved and that just makes it way more complex because at any time you have to throw more bodies at a problem, it's going to increase the complexity of the solution in this case. But then you've got like, like I imagine the data scientist isn't thinking about the schema as much for how to make it just fully optimized for speed.
Rohit Agrawal [00:20:05]: Yep, exactly. Like I'll give you, I'll give one example where we came across a customer who was like storing protobuf messages inside the message stream. Right. And well, it made sense because the team that was like putting data inside the stream was dealing with protobufs and they were like doing it that way. However, like on the consumer end we were having to deserialize all these protobufs into messages that we can like parse and ingest in our like ingest in the storage and serving solution. And when you spoke to the data scientists, like they just did not want to reason about why do you want to store Protobus in the first place? And it was just like an abstraction level of thinking which they're not used to. They are used to operating a little bit like higher level of abstraction, which completely makes sense. But they are either forced to reason about much lower levels of abstraction or they just have to deal with the performance hits that they are getting.
Rohit Agrawal [00:21:01]: And it just seems like they have to pay a tax one way or the other with a lot of the existing systems of bse.
Demetrios [00:21:08]: Yeah, what a great point. Because they're just going to do whatever's the easiest. It's like water flows downstream and is trying to minimize the friction that it encounters. And so if that is the only solution that they know works and they're probably going to take that upon them. And it makes me think also about how important it is on the organizational side for these different stakeholders to be talking to each other and to understand each other's goals and know that. All right, the data engineer is setting this up for me. So what, like talk to me more about what the data engineer can do. Right.
Demetrios [00:21:50]: Like if I'm a data scientist, I am diving into data engineering, which probably isn't my strong suit, but it's going to help me understand what these folks are able, capable of doing or it's going to help me understand what levers these folks have to pull.
Rohit Agrawal [00:22:12]: Yep, exactly. And like for example, we see cases where there are some people who don't care about what is inside the stream as long as they treat each of those messages as like a black box, basically. And their job is just to make sure that these black boxes are flowing through the system and there is some other team that like cares about what's inside the black box and what, how that affects like the end to end system. And we see like this again, again, again about like how it's kind of like, this is not my problem, it's your problem. It's not my problem, it's your problem. But at the end of the day, it's like hurting the application that's actually needing this data.
Demetrios [00:22:46]: Yeah. And that's not even dimension, like going upstream where the data is created.
Rohit Agrawal [00:22:50]: Yep, exactly.
Demetrios [00:22:51]: Trying to figure out like how if things change way upstream, then it, it's a domino effect all the way down.
Rohit Agarwal [00:22:58]: Correct. Yeah. And we see that, we see that when we'll see the application team basically saying that, hey, we are seeing events that are not fresh. We were used to seeing events about a second ago and now we're seeing events that are like an hour ago. And debugging that just takes so much effort sometimes for a lot of our customers, which is that, Is it the application that's processing the screen that's having delays or is it the application that's putting messages inside the screen that's having delays? Is it the consumer application that's having delays? And so it's kind of like this, you know, highway where like, even if like one place is like stuck, it can affect like backstream the entire thing. And figuring where the exact problem is can often be challenging.
Demetrios [00:23:41]: Oof. Yeah. And you have to go and then be this detective in a way. And the more tools that you're using, the more headaches.
Rohit Agrawal [00:23:52]: Yeah, it's just, it just gets, the problem just gets like exponentially difficult. Because, because you now need to check like all the different tool interactions. And now suddenly if you're using two tools or four tools, it's now like eight or 16 different interaction points you need to check.
Demetrios [00:24:06]: I feel for all those folks out there that are dealing with this on a day to day basis because it sounds painful. What else you got for me? What other tips and tricks and just like streaming ecosystem stuff. I mean, I love the fact that I am very green in this field and you live in it. And so just like simple stuff that you're telling me is blowing my mind.
Rohit Agrawal [00:24:37]: Yeah. Like another thing that we see is like how much data, how much data retention is there in your stream. Like for example, streams are meant to typically contain like recent data, but recent itself is like subjective. Like you might keep a day's worth of data in your stream, or you might keep like seven days worth of data in your stream, or you might keep like 30 days worth of data in your stream, etc. And you can just keep extending that. And again, like, you need to think effectively about like how much data you want. So let me back up a little bit. So a lot of our customers have to like stitch together streaming as well as batch data because what they want insights on is like just, they want insights on what happened in the last five minutes, which may be present just by looking at the stream data, but they also want to know insights about what happened in the last like seven days or ten days.
