The Long Tail of ML Deployment
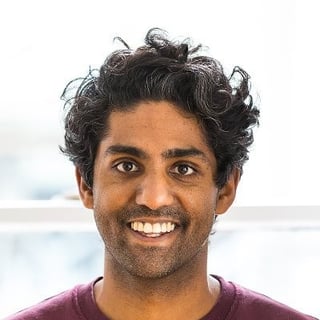
Tuhin Srivastava is the co-founder and CEO of Baseten. Tuhin has spent the better part of the last decade building machine learning-powered products and is currently working on empowering engineers to build production-grade services with machine learning.
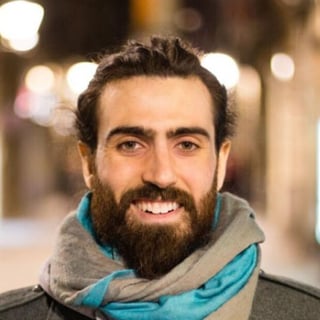
At the moment Demetrios is immersing himself in Machine Learning by interviewing experts from around the world in the weekly MLOps.community meetups. Demetrios is constantly learning and engaging in new activities to get uncomfortable and learn from his mistakes. He tries to bring creativity into every aspect of his life, whether that be analyzing the best paths forward, overcoming obstacles, or building lego houses with his daughter.
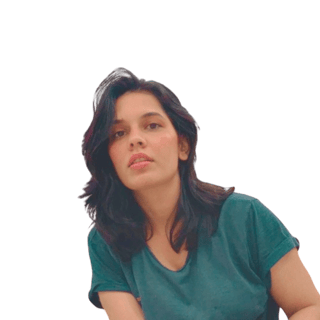
Abi is a machine learning engineer and an independent consultant with over 7 years of experience in the industry using ML research and adapting it to solve real-world engineering challenges for businesses for a wide range of companies ranging from e-commerce, insurance, education and media & entertainment where she is responsible for machine learning infrastructure design and model development, integration and deployment at scale for data analysis, computer vision, audio-speech synthesis as well as natural language processing. She is also currently writing and working in autonomous agents and evaluation frameworks for large language models as a researcher at Bolkay.
Prior to consulting, Abi was a visiting research scholar at UCLA working at the Cognitive Sciences Lab with Dr. Judea Pearl on developing intelligent agents and has authored research papers in AutoML and Reinforcement Learning (later accepted for poster presentation at AAAI 2020) and invited reviewer, area-chair and co-chair on multiple conferences including AABI 2023, PyData NYC ‘22, ACL ‘21, NeurIPS ‘18, PyData LA ‘18.
Baseten is an engineer-first platform designed to alleviate the engineering burden for machine learning and data engineers. Tuhin's perspective, based on research with Stanford students, emphasizes the importance of engineers embracing the engineering aspects and considering them from a reproductive perspective.
Okay. Yeah. My name is Tuhin Srivasta. Um, I am the, uh, one of the co-founders and CEO of Base 10. Um, that basically means I do a lot of, you know, nothing and a little bit of everything. Um, and the how I drink my coffee, I drink a black. Um, I, but, you know, straight, like I, I, I really enjoy coffee, so I would drink any.
Formal coffee. Um, I like espresso, but you know, being in America long enough, you end up just drinking a lot of black coffee, which is a very American thing. Hello everybody and welcome back to the Lops Community Podcast. I am Dimitri Osen. I am joined by none other than Abby. What is going on, Abby? We're recording the third intro today, so the same time.
Great. Or just to see you when you That is true. Listener. If you were wondering, we just pounded out like three different intros and we're getting pretty good at it right now. This introduction though, we just got off the call or the recording with Tune and I loved it. I really was fascinated by some of the stuff that he was talking about.
Did you have any key takeaways that I can add onto What were, what'd you like from the conversation? Actually, when I was looking at Base 10, I thought they built a platform for machine learning engineers and data engineers to uh, not have to do the boring stuff, which is the engineering stuff according to some people boring stuff, especially data scientists.
Um, but it seems like he had a very different perspective, which was based on their research with a lot of Stanford students. And I liked what he said, which is, Eventually you have to become an engineer and you have to think about everything from very product perspective. And that is the key audience that they've been built in the platform work, which is engineer first platform.
Yes. I, I love how he said, I mean, this was probably the hottest take of the conversation, which was. Data scientists are an artifact. It was like a blip in time and he's not sure that they're gonna be around much longer, or they're gonna be here for in 10, 20 years. I thought that was oof. He's going out on a limb there.
But yeah, the whole conversation that we had around basically empowering the software engineers with machine learning and ai, I thought that was awesome and really liked hearing his viewpoint on it. I think the, another good part for me, or the highlight with going a little bit into microservices and how he thinks about using microservices to build products of the future, especially as we are working with bigger models, it has become a little bit more obvious, but for them, they were doing it before it was cool.
Mm-hmm. Yeah. Yeah. He has been at it for a while. They started in 2019. They've been plugging away and. Now the space that he's in just absolutely blew up, so it's good to see. I'm glad that they're finding success, and I hope you all enjoy the conversation. Of course, the biggest thing for us that could help right now is if you know one person that would enjoy this episode, and if you can share it with that one person, that would mean.
So much to us right now. I have one question for you, DEIT. Did we get any reviews or comments? Oh, we did. Oh, I think we did. Hold on. Oh shoot. I gotta, no, gimme a sec. I'm gonna go find them. What do we got a, okay. Oh, we got some awesome reviews. This is cool. So wait, this one might be from you. What is this from you?
