The Future of AI and ML in Process Automation
speakers
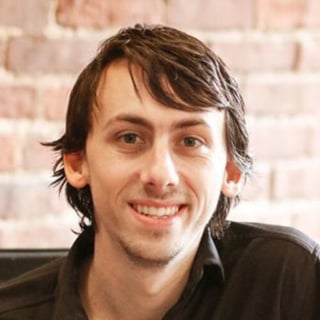
Slater Victoroff is the Founder and CTO of Indico, an enterprise AI solution for unstructured content that emphasizes document understanding.
Slater has been building machine learning solutions for startups, governments, and Fortune 100 companies for the past seven years and is a frequent speaker at AI conferences.
Indico’s framework requires 1000x less data than traditional machine learning techniques, and they regularly beat the likes of AWS, Google, Microsoft, and IBM in head-to-head bake-offs.
Full bio here: https://kitcaster.com/slater-victoroff/
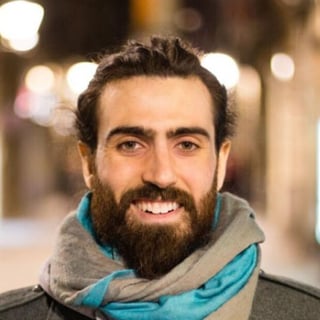
At the moment Demetrios is immersing himself in Machine Learning by interviewing experts from around the world in the weekly MLOps.community meetups. Demetrios is constantly learning and engaging in new activities to get uncomfortable and learn from his mistakes. He tries to bring creativity into every aspect of his life, whether that be analyzing the best paths forward, overcoming obstacles, or building lego houses with his daughter.
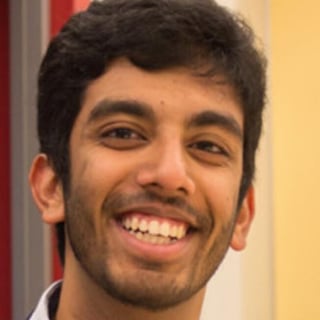
Vishnu Rachakonda is the operations lead for the MLOps Community and co-hosts the MLOps Coffee Sessions podcast. He is a machine learning engineer at Tesseract Health, a 4Catalyzer company focused on retinal imaging. In this role, he builds machine learning models for clinical workflow augmentation and diagnostics in on-device and cloud use cases. Since studying bioengineering at Penn, Vishnu has been actively working in the fields of computational biomedicine and MLOps. In his spare time, Vishnu enjoys suspending all logic to watch Indian action movies, playing chess, and writing.
SUMMARY
The Unstructured Imperative Recent advances in AI have dramatically advanced the state of the art around unstructured data, especially in the spaces of NLP and computer vision. Despite this, the adoption of unstructured technologies has remained low. Why do you think that is? How have the dynamics changed in the last five years? Multimodal AI Historic AI approaches have generally been constrained to one data modality (i.e. text or image). Recently, a wide range of papers in image captioning and document understanding have emphasized the need for more sophisticated "multimodal" techniques which can fuse information from multiple modalities. What is multimodal learning, and why is it so promising? Why are we seeing such an explosion of activity? What is Indico doing in this space? Machine Teaching As methods of supervision become more complex and multi-faceted, many researchers have begun investigating the inverse problem. That is how do we design supervision systems that more naturally follow human processes? What are some interesting trends in "the space", and where can we expect this field to go in the next few years?
TRANSCRIPT
Qoutes
“The key problem that we recognized when it came to using deep learning in an accessible way was training data fundamentally is too inaccessible. It requires far too much.”
“I think some things that are really really interesting are understanding which techniques have become redundant and which techniques are now more effective.”
“When you look at a lot of indexing techniques that we use for search engines, those are now amazingly useful because suddenly you can regenerate inputs for them that are 10 times better than you could before representing data in a richer way than you could ever imagine.”
“I think you can see these traditional techniques that are now ten times more powerful. And in other places maybe more on the traditional data science side where they become less useful.”
“For me, unstructured data is everything that’s not rows and columns.”
“There’s very clearly easier techniques in ML when you look at rigorously laid out forms.”
“Now, because the technology has advanced so far it is significantly easier to take up some of these modeling techniques and enough people have seen these dramatic successes. This is one problem set that you can address with a unified technology stack.”
“Modelling is simply not the limit anymore.”
“You’re going to see a lot more when you come up with this notion of changing the way that you want to do supervision.”
“The way that we tend to structure these ML problems, they’re very far from how humans think about the problem.”
“People don’t use supervised and unsupervised consistently. I think it’s a problem with the terms. It makes it sound like they are alternatives to each other, they’re really not. Any modern system you’re doing supervised learning on top of unsupervised learning, that’s just how it works. They serve fundamentally different functions.”
“Everyone knows in practice, you’re always dealing with less gold standard data than you want.”
“Active learning has failed to reject the null hypothesis. Let that sink in first. No one can prove definitively that active learning works.”
“You can get active learning to work on any data set, it’s this weird paradox. It turns out that the key is the way that you set active learning up on that data set what I would call human overfitting.”
“In practice, as often as you wanted to sharpen a decision, you wanted to change a decision boundary and obviously you can’t fix that. But at the same time, any given problem will have a reasonable trade-off between sharpening and exploring. You find that for the given problem. It will work for that problem just not generically.” “The user with the best understanding of the process is the person best situated to train the model.”
“The proper data scientist I think is going to be most effectively deployed designing experiments deciding what metric is important for us to track.”
“They say humans are much better at evaluating what is a weird example and what is not.”
“We set things up in a very definitive one-way flow, at the same time it’s very important to focus on the quality of the data and consistency of data because models will not fix a broken process, they will only replicate a working process.”
“Older techniques, if you’re relying heavily on some aspects of formatting, they break hard when things drift.”
“I think people a lot of times don’t recognize the cost of making a bespoke change to their ML model.”
“People often think about model understandability and explainability as interrogating model weights. I think that’s the absolute wrong way to think about it for a hundred different reasons.”
“The explanations that you are looking for are in your data, they’re not in your model. In the model, you’re just chasing phantoms when the data actually has the answers.”
“There is this joke if you have 24 hours to build a model, how do you do it? How do you build the best model? You spend 23 hours labeling data and 1-hour building a model.”
“I think a lot of people spend years trying to figure out how to get out of labeling 50 data points and it’s bonkers to me.”
“It was the limiting factors for us several years ago. Today, the models are so sophisticated and capable that data is our limiting factor.”
“The statistics and how the training and information flow, that is just as important as what database you’re using.”
“The number one thing to focus on is making sure you’ve got a good problem definition.”
“People want ML to be like a genie that shows up and an oracle that does everything perfectly.”
“You need to start with good consistently labeled data. Recognize that these are really big enterprise-grade systems to put together at the edge of what is possible from a compute perspective.”
“The failure rates are real. They are cautionary tales. You need help to do it well I would say, so look for help. There’s a lot of help out there I’ll say as well.”