Rohit Agrawal [00:25:26]: Right. Which may include looking at, okay, I'll look at the stream for the last like maybe a day and then I look at my batch data because my batch has data for like the last six days. Right. And so I think one effective thing is like deciding what's the cutoff point between what's stored in batch and what's stored in stream. Right. And so one way to lower cost could be that like I'm only going to keep the last two days worth of data in stream and then anything beyond that up until history is all going to be in my batch data. Or you could say that I'm not going to deal with the batch data and my application just needs statistics up to 30 days and I'm going to keep all that within the stream itself. And each of these decisions that you make affect cost in pretty substantial amount of ways.
Rohit Agrawal [00:26:11]: For example, the one easy way we help a lot of our customers is if you had a system that can effectively handle batch and streaming data and make it available to the application, where the application doesn't care about whether it's coming from batch or stream, then you can really start to arbitrage based on what you want in the stream and what you want in the batch. And you can start putting lesser and lesser data in your stream, like only the recent relevant data, and push more stuff to batch. But it requires you to have like a very advanced, I would say like data engineering pipeline which can really being able to combine this batch and stream data coming in effectively. So for example, we can operate with like even you know, a few hours of data in the stream as long as data beyond that is coming from the batch, which can, you know, really reduce cost for users if they are used to storing like 30 days, 7 days, 10 days worth of data in the stream.
Demetrios [00:27:09]: Dude. So do you see folks creating two different systems for the batch and the streaming?
Rohit Agrawal [00:27:19]: Yeah, so it's actually pretty. So interesting. So what we see is that if, let's say a machine learning engineer or data scientist said, hey, I need like five data points. One is the recent transaction history for the last five minutes, one is for the last hour, one is for the last 10 days, and one is like a lifetime sum of it, essentially, like the total. Right. The first two are more recent and the engineers would build a streaming pipeline for that, and then the other two are more historical, like seven day or lifetime. And they would build a separate batch pipeline for that.
Demetrios [00:27:52]: Right.
Rohit Agrawal [00:27:53]: And then these completely are like disparate pipelines. And the data scientists would have to write transformations, et cetera, separately for each of these pipelines. And then they would then use that in the machine learning model and separately train and serve based on that. Having these separate pipelines is often a huge challenge for customers in terms of duplication of efforts, infrastructure cost, as well as operational maintenance of each of these pipelines.
Demetrios [00:28:25]: There we go with that. Operational maintenance. Again, that is something not to be underestimated.
Rohit Agrawal [00:28:30]: Yeah. I mean, because the cost doesn't just stop at the day you launch this in production. That's kind of like the beginning of it, actually, where most people actually feel if they spend, let's say, three to six months and you put this in production, your work is done. But in reality, that's kind of the starting of the work. And then beyond that, to actually run this in production is actually the hardest part.
Demetrios [00:28:51]: Yeah, that's where you get those bills coming in and all of a sudden you're like, what the hell are we spending all this money on?
Rohit Agrawal [00:28:56]: Yes, exactly. The other thing that we're also seeing is a lot of the streaming ecosystem players are also maturing quite a bit and they're offering more and more services within them. Like a good example of this is if you today had a pipeline that was taking stream data and dumping it into S3 or iceberg, et cetera. Actually Confluent is offering managed services for that. So you can just have stream and just say, hey, dump it to Iceberg. And they have this new service called table flow. And so we're seeing that a lot of this fragmentation in the industry, where maybe there was some vendor for just producing stream messages and serving them out, there was another vendor for putting them in object storage, maybe there was another vendor for putting them in online storage. We're seeing some consolidation within that, which is effectively raising again, the abstraction on which you need to think of.
Rohit Agrawal [00:29:53]: So, for example, as I mentioned, confluent is offering now a fully managed service to basically take your stream and pump it into iceberg and you don't have to think about how data is flowing from your stream to iceberg and what would happen there, et cetera. I do think these managed services are expensive when you think about the cost, the one time cost of it. But if you truly are able to factor in the operational cost of it, I do think these services make sense. And we're increasingly seeing this across like for example, even within databricks, even within Confluent and even like Snowflake for example is offering some of these services. So for example, if you have a Kafka stream and if you want the records dumped into a snowflake table, they're offering like managed services for that. And then obviously they have the entire store of snowflake stacked beyond that. So we are seeing like effectively a lot of these vendors provide more end to end solutions and take on the complexity of stringing these tools together. And I do think if you're like a service, if you're operating a service, if you're operating a company where you are dealing with a lot of these disparate tools, then using some of these managed services by different vendors could be quite useful for you.