Did you write a review? No, it didn't. All right. I just saw the name. It's from Go Ali, Alix. Oh, okay. I can't pronounce that. But, uh, this is the most recent review. I'm a senior ML engineer who deals a lot with lops related items. Since we don't have a dedicated role for that on our team, this show and the Slack community in parentheses, have been great resources for inspiration and staying up to date on the constant evolution of tools and the best practices.
It's very useful to hear from other practitioners as we all try and navigate this landscape together. Whoever you are, go Ali, whatever. I get tongue tied. Oh, that is so cool. I love seeing that. That is absolutely too kind and I appreciate it. There's also another one from somebody that is, uh, Italian Grazi Zi.
That's what we got. Longtime listener. I'm glad the show is going strong. Robot emoji arm. And so fist bump. Yeah, if fist bump, if you wanna leave us a review, that would be super cool. It, it is very helpful to see this stuff. And, uh, I love reading these. I don't see, it says we have 10 of 'em, 10 ratings, but I only see three of 'em.
How do I see all of 'em? What's going on? Whatever. So I'm not gonna sit have these, uh, people get bored and sit through this. But that's the reviews and it would mean the world to us if you left a review. Also, share this with one person, leave a review, show us some love, and let's get into it with
Su to end to end. Dude, I'm just gonna, Technically having on, on the show. Guys, nice to meet you. Oh, happy man. You've got an interesting background, dude. You were in investment banking and now you're in machine learning infrastructure. Give us the breakdown. How the hell did that happen? Yeah. Um, it's, it is, it is not that interesting.
It's, you know, I, I was an engineer in college. I went to work in banking. Spent two years in banking. Um, Got bored outta my mind. You know, honestly, like was, was uh, like a lot of good, but just also just so boring. Um, the, I think the real positive of investment banking is that they just teach you to work very hard when you hate your life and you're not having a good time.
Um, and that translates really well to when you're having a great time and you're working on interesting things where you're like, I could work forever. Like, this is love. Yeah. Um, live CT now. Um, but yeah, I was looking at banking. Um, the bar is so low. Whoa. You just, it is just like, it, it, it's a really interesting dynamic, right?
So like every, there's a side note. Like every, every night at 6:00 PM your director would come to you and say, I need this by 6:00 AM tomorrow. Oh, at 9:00 AM tomorrow morning. And you'd be like, okay, cool. And so you'd stay up all night. You'd stay up all night, get the work done. And they didn't need it at 9:00 AM the next morning, but you have to do it anyway.
And if you do that every day for two years, it is one of the most horrible things. But, um, where it is just like work for the sake of work. And so, but you still have to do the work. I'm assuming you're making good money, but you're just getting grinded down. Yeah. This is one common complaint people do have about finance industry as well as about law.
Both do have that variability culture, which is like, Hey, make the associates work crazy hard. Yeah, yeah. No, I, I think that's right. I, I, I, they're, they're apprenticeship businesses, right? So it's like, like the, the general consensuses that you learn by doing, it's like, same as medicine as well, you know, where it's just like, we'll just work you to the ground and, you know, you.
You'll appreciate, you'll appreciate it afterwards. And then, and in law ways it actually like maintains the hierarchy of these, these professions. Yeah. Cause it's like you have to do the work. It's, um, it's pretty fascinating. But, um, I, um, did that for a bit and I was just like, I don't need to do this anymore.
And so I actually moved to Boston to work on some, um, biomedical research using machine learning. This is like 20 12, 20 11, um, um, using machine learning to predict. The prognosis of, uh, a neuromuscular disease. So we were trying to figure out really, really early on in someone's life, um, if they were gonna have, um, you know, one of these diseases and just for early intervention, um, published a few papers, um, you know, machine learning that was very different.
It was very much like the support vector machine, um, era, like gradient boosted trees were just becoming, were just becoming popular. Um, but really had a great time. And then, Just threw a series of twists and turns just kept getting deeper and deeper. You glossed over something right there real fast, which is like, yeah, I was sleeping under my desk and my boss was asking me for these reports by 9:00 AM and then I just like went and did this super advanced shit with machine learning.
Yeah. How did you know how to do this advanced stuff with machine learning in 20 10, 20 11? Yeah, so I studied signal processing in college, so I had like a, like a probability background. Um, and I had, I did a bit of information theory, um, um, here and there. And so I think like, from like a technical perspective, it wasn't, you know, it wasn't, you know, it was just, you know, different probability, different statistics, um, as opposed to, you know, something really, really new.
And so I, I actually. I spent like the last three or four months while I was at that job just learning re like relearning statistics, um, in a coffee shop on weekends. And then, you know, I, I was like, all right, I need to go, uh, recruit out of, to boss, uh, to work with an biomedical thing. No, actually I, it was, I called, I out downed a bunch of people, um, in, in Boston.
And then I ended up, I think I met one, one of the guys I met at a coffee shop, the one I ended up working with. Um, and so like, it was, it was, it was, you know, when you are, I dunno how old you guys are, but I was 23 then when you're 23, you just have so little to lose. You feel like you have so little to lose.
Um, and so nothing, at least for me, I was just like, all right, sure. Sounds good. I'll, I'll, I'll go and work at a lab for a bit. Yeah. Doesn't matter. What else am I doing? Selling my solo, sleeping under desks. Otherwise I Oh, classic man. That's so cool. So then you got into ml and then you started getting really into it, I'm assuming, like where happened then?
