The Future of Data Science Platforms is Accessibility
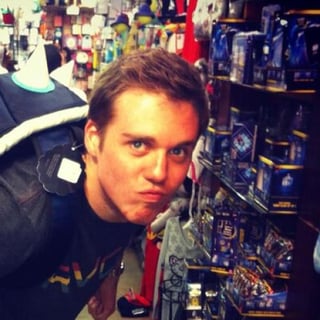
Data is a superpower, and Skylar has been passionate about applying it to solve important problems across society. For several years, Skylar worked on large-scale, personalized search and recommendation at LinkedIn -- leading teams to make step-function improvements in our machine learning systems to help people find the best-fit role. Since then, he shifted my focus to applying machine learning to mental health care to ensure the best access and quality for all. To decompress from his workaholism, Skylar loves lifting weights, writing music, and hanging out at the beach!
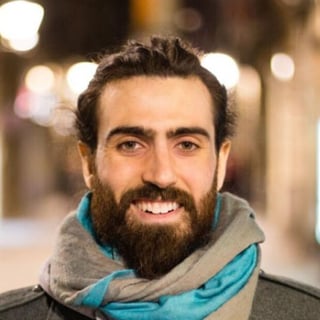
At the moment Demetrios is immersing himself in Machine Learning by interviewing experts from around the world in the weekly MLOps.community meetups. Demetrios is constantly learning and engaging in new activities to get uncomfortable and learn from his mistakes. He tries to bring creativity into every aspect of his life, whether that be analyzing the best paths forward, overcoming obstacles, or building lego houses with his daughter.
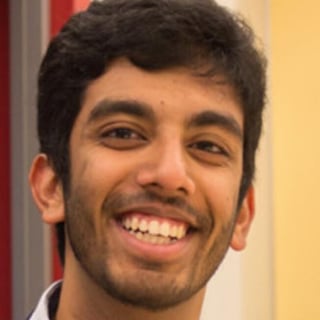
Vishnu Rachakonda is the operations lead for the MLOps Community and co-hosts the MLOps Coffee Sessions podcast. He is a machine learning engineer at Tesseract Health, a 4Catalyzer company focused on retinal imaging. In this role, he builds machine learning models for clinical workflow augmentation and diagnostics in on-device and cloud use cases. Since studying bioengineering at Penn, Vishnu has been actively working in the fields of computational biomedicine and MLOps. In his spare time, Vishnu enjoys suspending all logic to watch Indian action movies, playing chess, and writing.
The machine learning and data science space is blowing up -- new tools are popping up every day. While we seem to have every type of "Flow" and "Store" you could imagine, few people really understand how to glue this stuff together. Despite all the tools we have available, we still see companies failing to leverage data science effectively to drive business results. Instead of spending time driving business results, data scientists spend their time fiddling with Kubernetes, trying to debug that Spark serialization error figuring out how to map their code into the awkward "AI Pipeline" SDK. We have an industry filled with tools built by engineers... for engineers, rather than for data scientists. It's deeply disempowering. Meanwhile, data is still used effectively to drive decisions in many companies. Analysts have been solving very similar problems on the back of applications like Excel, Tableau, and Mode for literally decades. While there are still challenges in analytics, the MLOps space could learn something from analytics tools. Analytics tools better understand how to make their tools accessible. Analytics tools better understand the value of iterability. Analytics tools better understand that data problems are wicked problems: - we have to iterate on the formulation and solution simultaneously - they involve many stakeholders with different opinions - there's no "right" answer - the problems are never 100% solved. If we're going to really drive the most business value from data science, we need to understand how to design our teams and tools to effectively work against such problems. The future of data science platforms is accessibility and iterability.
Quotes
"Industries naturally go through bundling and unbundling and it seems like we have unbundled too much. There are just too many pieces."
"We need to focus on iteration and also accessibility as first-class concerns."
"There's a lot of complexity in data space absolutely. I also think that right now we're in a phase where there's so much noise that people are taking on more complexity than they really need just because they think that's what you're supposed to do."
"I got to see a lot of diverse perspectives. I don't necessarily think that there's something that needs to be corrected with machine learning engineers needing to understand analytics."
"I do think everybody, no matter what role you're in, you need to find a way to bring in more diverse perspectives from other roles."
"The more that you can reach out, the more that you start getting cross-pollination of ideas and experiences."
"If you're an MLOps team of one, your bar production quality should be much lower than a team of 10. That should be clear and obvious."
"We have to be careful not buying off more than we can chew. We have to scale our efforts down."
"I'm a big fan of crawl, walk, run. I think a lot of people try to generalize too early. If you haven't done it manually first, probably if you try to automate it, you don't have enough information yet and you're probably going to mess it up."
"I need to prototype and work through it manually before I can build a good automated solution."
"I strongly think you're going to have pain no matter what you pick."
"Part of the problem is people just think that the grass is greener on the other side because they didn't experience too much pain. So I don't think that there's a huge risk of that happening unless you don't even look at the space at all and just pick randomly."
"You should be spending the time on filling the gaps of whichever thing you're picking. So the key insight is that every product is going to have gaps that you're going to have to fill."
"The future of your company is uncertain. There's inherent noise and uncertainty. So we have to be careful of not assuming that there's more precision than we actually have."
"There is a trade-off of sharing data. If you don't share any data, you often are going to lose out on some benefits."
"Make sure we only collect the data that is useful and we know is useful for a specific purpose."
"Make sure that we have the right language and what not to communicate to people that is why it's useful to collect."
"Make sure not to retain data that we don't need."
"We need very very strong and clear controls about the data that you can opt-in and out of."
"One of the challenges in this space is you have such heterogeneous data. All these signals are super noisy. The labels that you're working with are very sparse."
"Our goal is to make a scalable solution to expand access to care."
"Data scientists often don't have the MLOps knowledge but It takes a village of mentality."