Demetrios [00:31:05]: We see that all the time in the MLOps community, Slack. So many folks will come through and ask the questions and it's, it's almost like, yeah, and, and the question is phrased in some shape or form of hey, I'm using XYZ managed service, be it Sagemaker, be it databricks, be it whatever. But it's so expensive. And I'm thinking about what we can do to make it cheaper. And inevitably someone will chime in on the thread and say, okay, you can use this open source alternative or you can try and roll your own in this way shape or form, but don't forget the cost of the humans that are going to be maintaining that. And so you think it's cheaper. Maybe on paper your cloud costs go down, but then your manager or your manager's manager is looking at it and saying we just spent less on the cloud costs, but we're spending way more in headcount.
Rohit Agrawal [00:32:05]: Yeah, and that's truly one thing that we see. The other thing we see is that a lot of companies want to focus on product velocity. Right? And so for example, if, like even if you set all this thing up, but you are anticipating that like I need to build, you know, three times or four times as many features and models, et cetera, and Launch them in production. Is this going to be a bottleneck in terms of me launching these new models and, like, pipelines and products, which is really a problem because then it delays your ability to go to market with these products quickly. And so for. We see this across the industry as well, where sometimes people just come to us because they feel like, hey, I know this. I can run this on my own, I can even hire people, and I can do all this stuff. But as a business, I just don't want to focus on things about these things, and I just want to focus on product velocity and being able to launch the best products out there to the end consumers and not have to worry about whether this product will get delayed because some other team will not staff this on their roadmap and will require more resources to maintain these things.
Demetrios [00:33:13]: Yeah. I saw a blog post the other day from Fly IO talking about how they created GPU offerings because they're, you know, like this cloud. And they basically said we were wrong about the whole GPU offerings and it was a lot of hard work, and we aren't going to continue with it. They're not discontinuing it, but they're not really advancing forward. And there was this nugget in that blog post that I thought was the coolest phrase I've heard in a while. It stuck with me. It said, a very useful way to look at a startup is that it's a race to learn stuff.
Rohit Agrawal [00:33:56]: Yeah, that makes more sense.
Demetrios [00:33:58]: Yeah, it's just a race to fail and fail and then learn what works. And so that's exactly what you're saying. Like, it echoes this product velocity. How fast can we learn if this product works or it doesn't?
Rohit Agrawal [00:34:11]: And. Yeah, exactly. And you want to remove as many bottlenecks. That's like slowing down your learning curve as much as possible. And, like, probably the only, like, bottleneck should be how quickly are you getting feedback from customers. But beyond that, you don't want to slow down your process of delivering value to customers.
Demetrios [00:34:28]: Yeah. Cause that's in your hands.
Rohit Agrawal [00:34:30]: Exactly. Yeah, yeah, yeah.
Demetrios [00:34:32]: The other stuff, maybe it's a little bit more difficult to get that feedback or that. That loop coming back. So it makes sense, man. So, all right, cool. Well, that is a. That's a solid one. I mean, the managed service one, too. Just how different products are going into different spaces always fascinates me because it feels like everybody's trying to become the one platform, like the databricks.
Demetrios [00:35:04]: And so in five years, all of a sudden, you can do Everything that you can do on databricks on Confluent now. I don't know if you have thoughts on that one.
Rohit Agrawal [00:35:13]: Yeah, I mean I think a good example of this, for example, is like Iceberg and I think Iceberg is on track to become like. I think what GitHub is to code, I think Iceberg will be to data basically for any organization essentially it'll be the central place in which everything is stored. And all the vendors, could it be databricks, could be Snowflake, could be confluent, essentially they have to read and write from that, but then everything is stored there. And we are seeing more and more of these services becoming agnostic to sort of like these implementation stuff and focus more on how can they be more compatible with each other, especially when, for example, storage is now moved to a common solution. And I think we will see more of these things where you could more easily transition from one vendor to the other because you don't have to think about how the data is going to be migrated from one vendor to the other, which used to be like the biggest challenge. Like if you're storing data in one vendor, just migrating that to the other vendor without losing it in a secure way, I think it's going to be a thing of the past. And if all of this data ends up being stored in like Iceberg, et cetera, or like in open standard formats on the cloud, then switching from one compute vendor to the other is I think going to be significantly easier.
Demetrios [00:36:35]: Wow. And maybe it's not even that. Okay, we're on databricks now. We're going to migrate to Snowflake. It's like for this job we use Snowflake and for this.
Rohit Agrawal [00:36:45]: Exactly, yeah. Because at the end of the day it could just be that if you took a 10,000 foot overview, could be like there is some compute job that spins up, reads data from Iceberg and writes it back to Iceberg. That job essentially could be a databricks spark job. It could be a Snowflake query, it could be like a bigquery something, it could, etc. And I think we're already starting to see the early signs of this where a lot of companies are now mandating that we want all of our storage to be in Iceberg because we don't want to be in a vendor lock in with like any particular vendor among data storage.