I started, I started working with machine learning in like a very applied sentence. So worked, worked on fraud detection, content moderation, um, stuff. Um, you know, the, the kind of like, I'd say TW 2010, early 2010s use cases of machine learning. You know, lead scoring, you know, those types of stuff. And I, I think, um, eventually it went and started another company that was acquired in 2018.
But like, it, it was, it was pretty, it was pretty interesting. Cause I think in 20, in 2010 to 2020, like no one really had any expectations of machine learning. And so, like most people were just treating it like a research function for the most part. Right. Even in companies, it was just like, Unless you're at Netflix and working on recommendations or, you know, Uber and working on, um, you know, ETA prediction and, um, those types of things, you know, you're going to places that were pretty underinvested.
So they'll be like, oh, we have a machine learning problem. We'll just hire, we'll hire a few people. We'll hire, um, we'll hire a few people and then let's, um, hope something comes out of it. And, you know, ultimately nothing did. Um, but for, for me at least, like, I like that wasn't. I really didn't want that to be the case.
So I ended up just becoming an engineer as well, uh, to be able to support myself as a machine learning engineer. So I was like, all right, if I'm gonna do ml, you know, I need to understand how to actually get this to value for someone as opposed to, you know, building a Jupyter, you know, um, training a model on a Jupyter Notebook, um, and showing a confusion matrix to someone like that wasn't the, that wasn't the end.
That's us end goal at all. For me. And so it was very much like, how do you, how do you build, how do you, how do you get this into products? That was, that's kinda like the thing really appeal, which is the transition towards ML engineering slash lops business at Gum Road, or was it at the next company Shape?
Yeah, go. I, I, I, I, I at Gum Road. Um, it was very much that we didn't have the resources for, to have like engineering help on the ML side. So the founder, a guy called . Um, um, was very much just like, you know, you can do whatever you want, but you're gonna have to do it by yourself. Um, and so I just did, I just figured, you know, I ended up learning, but, you know, a, a bunch of service side engineering a but a front engineering, you know, we hired another machine learning guy who's, you know, a friend of mine and one of my co-founders of this business, and he, he, and he had a PhD in mathematics and he was just like, Could twin, some models, should basically, should, you know, should become an engineer.
So he became an engineer and so we kind of ended up with, I'd say at the time, a differentiated skillset in that we were, we are machine learning people, but we also knew how to build products. And so that was at like 20, 20, 12 to 20. Yeah. Think most of people have very similar 20 to 2015 as well, which is like most people start out as a data scientist doing very much like.
Just, just basic analysis stuff, and then eventually you're in one of those two spots. Either you start working at bigger company, where you nationally wanna transition towards the cool stuff, where you end up building production where you can't sort of show your value and you feel like, okay, my product is actually being used instead of being thrown away because your model is no longer relevant.
Exactly. If you feel good. Yeah. It does have, uh, I, I think shelf life as compared to just the data science work. Um, yeah. And the others who are in our spot, which is my, my journey was very similar, which is start work as a data scientist and then it was like, Hey, nobody was there to do engineering. Learn everything by yourself.
Somebody had to one person on the team and then eventually everybody starts picking up. Yeah. And, and, and I think that's like, what's super interesting, we can maybe talk about, this is just around like, maybe like the last six or seven years, like last 10 years. It was about like, you know, the data scientists who became engineers.
Um, and now like I think the world, the power has completely shifted and now it's like, hey, every engineer needs to learn a bit of machine learning. Um, and like what that means, we're still trying to figure it out that we, it's flipped and like, it's crazy cuz like, I think this has flipped in like the last six months.
I haven't flipped in like, It Flipp very, very quickly. Um, but it is a, the paradigm just very, very different now. Um, and you know, I think, but I think that's right, which is like you either go to a team where you have support or you figure out how to support yourself. There's no data scientist for the sake of data scientists.
Go on. Um, Demetrius Yes. Go. Government is super go. Yeah. You, I, I cut you off earlier. Um, government is an interesting company. It's kind of gone. It's kind of had, it's kind of had, I mean, I, I know that. It is almost like a cult-like status with the indie hacker, uh, community thing. Just because you're able to Yeah.
Use gum road to quickly validate ideas or get those like digital products out there and, and then sell them. Yeah. And so you were mainly working on fraud problems in that and then, You learned how to be like an engineer? Yeah. Um, so we joined really early, you know, there was, we was working out the founder's department at the time, just a few of us.
Um, and then government had very, very early had this fraud problem because there was this two like open-ended platform where anyone could sign up and get paid. And so we would just get, you know, I think the first, like we, we came in one day and like, you know, the. Like, we'd grown like crazy and everyone was like, fuck, this is it.
You know, this is, sorry, my marriage, but this is it. This is the moment that we explode. Um, turns out like, you know, we'd had like, 99% of it was fraud. Um, and, you know, it was like really, really clear that once you create like f friction, friction and fraud, like they, like government tried to reduce the friction and the payment flow.
But that also meant, the downside to that was that it became very, very easy to fraud. And so we, we had to basically, um, build ml because he, Saul did not want to, you know, he was kind of uncompromising of wanting to, um, add friction to the process. So he was like, we just have to get very good at, uh, catching it.
And then like, I think in, uh, A secondary problem. There was like content moderation. So we, you just get some, again, anyone could upload anything, so you get some really weird shit like, you know, the internet's pretty weird. Um, and um, and you know, when you're dealing with payments and, and, and payment processes, they're not okay with a lot of stuff like drug para paraphernalia, political stuff, pawn stuff, you know, like they're like, they're just all, a lot of, a lot of weird, a weird, like, it was like a weird sub-industry and we just had to like try to catch it as quickly as possible and take it Duke Road.