Demetrios [00:37:22]: Man, that's wild to think. Like the analogy of Iceberg is to data what GitHub is to code.
Rohit Agrawal [00:37:31]: Yeah. I mean effectively, if you go to any organization today, by and large, mostly there might be several teams, like several different engineering teams, could be UI teams, front end teams, backend teams, et cetera. They're probably all storing their code on like GitHub and they're all searchable and they have like common mechanisms. They may have like different build processes, et cetera as well. Like that's building code, et cetera. But they're all effectively reading from GitHub and they're like making changes to the repo there. And I think like code was maybe ahead of its time and data is maybe catching up now. But I do imagine a world where like we don't have to think about like, is this data stored in one vendor and is this data stored in a different vendor? The data is just stored in iceberg and you can read right from anywhere, basically, as long as you have a standard data format and standard catalog.
Rohit Agrawal [00:38:19]: Essentially.
Demetrios [00:38:20]: Yeah, well, that's the dream. And then what it's like the data would be specialized for its specialized use cases. Like, oh, I've got this generative AI use case or I've got this fraud detection use case. And it's doing like you said, it's spinning up some kind of a compute.
Rohit Agrawal [00:38:40]: And yeah, I mean we're already seeing this for like structured data. I think it's kind of centralizing on this, I think unstructured data and different modalities of data like video, et cetera, we still have some room to go there. But I'm fairly certain for like structured data as well as like unstructured embeddings data, we are very, not very far from a world where we will see a lot of organizations just store everything in iceberg.
Demetrios [00:39:08]: Damn. Yeah, that's very cool to think about and what that unlocks.
Rohit Agrawal [00:39:15]: Yeah. And I think like, for example, like the databricks acquisition of like tabular, when databricks was themselves actually building delta, which was a different format than like iceberg. I think it's a great indication that even probably the vendors themselves are seeing where they need to head to and where the bug is going.
Demetrios [00:39:34]: The first thing that comes to my mind is this is happening with no like formal committees, no standardizing bodies. It's just happening because people see that this is the way forward.
Rohit Agrawal [00:39:50]: Yeah, I mean like a lot of the iceberg, like community came from like several different companies. I think there are like maybe 10 to 20 companies that are operating. But I think they're all realizing that we have to work together to achieve this solution or else we're going to end up in a world where it's possible that like 90% of the world is like using Iceberg, where we have our own storage format and they don't want to be in that world, like where they're like on a silo somewhere else. And so when, when there is enough momentum in this space, which I do think there is enough momentum in the space, a lot of people want to join the momentum as opposed to like, you know, stay away from it or like run against the tide.
Demetrios [00:40:31]: Now let's go back to something that you said earlier, which is folks are understanding that they are not necessarily in a position that they need to be like Google or be like Facebook and they don't need to be built for this gigantic amount of scale. Talk to me more about that.
Rohit Agrawal [00:40:53]: Yeah, I think like the, I think like the biggest example that's in front of our like faces is DuckDB and I think like in a world where, you know, let's say Google comes up with like BigQuery and like there's like Snowflake, there is databricks and there is presto Spark. A lot of these like very large scale batch data processing solutions, they typically came out from like very large companies because they needed it and they were kind of shoved down everybody else's throat that this is basically what you have to use. Right? Even if I don't have that much data or even if I don't have that much scale, I still need to use the system and deal with the complexity of like using the system. It's like if I wanted to go from San Francisco to New York instead of taking commercial flight, I need to fly a fighter jet and sort of deal with the complex. It's just not something that's needed. But some people may need it. And so that's why now I have to use it. And I think the recent popularity of DuckDB is a good example of it, right.
Rohit Agrawal [00:41:51]: Where people are seeing that yes, these snowflake bigquery solutions make sense for some kind of workloads and not all kinds of workloads. And I need to look at the workloads that I need to support and whether they fit better in DuckDB or Snowflake and bigquery, et cetera. And we see this as well where a lot of data pipelines don't need very complicated distributed multi parallel batch processing solutions. They're actually significantly simple to build on simpler tools. I think we've seen this in the batch world and I think we will see this in the streaming world as well where People will kind of like look at systems that are significantly simpler than Flink or Spark streaming, et cetera, and look at like building these systems on top of such primitives. Streaming I think is a little bit early where maybe there is nothing as popular in streaming as what DuckDB is in the batch world. And I think there's a wide gap there that there are some companies that are like addressing that, but nothing has broken out as much as DuckDB has on the bad side. And I think once we enter that world, I think it'll be interesting to see how people are choosing simpler tools like DuckDB, et cetera, versus more complicated solutions both across batch as well as stream.
Demetrios [00:43:16]: DuckDB is known for its user experience and I think people love that. The simplicity of it, or just like the developer experience too on top of it is magical.
Rohit Agrawal [00:43:28]: Correct? Yeah.
Demetrios [00:43:29]: And why do you think it is that in the streaming world we haven't seen that equivalent yet?
Rohit Agrawal [00:43:35]: I just think like streaming is slightly more difficult problem to solve. Especially I think you need to think about like ongoing state much more than you would need, think of, need to think of in a batch world. Batch workloads are typically a little bit more ephemeral. Like you can spin something up computer and then throw it away because you have the results. Streaming is more of an ongoing thing where you need to continuously keep maintaining state and that might itself grow over time, etc. It's just a little more difficult problem to solve. But I'm pretty convinced that like we will see something like that show up like in the next five to 10 years, possibly, or possibly even sooner.
Demetrios [00:44:13]: And I've heard a lot of folks talk about how streaming is a necessity and everything eventually will go to streaming. Do you believe that?
Rohit Agrawal [00:44:30]: I don't believe that, to be honest. I think maybe I have a little bit of a contrarian view. I don't see a world where people need to always only have streaming data and everything within streaming. The batch processing systems are getting very, very powerful in dealing with large amounts of data. The streaming systems are very good at getting the recent data. Right. I'll give you a database analogy which I think may make this slightly simpler. Right? So for example, we've always had transactional databases like Postgres, MySQL, et cetera.
Rohit Agrawal [00:45:05]: And we also had analytical databases like for example, Snowflake, Bigquery. Right? And analytical databases look at like fast historical data very effectively. And the transactional databases are very good for like changing one row or changing one data point, et cetera. And in the database Community. I feel like there was this time when people felt that we can just have one system do it all. And they had like this system called htap Hybrid Transaction Analytical Processing. So they can have one system that can do both. Like they can do transactional as well as analytical.
Rohit Agrawal [00:45:36]: And these systems did come out actually. However it turns out like these systems are, they can do a mediocre job at both analytics as well as transactional. And the buyer on the other side is looking for a best in class solution for analytical workloads and they're looking for a best in class solution for transactional workloads and they're not okay with a solution that does a mediocre job at both. Right. And so I feel that even if like streaming, even if we get to a world where everything can be done in streaming, I'm fairly certain that like there will be parts of it that are like more mediocre in performance and overall user experience than for batch. And so I do think that we will be probably entering a world where each of these systems will exist and they will just be significantly more powerful at doing what they do best, essentially.
Demetrios [00:46:28]: Yeah, just leaning in to their strong suits.
Rohit Agrawal [00:46:30]: Exactly, yeah.
Demetrios [00:46:33]: Is there anything else that we didn't touch on that you want to talk about?
Rohit Agrawal [00:46:39]: I think like one thing that we're seeing, interestingly that I think we may see more is bring your own cloud. And what I mean by that is a lot of times people have this like negative feeling about vendors because a lot of vendors want all the data processing to happen within their account and a lot of customers are like, you're dealing with sensitive data and I don't want the data to leave my account. With the warp stream acquisition we've seen confluent now offering BYOC solution or bring your own cloud so your data can still live within your account. Red Panda is doing BYOC and they're doing very well with that. We're seeing databricks already offer like a BYOC solution. Snowflake historically has not done that. They've kind of been in the world of like everything lives within Snowflake and give me all your data and I will store and process everything within my account. I think streaming solutions I think as well as batch I think will probably more move towards a BYOC solution and I think that will make for a lot of companies accepting vendors even more easy and a little bit less challenging than it is right now.
Demetrios [00:47:51]: So the BYOC is just like the vendor goes to wherever you are?
Rohit Agrawal [00:47:59]: Yeah, exactly like, basically like the vendor will deploy their stack in your cloud account instead of, like, asking you to move your data in their cloud account, essentially.
Demetrios [00:48:10]: Yeah. And that is a trend that makes a lot of sense to me.
Rohit Agrawal [00:48:15]: Yeah. I think, like, historically, vendors have been a little bit opposed to that, but I think they're realizing that the gravity is where the data is.
Demetrios [00:48:22]: Yeah.
Rohit Agrawal [00:48:23]: And it's easier to move their compute systems than to move any vendor's data into anybody else's account.
Demetrios [00:48:29]: Exactly. It's so hard to get that data to go somewhere else just because of the sensitivity of the data.
Rohit Agrawal [00:48:36]: Yep. Exactly. Yeah.