You had a hot take there. That I think is awesome that I want to go back into, which is that it went from data scientists needing to learn engineering. Now it's been flipped on its head and engineers need to learn ma machine learning, like keep going with that one. I wanna pull on that thread a little more.
Yeah, yeah, yeah. This, this is fascinating. Like this is something like, I've just like been thinking more on that, more about it, especially with our own customers as we've seen this, like develop and see who's having impact does that, My, my, my, my, my, my, um, take right now is that data scientists, and I was one of these people.
Um, and you know, like, I know a lot of your listeners might be data scientists. Do I think data scientists were a relic of the past? I think, you know, we, it was just this like flash and time moment where, you know, we didn't know how to actually get value from machine learning and it was like this research e function.
And so what we actually did was we're like, oh, like, you know, And, um, I think a, a Abby, um, said this too, which is like, you know, we looked at the people where we thought this stemmed out of and we're like, oh, there must be data people, you know, like the data people must do the data science, you know, and so you'd basically have these people who are traditionally analysts go and, you know, read something.
They're working in our, you know, a lot of the initial machine learning libraries were in our, like a psychic learn was the adaptation of a. A library, um, in, in, in our, and so, you know, they, they'd go and start doing machine learning. Um, turned out like, you know, analysis and, and machine learning are actually like very, very different things than they, it just ha happened to stem out of this.
And we built a whole industry for, you know, from 2012 to 2020 around supporting data. People who became data scientists to, to do machine learning. Um, and even the skillset, even honestly, even the product that we built three years ago was built under this premise. Um, turns out that like there's so much more leverage, there's so much more leverage when you engineers use machine learning because they can build their own products.
They can actually think about productizing it, and they're not reliant on getting other people to build for them. So, um, you know, my, my take is actually what we're gonna see now is that. You know, da, like the, the data people who became machine learning people, I think that's actually gonna shrink back.
They're just gonna go back to DA being data people. And machine learning is gonna become just like a massive, massive part of every engineer's, um, toolkit. And I think like, one, one thing that's super interesting is that, um, you know, you look at, we, we, we, so one of, one of our, uh, investors who we at Demetrius, who we met through gridlock, um, Um, they have a pretty like active recruiting effort, especially on campus.
And we, we do a lot on campus recruiting at Stanford. And one thing we get at like out of this is that we get like what the, like what their primary, what their, like for every en software engineer who's graduating as part of the career fair, what their primary focus is. And you know, we've been building, building this company for three and a half years now.
Um, and. So we have like three or four kind of data points here, like snapshots in time every May. What, what are, you know, what do the engineers know and what do they want to do? And I, I swear it is gone from like their top prior, their top focus being machine learning, probably about 20% in 20, um, 2019 to like this, like last year it was like, you know, 70 to 80%.
I'm guessing this year it was gonna be like 95%. There's no doubt in my mind that this year to go 90, and I think like that is just like the arc of the arc of the skillset, which is like engineers learning how to adapt these things that, you know, maybe not even having to understand like, how deep do I need to go into this model?
Being able to choose it like a black box, like taking, taking something and understanding how to appropriate it as opposed to, you know, starting from scratch. Well, dude, you said a lot there and. That's, I guess the next question is, sorry, how deep do these software engineers need to go? Because that is something that I think people ask a lot in the lops community, and one thing that I think about is how we just had this survey, right?
And it was all about people using large language models and how they're using them and what tools they're using around it. And you do see that. The barrier to entry has been lowered. It's not been lowered. It's just been absolutely disintegrated. Yeah, there is no barrier. Now, if you, even if you can write in English, you're good.
Like you can figure this out. So one thing though is that how, like how much value you can get if you know, How to go into TensorFlow and really turn some knobs and host your own like, yeah, open source model and maybe bring it down. Maybe you don't need this large language model. You can just use a smaller model and train it on your data, your company's data.
And so maybe we could go into that a little bit on how there is a bit of a juxtaposition. It's like there is this gateway drug, which now is like G P T, and you can go into machine learning and you can get. A feel for what AI can give you. And then if you want to go deeper down the rabbit hole, you can start to figure out, all right, well how do I play around with these open source models?
Yeah, yeah. Yeah. I, I think, I think it's a good question. I think, I think like, I, like, I, I can start with like an anecdote, right? So like, and on Dimitri, we talked about, um, this guy, this project last time we talked was like this, this fusion project. At the end of 20, uh, 2022, they kind of took off, which was, you know, it was based off stable diffusion and it was fine tuned to create spectrograms.
Let's fine tune and create spectrograms. And, um, and so basically what you could do, and you could still do it right now, you get a fusion.com, you can type in like, you know, Taylor Swift, you know, dancing to ball, uh, like making Indian music and like, it'll spit out something that sounds like. Someone that's Taylor Swifts and like, you know, you gotta use your imagination a bit.
Um, the, I I, I'd say that, that, like, to me, that's like a really good example. Like that's the, the, the, the engineer who, or the engineers who built that, um, you know, they didn't have a ton of machine learning experience. Like, you know what, like they have a lot of music experience, they have a lot of domain experience for our music and they have a lot of intuition around music creation.
Um, but they were able to more or less use, The machine learning part of it as a, and, and like, and mind you, they weren't just using the input, input stuff. Like they were, they were still fine tuning it like they were going and, you know, they had a wait, wait, waits and biases open. I, you know, I don't, I don't know if they had like a deep conceptual understanding of what was happening under the hood.
And, and you know, I don't mean this as a dis to them cuz, you know, I, I think very highly of those guys. Um, but really like they're able to create value by thinking of it, the product problem, not as much as like the research problem. And so I think we're like, thankfully we've gone it from this explore to this exploit state in the last, in the last year where we've gone from like, hey, like the research section was through explore part.
And the research stuff is still happening. Um, but we, we have got the abstractions and the understanding around these models now. That a lot of folks can exploit it without understanding, you know, how, how, like, you know what's going on on the end, you know, on the 27th layer and you know, like, what do I need to change there?
And, and I think that's fantastic and I think like there is still gonna be room for that. Um, explore that, explore state. But I think you could just go really, really far. You can just go really, really far without having, um, To go that deep. And we, you know, we see this with our customers too. It's just like the amount of engineers who are taking, like Wipo or who are taking stable diffusion or taking control net, um, or taking, um, llama, um, um, and, and being like, what can I do with this?
Um, is just, it's, it's astonishing. Um, it's, it's, it's really, it's really, um, you know, it's, it's, it's, it is unlike anything I've seen before, which is like, we just kind of like, We, we built the abstraction high enough or the value was, was, was high enough that we could just try to, we could finally treat these things as black boxes if maybe it's way, I love heard cambri explosion.
That is what's happened. That is the best way of explaining or describing what is going on right now. And when you talk about these software engineers that are now being able to exploit ML or ai. One thing that comes to my mind is how everybody's kind of on the same playing field, and so you don't really have any defensibility, it feels like, you know, uh, I look at those, uh, photo.
Yeah. You know, there was like, as soon as one person figures out that there is demand for a certain type thing, like the profile picture generated by stable diffusion, then there's copycats that pop up and. So I, I wonder how you think about that. And again, going back to like, Hey, should we really dive deep into the, under the, should we really dive deep under the hood and figure out what is going on to try and give us a leg up on the rest of the world?
Yeah. I, I, I think, um, I think, I think that will still exist, right? Like, I think there's, like, it's just like the. The, the long tail of use cases will be these kind of generic, commoditized businesses. Um, Eric Schmidt, uh, Zaha from Databricks, Eric Schmidt from Google, obviously, and, um, Mitra, I think Metro Ragu.
Um, they recently had like a, a blog post on this about, you know, how much do you think that this. This G p t kind of gateway drug, large language model off the shelf model service provider will be able to solve, as opposed to like, how much will you have to, you know, fine tune, how much will you have to go deep into?
And I think like the truth is, is that for the low value re I, I personally think, and I think this is their point, um, as well, that like for the low value, you know, long tail of use cases like the, the commoditized, the commoditized like gp, you know, G P T four, a p I call. Um, will, will become huge. But, you know, to, to turn that and make this, Hey, this works really good for medical, for this medical imaging.
Hey, this look like works really good for lawyers. Hey, this really looks, uh, works really well for, um, like, I don't know, design like I, or like for video generation. I think you're gonna have to go deeper and, and either come up with your own models and you know, just, or just like, Tune them or appropriate those models for your context.
And I think, you know, and like I think there, there is a case for like smaller, more potent, targeted models there as opposed to this general purpose. I do everything, I do everything model. Um, you know, do you want to, do you want, do you want to eat? You, do you want to eat it? Your, your, you know, the best. You know, I, I, I, um, I, I'm kind of like against PanAsian restaurants cuz like, you know, like, I don't think you can make Thai food and Japanese food, um, equally good.
Um, I think, you know, when, when you do want to get the, when you do want to get, uh, the best, when you do want to get the best Thai food, you'll still go to a Thai restaurant. You won't go to that, that restaurant that makes Thai food, Japanese food, and you know, Chinese food. And there is something I think that is, it's been, you've opened up a door.
In my mind. It's like there's so many different use cases out there that even if we try, the majority of people that are hacking around on stuff are not going to find those little use cases. And that is where the long tail is. And for those use cases, for the most part, A G P T is gonna be good enough because, There's not so many people searching around in that part of the universe.
Yeah. But then when you go to the use cases that have these huge unlocks, like you were talking about, like doing stuff for lawyers or for medical imaging, et cetera, et cetera, that's where you need to really add a lot more value than what you can just get off the shelf. Yeah. I, I, I think so. I think when you need concentrated, concentrated value and you care about speed, cost, and performance, Which is like the ho the the holy trifecta.
You're gonna have to go. Smaller, more targeted. Yeah, I mean it's, it's the core idea that everybody's talking about right now, which is how ENT would the generalized models be in the long run while they would be created, you know, giving you some sort of abstraction initially. And solving problems at that level.
Past that, we have to think of different things, which is, are we using another model and using it in like an actor critic setting? Where we are able to see first is to be able to set the card rails for the results generated from these models. Yeah. And the second is the question around. When to do prompting versus when to do fine tuning.
And where is the general kind of practice around knowledge distillation? Yeah. I, I think that's right. That, that, that, that seems right to me, which is that, you know, you're gonna, the, like, the first, the, the first question you're gonna almost make is like, is there an objective truth here? And will I, will I be able to, you know, I, this is like, I think, uh, um, Dennis from Mei.
I dunno if you guys have seen Mei. It's a, it's a cool company. Um, um, they, and you, you should have Dennis on the show. Cause I think he, he thinks about this in terms of product, like really, really, um, you know, neatly. Yeah. That's awesome. But one thing he thinks is just like, you know, if there is a task to be done, like if there is a specialized task to be done, um, the general stuff will break down for a number of reasons.
And then, You almost have the secondary question. It's like, what do I need to do to solve this? And it might be better prompting, it might be fine tuning, it might be a completely different model. Maybe it's maybe like you need this response within a hundred milliseconds and you need to, and you don't want it to be very, very expensive cause it's happening, you know, every three or four seconds.
And like, you're gonna have to get a smaller, more concentrated model. But I, I think, I think that at least to me intuitively, That, that seems right. I think like, you know, like we can, I can just say it because I think it's true on some degree as well. It's like open air models are really good right now.
They're like scary good, like, scary better, you know, than even like the more targeted stuff right now. And I think like there's a bit of catch up that needs to happen right now, um, for the things that we are talking about to be true. Um, I think, you know, like, but intuitively it does make sense to me. I think I agree with you, which is these are all the limitations of LLMs and you know, because again, you know, you don't have the backtracking accessibility that we did have in the conventional models.
But let's move a little bit towards the compute, which is the bigger elephant in the room. Any model that you're deploying now that Yeah, the fact that we've already proven in a way that bigger models might be a little bit better now, like. Trillion parameters. Yeah. But just the, just tiny bit bigger than we are using today could have bare performances.
So I wanna, yeah, you had very interesting blog post, which is how to choose the right horizontal scaling setup for high traffic models. I want, I want you to talk a little bit more about in terms of the context, because as you scale both the things increase, which is the compute as well as the storage.
Yeah, totally. Um, I, I didn't write that book post, I think it was written by someone, um, Elon team. Um, but, um, I, I, I think like what, what you're saying is, is like a hundred percent true, right? Which is like the. As these models get, as these models get bigger, they, and yeah, this is, this is a great plug for our company cuz the basis for, for our company, which is that, you know, a whole host of infrastructure problems, um, show up.
And so, you know, whether that be, you know, right now we're trying to deploy a, you know, I think it's like a 60 billion parameter, 60 billion, uh, billion parameter model with like floating point 32, FP 32 or something like that, which is. You know, huge. And I think like the, the, the challenges from like a scaling perspective, from a scale to zero perspective, from a cost perspective, from a, um, latency perspective, um, from a scale up, scale up perspective.
Like there's all these, like, you know, there's all these massive, um, barriers that, that, you know, stop you from using these models in some setting to build really, really great products. Um, And I don't. And I, and I think, um, you're right. I'd be like, this stuff is unsolved. Like now, now we're getting into territory where, you know, like when Lama came out like four weeks ago or whatever, like we were the first people to be able to serve that ef like, you know, really, really fast.
We created this chat lama thing. If you go chat lama.com, um, you know, you could, you could play around with it and you know, it got to the top because at the top of Hacking News, you know, for most of the day, and what you realize is that, you know, These models now that are bigger and like, I think AbbVie's, the current understanding is, is that the, the, the bigger models are performing better than the smaller, more specialized models.
Um, you, you, you're talking about deploying things that, or serving things at, at, um, in a way that'd be hard to serve in the past, but now, and like, because the, the regular, the, the most. The, the largest use case is almost like a consumer use case right now is at like a scale that we haven't thought about.
Um, and I, and I think, you know, again, a lot of these things are, I'd say from an OL's perspective and from an infrastructure perspective, like they, beyond my pay grade. And I, I couldn't tell you how we're doing it, but you know, we are, you know, there are lots of interesting things that we're doing from like a scale to zero perspective, from like a, um, auto scale perspective, from a cold star perspective.
Like how do. Like when, when I, when my, if I have this massive model and I only needed two out a day, do I need to have it running all day long? And when, when the scale is down, how long will it take for it to come back up? What's acceptable there? I think these are all the unsolved challenges and into getting these, getting these and, and like this is, I think what opening AI just so has done so well.
Like, you know, like, is that, you know, not only they made their models usable, like they didn't just create the best model. They made it usable. They gave you fine tuning infrastructure, they gave you serving infrastructure, they gave you great docs. Um, you don't have to think about scale up and scale down.
Um, you know, I don't think the rest of us and us included in, you know, um, a bunch of other products. Like we haven't, we haven't created that same moment of magic for engineers just yet to be able to use these models very, very effectively. Efficiency. Yeah. Another one of the bigger challenges that has been persistent in all our conversation with different engineers.
As well as data scientists is documentation how frequently do you document your code, um, and how do you document your models as well. And yes, there are options which is version controlling and model containerizations. Uh, so those all options are there. But you have something interesting, which is docs as code.
Do you wanna talk a little bit more about that as well and tell us what it is about? Yeah. Yeah, totally. Which is like one, one thing we've thought a lot about is, you know, um, how do we maintain a, a product that has like active living documents that all engineers can contribute to. Um, and so we, we, you know, we, we thought, we thought a lot about, you know, being able to create, um, be, you know, cleaning some scaffolding so that engineers could write one like doctor's code.
So it's, it's hosted in, in a, in a general purpose. Documentation system that you, you're contributing as code, but it's also integrated with the development process. Um, and I, and I think like, you know, Philip and I both, it's funny, both of those posts I think you talk about, were written by, written by Philip.
He's very, very passionate about this, which is like, how do you build docs, um, into the lifecycle of, of engineering? I think this is even more true from like a, from like a machine learning perspective, right? Because these artifacts, they kind of disappear. They kind of, these moments of time. And who knows what my, who knows what my, um, what my model was four weeks ago on, you know, my third laptop at at work.
Um, and like, how, how do, and we, we haven't solved this yet and I think anyone's done a good job yet, but like that documentation per perspective and that version management's perspective is definitely a massive open problem in machine learning. Um, still and like the stuff you are, Reference to Gabby is very much from like just our engineering workflow perspective, um, as opposed to within our product.
And I think like that's something we, we hear a lot from customers, which is, you know, I had a machine learning researcher or engineer, they came and trained a model, they disappeared. You know, what does their version do? Which version matters? You know, how do I find the other. I'm sure you guys talk about, you know, you guys hear about this so much, you know?
Yeah. From the, oh my god. From the community as well, which is like, yeah. Um, you know, and, and like there's stuff like weights and biases, which is making it easy from a training perspective, but still, like, I don't think most customers of ours and, you know, um, although we talk to, and most folks that you talk to would be able to tell you like which artifact is running in production.
Um, I, I, I, I think it's, you know, and I mean, another thing which I found interesting, looking at Base 10, I feel like you guys are an advocate for micro. Service-based architecture, and I don't know if that's across the stack. Yeah, or just across the models itself, because across the models, I feel like you're ahead of your type reason being, because now with LLMs now everything is, you have products built up on top of APIs.
Yeah. I, I think with everything, I think we think that like, you know, so like Baytown call is this way for, you know, it's like the way I like to think of it, it's like a toolkit. For, um, creating value from your models and like, you know, like, I think like the, a good analogy there is something like Versace, which Versace took your like, front end package and they said, Hey, where'd you gotta build a bunch of utilities and extend it from that to the real world?
Um, I think for us, like it goes from like all the model stuff definitely has to be a p i based and you know, like it's, everything needs to be a microservice. Like we, we not only does that model. Um, that model, um, itself and every single version have to have its own api, but the logging for it has to have an API so you can access the logs for it.
I think, you know, the, the, the place where this gets super interesting is that we also have these serverless functions that you can write on top of your model. So think about your traditional, like I, I'll take you back to like one use case. There's like one user has this massive large language model, gets hit a lot.
They're trying to use a performance optimization, so they need to build a caching layer on top of it. Very, very typical product thing. Where you gonna build that? Well, you can go and build that in your own monorepo somewhere, but Base 10 gives you a serverless function and access to a database, so you can build that caching layer into Base 10.
But those serverless functions that do the caching and call the model, they have their own APIs as well. And, and like by separating these concerns, We can scale up differently. So like maybe your, your model actually needs a lot less tra uh, to support a lot less traffic than your overall service. Maybe vice versa, but it gives the, the, the user a lot more control.
Over how this, this model will be used in the application of business context. One of the things I was concerned about when we say, Hey, we're going to advocate for microservices or API based solution across the entire stack, is how do they scale? Because microservices, again, to have their own limitations if we have too many APIs versus, you know, testing and production becomes a little bit more complex.
Second thing is it just increases operational complexity. Yeah. Managing their consistency is another issue. How do you work past those challenges? Yeah, I mean, I think that these are pretty challenging, but like, I think you just gotta treat it just like, um, you would with, it's like a traditional api, right?
Which is that, you know, it's you, you have your c i CD system, you have, you know, like what one thing that we don't do now that we are working towards is to have a much more get based workflow is like, you know, you, you don't ever think when you have a. When your web app is running, um, the user never has to think about, Hey, what version is running?
Right? It's just one, it's just one url, and then behind the scenes they give you access to what, you know, what is, what that is pointing to. I think that's what we're trying to get to as well, which is like, you know, you have versions on your model, but there's only one primary version and that is the thing that gets used by everything else.
If you want to call another version that still works. But you need to keep track of what is primary and what is the, the main version. I think the same thing, um, with what we call workloads or serverless functions is just like there is a production version and there's like everything else. Um, and there by, by maintaining this bifurcation, this, um, you know, there's separation between what is being served, live traffic by your app and what is not.
I think you can get around those things, but I think. This is also one of the benefits of having engineers as the primary archetype now, right? Because these, these are the workflows that they're used to. They're used to, you know, c I C D, they're used to maintaining the production version and everything.
They, and they understand these like very traditional kit based workflows, um, staging, production, you know, master whatnot. So, yeah, like, I, I, I think, I think it, it, I think it's reducing it more into an engineering problem. Than a data science problem. It's like what I would say to that, which is the complexity that dude, I mean, think about how many times I just know because over the last three years it's been like so many engineers and even like X data scientists or some people even brand themselves as recovering data scientists, they talk about how painful it is to get some of their teammates just to learn gi.
And hopefully that isn't going to be the problem anymore. Hopefully, we're not going to have to figure these things out or push data scientists to do those things that they don't want to do because they're just gonna get eaten by the software engineering world. Yeah. I hope I, I may maybe not, I hope there's not the right thing, but I, I think it just reduces a lot of, I think the, it's actually a simple, it's a, it's a evolution that simplifies things a lot more.
Um, in that it's, you know, and like I, I think like, you know, a, a a, Abby, you mentioned this earlier as well, which is that, you know, you'll, you and I, and I said too, it's like, you know, we found ourselves to be more effective when we became engineers and had to learn that. It's like, I don't think it's a bad thing that, you know, you have, you know, really clean workload around this.
And I think by that, by the persona being kind of like squashed a bit. Into, Hey, you are a, you are also an engineer and your machine learning is one of the things, you know? Um, I think that is like a, it does make life a bit easier, um, holistically, at least. All right, man. I wanna switch gears and I have to ask, because I love the fact that you have some incredible people that have backed Base 10.
How did that happen? I mean, did they just see in you this shining star and they want him. To go deeper or what, like, uh, are you incredibly well connected or are you a great salesman or a little bit of everything? No, so I, I, I think like, at the end of the day, like, um, we, we've, we've just been around for a while and so we, we, we, we have meaningful connections and like, you know, some of the people we've raise money for, you know, we've known for, you know, honestly close to a decade, like Sarah Cy Grove from Greylock now conviction.
Um, Who, you know, has written a number of checks into us. Like we, we started, we, we met her when we were at our first company in 2015, and then she backed us in 2019. And so it was just like a lot of relationship building. I think though, like overall, like for us, like what we got lucky with was that we were a pretty technical team that w that could build products.
Um, you know, we were, um, we were working in a market that had a lot of oxygen. We were working in a market that had a lot of oxygen and yeah, of course. Like we just knew, we'd just been around long enough that we knew, um, a lot of great people who showed early conviction, um, in us. I don't think there's like, you know, anything particularly special about us like this.
This stuff's very, very difficult. Yeah, this stuff's very, very difficult and I think you just gotta get lucky at, you know, a number of different of. You know, junctions. And so I, I love the humility where he says, I've got no secret sauce. It's just timing. Wait, wait. It's just perfect timing. There's nothing else.
It's all, it's all, it's all exactly. That's the right place, right time. You are. I was at the right coffee shop at the right time, you know, ordering the right thing. Well, and you're working exactly. You're working on a hard problem. And the problem got very popular. Yeah. We worked on a hard pro. The problem got pop popular and I anything like, there was like a, you know, you guys, you guys like remember this like three years ago, two years ago?
Is that, you know, there was so many machine learning ops companies and so many of them and still are like, we're just so enterprise focused for here and we're just like, Hey, we're gonna sell the enterprise. We're gonna go top down, we're gonna sell to the cio. Um, I'd tell you that, you know, there was like a small set of companies.
That were focused on the end user and like, you know, building for the engineer or the data scientist and, you know, obvi obviously. Um, and like, I think those are, to me at least, they're the interesting companies and you know, we're like all these companies, not even talking about Base 10 right now are fantastic, right?
Like Hugging Bates is a great company. It building for the end user. Um, you know, replicating all those, um, guys with like Ben and um, like. Awesome people working on really interesting problems with a differentiated point of view. Um, like it's, but I think for us, like, you know, we were, we were thinking about machine learning from our product perspective, and we have been, and we continue to be, you know, we haven't really made, we still have a long way to go.
Obviously we're, we're just getting started. But, um, the like, it, it is, it is somewhat, uh, it was somewhat different at the time and you know, you see like, Um, now companies like that are definitely getting funded, right? Or making a lot of splash, like, you know, is doing so great right now. It's fantastic to see.
But again, it's focused on the engineer. It's focused on the, it's focused on using ML as an API almost. Yeah, yeah, yeah. Well, tell us about, I mean, I know you guys are hiring and who you looking for? Yeah. You're looking for machine learning engineers. Yeah, exactly. So we're looking for, you know, folks who could do ml.
We're looking for, um, honestly like. Machine learning info is, you know, this is why your podcast is so great and your audience is so great and your community is so fantastic, is that like, you know, this is a, you know, there's just not that many MLM for people out there who are really good. Um, and I think like that this is like such a massive opportunity.
That's why it's so hard for us, Hiam. So any MLM for people, like, you know, we will value you or you'll have a good time, you're working out problems. Um, we'd love to chat. Um, you know, but I think in general, We, we take a pretty, um, what's the right word? We look for more utility players than specialists, um, at Base 10.
And so, like, you know, if you like to build stuff, you like to like, you know, reach out to me or anyone else on the team, we'd love to chat. We're pretty open-minded, um, about who we bring on. Yeah. And we'll leave. A link to the job description and the hiring page and the description of this, uh, of this podcast in case anyone wants to check it out.
Also, maybe one quick thing I'll ask as a follow up on that, are you hiring like in a particular location as well, or is it across states? It's, it's, anyway, you know, it, it's the states. It's, you know, we, we have a team and we have people in Canada, we have people in Armenia. You know, we have people in the us um, like we're, we're pretty open on location, so.
Nice. Um, Yeah, we're, we're we, we, building remote teams is hard, but we're committed to it. Yeah. Well, dude, I got one last question for you, and hopefully this doesn't destroy our relationship, but first time we talked, you told me that what, you were born, you were born in India, or you were born in Australia?
I can't remember exactly. I was born, I was born in Australia. Oh, okay. Yeah. So then that destroys the question that I was gonna ask. I was gonna ask if you were, well, you should ask it. I'll ask it anyway. No, I was gonna ask you if you were the guy that, that movie was based on Lion. Lion, um, oh, he was born in India.
No, I, I I wish that that, that, that's a good story. No. Do I wish, I don't think, I wish. That's, uh, but. Good movie. How was a great movie? Good movie. Yeah. I was just thinking while I was talking to you, I'm like, ah, yeah, the Ozzie accent is coming through and so I had to ask. Anyway, this has been awesome, man. I appreciate you coming on here.
I appreciate you chopping it up with us and teaching us a few things. It's always a pleasure chatting with you, and I look forward to doing it more. Awesome. Thank you so much for the time, Abby and Demetrius.